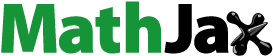
ABSTRACT
The reintroduction of cooperatives is part of the Georgian government’s new rural development policy, supported by several international donors’ interventions. This paper estimates the impact of small farmers’ participation in new collective actions. We employ farm-level data from 210 Georgian hazelnuts, grapes and honey farmers and use a treatment effects model that accounts for selection bias. We find a positive and significant impact of participation in new groups on farm revenue and net returns. The findings indicate that even though the cooperatives are still young, the government policies and donor interventions already bring some tangible economic benefits to small farmers.
1. Introduction
Small-scale farmers face various constraints, such as reliable access to inputs, relevant market information and low output prices, which hamper their ability to benefit from current market opportunities (Staal, Delgado, and Nicholson Citation1997; Alene et al. Citation2008). Poor infrastructure and remoteness often increase transaction costs (Barrett Citation2008; Key, Sadoulet, and De Janvry Citation2000). In addition, small farms with few assets often have limited access to services, including effective and reliable extension and rural credit, which are central to upgrading production systems (Wiggins, Kirsten, and Llambí Citation2010; Reardon et al. Citation2009). Addressing these failures requires institutionalising new innovative programmes and policies to improve the conditions of the small farm sector (Jayne, Mather, and Mghenyi Citation2010). Rural institutions, including farmer organisations, cooperatives, and other forms of collective action, have gained new popularity in the context of agri-food system transformation and are perceived as a panacea to the high transaction costs associated with small-scale agricultural production (Rao, Brümmer, and Qaim Citation2012; Markelova et al. Citation2009; FAO Citation2012a).
Many researchers consider the cooperation of farmers essential for communities to reduce poverty in rural areas and an important policy tool for developing countries to achieve agricultural and rural development (Abate Citation2018; Altman Citation2015; Wanyama, Develtere, and Pollet Citation2008). Available evidence suggests that the incidence of rural poverty in Georgia is higher (25.5%) compared to 17% in urban centres (The World Bank Citation2018). Interest in both – developing and developed countries in the cooperative movement has also grown recently, as cooperative organisations are expected to be more flexible than corporations, more responsive to local social and environmental challenges, and more resilient in times of economic crisis (Delbono and Reggiani Citation2013; Birchall and Hammond Ketilson Citation2009).
However, while some authors emphasise the positive aspects of cooperation, others document the drawbacks of collective actions, such as freeriding, low trust, higher regulatory costs associated with ownership rights and inefficiency of the lead agent (Nilsson Citation2001).
In the Eastern European region and the countries of the former Soviet Union, the concept of rural cooperation is still linked to the notion of the Soviet agricultural model, which left many farmers recalling the privatisation and restitution of farmland and private property (Hagedorn Citation2014; Lerman, Sedik, and Csaki Citation2016). This historical development affected agricultural structures with land-use fragmentation and complex land ownership (Hartvigsen Citation2014), leading to the inability of small farmers to increase their productive capacity and benefit from new opportunities in domestic and international markets (Lerman, Sedik, and Csaki Citation2016; Wolz, Möllers, and Mihai Micu Citation2019).
Nonetheless, in Georgia, like other Eastern European countries, the national governments and international donors (especially the EU, given its geopolitical strategy in the Eastern Partnership region) seek revival of the cooperative movement after the forced collectivisation and lost decades of dominance of the Soviet type of collective farms and their collapse in the 1990s. Recently, this call has also received massive support to create a favourable policy environment and direct support for new groups of farmers through several programmes and projects. However, in the region, only a few studies have analysed spontaneous or externally induced collective actions and their impact on members’ performance. For example, Michalek, Ciaian, and Pokrivcak (Citation2018) found that members of government-supported producer groups failed to show better performance, while members of producer groups that emerged spontaneously without government assistance exhibited better economic/income indicators than non-members. Bošková, Ahado, and Ratinger (Citation2020) also reported positive economic benefits of dairy farms in the Czech Republic. Despite these mixed findings, which can be ascribed to the different nature of producer groups and the analytical procedures used, evidence from post-Soviet countries is even scarcer, justifying further assessment.
This study contributes to the literature by examining the impact of supported collective actions and cooperation on the economic performance of small farmers in transition countries. In particular, the study sheds light on whether newly supported farmer groups from a country with a negative cooperative experience from socialist regimes can benefit its members economically. To the best of our knowledge, this research is the first to analyse the effects of new policies and the joint efforts of international donors and national government related to the re-establishment of farmer groups. Our study draws on a treatment effects model (Cong and Drukker Citation2000) to estimate the impact of membership in groups on the economic performance of small farmers while addressing selection bias arising from observed and unobserved factors.
The remainder of this paper is organised as follows. Section 2 presents the background of Georgian agriculture, the reality of small farmers and policy support for the establishment of the producer groups. In Section 3, we present the analytical framework and estimation strategy. The data and descriptive statistics of the variables used in the analysis are presented in Section 4, followed by the results (Section 5) and discussion in Section 6. Section 7 presents the paper’s conclusions and implications for current policies.
2. Background
2.1. The agriculture sector and farmers’ cooperation in Georgia
After the collapse of the Soviet Union and following the civil war and economic regress, Georgia is again a predominantly agricultural country, with almost half of the population living in rural areas (FAO Citation2012b). Arable land covers more than 3 million hectares (approximately 43%) of the country’s territory. A subtropical climate dominating a major part of the territory creates excellent conditions for producing a broad variety of subtropical crops (Bondyrev, Davitashvili, and Singh Citation2015; Ministry of Agriculture of Georgia Citation2015). However, since independence from the Soviet Union at the beginning of the 90’s, the economy suffered severe shocks, bringing productivity decrease by more than two-thirds (Millns Citation2013). The reduction in the sown area and the decline in overall agricultural production caused a dependency on imports, which exceeded one billion USD in 2013, and a decline in the self-sufficiency ratio of almost all types of products (Ministry of Agriculture of Georgia Citation2015). Agriculture employs more than 50% of the country’s labour force, but it contributes to total GDP by only 10%, lagging well behind other sectors of the economy.
Like other countries of the Commonwealth of Independent States (CIS) and Central and Eastern Europe states, Georgia has a long tradition of spontaneous farmers’ cooperatives from the 19th to the beginning of the 20th century. However, during the Soviet era, the original idea of bottom-up self-help small farmers’ associations dramatically changed from voluntary marketing, service, or credit cooperatives to state-controlled production collective farms (Lerman and Sedik Citation2014).
The break-up of the Soviet Union in the 1990s resulted in the collapse of a collectivised agricultural system when cooperatives of any type in Georgia almost ceased to exist ENPARD, (European Neighbourhood Programme for Agriculture and Rural Development) (Citation2015); Ministry of Agriculture of Georgia (Citation2014). As a result, several authors have argued (for example ENPARD, (European Neighbourhood Programme for Agriculture and Rural Development) Citation2015; Lampi Citation2012; Gardner and Lerman Citation2006) that until today cooperatives continue to be negatively associated with the Soviet-era collective farms and farmers, and the general public still feels distrust and a ‘mental block’ towards farm cooperatives. Gardner and Lerman (Citation2006) write: ‘The use of the word “co-operative” in Central and Eastern Europe will not only create the wrong impression, but it will also create barriers to progress’.
Nevertheless, traditional grassroots organisations and informal institutions developed spontaneously in Georgia before the socialist Soviet times, so strong social cohesion exists even today in parallel with formal institutions. For example, Lampi (Citation2012) analysed farmers’ cooperation and demonstrated that Georgian society typically consists of solid informal networks, such as the common herding Naghin system which has a long history in hilly pasture areas. An analysis of the bonding and bridging constructs of social capital in the Georgian environment is thoroughly provided, for example, in USAID Georgia (Citation2011) or Buschmann (Citation2008). Based on their findings, we can learn that Georgia has robust bonding ties, indicating close relationships among family, relatives, and friends. On the other hand, bridging capital representing willingness to cooperate with strangers is relatively low. It predetermines a tendency to rely on acquaintances in small businesses rather than to cooperate with members of the broader society in institutionalised ways. The first organised efforts to revive the cooperative sector and boost modern agricultural cooperatives in Georgia appeared in 2012 with the external assistance of international organisations and the donor community. The first organisations that supported horizontal integration of farmers include USAID, OPTO International, the Swiss Agency for Development and Cooperation (SDC), Denmark’s Development Cooperation (DANIDA), and the Czech Development Agency (CzDA). These organisations started programmes and individual projects focused on rural livelihood improvement by promoting producer groups (Millns Citation2013; FAO Citation2012a). Only a few modern functional cooperatives have been established spontaneously without donor support. The most recent programme for agricultural sector development with a cooperation component was the EU European Neighbourhood Programme for Agriculture and Rural Development (ENPARD), launched in 2013. It allocated 52 million EUR to support cooperatives’ formation, direct support of the national agricultural budget, technical assistance, and strengthening of national and regional state institutions (ENPARD, (European Neighbourhood Programme for Agriculture and Rural Development) Citation2015; Millns Citation2013; FAO Citation2012a).
The EU-Georgia Deep and Comprehensive Free Trade Area (DCFTA), recently established between the European Union and Georgia (also with Moldova and Ukraine), covers agricultural and food products. Bringing farmers together to market their produce collectively is perceived as a way to overcome some structural problems – particularly the weak position and capacity of the atomised farming sectors resulting from the abrupt privatisations of state enterprises in the 1990s (FAO Citation2012a). The horizontal integration of small farmers in the form of cooperatives recognised by the Georgian government took several necessary steps towards developing an enabling environment. The Ministry of Agriculture of Georgia adopted the Strategy for Agricultural Development for 2014–2020 which provided a basic framework for promoting the development of farmer group organisations. This strategy-initiated legislation created a campaign for awareness-raising, provision of specialised extension services, and facilitation of special incentive tools, including matching grant contributions and possibilities for tax exemptions (Ministry of Agriculture of Georgia Citation2014). In 2013, based on the recommendation of FAO and EU, the Georgian National Parliament adopted the Law of Georgia on Agricultural Cooperatives which, together with the older Law on Entrepreneurs, created a basic legal framework. At the same time, the Agency for Development of Agricultural Cooperatives (ADAC) was established (ENPARD, (European Neighbourhood Programme for Agriculture and Rural Development) Citation2015).
Joint efforts by international donors and the national government led to an unprecedented growth of agricultural cooperatives. In mid-2014, only 100 agricultural cooperatives registered with the ADAC; this number increased ten times in the following year (Misheladze Citation2015). However, an important challenge to the sound and sustainable rebirth of the agricultural cooperatives’ sector is the limited number of members per cooperative. The average membership decreased during the 2014–2015 period from 10 to 6. In addition, some authors (see, for example, Millns Citation2013) and development specialists observed that about half of members in most cooperatives are passive, registering mainly to fulfil the obligatory quotas on the number of members set by the government and donors. Key founding members tend to take over the management and control of cooperatives, leading to limited compliance with democratic principles, non-transparent decision-making and the creation of individual investor-owned firms masked as cooperatives (Lampi Citation2012; Murray and Giannakas Citation2007). Organisational problems, such as lack of management competencies and experience among the members of the board of directors or poorly developed governing principles, are also documented by Millns (Citation2013, Citation2006). FAO (Citation2012a) concluded that less than 20% of the cooperatives established by donor projects showed significant activity after those projects closed.
Newly established cooperatives dominate the traditionally strong agricultural sectors with export potential – apiculture (honey), viticulture (grapes and wine) and hazelnuts. However, most cooperatives still serve only short local value chains or reach exports through local intermediaries. They sell their products mostly directly in villages at the farm gate to local consumers and intermediaries or with regional markets in close cities to wholesalers and processing companies.
3. Analytical framework and estimation strategies
3.1. Cooperative membership decision
As a necessary first step of our analysis, we employed a random utility framework to model farm household decisions to participate in collective action. It is usually applied to analyse the adoption of innovation under conditions of uncertainty (Feder, Eugene Just, and Zilberman Citation1985). A farmer will decide to participate in cooperatives if the benefit from participation is greater than the benefits from non-participation. The utility gain from cooperation can be expressed as a function of observed covariates in a latent variable function such as:
where is an indicator of the latent cooperative membership,
is a vector of parameters to be estimated,
is an error term and
is a binary variable equal to 1 if a farmer is a member of the cooperative and equal to
for non-members.
is the vector of exogenous variables (farm and household characteristics) believed to influence participation in cooperatives (see ). The choice of these variables and expected causality are informed by previous empirical literature (Mojo, Fischer, and Degefa Citation2017; Abebaw and Haile Citation2013; Benin et al. Citation2012; Fischer and Qaim Citation2012).
Table 1. The average size of cooperatives.
Table 2. Descriptive statistics of variables used in the analysis.
Among our variables, we identify social capital as an essential precondition for participation. Social capital may be defined as the level of mutual trust among people (Coleman Citation1988), the capacity for cooperation, or the norms of reciprocity and networks that enable group members’ cooperation and collective action (Woolcock and Narayan Citation2000). Social capital is a vital nonmaterial stock that can minimise monitoring costs, improve cooperation and consequently productivity and economic or even environmental outcomes (Pretty et al. Citation2020; Mojo, Fischer, and Degefa Citation2015; Chloupkova, Lind Haase Svendsen, and Tinggaard Svendsen Citation2003). The concept of social capital is important in the context of group formation in Georgia and other Eastern European countries, given the past negative experiences of socialist regimes. Many authors (Lerman and Sedik Citation2014; Sommerville et al. Citation2011) identified the negative experience of farmers with Soviet collective farms as a substantial obstacle to the current development of cooperatives in Georgia. However, trust was emphasised as a critical element of farmers’ intentions and attitudes towards participating in collective actions in Central and Eastern European countries (Möllers et al. Citation2017). The authors further underscored that the success and sustainability of cooperation depend on interpersonal trust among people.
In this study, we approach social capital in the manner of Liang et al. (Citation2015) whose framework identifies various aspects of social capital – external dimension, relational dimension, and cognitive dimension – and examined the effect of these on members’ participation in collective activities in China’s Zhejiang province. The external dimension is operationalised in this study as ‘peer network’ (i.e. contact with neighbours in the catchment area), relational dimension as ‘trust’ (interpersonal trust among farmers in the area), and cognitive dimension as ‘perception’ (farmer beliefs that membership generates economic benefits). Among these predetermined characteristics of participation in cooperatives, it is essential to point out that farmers could receive more extension visits due to cooperative membership, so the number of extension visit is jointly determined with the decision to participate in a cooperative, thus making it potentially endogenous. We correct this potential endogeneity in our analysis using the two-stage control function approach proposed by Wooldridge (Citation2015). The first stage entails estimating the extension visits separately with an identification variable (here, the number of farm plots owned by the farmer) and other explanatory variables employed in the probit model (see in Appendix A for the first stage regression). This identification instrument should significantly influence extension visits and not directly affect membership in a cooperative. In the second stage analysis, we incorporated the extension visits variable together with their predicted residuals from the first stage into the estimation of the cooperative membership model.
To reiterate, the main objective of this study is to assess the economic impact (farm revenue and net returns) of collective actions on small farmers. Given that these outcome variables are a linear function of farm and household characteristics () conditional on cooperative membership (
) in EquationEquation (1)
(1)
(1) , our outcomes of interest can be expressed as:
where and
are parameters to be estimated,
is an error term and
is a vector of outcome variables. The performance indicators were acquired by asking farmers to provide information on the inputs and outputs of their production. Thus, farm revenue was measured as the value of crops/products per hectare valued at market prices. At the same time, net returns were operationalised as the value of crops/products per hectare valued at market prices minus variable cost. Recent studies have used similar economic indicators (e.g. Ma and Abdulai Citation2016; Michalek, Ciaian, and Pokrivcak Citation2018).
As farmers may self-select into cooperative membership, unobservable characteristics such as inherent abilities and motivation are likely to affect the decision to join cooperatives and the outcome variables under study. Thus, the error terms in EquationEquations (1)(1)
(1) and (Equation2
(2)
(2) ) may be correlated, resulting in potential endogeneity of the cooperative membership variable. To this end, using ordinary least squares (OLS) can generate bias and incorrect estimates. One commonly used impact evaluation technique to account for this selection bias is propensity score matching (PSM). Recent studies (Mojo, Fischer, and Degefa Citation2017; Fischer and Qaim Citation2012; Verhofstadt and Maertens Citation2014) have employed PSM to control observable attributes in observational studies.
However, the PSM technique does not account for selection bias due to unobserved characteristics. In this study, the treatment effects model (see below for more details) is preferred to the PSM technique because the former addresses the issue of selection bias due to observed and unobserved attributes (e.g. innate abilities, motivation of farmers and risk preference) with the inclusion of appropriate instrumental variables. Failing to account for unobservable factors would lead to biased and inconsistent estimates of the impact of cooperative membership. On the other hand, the potential limitation of the treatment effect model is cofounding with direct treatment effects because these effects cannot be estimated separately. In addition, the treatment effect model is applicable in the case of endogenous binary treatment on a continuous, fully observed outcome variable (Cong and Drukker Citation2000).
3.2. Treatment effects model
As indicated in the previous paragraph, the treatment effects model eliminates observable and unobservable bias in sample selection. It simultaneously estimates the cooperative membership model and outcome functions (Cong and Drukker Citation2000). The method also provides a direct marginal effect of participation in cooperatives on the outcome variables under study. Under the treatment effects model, the error terms in EquationEquations (1)(1)
(1) and (Equation2
(2)
(2) ) (i.e.
and
) are assumed to have a bivariate normal distribution with a zero mean and correlation, such that
. Selection bias due to unobservable factors arises if
is significantly different from zero (Ma and Abdulai Citation2017; Cong and Drukker Citation2000). In a situation where
is negative, this means a negative selection bias, suggesting that farmers that obtain lower farm revenues and net returns compared to the sample average have a higher probability of joining cooperatives (Ma and Abdulai Citation2017). The opposite is true for a positive selection bias.
Following the framework of Ma and Abdulai (Citation2017) and Cong and Drukker (Citation2000), the expected outcomes for the ith farmer conditional on participation and non-participation can be expressed respectively as:
where is the standard normal probability density function and
is the standard normal cumulative density function;
is the covariance term between the error term
in EquationEquation (1)
(1)
(1) and the error term
in EquationEquation (2)
(2)
(2) . The ratio of
and
refers to the inverse Mills.
and
are parameters to be estimated; and
is a vector of explanatory variables defined previously. The difference in expected farm revenue and net returns between the sample (N) of members and non-members (i.e. the difference between EquationEquations (3)
(3)
(3) and (Equation4
(4)
(4) ) known as the average treatment effect (ATE) can be estimated as:
The treatment effects model requires at least an instrument to improve identification in the selection equation that does not appear in the outcome functions. In particular, the instrument treats unobservable attributes that may bias the impact of cooperative membership on the outcomes of interests. The instrument is expected to influence cooperative membership but should not directly affect outcome variables. This study uses the distance to the nearest large market as an instrument. We expect that the farther the distance to large markets, the more likely farmers would be willing to participate in collective activities, given the potential role that cooperatives can play in facilitating farmers’ transportation and access to high-quality inputs, a place to sell their products, and market-related information (e.g. sales price). A similar instrument was used by Shiferaw et al. (Citation2014) in their study on the adoption of improved wheat varieties and their impact on household food security in Ethiopia. The validity of the instrument was verified through a probit model for the cooperative membership model and an OLS regression for the outcome variables. The result of the validity test shows that the instrument affects cooperative membership () in EquationEquation (1)
(1)
(1) but not significant in the farm revenue function
for members and non-members, respectively. Also, considering net returns as an outcome variable
for members and non-members, respectively.
4. Data and variables description
The data used in this study come from a cross-sectional household survey of hazelnuts, grapes and honey farmers conducted in September and October 2018 in the Western and Eastern regions of Georgia. These regions were selected specifically due to their high intensity of newly emerged cooperatives and share in national agricultural production (Kochlamazashvili, Zhorzholiani, and Kakulia Citation2017), as well as shared similarities (e.g. assets received from ENPARD 1, short marketing channels and negotiation position of farmers vis-à-vis buyers) in the value chain organisation.
The sample for the study was drawn using a multi-stage cluster sampling procedure complemented by structured questionnaire administration. Data on registered cooperatives (i.e. 91 cooperatives established between 2013 and 2014 which received material and non-material support from the ENPARD programme) provided by the International School of Economics in Tbilisi (ISET), Georgia, were pre-selected to draw the sample of cooperative members from the two regions. We contacted 37 of the 91 cooperatives based on their target products and coverage of the country’s agroecological zones. shows that the average size of the sampled cooperatives is similar to the average size of all 91 cooperatives indicating that it is a representative subset. In each of the 37 sampled groups, around three members were randomly selected, resulting in 93 members (35 honey producers, 30 hazelnut producers and 28 grape producers). Non-members were randomly chosen in consultation with local cooperative support organisations within the same regions as the cooperative members, reaching a total of 117 non-cooperative members (30 honey producers, 33 hazelnuts producers and 54 grape producers). In addition to the household survey, key informant interviews were conducted with cooperative managers to examine the context of the three sectors’ value chains (from suppliers to the local market) to gain a deeper understanding of the interpretation of the results. The farmers interviewed are small producers of grapes, hazelnuts and honey, mainly engaged in production and marketing. presents descriptive statistics of variables used in the analysis. It reports the binary treatment variable, the outcome variables of interest, the explanatory variables, the control variables and the instrumental variable. It shows systematic differences between cooperative members and non-members, signifying the existence of potential self-selection bias.
5. Results
5.1. Characteristics of cooperatives within their respective value chains
For the interpretation of the economic performance of the new collective actions and cooperation among small Georgian farmers, it is first necessary to understand the context, institutional arrangement of groups and shared characteristics of the three analysed value chains. shows the general descriptive statistics of cooperatives within the value chains of the three sectors after the interviews with group managers. We can see that 47% of apiculture cooperative members and 67% of hazelnut members sell their products through their cooperatives. Meanwhile, only about 30% of viticulture producers sell only through cooperatives, and 50% use both – group and individual marketing channels.
Table 3. Descriptive statistics of cooperatives within their respective value chains.
Regarding openness of the groups and expanding the membership base and the business transactions, apiculture and viticulture cooperatives (53.3% and 60% correspondingly) expressed interest in increasing their total membership relative to 25% of hazelnut producers. We used this indicator as another proxy for the perceived success of the groups. All cooperatives sell their products to local consumers, with only 20% of the viticulture sector selling to restaurants and local consumers and 33.3% of hazelnut producers selling to traders from the capital city. Apart from the material assets provided under the ENPARD programme to the respective cooperatives, each sector received more than 20% (of the total budget of the ENPARD 1 programme) subsidy for investment as a form of support from the programme.
5.2. Choice of cooperative membership and farm economic performance
The estimates of the determinants of participation in cooperatives and the economic impact of cooperation on performance indicators are presented in . The estimation results show that the residual coefficient of the potential endogenous variable (extension visits) predicted from the first stage probit regression is statistically insignificant, suggesting that extension visits are not endogenously determined in farmers’ decision to participate in cooperatives. We find that participation in cooperatives is positively and significantly influenced by farmers’ educational status, household size, distance to market and visits by extension agents. Accordingly, peer networks and trust also positively affect participation in cooperatives.
Table 4. Impact of cooperative membership on farm revenue (in USD).
Table 5. Impact of cooperative membership on net returns (in USD).
Concerning the impact of participation on members’ economic performance, the results show that the coefficients of are significantly different from zero, which suggests the presence of sample selectivity bias arising from unobservable characteristics. The negative sign of
indicates that farmers who obtain lower farm revenues and net returns than the sample average have a higher probability of joining the cooperatives. Moreover, the null hypothesis for
is rejected at the 5% and 1% levels, suggesting a correlation between the cooperative membership model and the outcome variables.
The results of the treatment effects model in the third column in show that participation in cooperatives has a positive and statistically significant impact on farm revenue and net returns with marginal effects of 5,189.152 USD and 4,399.009 USD, respectively. Concerning the average values of the entire sample (), these marginal effects reflect an increase in farm revenue of 86.6 % and net returns of 79.2%. The ATE estimates from the PSM estimation approach in the lower part of show that the ATE values are lower than the values calculated by the treatment effects model. This finding suggests that unobservable attributes affect the choice of participation and performance indicators, resulting in negative selection bias leading to an underestimated ATE in the PSM estimates.
The results further show that education tends to have a negative and significant impact on both farm revenue and net returns. Similarly, peer networks and the perception of the benefits from cooperation negatively affect farm revenue and net returns.
6. Discussion
As the first step in our analysis, we conducted a qualitative assessment with the leaders/managers of cooperatives and other stakeholders in the value chain of all three sectors. We observed that all three sectors share similar conditions and potential for product realisation. The production is typically dominated by atomised small farmers at the mercy of intermediaries who come and collect the farm products. Traditional farm gate sales and local traders dominate as available marketing channels. Even though all three products are demanded on international markets and have export potential, the lack of new marketing channels and low prices given by intermediaries were indicated as dominant obstacles by most interviewed farmers.
Most of the cooperatives covered by our research indicated a willingness to expand their operations and membership. Only the cooperatives in the hazelnut sector, facing the challenges of pest infestation during our data collection, were more hesitant. The other two sectors perceive that the new members have the potential to enlarge their business activities, decrease competition, and improve market access, thus increase the economies of scale and decrease transaction costs.
The empirical results indicate that participation in a cooperative has a positive and statistically significant impact on farm revenue and net returns. The positive impact of cooperatives on farm revenue and net returns is in line with previous empirical studies (Bachke Citation2019; Verhofstadt and Maertens Citation2014; Ma and Abdulai Citation2016) in Mozambique, China and Rwanda, respectively. This study is able to estimate impact by using a treatment effects model which addresses selection bias due to observed and unobserved attributes (e.g. innate abilities, motivation of farmers and risk preference) with the inclusion of appropriate instrumental variables.
The results further indicate that peer networks and the perceived benefits from cooperatives negatively affect farm revenue and net returns. One possible reason for the negative effect of peer networks on farm revenue and net returns is that contacts with neighbours may not exclusively involve information exchange relating to agriculture. Also, the assimilation and implementation rate of the different information from peers largely depends on the individual farmer’s inherent ability. It does not always tend to influence information application and transformation into farm productivity. The inverse relationship between the perceived benefits of cooperatives and farm outcomes suggests that low-performing farmers tend to have higher expectations from the cooperatives to improve their situation. It may lead to relatively passive farmers, because such perceptions tend to influence their attitudes and farm decisions and thus affect farm productivity. In addition, the insignificant effect of the crop/product cultivated suggests that the benefits from cooperative membership and the willingness to join differ, while the crop/product type does not exclusively determine them. From a long-run perspective, it can be inferred that crop selection is not endogenous with cooperative membership. However, the causal direction is not straightforward for these variables.
Regarding the effect of the control variables on farm productivity, only education and the farmer’s age influenced the outcomes of interests. Farmers in our sample reached mean years in formal education of 13.07, indicating relatively well-educated farmers. A high share of them has even finished university education. However, after the collapse of the Georgian economy in the 1990s, many formerly university-educated positions in cities ceased to exist. People had to return to rural areas and make their living from subsistence agriculture. Therefore, the higher education of such farmers does not necessarily mean education in agriculture and thus better know-how for efficient cultivation compared to the less educated farmers by profession. This finding is in line with Gedara et al. (Citation2012). The authors argued that education is not an ideal index for human capital as the general tutelage provided in schools may not directly apply to the cultivation of specific crops or agricultural training. The positive effect of age (a proxy of farm management experience) suggests that more experienced farmers can improve their decision-making and resilience in choosing farm techniques, resulting in higher farm revenue and net returns.
Turning to the results of the participation model, we included the farmer’s age, gender, education, location, household size and asset ownership as control variables. We found that farmers’ educational status positively and significantly influences cooperative membership (column one in ). The positive effect of education is consistent, for instance, with the findings of Chagwiza, Muradian, and Ruben (Citation2016). As Abate, Nicola Francesconi, and Getnet (Citation2014) and Mojo, Fischer, and Degefa (Citation2017) have already confirmed, the tendency to become a cooperative member also increases with larger household size. Social capital and the openness of the farmer to advice from others (peer network), operationalised as the number of contacts with extension officers and other farmers (external dimension of the social capital), also have a positive influence, as already reported in other recent empirical studies (Mojo, Fischer, and Degefa Citation2017; Abdul-Rahaman and Abdulai Citation2018). However, our conclusions of causality seem weaker here since many new cooperatives received more training and extension support from the donors only after they established their groups.
Besides personal, household, and social network characteristics, farmers that hold optimistic beliefs about benefits derived from the membership (their cognitive dimension of the social capital), as well as trust other farmers in the area, also tend to sacrifice their independence and autonomous decision about their farms and join the collective activities. This is consistent with, for instance, the findings of Liang et al. (Citation2015) and Möllers et al. (Citation2017) in modelling cooperation in China and Romania. On the other hand, other characteristics of the farming business like ownership of assets, crop or product cultivated, farm size and age of the farmer do not seem to be related to the propensity to join new cooperatives.
Emerging economic advantages of horizontal integration within the value chains can be observed, even though the groups and total area under the cultivation of their members are still relatively small. Therefore, it can be assumed that the benefits of each group’s potential economies of scale of larger land cultivation remain limited. The measured positive impact on members’ productivity may be due to the support provided by the ENPARD programme which allows for better processing and storage, leading to higher product quality and improved marketing. This effect might be more substantial than producer groups’ better bargaining power through higher production volumes. The economic benefits derived from economies of scale are also frequently limited by the small number of farmers committed to selling through the cooperative. Despite benefiting from new machinery and new knowledge provided from training, many members still fail to deliver their products through the cooperative, and they reach their selling agreements. This form of ‘free-riding’ limits the overall potential of new groups. It also creates the danger of dissolving groups in the near future when the group managers cannot cover the running costs of these new rural businesses.
Our positive findings do not reflect the fact that many newly created cooperatives had already failed to survive the end of external support. The ENPARD final evaluation (Kochlamazashvili, Zhorzholiani, and Kakulia Citation2017) reported that up to 50% of new cooperatives failed to develop into efficient producers’ groups.
Developing internal rules, increasing members’ commitment, and enlarging the membership base are now necessary to increase economic benefits resulting from higher volumes and the efficient use of technology. Even though our data indicate a positive opinion, the genuine willingness of founders to open the group to new members may be limited. This reluctance is exacerbated by the fact that many cooperatives are based on closed family units. Family bonds might be necessary to reduce initial distrust and communication costs; however, the created bonding capital will be difficult to turn into bridging social capital during opening groups to non-family members. This study’s limited sample size and national representativeness merit future research with a larger dataset to verify and extend the empirical findings in different farming contexts.
7. Conclusions
This paper assessed the impact of supported collective action on the economic performance of farmers in Georgia using farm revenue and net returns as main economic indicators. We used the treatment effects model to assess the impact of small farmers’ participation in collective activities while accounting for potential selection bias emanating from observable and unobservable characteristics. We identified a negative selection bias, suggesting that farmers with lower farm revenues and net returns than the sample average have a higher probability of joining a cooperative. This is reasonable as participation in a cooperative organisation and other forms of collective action is expected to enhance the productivity of small farmers.
The empirical results revealed that participation in cooperatives is positively and significantly associated with higher farm revenue and net returns. Regarding the factors affecting participation in cooperatives, the results show that education, household size, number of contacts with extension officers and other farmers, and trust significantly influence farmers’ decisions to join cooperatives.
Our results provide positive information about the effectiveness of the new cooperation of small farmers in producer groups established by Georgia’s focus on producers’ groups, supported by the external assistance of the EU ENPARD programme, and facilitated on the ground by material and training support by a consortium of development NGOs. Our research and the reports on the increasing share of production traded by cooperatives, albeit still quite low, indicate that there are economic benefits of membership in producer groups. The new cooperatives generally demonstrate initial tangible success, which may start a process of changing minds in favour of more entrepreneurship, but these young groups must first overcome the impediments to their membership. Establishing mechanisms to address farmer attitudes, such as freeriding and low member commitment in farmer groups, is a policy opportunity; attitudes undermine the sustainability and expected effects of social capital formation. Cooperative rural institutions can be promoted by disseminating the estimated benefits of collective action to other farmers and can be enhanced by improved infrastructure for extension services which we found to encourage farmers’ decision to join cooperatives. Even small farmers, particularly in remote areas, can benefit from cooperatives and other forms of collective action by relaxing the constraints associated with information and transaction costs and by improving their access to larger market outlets.
Acknowledgements
The authors gratefully acknowledge the helpful comments from the anonymous reviewers and the journal editor on previous drafts.
Disclosure statement
No potential conflict of interest was reported by the author(s).
Correction Statement
This article has been corrected with minor changes. These changes do not impact the academic content of the article.
Additional information
Funding
Notes on contributors
Samuel Ahado
Samuel Ahado, Ph.D. holds a PhD in Sustainable Rural Development from the Faculty of Tropical AgriSciences (FTA) of the Czech University of Life Sciences (CZU). His research interests are in applied economics, focusing on agricultural economics and policy analysis, particularly in rural institutions, economic development and food industry competitiveness. He is a member of the Cooperative Research Group of FTA/CZU.
Jiri Hejkrlik
Jiri Hejkrlik, Ph.D. graduated with a doctorate from the Czech University of Life Sciences Prague (CZU) and is currently serving as Vice-dean at the Faculty of Tropical AgriSciences (FTA). He is an active researcher in the Department of Economics and Development, where he focuses on agricultural trade, market access for small farmers, rural institutions and small farmers’ cooperatives in developing countries. He has also been the manager and evaluator of international agricultural projects in Eastern Europe (Georgia, Moldova, Bosnia and Herzegovina, and Ukraine), Central Asia (Mongolia, Uzbekistan and Kazakhstan) and Africa (Angola, Ethiopia and Kenya). He is a member of the Cooperative Research Group of FTA/CZU.
Tomas Ratinger
Tomas Ratinger, Ph.D. is an associate professor at the Department of Economics of FTA CZU and senior economist in the Technology Centre CAS. Before, he was a researcher and head of the department at the Institute of Agricultural Economics and Information in Prague. Between 4/2005 and 1/2009 he was a leader of the research group for sustainable agriculture and rural development (SUSTAG) JRC/IPTS v Seville, Spain. In 2000/2001 Tomáš Ratinger was an academic visitor at the Imperial College (London, Wye) and in the period 1996 – 1997, he worked for FAO. The main research interests of Tomas Ratinger are socioeconomic modelling and policy analysis, particularly in the area of economic development, investment activity, food industry competitiveness and provision of public goods from agriculture and forestry. Currently, he carries out the science-based evaluation and impact assessment of economic, and rural development, and of R&D&I policies at national and international levels.
Tamila Arnania Kepuladze
Tamila Arnania-Kepuladze, Ph.D. is a professor of the Department of Economics at the Akaki Tsereteli State University (Kutaisi, Georgia). She graduated from Rostov State University (Russia) in the field of Economics, received the degree of Candidate of Economics sciences in Ivane Javakhishvili Tbilisi State University (Georgia) in the field of Labour Economics, and then PhD degree in Tomas Bata University in Zlin (Czech Republic) in field Economics and Management. Prof. T. Arnania-Kepuladze is the author of over 100 publications, including 2 monographs and 5 textbooks. The main interest sphere of T. Arnania-Kepuladze includes labour market, institutions, gender, and poverty.
References
- Abate, G. T. 2018. “Drivers of Agricultural Cooperative Formation and Farmers’ Membership and Patronage Decisions in Ethiopia.” Journal of Cooperative Organisation and Management 6 (2): 53–63. doi:https://doi.org/10.1016/j.jcom.2018.06.002.
- Abate, G. T., G. N. Nicola Francesconi, and K. Getnet. 2014. “Impact of Agricultural Cooperatives on Smallholders’ Technical Efficiency: Empirical Evidence from Ethiopia.” Annals of Public and Cooperative Economics 85 (2): 257–286. doi:https://doi.org/10.1111/apce.12035.
- Abdul-Rahaman, A., and A. Abdulai. 2018. “Do Farmer Groups Impact on Farm Yield and Efficiency of Smallholder Farmers? Evidence from Rice Farmers in Northern Ghana.” Food Policy 81: 95–105. doi:10.1016/j.foodpol.2018.10.007.
- Abebaw, D., and M. G. Haile. 2013. “The Impact of Cooperatives on Agricultural Technology Adoption: Empirical Evidence from Ethiopia.” Food Policy 38 (2013): 82–91. doi:https://doi.org/10.1016/j.foodpol.2012.10.003.
- Alene, A. D., V. M. Manyong, G. Omanya, H. D. Mignouna, M. Bokanga, and G. Odhiambo. 2008. “Smallholder Market Participation Under Transactions Costs: Maize Supply and Fertilizer Demand in Kenya.” Food Policy 33 (4): 318–328. doi:https://doi.org/10.1016/j.foodpol.2007.12.001.
- Altman, M. 2015. “Cooperative Organizations as an Engine of Equitable Rural Economic Development.” Journal of Co-Operative Organization and Management 3 (1): 14–23. doi:10.1016/j.jcom.2015.02.001.
- Bachke, M. E. 2019. “Do Farmers’ Organizations Enhance the Welfare of Smallholders? Findings from the Mozambican National Agricultural Survey.” Food Policy 89 (November): 1–14. doi:10.1016/j.foodpol.2019.101792.
- Barrett, C. B. 2008. “Smallholder Market Participation: Concepts and Evidence from Eastern and Southern Africa.” Food Policy 33 (4): 299–317. doi:https://doi.org/10.1016/j.foodpol.2007.10.005.
- Benin, S., E. Nkonya, G. Okecho, J. Randriamamonjy, E. Kato, G. Lubade, and M. Kyotalimye. 2012. “Impact of The National Agricultural Advisory Services (Naads) Programme of Uganda: Considering Different Levels of Likely Contamination with the Treatment.” American Journal of Agricultural Economics 94 (2): 386–392. doi:10.1093/ajae/aar094.
- Birchall, J., and L. Hammond Ketilson. 2009. Resilience of the Cooperative Business Model in Times of Crisis, 1–41. Geneva Switzerland: International Labour Organization.
- Bondyrev, I., Z. Davitashvili, and V. P. Singh. 2015. The Geography of Georgia: Problems and Perspectives. Geneva, Switzerland: Springer.
- Bošková, I., S. Ahado, and T. Ratinger. 2020. “The Effects of the Participation in Producer Organisations on the Performance of Dairy Farmers in the Czech Republic and Future Challenges.” Agricultural Economics (Zemědělská ekonomika) 66 (8): 345–354. doi:10.17221/2/2020-agricecon.
- Buschmann, A. 2008. Headache and Risk-Aversion: Why Peasants in Georgia are Reluctant to Cooperate Formally? Or the Role of “Cooperation” in the Agriculture of Georgia. MSc. Tbilisi, Georgia: Javakhishvili Tbilisi State University.
- Chagwiza, C., R. Muradian, and R. Ruben. 2016. “Cooperative Membership and Dairy Performance Among Smallholders in Ethiopia.” Food Policy 59: 165–173. doi:10.1016/j.foodpol.2016.01.008.
- Chloupkova, J., G. Lind Haase Svendsen, and G. T. Tinggaard Svendsen. 2003. “Building and Destroying Social Capital: The Case of Cooperative Movements in Denmark and Poland.” Agriculture and human values 20 (3): 241–252. doi:10.1023/A:1026141807305.
- Coleman, J. S. 1988. Social Capital in the Creation of Human Capital. Chicago: The University of Chicago Press.
- Delbono, F., and C. Reggiani. 2013. “Cooperative Firms and the Crisis: Evidence from Some Italian Mixed Oligopolies.” Annals of Public and Cooperative Economics 84 (4): 383–397. doi:10.1111/apce.12020.
- FAO. 2012b. ”Assessment of the Agriculture and Rural Development Sectors in the Eastern Partnership Countries. FAO Regional Office for Europe and Central Asia (REU), Hungary.“ Vols. 1-63, 1–63. Budapest, Hungary: Food and Agriculture Organization of the United Nations.
- Feder, G., R. E. Eugene Just, and D. Zilberman. 1985. “Adoption of Agricultural Innovations in Developing Countries: A Survey.” Economic development and cultural change 33 (2): 255–298. doi:https://doi.org/10.1086/451461.
- Fischer, E., and M. Qaim. 2012. “Linking Smallholders to Markets: Determinants and Impacts of Farmer Collective Action in Kenya.” World Development 40 (6): 1255–1268. doi:10.1016/j.worlddev.2011.11.018.
- Gardner, B., and Z. Lerman. 2006. “Agricultural Cooperative Enterprise in the Transition from Socialist Collective Farming.” Journal of Rural Cooperation 34 (1): 1–18. doi:10.22004/ag.econ.44674.
- Gedara, K. M., C. Wilson, S. Pascoe, and T. Robinson. 2012. “Factors Affecting Technical Efficiency of Rice Farmers in Village Reservoir Irrigation Systems of Sri Lanka.” Journal of Agricultural Economics 63 (3): 627–638. doi:https://doi.org/10.1111/j.1477-9552.2012.00343.x.
- Hagedorn, K. 2014. “Post-Socialist Farmers’ Cooperatives in Central and Eastern Europe.” Annals of Public and Cooperative Economics 85 (4): 555–577. doi:10.1111/apce.12051.
- Hartvigsen, M. 2014. “Land Reform and Land Fragmentation in Central and Eastern Europe.” Land Use Policy 36: 330–341. doi:10.1016/j.landusepol.2013.08.016.
- Irakli (Rati) Kochlamazashvili, Daviti Zhorzholiani, and Nino Kakulia, eds. 2015. “ENPARD, (European Neighbourhood Programme for Agriculture and Rural Development). ”Supporting Agriculture Empowering Farmers Improving Lives,“ Agriculture and rural development are important priority areas for the EU in Georgia. These are supported through ENPARD, Vol. 8. 1–8. Tbilisi: ENPARD Publications.
- Jayne, T. S., D. Mather, and E. Mghenyi. 2010. “Principal Challenges Confronting Smallholder Agriculture in Sub-Saharan Africa.” World Development 38 (10): 1384–1398. doi:https://doi.org/10.1016/j.worlddev.2010.06.002.
- Key, N., E. Sadoulet, and A. D. De Janvry. 2000. “Transactions Costs and Agricultural Household Supply Response.” American Journal of Agricultural Economics 82 (2): 245–259. doi:https://doi.org/10.1111/0002-9092.00022.
- Kochlamazashvili, I. R., D. Zhorzholiani, and N. Kakulia. 2017. “EU-Supported Agricultural Cooperatives: A Case of Georgia.” In The European Union for Georgia ENPARD, 1–62. Tbilisi, Georgia: International School of Economics at TSU Policy Institute.
- Lampi, M. 2012. ”Farmers’ Cooperation in Georgia.“ Agriculture Sector Bulletin, In edited by FAO and Ministry of Agriculture, Government of Georgia, Vol. 27, 1–28. Rome: Food and Agriculture Organization Publications.
- Lerman, Z., and D. Sedik. 2014. Cooperatives in CIS and Georgia: Overview of Legislation, 1–26. doi:10.22004/ag.econ.290024.
- Lerman, Z., D. Sedik, and C. Csaki. 2016. Agricultural Cooperatives in Transition Countries. Saarbrucken, Germany: Lambert Academic Publishing (LAP).
- Liang, Q., Z. Huang, L. Haiyang, and X. Wang. 2015. “Social Capital, Member Participation, and Cooperative Performance: Evidence from China’s Zhejiang.” International Food and Agribusiness Management Review 18 (1): 49–78. doi:10.22004/ag.econ.197768.
- Ma, W., and A. Abdulai. 2016. “Does Cooperative Membership Improve Household Welfare? Evidence from Apple Farmers in China.” Food Policy 58: 94–102. doi:10.1016/j.foodpol.2015.12.002.
- Ma, W., and A. Abdulai. 2017. “The Economic Impacts of Agricultural Cooperatives on Smallholder Farmers in Rural China.” Agribusiness 33 (4): 537–551. doi:https://doi.org/10.1002/agr.21522.
- Markelova, H., R. Meinzen-Dick, J. Hellin, and S. Dohrn. 2009. “Collective Action for Smallholder Market Access.” Food Policy 34 (1): 1–7. doi:10.1016/j.foodpol.2008.10.001.
- Michalek, J., P. Ciaian, and J. Pokrivcak. 2018. “The Impact of Producer Organizations on Farm Performance: The Case Study of Large Farms from Slovakia.” Food Policy 75: 80–92. doi:10.1016/j.foodpol.2017.12.009.
- Millns, J. 2006. ”Promoting Farmer Entrepreneurship Through Producer Organisations in Central and Eastern Europe.” In edited by Janos Juhasz, Vol. 32, 1–59. Rome: Food and Agricultural Organization.
- Millns, J. 2013. ”Agriculture and Rural Cooperation Examples from Armenia, Georgia and Moldova.“ Policy Studies on Rural Transition No. 2013-2, In edited by John Millns, Vol. 37, 1–37. Republic of Moldova: Food and Agriculture Organisation of the United Nations (FAO)
- Ministry of Agriculture of Georgia. 2014. ”Strategy for Agricultural Development in Georgia, 2014-2020.” Vol. 30, 1–37. Tbilisi:Ministry of Agriculture for Georgia publications.
- Ministry of Agriculture of Georgia. 2015. ”Strategy for Agricultural Development in Georgia, 2015-2020.” Vol. 38, 1–38. Tbilisi: Ministry of Agriculture of Georgia publications.
- Misheladze, G. 2015. Presentation of Agency for Development of Agricultural Cooperatives. ATSU University in Kutaisi.
- Mojo, D., C. Fischer, and T. Degefa. 2015. “Social and Environmental Impacts of Agricultural Cooperatives: Evidence from Ethiopia.” International Journal of Sustainable Development & World Ecology 22: 1–13. doi:10.1080/13504509.2015.1052860.
- Mojo, D., C. Fischer, and T. Degefa. 2017. “The Determinants and Economic Impacts of Membership in Coffee Farmer Cooperatives: Recent Evidence from Rural Ethiopia.” Journal of Rural Studies 50: 84–94. doi:10.1016/j.jrurstud.2016.12.010.
- Möllers, J., D. Traikova, B. Ana-Maria Bîrhală, and A. Wolz. 2017. “Why (Not) Cooperate? A Cognitive Model of Farmers’ Intention to Join Producer Groups in Romania.” Post-Communist Economies 30 (1): 56–77. doi:http://doi.org/10.1080/14631377.2017.1361697.
- Murray, F., and K. Giannakas. 2007. “Agency and Leadership in Cooperatives: Endogenizing Organizational Commitment.” In Vertical Markets and Cooperative Hierarchies. The Role of Cooperatives in the Agri-Food Industry, edited by K. Karantininis and J. Nilsson, 93–113. Dordrecht: Springer.
- Nilsson, J. 2001. “Organisational Principles for Cooperative Firms.” Scandinavia Journal of Management 17 (3): 329–356. doi:10.1016/S0956-5221(01)00010-0.
- Pretty, J., S. Attwood, R. Bawden, H. van den Berg, Z. P. Bharucha, J. Dixon, C. B. Butler Flora, et al. 2020. ”Assessment of the Growth in Social Groups for Sustainable Agriculture and Land Management.” Global Sustainability 3 (e23): 1–16. doi:https://doi.org/10.1017/sus.2020.19.
- Rao, E., B. Brümmer, and M. Qaim. 2012. “Farmer Participation in Supermarket Channels, Production Technology, and Efficiency: The Case of Vegetables in Kenya.” American Journal of Agricultural Economics 94 (4): 891–912. doi:10.1093/ajae/aas024.
- Reardon, T., C. B. Brendan Barrett, J. A. Antonio Berdegué, and J. Swinnen. 2009. “Agrifood Industry Transformation and Small Farmers in Developing Countries.” World Development 37 (11): 1717–1727. doi:https://doi.org/10.1016/j.worlddev.2008.08.023.
- Cong, R., and D. M. Drukker. 2000. “Sg141-Treatment Effects Model.” Stata Technical Bulletin 10 (55): 25.
- Shiferaw, B., M. Kassie, M. Jaleta, and C. Yirga. 2014. “Adoption of Improved Wheat Varieties and Impacts on Household Food Security in Ethiopia.” Food Policy 44: 272–284. doi:10.1016/j.foodpol.2013.09.012.
- Sommerville, P., R. E. Lee, R. Shavgulidze, and I. Kvitaishvili.2011.Analytical Foundations Assessment – Agriculture (Rural Productivity), 1–161.Georgia.
- Staal, S., C. Delgado, and C. Nicholson. 1997. “Smallholder Dairying Under Transactions Costs in East Africa.” World Development 25 (5): 779–794. doi:https://doi.org/10.1016/S0305-750X(96)00138-6.
- Susan Beccio, ed. 2012a. FAO. “Agricultural Cooperatives: Paving the Way for Food Security and Rural Development.” In 2012 International Year of Cooperatives, 1–2. Rome, Italy: Food and Agriculture Organisation of the United Nations.
- USAID Georgia. 2011. ”Social Capital in Georgia: Final Report and Recommendations.” In edited by Hans Gutbrod, 1–44. Washington, D.C: USAID Publications.
- Verhofstadt, E., and M. Maertens. 2014. “Smallholder Cooperatives and Agricultural Performance in Rwanda: Do Organisational Differences Matter?” Agricultural Economics 45 (S1): 39–52. doi:10.1111/agec.12128.
- Wanyama, F. O., P. Develtere, and I. Pollet. 2008. “Encountering the Evidence: Cooperatives and Poverty Reduction in Africa.” Accesed 15 September 2020. http://www.cooperatiefondernemen.be/count/click.php?id=3
- Wiggins, S., J. Kirsten, and L. Llambí. 2010. “The Future of Small Farms.” World Development 38 (10): 1341–1348. doi:10.1016/j.worlddev.2009.06.013.
- Wolz, A., J. Möllers, and M. Mihai Micu. 2019. “Options for Agricultural Service Cooperatives in a Postsocialist Economy: Evidence from Romania.” Outlook on agriculture 49 (1): 57–65. doi:10.1177/0030727019861973.
- Woolcock, M., and D. Narayan. 2000. “Social Capital: Implications for Development Theory, Research, and Policy.” In The World Bank Research Observer, edited by T. W. Bank, 225–249. Harvard University USA: The World Bank.
- Wooldridge, J. M. 2015. “Control Function Methods in Applied Econometrics.” The Journal of Human Resources 50 (2): 420–445. doi:https://doi.org/10.3368/jhr.50.2.420.
- World Bank Group. 2018. Georgia from Reformer to Performer. A Systematic Country Diagnostics. World of Banking. https://openknowledge.worldbank.org/handle/10986/29790
Appendix A
Table A1. Addressing potential endogeneity in extension variable: First-stage regressions to predict membership and extension visits.