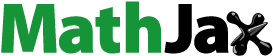
ABSTRACT
Extreme storms are becoming more frequent and intense with climate change. Assessing lake ecosystem responses to extreme storms (resistance) and their capacity to recover (resilience) is critical for predicting the future of lake ecosystems in a stormier world. Here we provide a systematic, standardized, and quantitative approach for identifying critical processes shaping lake ecosystem resistance following extreme storms. We identified 576 extreme wind storms for 8 lakes in Europe and North America. We calculated the resistance and resilience of each lake’s surface water temperature and oxygen saturation following each storm. Sharp decreases and increases in epilimnetic temperature and oxygen saturation caused by extreme storms resulted in unpredictable changes in lake resilience values across lakes, with a tendency not to return to pre-storm conditions. Resistance was primarily shaped by mean annual chlorophyll a concentration and its overall relationship with other physiochemical lake and storm characteristics. We modeled variation in resistance as a function of both lake and storm conditions, and the results suggested that eutrophic lakes were consistently less resistant to extreme storms compared to oligotrophic lakes. The lakes tended to be most resistant to extreme storms when antecedent surface waters were warm and oxygen saturated, but overall resistance was highest in lakes with low mean annual concentrations of chlorophyll a and total phosphorus. Our findings suggest physiochemical responses of lakes to meteorological forcing are shaped by ecological and/or physical feedback and processes that determine trophic state, such as the influence of differences in nutrient availability and algal growth.
Introduction
Lakes and their associated riverine and terrestrial ecosystems provide many socioecological services via clean drinking water and aesthetic/recreational value as well as supporting a wide array of biodiverse habitats. Such services are dependent on and influenced by the capacity of lakes to be resistant and resilient in the face of changing environmental conditions. Resistance is the ability of varying lakes to absorb stress while resilience indicates the ability of ecosystems to recover from, or adapt to, external and internal forces (Holling Citation1973, Citation1996, Pimm Citation1984, Gunderson Citation2000, Murphy Citation2012). Lake surface water temperature and oxygen saturation are good diagnostic tools of lake ecosystem resistance and resilience following extreme storms because they are a product of changing atmospheric conditions, thermal dynamics, and metabolic processes (Livingstone et al. Citation2003, Shade et al. Citation2012, Meerhoff et al. Citation2022, Thayne et al. Citation2022).
Eutrophication and climate change are 2 pressing disturbances directly and indirectly changing surface water temperature and oxygen saturation dynamics in many lakes. Eutrophication tends to be a result of point source pollution and poor land use practices in the watershed and has led to drastic water quality shifts in many lakes (Carpenter et al. Citation1998, Foley et al. Citation2005, Jöhnk et al. Citation2008, Rigosi et al. Citation2014). The subsequent changes in turbidity (e.g., from phytoplankton biomass and/or sediment inputs) and/or primary production are driving factors shaping resistance and resilience following extreme storms (Shade et al. Citation2010, Citation2012, Tsai et al. Citation2011, Thayne et al. Citation2022). While some lakes may experience atmospheric stilling (Vautard et al. Citation2010, Woolway et al. Citation2019, Janatian et al. Citation2020, Stetler et al. Citation2021), climate change is simultaneously impacting pre-storm lake processes (Wagner and Adrian Citation2009, Kraemer et al. Citation2021, Mesman et al. Citation2021, Woolway et al. Citation2021a, Citation2021b) and increasing the frequency, intensity, and duration of extreme storms globally (Webster et al. Citation2005, Zhang et al. Citation2013, Lehmann et al. Citation2015, Zeng et al. Citation2019). The extent to which extreme storms impact the resistance and resilience of water temperature and oxygen saturation conditions is currently unknown, but storms likely play a role in shaping resistance and resilience through abrupt changes in physiochemical and biological conditions (Havens et al. Citation2011, de Eyto et al. Citation2016, Kasprzak et al. Citation2017, Andersen et al. Citation2020, Calderó-Pascual et al. Citation2020).
Short- to long-term changes in water temperature and oxygen saturation as a result of extreme storm events tend to be related to changes in transparency via overland flooding of materials into the lake, sediment resuspension, and the formation or deformation of phytoplankton blooms (Williamson et al. Citation2016, Doubek et al. Citation2021, Mesman et al. Citation2021, Sullivan et al. Citation2022). Heavy rainfall can lead to flooding and/or influxes of nutrients and colored dissolved organic matter, resulting in changes in water quality, trophic dynamics, and the timing and/or onset of phytoplankton blooms (Gaiser et al. Citation2009a, Citation2009b, Jennings et al. Citation2012, Larsen et al. Citation2020). Depending on the lake, the time scale of said rain effects may only be short-lived, especially when a lake experiences flushing (Stockwell et al. Citation2020). Likewise, in shallow lakes, high wind speeds can lead to the resuspension of nutrient-laden lake sediments, sometimes driving the formation of cyanobacterial blooms (Zhu et al. Citation2014). While extreme storms are a driving force of change in lakes, whether a lake responds in an extreme way is largely dependent on the antecedent lake characteristics (Shade et al. Citation2012, Perga et al. Citation2018, Thayne et al. Citation2022). Climate change is affecting antecedent lake characteristics and processes in important ways that affect how lakes will respond to storms (Woolway et al. Citation2020). Warming surface waters and changing mixing regimes have led to changes in chlorophyll a (Chl-a) concentrations and global decreases in dissolved oxygen in many lakes (Paerl and Huisman Citation2009, Woolway and Merchant Citation2019, Jane et al. Citation2021). The resulting variability in surface Chl-a concentration can have meaningful effects on the resistance and resilience of water temperature and oxygen saturation following extreme storms (Thayne et al. Citation2022). Ultimately, how water temperature and oxygen saturation resistance and resilience are shaped is a result of many different processes acting on the system at different biophysical, temporal, and spatial extents (Carpenter and Cottingham Citation1997, Stockwell et al. Citation2020).
In our previous study (Thayne et al. Citation2022), we discovered several crucial antecedent lake characteristics that significantly influence the ability of a lake to resist and recover from severe storms. Specifically, our investigation of Müggelsee, a shallow eutrophic lake in Germany, revealed that the antecedent lake characteristics held greater significance than the actual storm conditions in determining the lake’s resistance and resilience. Müggelsee’s biological and physiochemical properties showed enhanced resistance and resilience with increasing antecedent turbidity and/or Chl-a concentration, suggesting the lake was less likely to change (i.e., increased resistance and resilience) under turbid versus clear conditions. This result, together with the finding that antecedent lake characteristics were more important than storm conditions, suggests that extreme storms may affect lake resistance and resilience differently depending on feedback and processes that shape lake trophic state (Mettelbach et al. Citation1995, Carpenter et al. Citation2001, Nõges et al. Citation2011, Perga et al. Citation2018, Thayne et al. Citation2022).
The surface lake dynamics driving resistance and resilience of water temperature and oxygen saturation following storms across a gradient of lake trophic states are likely dependent on the antecedent biological and physiochemical structural differences along the gradient (Walker et al. Citation1981, Citation1997, Scheffer et al. Citation1993, Thayne et al. Citation2022). The trophic state of a lake is generally defined by its phosphorus and Chl-a concentration and clarity (i.e., Secchi depth; Carlson Citation1977) and their overall effect on food web interactions and biodiversity (Carpenter Citation1987, Beaver and Crisman Citation1989, Biddanda et al. Citation2001, Linz et al. Citation2017). Thus, resistance and resilience among the varying trophic states are likely related to differing pathways of secondary succession or the ability of the existing physiochemical and biological feedback of the lake to self-organize and maintain previous relationships and processes following sharp changes in lake conditions (Tsai et al. Citation2011, Shade et al. Citation2012, Shatwell et al. Citation2016, Sullivan et al. Citation2022, Thayne et al. Citation2022). Therefore, to understand the synergistic effects of climate forcing and shifting lake conditions on the resistance and resilience of surface water temperature and oxygen saturation, we analyzed the effects of 576 extreme storms split across 8 lakes along a trophic state gradient. Herein, we addressed whether trophic state shapes resistance and resilience of surface water temperature and oxygen saturation relative to extreme storm disturbances. We hypothesized that eutrophic lakes, characterized by higher nutrient levels and substantial algal growth, would exhibit lower resistance to extreme storms due to the storm potential to disrupt and break down biological controls on surface water physiochemical dynamics. However, we anticipated that eutrophic lakes would demonstrate higher resilience because the abundant algae in these lakes could efficiently utilize excess nutrients and facilitate a quicker recovery of surface water dynamics following storms. By contrast, we expected oligotrophic lakes, with lower nutrient levels and decreased algal growth, to display higher resistance but lower resilience. By synthesizing resistance and resilience of lake surface water temperature and oxygen saturation using in situ high-frequency data following extreme storms, we provide an opportunity to guide theory development by linking shifting lake and storm conditions to surface water temperature and oxygen saturation resistance and resilience.
Methods
Overview
We tested the effects of trophic state conditions, antecedent lake characteristics, and storm conditions on standardized indices of water temperature and oxygen saturation resistance and resilience across 8 lakes exposed to extreme storms between 2002 and 2019. Extreme storms were classified by using wind speeds estimated to have 100-day return periods for a given lake, which are wind speeds predicted to be within the 99th percentile for a given lake/region according to general extreme value (GEV) theory (discussed later). Resistance and resilience were calculated using methods (Orwin and Wardle Citation2004, Thayne et al. Citation2022) that fall under interpretations of resistance and resilience introduced by Holling (Citation1973, Citation1996), commonly referred to as ecological resilience (Gunderson Citation2000, Murphy Citation2012). Ecological resilience is defined as an ecosystem’s ability to absorb change (i.e., to be resistant) and persist (i.e., to be resilient) while still maintaining relationships between populations and/or state variables. While the term resistance does not appear in the definition, we can infer from the word “absorb” the ability to be resistant. For example, if a lake is better able to absorb the energy of a storm, then the magnitude of peak displacement will be lower (i.e., high resistance) when compared to a lake that cannot absorb the same amount of energy (i.e., low resistance). Using this definition as a guide, we developed a semiautomated process to calculate resistance and resilience of surface water temperature and oxygen saturation in response to extreme storms (Thayne et al. Citation2022). To determine the relative importance and partial effects of lake trophic state (i.e., annual mean phosphorus concentrations, Chl-a, and Secchi depth) while controlling for antecedent lake characteristics (i.e., surface water temperature, Schmidt stability, percent oxygen saturation, photosynthetically active radiation [PAR], and mean lake depth) and storm conditions (i.e., wind direction/speed, storm duration, day of peak wind speeds, accumulated rain, time between storms, and storm year), we fit boosted regression trees (BRT) to predict resistance and resilience. Resistance and resilience of surface water temperature and oxygen saturation across a lake trophic state gradient reflects the varying physiographic and/or watershed conditions shaping each lake’s evolved biophysical relationships for responding to extreme storms. Thus, by synthesizing resistance and resilience together with information on trophic state, antecedent lake characteristics, and storm conditions, we provide a systematic, standardized, and quantitative approach for analyzing ecological resilience and the effects of extreme storms on surface water dynamics.
Study sites and data collection
Each of the 8 lakes was equipped with high-frequency monitoring (HFM) stations anchored in central locations to collect water temperature, percent oxygen saturation, and PAR every 1–60 min at surface depths between 0.5 and 1.5 m, depending on where epilimnetic characteristics were measured in each lake. Hourly mean lake conditions were calculated for each lake parameter to ensure conformity in data collection frequency between the lakes. In addition to surface lake conditions, 7 of the lakes (excluding Lake Võrtsjärv) collected hourly water temperature profiles of the entire water column, which were used to calculate thermal stratification, or Schmidt stability (Winslow et al. Citation2018). Measurements of PAR were taken above the lake surface, and in the case of Müggelsee and Lough Feeagh, solar radiation was measured in W/m2 but converted to μmol/m2/s using an approximation of 1 W/m2 ≈ 4.6 μmol/m2/s (Sager and McFarlane Citation1997). PAR in this case could be considered either a storm condition or an antecedent lake characteristic. Here, we interpreted PAR (i.e., incoming solar radiation) as an antecedent lake condition because of its effects on water temperature and phytoplankton growth; thus, we could consider PAR a “non-stormy” condition. Lake and storm data were collected on the lakes, or nearby meteorological stations between 2002 and 2019, depending on the lake. Total phosphorus (TP) concentration in the lakes was collected with different sampling rates and strategies: weekly in Müggelsee; every 2 weeks in Lake Erken; and monthly in Trout Lake, Trout Bog, Lake Annie, Lough Feeagh, and Võrtsjärv. Mendota had the most sporadic phosphorus collection but was generally sampled 3–4 times a year in spring, summer, and fall.
Storm conditions were measured and calculated during a defined storm period centered around the peak in wind speed/dynamic pressure (discussed later), defined as starting when dynamic pressure at the surface of the lake was ∼0 prior to the peak and ended when dynamic pressure returned to 0 after the peak (Thayne et al. Citation2022). Dynamic pressure (Pa) is a measure of the wind’s kinetic energy over the surface of the lake (i.e., 0.5ρV2, where ρ is air density = 1.2 kg/m3 and V = wind speed in m/s; Palutikof et al. Citation1999). Essentially the wind peaks served as a way to classify storms and accompanying conditions and characteristics such as accumulated rainfall, storm duration, time elapsed between storms, day of peak wind speeds, and year the storm occurred. Therefore, storm duration was the mean hourly length of the storm period, and the day the peak wind speeds occurred was used as a proxy for storm seasonality. Time between storms was quantified by accounting for the months elapsed since the last analyzed storm within a given year. When we used dynamic pressure rather than wind speed when fitting the GEV model, the number of storms considered extreme increased slightly for each lake, thus increasing the variability in storm type and lake surface conditions considered. By doing so, we likely included storms that may or may not fit the arbitrary definition of extreme. Furthermore, the importance of antecedent lake characteristics blurs further what exactly is an extreme disturbance for any given lake. Nonetheless, mean hourly wind speeds, wind directions, and rain were collected from the HFM stations or nearby meteorological stations (SMHI Centre Citation2022; i.e., Lake Erken) or from nearby airports (National Centers For Environmental Information Citation2022; i.e., the North American lakes). In relation to wind directions collected above the lake surface, all monitoring stations were centrally located in the lakes, and thus obstructions (e.g., forest or hills) did not obfuscate the collection of wind direction. We used wind direction to capture the effects of fetch with the understanding that the greatest fetch of a lake will be most impacted by wind directions corresponding to that fetch. Nearby meteorological stations and airports were used in cases where the HFM station data were not sufficient in length (Palutikof et al. Citation1999; i.e., ≥10 years) for classifying extremes and/or data were unreliable. The station for Lake Erken is located ∼0.5 km from the lake shore, and airport data for Trout Lake and Trout Bog is ∼4.8 km from the lakes. For Lake Annie we used airport data ∼107 km from the lake because all the nearby meteorological stations with publicly downloadable data are located on the coast where wind speeds are different from those inland. Therefore, we chose the closest inland airport that had publicly available data. While we may have identified some storms for Lake Annie that were not extreme for the area, these would be outlying events and mostly negligible during the modeling process. Furthermore, these outlying events would result in a 1 for resistance and resilience, or not extreme for Lake Annie. To put this into context, of the 129 storms identified, 11 resulted in water temperature and oxygen saturation with resistance and resilience between 0.90 and 1.0. Here, resistance and resilience were quantified on a standardized scale between −1 and 1, where 1 indicates total resistance and resilience, and ≤0 represent times of >100% displacement for resistance and times of 0% recovery for resilience. Thus, the station, while somewhat distant from Lake Annie, captured weather conditions relevant to storm responses in the lake. For Lough Feeagh and the lakes in North America, wind speeds were collected in knots or mph, which we converted to m/s. Wind direction was included for all lakes except Võrtsjärv. Hourly rain averages were summed over the storm period to gain an understanding of the cumulative effects of rain.
Extreme storm classification
Extreme storms were classified for each lake/region by quantifying the return period or the maximum wind speed/dynamic pressure that exceeded, on average, once every T days (Equationequation 1(1)
(1) ) during the growing season of phytoplankton (i.e., Mar–Oct) for each lake (Palutikof et al. Citation1999, Thayne et al. Citation2022). We estimated return periods by fitting GEV distribution models for each of the lakes’ mean maximum wind speed/dynamic pressure data, where the location, scale, and shape parameters of their respective distributions were estimated using L-moment statistics (Hosking Citation1990, Palutikof et al. Citation1999, Gilleland and Katz Citation2006, Citation2016). L-moment statistics used for parameter estimation provide better estimates of extremes when high frequency time series are <20 years, as in our study. Therefore, the probability of a dynamic pressure quantile (XT) with a return period (T) is given by:
(1)
(1)
(2)
(2) where XT is the return period for a given dynamic pressure quantile, β is the mode (location parameter) of the GEV distribution, α is the dispersion (scale parameter), and k is the distribution shape parameter, which is either of type Gumbel (k = 0), Fréchet (k > 0), or Weibull (k < 0; Hosking Citation1990, Palutikof et al. Citation1999, Gilleland and Katz Citation2006, Citation2016). If the L-moment statistics estimates a distribution of type Gumbel (k = 0), the equation is reduced to Equationequation 2
(2)
(2) . While all classified storms were analyzed at hourly levels, we modeled daily return periods in dynamic pressure. Therefore, to be considered extreme we selected a threshold return period of 100 days, or wind peaks that exceeded a given wind speed for each lake, estimated to occur every 100 days or more. For example, Müggelsee wind speeds exceeding 8.5 m/s, or 45.2 N/m2 as it relates to dynamic pressure, were estimated to occur every 100 days or more. All models were fit using the R statistical software package extRemes version 2.0 (Gilleland and Katz Citation2006, Citation2016, Thayne et al. Citation2022). Not all the storms could be labeled extreme, depending on the arbitrary definition used to define extremes. Nonetheless, the methods used here have been used previously to classify wind extremes (Laib and Knavski Citation2016) and those laid out in the 2012 Intergovernmental Panel on Climate Change (IPCC) report on environmental extremes (IPCC Citation2012). Overall, we analyzed mean wind speeds between 5.7 and 21.1 m/s.
Quantifying resistance and resilience
To calculate resistance to storms and resilience following the storms, we used hourly in situ measurements capturing the response of surface water temperature and oxygen saturation collected between 0.5 and 1.5 m depth. We calculated storm responses of surface water temperature and oxygen saturation into resistance and resilience indices to standardize comparisons among lake ecosystems with differing biogeochemical feedback and processes forming their trophic state (Orwin and Wardle Citation2004, Tsai et al. Citation2011, Cantarello et al. Citation2017, Guillot et al. Citation2019, Thayne et al. Citation2022). We focused on the epilimnion of lakes, generally where the strongest storm/mixing effects are observed. To capture the transient nature of surface lake dynamics we used antecedent lake characteristics based on the 3-day mean of each lake state predictor variable prior to the beginning of each storm analyzed. Thus, water temperature and oxygen saturation resistance for the 8 lakes was quantified as the peak displacement in epilimnetic water temperature or oxygen saturation induced by the initial storm disturbance when compared to the 3-day mean of antecedent water temperature or percent oxygen saturation conditions, respectively (a–b; Equationequation 2(2)
(2) ; Orwin and Wardle Citation2004, Thayne et al. Citation2022). Note that calculations of water temperature resistance and resilience are unit dependent, and we used °C.
Figure 1. Schematic depicting how to apply equations Equation3(3)
(3) and Equation4
(4)
(4) to calculate resistance and resilience indices of lake water temperature and percent oxygen saturation (y-axis, blue line) responding to a storm disturbance (vertical black dashed line), and peaking (P0) at time t0 and recovering (Px, horizontal red solid line) between time tx and te. Vertical green dashed lines represent times (x-axis) at which D0 and Dx are calculated. Resistance (RS) is therefore the difference between the absolute peak displacement in water temperature or percent oxygen saturation and their respective 3-day mean control conditions (C, horizontal red dashed line), or |D0| = |C − P0|. Resilience (RL) is then calculated by taking the absolute difference between C and the mean value of Px averaged over a 72 h window with the lowest standard error (σM, horizontal black solid line) in water temperature or percent oxygen saturation, or |Dx| = |C − Px|. Both resistance and resilience indices range between −1 and 1, where a 1 indicates total resistance and resilience. A resistance value of 0 represents times when water temperature or oxygen saturation conditions were displaced by 100%, either increasing or decreasing relative to antecedent conditions. Therefore, a resilience value of 0 indicates water temperature or oxygen saturation remained at peak displacement levels, or there was 0% recovery. Thus, negative values of resistance represent times of more than 100% displacement, while negative values of resilience represent times when water temperature or oxygen saturation continued to drift further from peak displacement levels, or overcompensated in their recovery by overshooting the 3-day mean antecedent control conditions.

In some storm scenarios water temperature and/or oxygen saturation displayed approximately equidistant peaks but opposite displacement levels, one positive and one negative. Or in other words, during some storms, water temperature and/or oxygen saturation increased above and then decreased below pre-event conditions (or vice versa), making peaks and recoveries difficult to isolate. For example, wind may initially increase surface oxygen saturation in the lake, but following the wind peak, oxygen saturation decreases as a result of wind-driven change in water temperature. These responses in surface dynamics may result from upwelling or lake seiche, and in such cases we used ad hoc BRT models to determine whether and when storms typically increased or decreased the response variable (Thayne et al. Citation2022). Thus, in some of the lakes (e.g., Müggelsee, Feeagh, Erken, Mendota, and Võrtsjärv) these models included the effects of lake characteristics such as turbidity, Chl-a, phycocyanin, and dissolved organic matter, providing a holistic view of how each lake characteristic partially affected water temperature or oxygen saturation. This information provided a diagnostic tool to determine the direct and indirect effects of the storm and lake conditions effecting the response of water temperature or oxygen saturation and allowed us to determine the appropriate displacement value for resistance, and ultimately resilience. Returning to the previous example, although wind directly contributed to the initial rise in oxygen saturation, the indirect impact of wind on water temperature played a more significant role than wind alone. Consequently, the decline in oxygen saturation was utilized in determining resistance. These models were fitted with a maximum of 10 000 trees, a tree complexity of 2, and a learning rate between 0.82 and 0.1 × 10−9; bag fractioning was set at 0.5 (Elith et al. Citation2008, Hijmans et al. Citation2017, Thayne et al. Citation2022). Model selection methods are discussed later.
Resilience was quantified by defining a 72 h sliding “recovery” window immediately following the peak displacement of surface water temperature or oxygen saturation, during which we calculated the standard error in water temperature or oxygen saturation in each window. The window resulting in the lowest mean standard error compared to the previous windows was then selected as the recovery level (a and c; Equationequation 3(3)
(3) ). To understand how sensitive resilience calculations were to the size of the recovery window we conducted a sensitivity analysis comparing 72, 120, and 168 h sliding windows (Supplemental Table S1). Although the results were somewhat sensitive to the size of the window, we used 72 h to contain resilience calculations to the identified storms to the exclusion of other possible extreme disturbances (e.g., stratification and/or phytoplankton blooms), which warrant their own calculations of resilience (Batt et al. Citation2017). Sensitivity between the windows was dependent on the storm and overall variability in post-storm water temperature and oxygen saturation. Most important, despite some sensitivity, all 3 windows identified the same lake conditions for a recovery point. Calculating resilience with this method allows assumptions that ecosystems continuously undergo gradual change through time with a tendency to recover toward antecedent conditions, but also allowed the system to find new balance by adapting, or reorganizing, through changes in population relationships and/or state variables following storm disturbances (Holling Citation1973, Citation1996, Pimm et al. Citation1984, Gunderson Citation2000, Murphy Citation2012, Thayne et al. Citation2022):
(3)
(3)
(4)
(4)
The quantification of resistance and resilience was a semiautomated process with predefined time windows (R code: https://github.com/mthayne527/Resistance_Resilience_Estimation). In cases where recovery was incomplete because of time constraints, we extended the post-storm period until a resilience level was determined (Thayne et al. Citation2022). While calculating resilience this way introduced some subjectivity, the approach constrained our calculations to the storm under analysis and avoided assumptions about global equilibria, a key tenet when calculating interpretations of ecological resilience. Prior to quantifying resistance and resilience, surface water temperature and oxygen saturation were seasonally adjusted so that resilience could be interpreted as a return to conditions expected outside of seasonal driven variation (Thayne et al. Citation2022). Water temperature for all lakes was adjusted for seasonality, whereas percent oxygen saturation was adjusted for both annual and diurnal oscillations (Thayne et al. Citation2022). Seasonal decomposition was performed using functions in the forecast (version 8.5) package found in the R statistical environment (Hyndman and Khandakar Citation2008, Nieto and Mélin Citation2017). Seasonal decompositions were conducted by first transforming the respective time series into multiseasonal time series (R function msts), which were then used to identify season and trend components using Loess (R function mstl; Thayne et al. Citation2022). We then subtracted the identified seasonal component from the original time series.
Predicting variability in resistance and resilience
We fit 2 independent models consisting of 15 BRTs to predict variability in lake-to-lake surface water temperature and oxygen saturation resistance and resilience following extreme storms. Predictors of water temperature and percent oxygen saturation resistance and resilience were the varying lake trophic proxies, antecedent lake characteristics, and storm conditions (n = 1151; and ). Trophic conditions in the models were represented by annual means of Chl-a concentration, TP concentration, and Secchi depth. Antecedent lake characteristics included water temperature, percent oxygen saturation, Schmidt stability, and PAR. All antecedent lake characteristics were seasonally adjusted following the methods described previously to be consistent with the quantification of resistance and resilience. Additionally, we included mean lake depth as a numerical variable in case the gradient in lake depths was important for determining resistance and resilience. We further tested other lake characteristics such as lake surface area and shoreline length, but both were equally as unimportant as mean lake depth.
Figure 2. (a–h) Distributions of antecedent lake characteristics and year of data collection/storm exposure (x-axis) for each of the 8 lakes (y-axis). The lakes followed along a (f, i) phosphorus, (g, j) chlorophyll a, (h, k) Secchi depth, and (g) lake depth (i.e., 2.8–15 m) gradient. Both phosphorus (f) and chlorophyll a (g) were scaled for visual purposes by taking the square root of the raw values. While phosphorus, chlorophyll a, and Secchi depth were included in the BRT models, panels i–l are included for visuals only. The colored dots in panels i–l are organized by increasing mean values.

Figure 3. Extreme wind storm characteristics related to each lake. (a–f) Conditions extracted from the identified extreme storms. Each panel depicts the lakes (y-axis) and their associated storm conditions (x-axis).

Lake variables were chosen based on their importance in previous work (Shade et al. Citation2012, Thayne et al. Citation2022) and their availability at an appropriate temporal resolution (hourly) and consistency across all lakes. Furthermore, both water temperature and oxygen dynamics are critical in many biogeochemical feedbacks and processes that might be disrupted as a result of sharp changes in their conditions. To ensure we accounted for times that surface water temperature and oxygen saturation did not correlate in their directional response to storms, we included a factor variable (i.e., WT/O2 response) representing the 2 response variables, water temperature and oxygen saturation resistance and resilience in the models, respectively. We accounted for seasonality in the model by including the proxy Julian day when peak wind speeds were observed. Storm characteristic predictors in the model included maximum wind speed, wind direction, duration, day of peak wind speeds, and time elapsed between storms. We also converted the year the storm occurred into decimal year and included it as a numerical predictor in the models. Last, we used antecedent lake characteristics rather than post-storm lake characteristics to predict resilience for 2 reasons. First, we found the more logical approach was to use antecedent lake characteristics because of the way resilience is calculated (i.e., a difference between antecedent control conditions and post storm conditions). Second, using post-lake conditions to predict resilience is a circular argument (i.e., post conditions are dependent on post conditions). Nonetheless, we also fit the BRTs with post-storm lake conditions to see whether predictability of resilience improved with those conditions, but they did not.
We conducted multicollinearity tests to ensure we did not reduce the predictive power of any given predictor variable. To test multicollinearity among predictor variables we used Pearson and Spearman rank correlation matrices to gain a better understanding of the linear and nonlinear relationships between predictors. Pearson correlations between the predictor variables showed mostly low coherence between them (r ≤ ±0.39). However, water temperature and wind speed/wind direction/mean lake depth showed moderate negative correlations (r ≈ −0.50). Additionally, mean lake depth and wind speed/wind direction showed moderate positive correlations (r ≈ 0.50). The correlations likely represent the regional differences among the lakes themselves and the wind regimes they were exposed to, respectively. For completeness, we additionally fit BRT models with water temperature and wind speed standardized across lakes to ensure these moderately high correlations were not impacting the effect of lake depth. We found that these correlations were not affecting the results, so original values of water temperature and wind speed were retained in the models.
Model fitting and parameter optimization were performed using 10-fold cross-validation to minimize BRT model overfitting (Elith et al. Citation2008). Before fitting the models and to enhance predictive performance, we selected a random sample of 52 storms and 6 of the 8 lakes where the number of storms sampled was equal to the least number of possible outcomes for water temperature and oxygen saturation (i.e., storms identified for Lake Erken). We then used 10-fold cross-validation to fit the models, where data were split 30–70% for resistance and 50–50% for resilience (i.e., ∼30–50%, or 16–25, of the 52 randomly sampled storms were considered for each lake and model iteration). The data were split for resilience 50%/50% because including slightly more data per model iteration reduced error, learning rate, and number of trees needed to find a model fit and improved overall predictive performance following cross-validation. Model parameters were tuned to maximize model performance in cross-validation. Models were optimized by iteratively running each model with decreasing learning rates beginning at 0.82 and decreasing by a factor of 2 until reaching 0.1 × 10−9. An optimum learning rate was selected when ≥1000 trees and holdout predictive deviance from cross-validation were minimized (Elith et al. Citation2008). Tree complexities of 1, 3, 5, and 7 were tested to evaluate whether increased interactions between predictor variables improved mean deviance standard error and predictive performance in cross-validation (Supplemental Tables S2–S3). Therefore, model selection was based on the combination of learning rate, number of trees, and tree complexity that resulted in the lowest holdout mean predictive deviance and highest predictive performance (Elith et al. Citation2008, Thayne et al. Citation2022). Results were averaged across the 15 independent models to ensure patterns and interactions described were robust to the exclusion of some lake and storm conditions. We found that models predicting resistance performed well in cross-validation in which the correlation between predicted and observed values in holdout test datasets was r = 0.52. The models predicting resilience resulted in comparatively lower predictive performance in cross-validation when the correlation between predicted and observed values in holdout test datasets was r = 0.25. To further understand predictive performance following cross-validation, we averaged across the models the mean absolute error and mean % deviance explained and made predictions using the models to determine the linear relationship between observed and predicted values following cross-validation (Supplemental Table S3). Predictions were conducted using all predictors used in the models. Mean % deviance explained was calculated using 2 methods. The first (Elith et al. Citation2008), a more conservative method than the second (Nieto and Mélin 2017), is calculated by taking the total deviance minus the cross-validated residual deviance and then dividing the outcome by the total deviance. The second method is calculated by subtracting from 1 the quotient of residual deviance divided by total deviance (Supplemental Table S3).
Further exploration of the results, such as identifying important interactions, was conducted by first extracting the interaction “rank list” from each of the 15 BRT models. We then summed the importance of each ranked interaction to identify the overall cumulative importance of the varying interactions across the 15 models. Exploring individual predictions of resistance and resilience relative to a specific lake and storm condition(s) was performed by first setting all predictors of non-interest to NA. We then fit predictive models using the optimal number of trees from each of the 15 models to predict resistance and resilience relative to the lake or storm condition(s) in focus. Optimization and selection of BRT hyperparameters (e.g., cutoffs captured by individual trees in the BRT) was automated by using the function gbm.step as part of the R package dismo (version 1.1-4; Hijmans et al. Citation2017). All statistics and associated graphics were produced in the R statistical computing environment (Wickham Citation2016, R Core Team Citation2019, Kassambara Citation2020).
Results and discussion
Antecedent lake and storm conditions
Whether a storm is extreme or not largely depends on the state of the antecedent surface lake conditions at the time of storm exposure (). Mean antecedent surface water temperature in the lakes was 18.1 °C, with a true range of −0.8–31.9 °C and an interquartile range of 13.2–23.1 °C. Mean antecedent oxygen saturation was 99.6%, with a true range of 41.1–228.7% and an interquartile range of 91.9–105.4%. Mean antecedent Schmidt stability was 161.9 J/m2, with a true range of 0–1271 J/m2 and an interquartile range of 4.8–227.9 J/m2. Mean antecedent regional PAR was 297 μmol/m2/s, with a range of 0–972 μmol/m2/s and an interquartile range of 157–405 μmol/m2/s; Trout Lake and Võrtsjärv had the most varied light conditions prior to storm exposure. In addition to antecedent conditions, we also considered the year in which the wind peak occurred, thus capturing the number of storms analyzed for each lake and time span of lake conditions considered. The number of storms each lake was exposed to was Annie = 129, Erken = 26, Feeagh = 91, Mendota = 84, Müggelsee = 113, Trout Bog = 35, Trout Lake = 52, and Võrtsjärv = 47. The lakes followed a phosphorus, Chl-a, Secchi depth, and lake depth (i.e., 2.8–15 m) gradient ().
Depending on lake location, storms included tropical storms, unnamed cyclonic depressions, derechos, heavy rainfall events, and named European windstorms. We also analyzed shorter duration storms where peak wind speeds lasted only hours, such as microbursts and squalls (). The varying storms were accompanied by mean wind speeds of 10.6 m/s with a true range of 5.1–21.1 m/s and an interquartile range of 10–11.6 m/s, where Lough Feeagh on the coast of Ireland experienced the highest wind speeds and Võrtsjärv and Müggelsee recorded the lowest wind speeds to be considered extreme. Storms had a mean wind direction of south by southwest with a true range of 360° and an interquartile range of south by southeast to southwest. Storm durations had a mean of 135 h, with a true range of 13–365 h and an interquartile range of 96–164 h. Associated with many of the storms were substantial amounts of accumulated rain (rain was scaled by taking the square root of original values), and in some cases were associated with major flooding events in places such as Wisconsin (USA) and Ireland. Mean rain accumulation was 17.5 mm, with a true range of 0–503 mm and an interquartile range of 1.7–21.3 mm. Time between storms varied between the different lake locations and had a mean of 0.9 months, with a true range of 0.1–5.6 months and an interquartile range of 0.4–1 months. Lake Annie was most frequently exposed to extremes storms, and Lough Feeagh experienced the longest time between extreme storms. Last, the day that peak wind speeds occurred spanned the seasonal spectrum and had a mean Julian day of 198, with a true range of Julian day 56–305 and an interquartile range of 138–262; thus, most storms occurred approximately between May and September. Both surface water temperature and oxygen saturation decreased or increased sharply relative to storm initiation and were generally correlated in their directional response (i.e., positive or negative) and recovery trajectories; however, the degree of change in observed resistance and resilience varied among the lakes (a–c and a–i).
Figure 4. (a) North America and (c) Europe with colored dots corresponding to the 8 lakes and their locations, respectively. (b) Distributions of observed resistance and resilience (quantified on a standardized scale from −1 to 1, see legend) of percent oxygen saturation and water temperature. The red line marks 0, which represents 100% displacement relative to antecedent lake characteristics and 0% recovery as it relates to resistance and resilience respectively, organized by each lake’s annual mean chlorophyll a concentration, with Lough Feeagh having the lowest concentrations. The more overlap between resistance and resilience the more likely the lake had low resistance but was more resilient following storms, whereas separation between distributions of resistance and resilience, with sharp peaks in resistance and flat distributions in resilience, represents lakes with high resistance but low resilience.

Figure 5. Classified storm examples for Lake Annie, Lough Feeagh, and Müggelsee. (a) Satellite imagery of Tropical Storm Fay, Lake Annie, Florida (2008); (b) an unnamed cyclonic depression coalescing over Lough Feeagh, Ireland (2012); and (c) European Wind Storm Xavier (2017) with formation/direction depicted by the blue, red, and dashed black arrows. The red and blue arrows depict warmer and cooler storm fronts, respectively, which form the jet stream depicted by the black dashed arrow. (d–f) Day and month (x-axis) of the storm time series where the black line is mean wind speed (y-axis) and dynamic pressure (secondary y-axis), and the red dotted line denotes the dynamic pressure threshold used to classify events for analysis. The green shaded area represents the area of the storm in which storm conditions including wind direction, wind speed, peak wind speed, storm duration, and accumulated rain were extracted. (g–i) Day and month (x-axis) of the time series, where the green line is percent oxygen saturation (x-axis) and the blue line is water temperature (secondary y-axis). The green shaded area represents the storm period.

Resistance and resilience indices
A simple approach to interpret the resistance and resilience indices is to examine how much their distributions overlap in each lake (). We observed a notable increase in overlap as mean annual Chl-a concentration increased, indicating a decline in resistance and an enhancement of resilience (). A finer description of the distributions revealed surface water temperature across lakes had a mean resistance of 0.77. In other words, water temperature conditions were displaced on average by 23% at peak displacement relative to 3-day mean antecedent lake characteristics, ranging from −0.53 (i.e., ∼150% change) to 1.0 (100% resistant), and an interquartile range from 0.69 to 0.90. Water temperature resilience was low across lakes and had a mean resilience of 0.28, which means that on average the lakes were able to recover 28% of antecedent water temperature conditions following the storms, ranging from −0.88 (i.e., drifting, or overcompensation in resilience level, discussed earlier) to 1.0 (100% resilient) and an interquartile range from 0.04 to 0.52. Surface oxygen saturation had a mean resistance of 0.74, which means oxygen saturation conditions were displaced on average by 26% at peak displacement, ranging from −0.32 (∼130% change) to 1.0 with an interquartile range from 0.62 to 0.92. Oxygen saturation conditions were moderately resilient with a mean resilience of 0.46 (i.e., on average the lakes were able to recover 46% of antecedent oxygen saturation conditions following the storms) ranging from −0.84 to 1.0 (100% resilient) with an interquartile range from 0.22 to 0.72. While the 2 variables tended to have similar directional responses to storms (i.e., resistance), water temperature tended to show more incomplete recovery (i.e., diminished resilience) in comparison. However, understanding how trophic state conditions, antecedent lake characteristics, and storm conditions relate is important before exploring the partial effect patterns identified by the BRTs.
Trophic state proxies and storm conditions shape antecedent lake characteristics
Feedback and processes that determine trophic state and meteorological forces are widely understood to impact the functioning of lake ecosystems (Carpenter Citation1998, Stockwell et al. Citation2020). While trophic state is generally defined here as mean annual Chl-a, TP, and Secchi depth, their relationship to antecedent lake characteristics (i.e., 3-day mean prior to storm) such as surface water temperature and dissolved oxygen, thermal stratification (i.e., Schmidt stability), and PAR have a more immediate influence on shaping resistance and resilience of the lakes (Thayne et al. Citation2022). Annual means of Chl-a (i.e., a proxy for phytoplankton biomass), TP concentration, and transparency (i.e., Secchi depth) had significant (p < 0.001) positive and negative nonlinear correlations with antecedent lake characteristics (). Thus, higher Chl-a concentration and nutrient concentrations were associated with decreased transparency, thermal stratification, incoming PAR (i.e., affected by regional climate at each lake), and surface water temperature (). PAR, while considered an antecedent lake characteristic, was measured above the water surface and thus suggests that increased Chl-a and nutrient concentrations were associated with the regional climate of the lake. Thus, PAR is affected by more or fewer cloudy days and/or frequency of storm exposure. Increased transparency was significantly and positively correlated to antecedent Schmidt stability, regional PAR, surface water temperatures, and to a lesser extent oxygen saturation (). Wind speed was significantly and negatively correlated with decreases in mean annual Chl-a while antecedent water temperature and Schmidt stability were negatively correlated with both wind speed and wind direction (). Thus, high wind speeds with directions between southwest to northwest were associated with lakes with lower phytoplankton biomass, cooler antecedent water temperatures, and decreased thermal stratification. Increased levels of accumulated rain were then associated with decreased lake transparency and cooler surface water temperatures. Taken as a whole, the correlations among lake and storm conditions suggest that low transparency, warmer antecedent water temperatures, decreased thermal stratification, and/or regional PAR were associated with eutrophic lakes and/or increased storm exposure. Conversely, increases in the same lake characteristics (excluding water temperature) were associated with increased transparency (i.e., oligotrophy) and/or decreased storm exposure (). Exploring these correlations provides a broader context to understand the partial effects identified from the BRTs.
Figure 6. Spearman rank correlation showing relationships between trophic state proxies, antecedent lake characteristics, and storm conditions (figure is hierarchically clustered for readability). Relative strength (see color bar) of positive (red) and negative (blue) nonlinear relationships between trophic state proxies (i.e., mean annual total phosphorus, mean annual chlorophyll a, and mean annual Secchi depth), antecedent lake characteristics (i.e., Schmidt stability, water temperature, oxygen saturation, PAR, and mean lake depth), and storm conditions (i.e., wind direction, wind speed, accumulated rain, storm duration, day of peak wind speeds, time between storms, and storm year). Bolder values are stronger correlations, while grey values are weaker correlations. Total phosphorus = TP, chlorophyll a = Chl-a, depth = d., and wind speed = WS. Overall, the figure gives a broad understanding of how slower changing trophic sate proxies relate, or not, to faster changing antecedent lake characteristics. For example, mean annual TP is negatively correlated with Schmidt stability, PAR, and mean annual Secchi depth. The figure further shows how storm conditions relate to both slow changing trophic state proxies and faster changing antecedent lake characteristics, providing an understanding of how storm conditions can influence both gradual and rapid changes in lake surface dynamics. For example, accumulated rain was negatively correlated with antecedent water temperatures and mean annual Secchi depth.

Predicting resistance and resilience
The BRTs differed in predictive performance during and following cross-validation (Supplemental Table S2–S3). Following cross-validation, we calculated the percent deviance explained using 2 methods, one conservative and one less conservative. The models predicting resistance were able to explain 26% and 45% of the deviance in resistance values (Supplemental Table S2–S3). Conversely, despite stable model performance, surface water temperature and oxygen saturation resilience values were unpredictable across lakes, with models explaining 6–25% of the deviance in resilience. The unpredictability is likely related to the independence in resilience values across the different lakes (b). Taken as a whole, predicting the resilience of ecosystem dynamics is a complex task when we consider the infinite possibilities and pathways by which individual lake ecosystems may or may not recover following a disturbance. Thus, predicting the post-storm recovery trajectory of lake surface water temperature and oxygen saturation may be better understood on an ecosystem-to-ecosystem basis (Thayne et al. Citation2022). Nonetheless, we provide the results of resilience for cautious comparison to resistance (Supplemental Fig. S1–S2). Because the models poorly predicted resilience, the remainder of the discussion is focused on resistance.
Uncertainty in model predictions for resistance can be attributed to 2 causes. First, not all trophic state proxies, antecedent lake characteristics, and storm conditions were important for explaining the variation in resistance among lakes, at least for the water temperature and oxygen saturation proxies used here (refer to Supplemental Fig. S3–S8). For example, thermal stratification (i.e., Schmidt stability) only partially affected resistance in 5 of the 8 lakes, of which 2 (i.e., Müggelsee and Erken) showed decreasing resistance with increasing stratification. Second, some trophic state variables, antecedent lake characteristics, and/or storm conditions led to opposing effects between lakes and/or between water temperature and oxygen saturation.
Trophic state effect on thermal and dissolved oxygen resistance
Resistance in surface water temperature and oxygen saturation was primarily shaped by mean annual Chl-a concentration followed by antecedent oxygen saturation, water temperature, and mean annual phosphorus concentration (a–b and a–f). Although BRTs do not pinpoint causation, the importance of Chl-a in shaping resistance to storms is likely a result of its correlation with physiochemical parameters (e.g., TP, PAR, Schmidt stability, and wind speed; and c–e) as well as direct links to biological feedbacks and processes that determine Chl-a concentration in lakes of varying trophic state (Jones et al. Citation2005, Nõges et al. Citation2011, Shatwell et al. Citation2016). Resistance tended to diminish with increasing mean annual Chl-a and TP (b and c). Consequently, resistance in eutrophic lakes, which was associated with warmer surface temperatures, decreased stratification, and incoming PAR, experienced greater initial displacement on average following storms (b and b–d). In eutrophic lakes compared to oligotrophic lakes, Chl-a concentration (e.g. phytoplankton) has a greater influence over physiochemical processes such as thermal dynamics and/or light or nutrient and oxygen availability (; Carpenter Citation1998, Nõges et al. Citation2011, Shade et al. Citation2012, Shatwell et al. Citation2016, Thayne et al. Citation2022). Additionally, high Chl-a and subsequent respiration and decomposition of phytoplankton can drive eutrophic lakes out of equilibrium with the atmosphere (i.e., < or > 100% oxygen saturation; Nõges et al. Citation2011, Shatwell et al. Citation2016), which here diminishes resistance to storms (b and a). The increased interdependency between Chl-a and physical parameters, especially in but not limited to eutrophic lakes, may present greater opportunity for storms to initially displace biophysical relationships controlling thermal and oxygen conditions. For example, in eutrophic lakes, high Chl-a concentration (e.g., >5–10 µg/L) can trap solar energy at the surface of eutrophic lakes, subsequently increasing surface water temperature and lake resistance (Jones et al. Citation2005, Nõges et al. Citation2011; b). Thus, the ability of storms to both breakdown and/or stimulate phytoplankton growth increases the opportunity in eutrophic lakes for storms to initially displace biophysical controls on surface water temperature and oxygen saturation dynamics (Mesman et al. Citation2021, Stelzer et al. Citation2022, Thayne et al. Citation2022). Even so, in both eutrophic and oligotrophic lakes with lower mean annual Chl-a concentration, higher transparency (i.e., Secchi depth), and increased PAR, physical mechanisms such as water temperature, stratification and/or mixing state are likely the dominant drivers of resistance to extreme storms ( and b, d–f). Taken as a whole, the importance of trophic state proxies and their effect on shaping light and thermal dynamics is an important finding because they highlight links to previous research on feedback between ecological and physical lake processes (Jones et al. Citation2005, Nõges et al. Citation2011, Shade et al. Citation2012, Shatwell et al. Citation2016).
Figure 7. (a) Box plots of percent relative importance (x-axis) of each of the trophic state proxies, antecedent lake characteristics, and storm conditions (y-axis) predicting resistance. The varying color corresponds to the colors of the partial dependency plots in and . Relative importance for each lake and storm variable in the BRT models is a function of the frequency with which it was included in the BRTs individual regression trees and the overall improvement that resulted from its inclusion. The box plots give the probability of percent relative importance of a given predictor and fitted BRT model (black dots); standard error is represented by the error bars. Water temperature/oxygen saturation (WT/O2) response was a factor variable in the BRT models that accounted for the possibility of differing responses in water temperature and oxygen saturation resistance. (b) Partial dependency of surface water temperature and oxygen saturation resistance (y-axis) of 15 models (green lines) relative to annual mean chlorophyll a (x-axis). The black line represents the results of a fitted general additive model (y ∼ s(x)) across the 15 outcomes of the fitted BRT models and provides an overall sense of the variability explained by all the models combined.

Figure 8. (a–f) Order of importance of the partial dependency (y-axis) of resistance in each of the 15 models (colored lines) relative to antecedent lake characteristics (x-axis), respectively. The black line represents the results of a fitted general additive model (y ∼ s(x)) across the 15 outcomes of the fitted BRT models and provides an overall sense of the variability explained by all the models combined. The greatest effects on surface water temperature and oxygen saturation resistance following storms are driven by (a) antecedent oxygen saturation conditions. Enhanced resistance tended to partially depend on increasing oxygen saturation conditions, increasing (b) surface water temperatures and increasing (d) light availability (i.e., PAR). Diminished resistance tended to be partially dependent on (c) increasing total phosphorus concentrations, (e) Schmidt stability, and (f) increasing transparency (i.e., Secchi depth). Mean lake depth was 1% important for resistance and showed no directional effects and thus was not included in the figure.

Figure 9. Partial dependency of water temperature and oxygen saturation resistance relative to measured storm conditions. Diminished resistance was partially dependent on (a) when storms were >150 h, (b) when storms accompanied by winds from east to southwest, (c) when storms had increasing amounts of accumulated rain, (d) when increasing amounts of time had passed since the last storm analyzed, and when storms (e) occurred in the fall, and (f) took place after 2010. Resistance in the lakes tended to enhance with (g) increasing maximum wind speeds.

Antecedent lake conditions
Trophic state proxies enabled a general assessment of how surface water temperature and oxygen saturation resistance is influenced across a trophic state gradient. However, antecedent lake conditions provided a more detailed understanding of how resistance is shaped by lake conditions that closely reflect real-time conditions. Surface water temperature and oxygen saturation in lakes are driven by biogeochemical feedback and processes. The 8 lakes tended to be more resistant to displacement at ≥100% surface oxygen saturation versus <100%, which suggests that enhanced resistance is partially shaped by increased ecosystem productivity and/or more frequent oxygen equilibrium with the atmosphere, respectively (a, b, e; Tsai et al. Citation2011, Shade et al. Citation2012, Thayne et al. Citation2022). For example, when the lakes had surface oxygen saturation >100%, saturation and water temperatures were warm, and enhanced resistance was likely driven by metabolic processes and biophysical relationships influencing dissolved oxygen dynamics (Jones et al. Citation2005, Shade et al. Citation2012, Shatwell et al. Citation2016, Sullivan et al. Citation2022). Conversely, when lake surface oxygen saturation was <100%, resistance was likely more dependent on diminished primary productivity and/or changing physics and phenology such as incoming PAR, thermal stratification/mixing, and/or transparency (a, d–f). In relation to the partial effect of stratification (i.e., Schmidt stability) on resistance, 2 possibilities emerge. First, the negative correlation between Chl-a and Schmidt stability may play a critical role in shaping the observed pattern (). Second, lakes demonstrated higher resistance levels during mixing than to stratification (e). The latter argument makes sense because less change is possible, so to say, under mixed conditions than during times storms were able to breakdown stratification and draw cooler, less oxygenated waters to the surface. Overall, the importance of oxygen saturation, water temperature, and Schmidt stability suggests changes in productivity and mixing state are key factors shaping lake resistance to extreme storms under varying lake surface conditions.
Climatological setting and background seasonal variation
Lake location, regional storm conditions, and the surrounding topography can play a prominent role in shaping how lakes respond to storms. Thus, lake orientation relative to predominant wind direction of a storm is critical to determining lake response (Andersen et al. Citation2020, Thayne et al. Citation2022). The BRT analysis showed that resistance, or the peak displacement of surface water temperature and oxygen saturation, was greatest when storms were accompanied by winds out of the east to southwest and storm duration was >150 h (a–b). Winds out of the east to southwest corresponded, by chance, to the predominant wind directions and longest fetch of 7 of the 8 lakes. However, resistance was more dependent on storm duration than wind direction (–b). While we expected increasing wind speeds to diminish the resistance of the lakes, resistance surprisingly increased with increasing windspeeds (g), but this phenomenon is the background effect of the positive correlation of lake depth with wind speed. Therefore, we suspect the pattern was driven by the relationship between wind speed and increasing thermocline depth (Andersen et al. Citation2020). Changes in antecedent thermocline depth were positively related to increasing wind speeds in Lough Feeagh, such that if the thermocline was close to the surface (i.e., 4–15 m), the effect of wind speed on surface water temperature and thermal stratification was greater (Andersen et al. Citation2020). Thus, when deeper lakes have a shallow thermocline, storms have a higher likelihood to mix surface waters and break down stratification. Although we did not include thermocline depth as a predictor of resistance, we captured a similar effect by including lakes of varying depth, stratification regimes (i.e., Schmidt stability), and storm exposure. Nonetheless, if climate change indeed increases peak wind intensities, a lake’s ability to resist sharp displacements in surface water temperatures and oxygen concentrations may diminish, with cascading effects on varying lake biophysical processes (Shade et al. Citation2010, Citation2012, Tsai et al. Citation2011, Thayne et al. Citation2022). In relation to storm frequency, we found that a longer time between storms reduced surface water temperature and oxygen saturation resistance (d). As time between storms increases, lakes are more likely to strengthen stratification, and while stratification resists mixing effects, much more can be displaced under stratified versus mixed conditions (e). Thus, in areas where atmospheric stilling is expected (e.g., Lake Võrtsjärv), extreme storms may have a greater impact on the resistance of surface water dynamic as more time elapses between storms (Vautard et al. Citation2010, Woolway et al. Citation2019, Zeng et al. Citation2019, Janatian et al. Citation2020, Stetler et al. Citation2021).
Storms accompanied by high accumulations of rain resulted in diminished resistance of surface water temperature and oxygen saturation conditions across lakes (c), but some uncertainty surrounding the effects of rain remains. Our analysis of rain predictions and their impact on resistance across lakes revealed that higher rainfall levels could occasionally enhance the resistance of Lake Annie and Müggelsee (Supplemental Fig. S6). This finding confirms previous research suggesting that elevated precipitation levels may actually strengthen rather than diminish the resistance of specific lakes (Thayne et al. Citation2022). The primary effect of extreme storms and rain events on more transparent lakes tends to be changes in transparency and subsequent light availability (; Gaiser et al. Citation2009a, Citation2009b, Havens et al. Citation2011, Williamson et al. Citation2016, Kasprzak et al. Citation2017, Larsen et al. Citation2020). Extreme rainfall can strongly alter transparency and light availability in lakes via the introduction of dissolved and/or particulate matter (Williamson et al. Citation2016, Zwart et al. Citation2017, Larsen et al. Citation2020). Decreased transparency increased surface water temperature and oxygen saturation resistance to storms (f; Thayne et al. Citation2022). Aside from its direct negative effect on water transparency, rainfall leading to flooding may also increase surface temperatures and reduce the mixed depth layer, the Chl-a maximum, and deep-water oxygen concentration (Williamson et al. Citation2016), with subsequent positive or negative effects on surface temperature and oxygen resistance. Whether the effect is positive or negative depends on the lake; therefore, decreases in transparency as a result of extreme rainfall may influence the resistance of surface water temperature and oxygen saturation conditions during future storm events. However, the effect of rain and other storm effects were partially dependent on the seasonal variation and year in which peak wind speeds occurred.
After 2010, surface water temperature and oxygen saturation resistance to storms began to decrease. While Earth experienced a recovery of terrestrial wind speeds in 2010, we did not find a consistent pattern to suggest this was why we observed a decrease in resistance (Zeng et al. 2019). However, resistance was shaped across the backdrop of seasonal variation in both lake and storm conditions (e–f). Early spring and late fall in the lakes, except for subtropical Lake Annie, are dominated by mixed, cool, and clear conditions, and thus resistance is primarily shaped by physical conditions at those times of the year. Excepting Lakes Erken and Annie, cooler spring conditions were more resistant than warmer late summer to early fall conditions (e and ). In previous work, we found that when silica concentrations were low (e.g., diatom bloom presence), water temperatures were cool, storm exposure occurred in spring to midsummer, and the lake physiochemical conditions were more resistant and resilient following storms (Shatwell et al. Citation2016, Sullivan et al. Citation2022, Thayne et al. Citation2022). We suggested this finding is likely related to the strong linkage to lake diatom community and antecedent spring time thermal conditions (Thayne et al. Citation2022). Here, we found that under late spring to midsummer bloom conditions (e.g., oxygen saturation ≥ 100%), water temperature and oxygen saturation conditions were more resistant than similar conditions in late summer and fall (). A similar link may exist here, where spring to midsummer primary productivity and thermal conditions lead to enhanced resistance to storms. The dynamic between changing phenology related to differing sources of primary productivity and storm timing further confirms that the resistance dynamics of the lakes are shaped by transitory mixing dynamics and phytoplankton conditions. For example, in lakes that captured early spring and late fall storms, we saw clear changes across season, where resistance was lowest during times of seasonal mixing/low stratification and/or times of low gross primary production (). However, in Müggelsee, which tends to be mixed, we saw a less definitive resistance relationship between resistance and seasonality. Overall, results suggest the importance of changing lake and storm phenology and the potential role of climate change in impacting processes such as the timing of plankton blooms or the onset of stratification and their effect on shaping surface water temperature and oxygen saturation resistance to extreme storms ().
Figure 10. Heatmaps depicting the resistance (see legend) of each of the lakes relative to the interaction between day of peak wind speeds (x-axis) and oxygen saturation (y-axis), organized by mean annual chlorophyll a (Chl-a) concentration in the lakes (see legend). We found lakes were more resistant under cooler/less saturated spring time conditions (i.e., mixed conditions) than similar but warmer conditions in late summer to early fall, with the exception of subtropical Lakes Annie and Erken. Lake Annie shows no seasonal effect but exhibited enhanced resistance during oxygen saturation >100% and decreases through the year as hurricane season approaches. Lake Erken showed enhanced resistance during fall and late summer conditions when oxygen saturation was <100%. The overall interpretation of the interaction between day of peak wind speeds (i.e., storm seasonality) and oxygen saturation is that spring to midsummer primary productivity/lake conditions led to increased resistance of water temperature and/or oxygen saturation in the lakes. Therefore, shifts in seasonal lake and storm phenology may influence the varying lake’s abilities to resist extreme storm disturbances.

Figure 11. Diagram (inspired by Stockwell et al. Citation2020) showing how water temperature and oxygen saturation resistance and resilience following extreme storms is shaped along a trophic state gradient, conceptualizing how positive and negative correlations between (a) extreme storm conditions, (b) lake characteristics, and (c) antecedent lake conditions shape (d) short-term lake stability states across a (e–f) trophic state gradient. Extreme storm characteristics (a) had negative correlations (red dashed arrows, C1 and C2) with nontransitory and/or slow changing lake characteristics (b) and transitory antecedent lake conditions (c). Lake characteristics (b) and antecedent lake conditions (c) had both positive and negative correlations with each other (blue double-pointed dashed arrow, C3), which together with storm conditions (black dashed arrows, C2,3) shaped short-term surface lake stability states in water temperature and oxygen saturation (d; i.e., resistance and resilience). The degree to which a given lake experiences seasonally clear and turbid conditions is largely dependent on its trophic state, or whether it is (e) oligotrophic or (f) eutrophic, driven by positive and negative correlations between C2,3. Oligotrophic lake processes (f; dark blue) optimized high resistance to storms at the expense of low resilience, and while unpredictable, eutrophic lake processes (g; dark green) in this study optimized high resilience following storms at the expense of low resistance. Photo credit for (b) Lake Erken to R. Rohdin; (f) to M. Anderson, and (g) to Aerial Associates Photography, Inc. by Zachary Haslick.

Conclusion
Resistance of surface water temperature and oxygen saturation conditions to extreme storms seems to be primarily shaped by changing mean annual Chl-a and its influence on and/or relationship with other physiochemical lake characteristics. The findings emphasize the significance of physiographic and geochemical processes in determining biophysical feedbacks regulating the trophic state of lakes. Furthermore, the research reveals unpredictable variability in resilience among lake ecosystems, urging a shift in focus from solely emphasizing resilience to considering strategies that enhance ecosystem resistance, an often-overlooked aspect of disturbance ecology. The distinct variations in biophysical lake processes among lakes, including Chl-a concentration (indicative of trophic state), oxygen saturation, thermal regimes, transparency, and regional PAR, significantly influence the resistance of surface water temperature and oxygen saturation following extreme storms. Moreover, the study demonstrates the sensitivity of surface water temperature and oxygen saturation resistance to various storm conditions, including wind direction and speed, storm duration, day of peak wind speeds, levels of accumulated rain, and alterations in storm frequency. Overall, we provide a systematic, standardized, quantitative approach to unravel processes shaping lake surface water temperature and oxygen saturation resistance following extreme storms. Furthermore, synthesizing lake storm responses into standardized indices of resistance and resilience allows many other questions and lake comparisons to be explored. Given the unprecedented challenges socioecological systems such as lakes are set to encounter as a result of climate change, exploring questions that untangle the complexities of dynamic systems will begin to clarify the challenges we face in protecting lake ecosystem resistance.
Code availability
Code for quantifying resistance and resilience is available at R code: https://github.com/mthayne527/Resistance_Resilience_Estimation. Further, code can be found in the supplemental materials of the following open access publication: https://doi.org/10.1002/lno.11859.
Supplemental Material - Fig. S1
Download JPEG Image (448.2 KB)Supplemental Material - Fig. S2
Download JPEG Image (521.3 KB)Supplemental Material - Fig. S3
Download JPEG Image (278.7 KB)Supplemental Material - Fig. S4
Download JPEG Image (246.4 KB)Supplemental Material - Fig. S5
Download JPEG Image (317.2 KB)Supplemental Material - Fig. S6
Download JPEG Image (304.8 KB)Supplemental Material - Fig. S8
Download JPEG Image (292 KB)Supplemental Material - Table S1
Download MS Word (13.2 KB)Supplemental Material - Table S2
Download MS Word (13.7 KB)Supplemental Material - Table S3
Download MS Word (13.5 KB)Acknowledgements
We thank J.A. Stelzer for his insights and long discussions surrounding the topic of resilience. We also acknowledge and thank Dr. Evelyn Gaiser of Florida International University and Matthew Dietrich, the data manager at Archbold Biological Station, for providing feedback and facilitating the total phosphorus data for Lake Annie. We thank all of the big and small contributions from colleagues from the Leibniz-Institute of Freshwater Ecology and Inland Fisheries and the University of Geneva for contributing their knowledge and expertise in furthering this research. The collection and availability of data from Lake Erken has been made possible by the Swedish Infrastructure for Ecosystem Science (SITES https://www.fieldsites.se/en-GB).
Data availability
Data used in this study were collected as part of long-term programs dedicated to furthering the understanding of lake dynamics. Data for the lakes in Wisconsin, USA, are freely available and associated with the North Temperate Lakes Long-Term Ecological Research Network (https://lter.limnology.wisc.edu/data; Magnuson et al. Citation2019a, Citation2019b, Citation2020a, Citation2020b, Citation2020c, Citation2021a, Citation2021b). Data from Lake Annie was provided by the Archbold Biological Station/Reserve, also known as the MacArthur Agro-Ecology Research Center. Data for Lake Annie was provided by Evelyn Gaiser and the Archbold Biological Station. High frequency data for Lake Annie can be downloaded at (http://data.cuahsi.org/; Ames et al. 2012). Data collection on Lough Feeagh is part of the long-term monitoring program carried out by staff of the Marine Institute, Ireland. Lough Feeagh data used in this study can be found at http://data.marine.ie/geonetwork/srv/eng/catalog.search#/metadata/ie.marine.data:dataset.2817 and http://data.marine.ie/geonetwork/srv/eng/catalog.search#/metadata/ie.marine.data:dataset.3757 (de Eyeto et al. Citation2019, Citation2020). Conventional state monitoring data (monthly nutrients) from Lake Võrtsjärv are publicly available through a to the request from Estonian Environment Agency (https://keskkonnaagentuur.ee). High-frequency data collection on Lake Võrtsjärv was provided by the Center for Limnology, Estonian University of Life Sciences. Data for Müggelsee are not publicly available. Data used in this study from Lake Erken are available at https://data.fieldsites.se/portal/. Lake Võrtsjärv high-frequency data used in this study can be found at https://doi.org/10.6073/pasta/6e65d6c6e60e5722ec2c24bc60e63957.
Disclosure statement
No potential conflict of interest was reported by the author(s).
Additional information
Funding
References
- Ames DP, Horsburgh JS, Cao Y, Kadlec J, Whiteaker T, Valentine D. 2012. Hydrodesktop:web services-based software for hydrologic data discovery, download, visualization, and analysis. Environ Model Softw. 37:146–156.
- Andersen MR, de Eyto E, Dillane M, Poole R, Jennings E. 2020. 13 years of storms: an analysis of the effects of storms on lake physics on the Atlantic fringe of Europe. Water. 12(2):318.
- Batt RD, Carpenter SR, Ives AR. 2017. Extreme events in lake ecosystem time series. Limnol Oceanogr Lett. 2(3):63–69.
- Beaver JR, Crisman TL. 1989. Analysis of the community structure of planktonic ciliated protozoa relative to trophic state in Florida lakes. Hydrobiologia. 174(3):177–184.
- Biddanda B, Ogdahl M, Cotner J. 2001. Dominance of bacterial metabolism in oligotrophic relative to eutrophic waters. Limnol Oceanogr. 46(3):730–739.
- Calderó-Pascual M, de Eyto E, Jennings E, Dillane M, Andersen MR, Kelly S, Wilson HL, Mccarthy V. 2020. Effects of consecutive extreme weather events on a temperate dystrophic lake: a detailed insight into physical, chemical and biological responses. Water. 12(5):1411–1434.
- Cantarello E, Newton AC, Martin PA, Evans PM, Gosal A, Lucash MS. 2017. Quantifying resilience of multiple ecosystem services and biodiversity in a temperate forest landscape. Ecol Evol. 7(22):9661–9675.
- Carlson RE. 1977. A trophic state index for lakes. Limnol Oceanogr. 22(2):361.
- Carpenter SR. 1987. Regulation of lake primary productivity by food web structure. Ecology. 68(6):1863–1876.
- Carpenter SR, Caraco NF, Correll DL, Howarth RW, Sharpley AN, Smith VH. 1998. Nonpoint pollution of surface waters with phosphorus and nitrogen. Ecol Appl. 8(3):559–568.
- Carpenter SR, Cole JJ, Hodgson JR, Kitchell JE, Pace ML, Bade D, Cottinngham KL, Essington TE, Houser JN, Schindler DE. 2001. Trophic cascades, nutrients, and lake productivity: whole-lake experiments. Ecol Monogr. 71(2):163–186.
- Carpenter SR, Cottingham KL. 1997. Resilience and restoration of lakes. Ecol Soc. 1(1):2.
- de Eyto E, Dillane M, Cooney J, Hughes P, Murphy M, Nixon P, Sweeney D, Poole R, Rouen M. 2019. Water quality and meteorological data from the Lough Feeagh automatic water quality monitoring station (AWQMS), 2004–2019. Ireland: Marine Institute; Dundalk Institute of Technology. http://data.marine.ie/geonetwork/srv/eng/catalog.search#/metadata/ie.marine.data:dataset.3757
- de Eyto E, Dillane M, Moore T, Wilson H, Cooney J, Hughes P, Murphy M, Nixon P, Sweeney D, Poole R. 2020. Lough Feeagh water temperature profiles. Ireland: Marine Institute; Dundalk Institute of Technology. http://data.marine.ie/geonetwork/srv/eng/catalog.search#/metadata/ie.marine.data:dataset.2817
- de Eyto E, Jennings E, Ryder E, Sparber K, Dillane M, Dalton C, Poole R. 2016. Response of a humic lake ecosystem to an extreme precipitation event: physical, chemical, and biological implications. Inland Waters. 6(4):483–498.
- Doubek JP, Anneville O, Dur G, Lewandowska AM, Patil VP, Rusak JA, Salmaso N, Seltmann CT, Straile D, Urrutia-Cordero P, Venail P. 2021. The extent and variability of storm-induced temperature changes in lakes measured with long-term and high-frequency data. Limnol Oceanogr. 66(5):1979–1992.
- Elith J, Leathwick JR, Hastie T. 2008. A working guide to boosted regression trees. J Anim Ecol. 77(4):802–813.
- Foley JA, DeFries R, Asner GP, Barford C, Bonan G, Carpenter SR, Chapin FS, Coe MT, Daily GC, Gibbs HK, Helkowski JH. 2005. Global consequences of land use. Science. 309(5734):570–574.
- Gaiser EE, Deyrup ND, Bachmann RW, Battoe LE, Swain HM. 2009a. Effects of climate variability on transparency and thermal structure in subtropical, monomictic Lake Annie, Florida. Fund Appl Limnol. 175(3):217–230.
- Gaiser EE, Deyrup ND, Bachmann RW, Battoe LE, Swain HM. 2009b. Multidecadal climate oscillations detected in a transparency record from a subtropical Florida lake. Limnol Oceanogr. 54(6):2228–2238.
- Gilleland E, Katz RW. 2006. Analyzing seasonal to interannual extreme weather and climate variability with the extremes toolkit. 86th AMS annual meeting, Atlanta, GA, USA, 29 Jan–2 Feb 2006 [Paper P1.51]. https://ams.confex.com/ams/pdfpapers/100686.pdf
- Gilleland E, Katz RW. 2016. Extremes 2.0: an extreme value analysis package in R. J Stat Softw. 72(8):1–39.
- Guillot E, Hinsinger P, Dufour L, Roy J, Bertrand I. 2019. With or without trees: resistance and resilience of soil microbial communities to drought and heat stress in a Mediterranean agroforestry system. Soil Biol Biochem. 129:122–135.
- Gunderson LH. 2000. Ecological resilience - in theory and application. Annu Rev Ecol Syst. 31:425–439.
- Havens KE, Beaver JR, Casamatta DA, East TL, James RT, McCormick P, Phlips EJ, Rodusky AJ. 2011. Hurricane effects on the planktonic food web of a large subtropical lake. J Plankton Res. 33(7):1081–1094.
- Hijmans JR, Phillips S, Leathwick J, Elith J. 2017. Dismo: species distribution modeling. R Package version. 1:1–4. https://CRAN.R-project.org/package=dismo
- Holling CS. 1973. Resilience and stability of ecological systems. Annu Rev Ecol Syst. 4:1–23.
- Holling CS. 1996. Engineering resilience versus ecological resilience. In: Schulze P, editor. Engineering within ecological constraints. Washington (DC): National Academy of Engineering, National Academies Press; p. 31–44.
- Hosking JRM. 1990. L-Moments: analysis and estimation of distributions using linear combinations of order statistics. J R Stat Soc Series B Stat Methodol. 52(1):105–124.
- Hyndman RJ, Khandakar Y. 2008. Forecast: forecasting functions for time series and linear models. J Stat Softw. 27(3):1–22.
- [IPCC] Intergovernmental Panel on Climate Change. 2012. Managing the risks of extreme events and disasters to advance climate change adaptation. In: A special report of Working Groups I and II of the Intergovernmental Panel on Climate change. Cambridge (UK): Cambridge University Press.
- Janatian N, Olli K, Cremona F, Laas A, Nõges P. 2020. Atmospheric stilling offsets the benefits from reduced nutrient loading in a large shallow lake. Limnol Oceanogr. 65(4):717–731.
- Jane SF, Hansen GJ, Kraemer BM, Leavitt PR, Mincer JL, North RL, Pilla RM, Stetler JT, Williamson CE, Woolway RI, Arvola L. 2021. Widespread deoxygenation of temperate lakes. Nature. 594(7861):66–70.
- Jennings E, Jones S, Arvola L, Staehr PA, Gaiser E, Jones ID, Weathers KC, Weyhenmeyer GA, Chiu CY, de Eyto E. 2012. Effects of weather-related episodic events in lakes: an analysis based on high-frequency data. Freshw Biol. 57(3):589–601.
- Jöhnk KD, Huisman J, Sharples J, Sommeijer B, Visser PM, Stroom JM. 2008. Summer heatwaves promote blooms of harmful cyanobacteria. Glob Change Biol. 14(3):495–512.
- Jones I, George G, Reynolds C. 2005. Quantifying effects of phytoplankton on the heat budgets of two large limnetic enclosures. Freshw Biol. 50(7):1239–1247.
- Kasprzak P, Shatwell T, Gessner MO, Gonsiorczyk T, Kirillin G, Selmeczy G, Padisák J, Engelhardt C. 2017. Extreme weather event triggers cascade towards extreme turbidity in a clear-water lake. Ecosystems. 20(8):1624–1639.
- Kassambara A. 2020. ggpubr: ‘ggplot2’ based publication ready plots. R package version 0.2.5.
- Kraemer BM, Pilla RM, Woolway RI, Anneville O, Ban S, Colom-Montero W, Devlin SP, Dokulil MT, Gaiser EE, Hambright KD, Hessen DO. 2021. Climate change drives widespread shifts in lake thermal habitat. Nat Clim Change. 11(6):521–529.
- Laib M, Kanevski M. 2016. Spatial modelling of extreme wind speed distributions in Switzerland. Energy Procedia. 97:100–107.
- Larsen ML, Baulch HM, Schiff SL, Simon DF, Sauvé S, Venkiteswaran JJ. 2020. Extreme rainfall drives early onset cyanobacterial bloom. Facets. 5(1):899–920.
- Lehmann J, Coumou D, Frieler K. 2015. Increased record-breaking precipitation events under global warming. Clim Change. 132(4):501–515.
- Linz AM, Crary BC, Shade A, Owens S, Gilbert JA, Knight R, McMahon KD. 2017. Bacterial community composition and dynamics spanning five years in freshwater bog lakes. MSphere. 2(3):e00169-17.
- Livingstone DM. 2003. Impact of secular climate change on the thermal structure of a large temperate Central European lake. Clim Change. 57:205–225.
- Magnuson J, Carpenter S, Stanley E. 2019a. North Temperate Lakes LTER: chemical limnology of primary study lakes: major ions 1981; current ver. 34. Environmental Data Initiative. doi:10.6073/pasta/a457e305538a0d8e669b58bb6f35721f
- Magnuson J, Carpenter S, Stanley E. 2019b. North Temperate Lakes LTER: high frequency meteorological and dissolved oxygen data – Trout Lake buoy 2004; current ver. 38. Environmental Data Initiative. doi:10.6073/pasta/ca866e3441ce3ed20f79bf43741bf181
- Magnuson J, Carpenter S, Stanley E. 2020a. North Temperate Lakes LTER: high frequency data: meteorological, dissolved oxygen, chlorophyll, phycocyanin – Lake Mendota buoy 2006; current ver. 31. Environmental Data Initiative. doi:10.6073/pasta/c03b39550e79d002d82a2281f8546c78
- Magnuson J, Carpenter S, Stanley E. 2020b. North Temperate Lakes LTER: high frequency water temperature data – Lake Mendota buoy 2006; current ver. 29. Environmental Data Initiative. doi:10.6073/pasta/8ceff296ad68fa8da6787076e0a5d992
- Magnuson J, Carpenter S, Stanley E. 2020c. North Temperate Lakes LTER: high frequency water temperature data – Trout Lake buoy 2004; current ver. 26. Environmental Data Initiative. doi:10.6073/pasta/6ad563f6059685a116754ff3b391d15e
- Magnuson J, Carpenter S, Stanley E. 2021a. North Temperate Lakes LTER: high frequency meteorological and dissolved oxygen data – Trout Bog buoy 2003–2014; ver. 22. Environmental Data Initiative. doi:10.6073/pasta/3e63d39d6a81de03f214808d0138bc1d
- Magnuson J, Carpenter S, Stanley E. 2021b. North Temperate Lakes LTER: high frequency water temperature data – Trout Bog buoy 2003–2014; ver. 27. Environmental Data Initiative. https://doi.org/10.6073/pasta/db38add6b67134d205086de3d7366001
- Meerhoff M, Audet J, Davidson TA, de Meester L, Hilt S, Kosten S, Liu Z, Mazzeo N, Paerl H, Scheffer M, Jeppesen E. 2022. Feedback between climate change and eutrophication: revisiting the allied attack concept and how to strike back. Inland Waters. 12(2):187–204.
- Mesman JP, Ayala AI, Goyette S, Kasparian J, Marcé R, Markensten H, Stelzer JAA, Thayne MW, Thomas MK, Pierson DC, Ibelings BW. 2021a. Drivers of phytoplankton responses to summer wind storms in a stratified lake: a modelling study. Limnol Oceanogr. 67(4):856–873.
- Mesman JP, Stelzer JAA, Dakos V, Goyette S, Jones ID, Kasparian J, McGinnis D F, Ibelings BW. 2021b. The role of internal feedbacks in shifting deep lake mixing regimes under a warming climate. Freshw Biol. 66(6):1021–1035.
- Mittelbach GG, Turner AM, Hall DJ, Rettig JE, Osenberg CW. 1995. Perturbation and resilience: a long-term, whole-lake study of predator extinction and reintroduction. Ecology. 76(8):2347–2360.
- Murphy S. 2012. Foundations of ecological resilience. Q Rev Biol. 87(1):77–78.
- National Centers for Environmental Information. 2022. FAQ climate data online (CDO) [accessed 27 Jan 2022]. Ashville (NC): National Climatic Data Center (NCDC). https://www.ncdc.noaa.gov/cdo-web/faq
- Nieto K, Mélin F. 2017. Variability of chlorophyll-a concentration in the Gulf of Guinea and its relation to physical oceanographic variables. Prog Oceanogr. 151:97–115.
- Nõges P, Nõges T, Ghiani M, Paracchini B, Grande JP, Sena F. 2011. Morphometry and trophic state modify the thermal response of lakes to meteorological forcing. Hydrobiologia. 667(1):241–254.
- Orwin KH, Wardle DA. 2004. New indices for quantifying the resistance and resilience of soil biota to exogenous disturbances. Soil Biol Biochem. 36(11):1907–1912.
- Paerl HW, Huisman J. 2009. Climate change: a catalyst for global expansion of harmful cyanobacterial blooms. Environ Microbiol Rep. 1(1):22–37.
- Palutikof JP, Brabson BB, Lister DH, Adcock ST. 1999. A review of methods to calculate extreme wind speeds. Meteorol Appl. 6(2):119–132.
- Perga ME, Bruel R, Rodriguez L, Guénand Y, Bouffard D. 2018. Storm impacts on alpine lakes: antecedent weather conditions matter more than the event intensity. Glob Change Biol. 24(10):5004–5016.
- Pimm SL. 1984. The complexity and stability of ecosystems. Nature. 307(5949):321–326.
- R Core Team. 2019. R: a language and environment for statistical computing. Vienna (Austria): R Foundation for Statistical Computing. https://www.R-project.org/
- Rigosi A, Carey CC, Ibelings BW, Brookes JD. 2014. The interaction between climate warming and eutrophication to promote cyanobacteria is dependent on trophic state and varies among taxa. Limnol Oceanogr. 59(1):99–114.
- Sager J, McFarlane JC. 1997. Radiation. In: Langhans RW, Tibbitts TW, editors. Plant growth chamber handbook: radiation. Iowa State University: North Central Regional Research Publication No. 340. Iowa Agriculture and Home Economics Experiment Station Special Report no. 99; Cambridge (UK): Cambridge University Press; p. 1–29.
- Scheffer M, Hosper SH, Meijer ML, Moss B, Jeppesen E. 1993. Alternative equilibria in shallow lakes. Trends Ecol Evol. 8(8):275–279.
- Shade A, Chiu CY, McMahon KD. 2010. Seasonal and episodic lake mixing stimulate differential planktonic bacterial dynamics. Microb Ecol. 59(3):546–554.
- Shade A, Read JS, Youngblut ND, Fierer N, Knight R, Kratz TK, Lottig NR, Roden EE, Stanley EH, et al. 2012. Lake microbial communities are resilient after a whole-ecosystem disturbance. ISME J. 6(12):2153–2167.
- Shatwell T, Adrian R, Kirillin G. 2016. Planktonic events may cause polymictic-dimictic regime shifts in temperate lakes. Sci Rep. 6, 24361.
- SMHI Centre. 2022. Utforskaren - Öppna data SMHI [accessed 27 Jan 2022]. https://www.smhi.se/data/utforskaren-oppna-data/
- Stelzer JAA, Mesman JP, Gsell AS, de Senerpont Domis LN, Visser PM, Adrian R, Ibelings BW. 2022. Phytoplankton responses to repeated pulse perturbations imposed on a trend of increasing eutrophication. Ecol Evol. 12(3):e8675.
- Stetler JT, Girdner S, Mack J, Winslow LA, Leach TH, Rose KC. 2021. Atmospheric stilling and warming air temperatures drive long-term changes in lake stratification in a large oligotrophic lake. Limnol Oceanogr. 66(3):954–964.
- Stockwell JD, Doubek JP, Adrian R, Anneville O, Carey CC, Carvalho L, De Senerpont Domis LN, Dur G, Frassl MA, Grossart HP, Ibelings BW. 2020. Storm impacts on phytoplankton community dynamics in lakes. Glob Change Bio. 26(5):2756–2784.
- Sullivan KL, Gaiser EE, Swain HM. 2022. Dissolved organic carbon as a driver of seasonal and multiyear phytoplankton assembly oscillations in a subtropical monomictic lake. Limnol Oceanogr. 67(S1):S416–S429.
- Thayne MW, Kraemer BM, Mesman JP, Ibelings BW, Adrian R. 2022. Antecedent lake conditions shape resistance and resilience of a shallow lake ecosystem following extreme wind storms. Limnol Oceanogr. 67(S1):S101–S120.
- Tsai JW, Kratz TK, Hanson PC, Kimura N, Liu WC, Lin FP, Chou HM, Wu JT, Chiu CY. 2011. Metabolic changes and the resistance and resilience of a subtropical heterotrophic lake to typhoon disturbance. Can J Fish Aquat Sci. 68(5):768–780.
- Vautard R, Cattiaux J, Yiou P, Thépaut JN, Ciais P. 2010. Northern Hemisphere atmospheric stilling partly attributed to an increase in surface roughness. Nat Geosci. 3(11):756–761.
- Wagner C, Adrian R. 2009. Cyanobacteria dominance: quantifying the effects of climate change. Limnol Oceanogr. 54(6 Part 2):2460–2468.
- Walker BH, Langridge JL, McFarlane F. 1997. Resilience of an Australian savanna grassland to selective and non-selective perturbations. Austral Ecol. 22(2):125–135.
- Walker BH, Ludwig D, Holling CS, Peterman RM. 1981. Stability of semi-arid savanna grazing systems. J Ecol. 69(2):473–498.
- Webster PJ, Holland GJ, Curry JA, Chang HR. 2005. Atmospheric science: changes in tropical cyclone number, duration, and intensity in a warming environment. Science. 309(5742):1844–1846.
- Wickham H. 2016. ggplot2: elegant graphics for data analysis. New York (NY): Springer-Verlag.
- Williamson CE, Overholt EP, Brentrup JA, Pilla RM, Leach TH, Schladow SG, Warren JD, Urmy SS, Sadro S, Chandra S, Neale PJ. 2016. Sentinel responses to droughts, wildfires, and floods: effects of UV radiation on lakes and their ecosystem services. Front Ecol Environ. 14(2):102–109.
- Winslow L, Read J, Woolway R, Brentrup J, Leach T, Zwart J, Albers S, Collinge D. 2018. rLakeAnalyzer: lake physics. R package version 1.11.4. https://CRAN.R-project.org/package=rLakeAnalyzer
- Woolway RI, Jennings E, Shatwell T, Golub M, Pierson DC, Maberly SC. 2021a. Lake heatwaves under climate change. Nature. 589(7842):402–407.
- Woolway RI, Kraemer BM, Lenters JD, Merchant CJ, O’Reilly CM, Sharma S. 2020. Global lake responses to climate change. Nat Rev Earth Environ. 1(8):388–403.
- Woolway RI, Merchant CJ. 2019. Worldwide alteration of lake mixing regimes in response to climate change. Nat Geosci. 12(4):271–276.
- Woolway RI, Merchant CJ, van den Hoek J, Azorin-Molina C, Nõges P, Laas A, Mackay EB, Jones ID. 2019. Northern Hemisphere atmospheric stilling accelerates lake thermal responses to a warming world. Geophys Res Lett. 46(21):11983–11992.
- Woolway RI, Sharma S, Weyhenmeyer GA, Debolskiy A, Golub M, Mercado-Bettín D, Perroud M, Stepanenko V, Tan Z, Grant L, Ladwig R. 2021b. Phenological shifts in lake stratification under climate change. Nat Commun. 12(1):2318.
- Zeng Z, Ziegler AD, Searchinger T, Yang L, Chen A, Ju K, Piao S, Li LZ, Ciais P, Chen D, Liu J. 2019. A reversal in global terrestrial stilling and its implications for wind energy production. Nat Clim Change. 9(12):979–985.
- Zhang X, Wan H, Zwiers FW, Hegerl GC, Min SK. 2013. Attributing intensification of precipitation extremes to human influence. Geophys Res Lett. 40(19):5252–5257.
- Zhu M, Paerl HW, Zhu G, Wu T, Li W, Shi K, Zhao L, Zhang Y, Qin B, Caruso AM. 2014. The role of tropical cyclones in stimulating cyanobacterial (Microcystis spp.) blooms in hypertrophic Lake Taihu, China. Harmful Algae. 39:310–321.
- Zwart JA, Sebestyen SD, Solomon CT, Jones SE. 2017. The influence of hydrologic residence time on lake carbon cycling dynamics following extreme precipitation events. Ecosystems. 20(5):1000–1014.