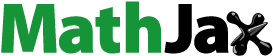
ABSTRACT
Lakes pivotal for recreation and economically relevant activities are often remote and not well studied, which hinders the application of predictive lake models for their management. Here, we provide an approach to simulate—by means of the process-oriented model MyLake—water temperature, ice cover duration, dissolved oxygen, and light attenuation in 198 data-poor lakes based on parameters obtained for a subgroup of 12 data-rich lakes and morphometric data. Specifically, the model is first calibrated using a genetic algorithm on well-studied lakes. Simple relationships between the fitted parameters and lake-catchment morphometric properties are then derived, and the results of simulations using fitted and derived parameters are compared. The loss in goodness-of-fit, expressed as root mean square error (RMSE) incurred by using estimated rather than calibrated parameters, is 0.17 °C for water temperature and 0.82 mg L−1 for dissolved oxygen. These general relationships are then used to provide the model parameters for 198 data-poor lakes distributed throughout Sweden and to model these lakes. Overall, this proof of concept allows simulating lakes selected based on their relevance for lake management rather than based on the availability of extensive field datasets.
Introduction
Ecosystem services provided by lakes (Dodds et al. Citation2013) are defined by a wide array of morphological variables such as area, bathymetry, and fetch (Zentner et al. Citation2019) and physicochemical variables such as water temperature, dissolved oxygen (DO) concentration, and water clarity (Adrian et al. Citation2009, Stasko et al. Citation2012, Holubová et al. Citation2021). These variables respond to external drivers such as air temperature, ice cover, and water colour, which are closely linked to both weather and climate. Rapid changes in these variables have been observed, particularly in the boreal lakes (Adrian et al. Citation2009, Stasko et al. Citation2012). As such, climate change leads to warmer, oxygen-depleted (Schindler Citation2009, O’Reilly et al. Citation2015), and darker lakes (Van Dorst et al. Citation2020).
Warmer air temperature increases heating of the surface water and causes stronger thermal stratification, with a sharper thermocline reducing heat transfer to the hypolimnion and resulting in a warmer epilimnion and possibly a cooler hypolimnion (Magee et al. Citation2018). An increasing disparity in temperature between the epilimnion and hypolimnion has thus been reported (Kraemer et al. Citation2015, Richardson et al. Citation2017, Bartosiewicz et al. Citation2019, Pilla et al. Citation2020), along with changes in the timing and duration of seasonal stratification, namely earlier spring and later autumn turnovers (Magee et al. Citation2018). Finally, warmer temperatures also impact ice cover duration (Bartosiewicz et al. Citation2020), with a cascading effect on light and DO (discussed later).
The ice break-up and freeze-up dates influence the heat regulation of the water column and the timing of spring and autumn turnover (Beier et al. Citation2012). The duration of ice cover and timing of turnover also influence light penetration in spring as well as oxygen availability from changes in diffusion and photosynthesis (Bouffard et al. Citation2019). Ice cover duration is thus linked to the ventilation of the water column in early spring and the availability of oxygen after autumn turnover (Pilla and Williamson Citation2021). Longer open-water periods may increase oxygen input from the atmosphere (e.g., Couture et al. Citation2015) and promote autotrophy. Because ice phenology is strongly related to air temperature, cloud cover, wind, and precipitation, it is therefore also sensitive to climate change. A noticeable reduction in ice cover duration has been reported for lakes in the Northern Hemisphere over the last century (Woolway et al. Citation2020).
The increase of coloured dissolved organic matter (CDOM) in lakes reduces light availability (Thrane et al. Citation2014), which is antagonistic to the effect of shorter ice cover duration. As such, increasing CDOM also affects heat content and distribution in the lake (Solomon et al. Citation2015, Knoll et al. Citation2018, Bartosiewicz et al. Citation2019, Pilla et al. Citation2020) because more heat is absorbed in a darker epilimnion, favouring a sharper thermocline. The observed increase in CDOM in boreal lakes, referred to as “browning,” has been shown to thin the photic zone and shift predatory fish optical habitat (Weidel et al. Citation2017, Van Dorst et al. Citation2020). Finally, increasing dissolved organic carbon (DOC) loads along with CDOM influences DO concentration (Couture et al. Citation2015). DOC, comprising labile organic matter, fuels microbial metabolism and increases DO consumption in both the water column and the sediment. Longer stratification increases anoxia as the presence of the thermocline hinders bottom water ventilation, highlighting a synergy between air temperature and DOC in enabling oxygen depletion.
The response of water temperature, DO, ice cover duration, and water colour to ongoing environmental and climate change is a current preoccupation of managers who need to focus limited resources on lakes most likely to respond positively to conservation efforts. The preservation of cold-water fish habitats, for instance, provides an impetus for management efforts (Stefan et al. Citation2001, Kraemer et al. Citation2021). Such fish are found in boreal lakes and seek cold, well-oxygenated (Jacobson et al. Citation2010), and well-lit waters (Stasko et al. Citation2012). During summer, the habitat of those fish may be markedly reduced as the temperature preference limits the habitat to the hypolimnetic layers where the DO concentration is likely to diminish with increasing warming. Ultimately, the volume of suitable habitat may shrink to lethal levels (Nürnberg Citation1995). Reduced fish habitat impacts not only their biomass, but also the ecosystem components that depend on top-down control, such as community composition, prey abundance, and the biomass of primary producers (Karlsson et al. Citation2009, Vasconcelos et al. Citation2019).
Assessing the impact of climate changes on the availability of oxythermal (Stefan et al. Citation1995, Magnuson et al. Citation1997, Jacobson et al. Citation2010) and optical (Stasko et al. Citation2012, Weidel et al. Citation2017, Keyler et al. Citation2019, Van Dorst et al. Citation2020) habitats helps inform science-based management decisions relevant for both recreational and economical purposes. This assessment thus calls for accessing multiple lakes simultaneously, especially when considering the ongoing impact of climate change.
Process-oriented lake models are powerful tools to forecast the evolution of lake dynamics under climate change. Modeling of lakes at the regional scale has been performed using 1D lake models (Moore et al. Citation2021). The hydrodynamic modules of such models can be transferred from site to site without extensive site-specific calibration (Winslow et al. Citation2017), which likely is not the case for the prediction of other variables such as DO because their calibration generally requires lake-specific field data (Fang and Stefan Citation2009, Fang et al. Citation2012). These data are typically not available at the regional scale (Robson Citation2014), where dataset availability ranges from minimal, such as only morphology and sparse chemistry surveys (Jacobson et al. Citation2010, Van Zuiden et al. Citation2016), to comprehensive, where moored instruments provide high-density time series (e.g., Fang and Stefan Citation2009, Fang et al. Citation2012, Couture et al. Citation2015).
We propose an approach to model these variables at the regional scale using the sparse and/or meagre available data to provide a proof-of-concept to enable lake managers and governmental agencies to utilize datasets comprising lakes with sparse data and lakes with extensive time series. We describe the approach enabling the modeling of a large number of lakes, not only for water temperature and ice cover duration but also for state variables requiring lake-specific model calibration, namely DO and light, using a group of data-rich and data-poor lakes from throughout Sweden. First, the data from 12 data-rich lakes were used for model calibration, and the model parameters for 198 data-poor lakes were then derived from a simple statistical method, enabling the simulation of their physicochemical dynamics under climate change scenarios.
Methods
Dataset
We chose data from 210 dimictic, oligotrophic, boreal lakes distributed across Sweden. These lakes were selected based on the coexistence between Arctic char (Salvelinus alpinus) and pike (Esox lucius) as well as their relevance for recreational fisheries. They span a large range of areas, from 0.4 to 1900 km2. The morphometric and catchment data, such as maximum and average lake depths, lake and catchment area, and residence time, were retrieved from the Swedish Meteorological and Hydrological Institute (SMHI Citation2017) database. For modelling purposes, the area at each 1 m depth increment (Az, m2) was calculated after by multiplying the lake surface area (AL, m2) with the proportional area at the depth (z, m) using a coefficient estimating the basin shape (S) based on the maximum (zmax, m) and mean depth (zmean, m) (Lester et al. Citation2004):
(1)
(1) where
(2)
(2) Finally, sediment area (AS, m2) was estimated as the sum of the difference in the horizontal area between 2 consecutive depth levels (Saloranta and Andersen Citation2007). Within the 210 lakes, only 12 had temperature, light, and oxygen profiles or time series. Model calibration was thus constrained by the availability of field data. The 12 lakes with data are hereafter referred to as the “focus lakes,” which are distributed throughout the study area () and present a range of lake shape, depth, area, and residence time ().
Field and hydrographic data
Volumetric inflow rates and temperature of inflowing waters for all lakes were obtained from the SMHI databases of simulated runoff into Swedish lakes. Vertical profiles of water temperature and DO concentrations, along with Secchi depth (SD), were measured as part of the monitoring of the 12 focus lakes. The data cover at least 10 years from 1989 to 2017, with datasets ranging from 48 days of data (lake #1) to 7017 days (lake #12). The other lakes did not have sufficient coverage of temperature, oxygen, and SD data for this period. Ice-on and ice-off dates were available for 52 of the 210 lakes, including the 12 focus lakes, for different durations between 1866 and 2011 (SMHI Citation2019). For most of the lakes, observations of temperature and DO were available only for the upper part of the water column, which is above the estimated seasonal thermocline.
Figure 1. Location of the 210 study lakes throughout Sweden. Focus lakes and data-poor lakes are identified with black and white diamonds, respectively.

Table 1. Morphometric characteristics of the 12 focus lakes. The coordinates indicate the main inflow of the lakes.
Meteorological forcing data
Meteorological forcing data were retrieved from the World Climate Research Program Coordinated Regional Downscaling Experiment (EURO-CORDEX) project (Jacob et al. Citation2014) at 0.11° resolution, centred on the European domain of ∼27–72°N, ∼2°W–45°E and available at the daily time scale directly usable by the lake models. We used time series from the CORDEX data for each lake and its catchment. If the lake or catchment area was entirely contained within one of the CORDEX pixels, then the model used the data from that pixel; if the lake or catchment geometry covered multiple CORDEX pixels, we used the fractional area of the lake in each pixel, ignoring islands, and calculated a weighted mean.
We used 6 global climate models (GCMs) and corresponding regional circulation models (RCMs) for Europe that comprise a subset of CORDEX data (Supplemental Table S1), omitting 1 or 2 simulation(s) for a given period and greenhouse gas (GHG) scenario. For instance, daily near-surface relative humidity was unavailable for the Max Planck Institute (MPI) model and was set to 50% throughout, while cloud cover and near-surface humidity were not available for the Institut Pierre-Simon Laplace (IPSL) model and were set to 0.65% and 50%, respectively (Karlsson Citation2003; description of the GCM–RCM combinations in Jacob et al. Citation2014). We used 5 decades of data covering historical periods (1971–1980 and 2001–2010) and free-running simulated decades (2031–2040, 2061–2070, and 2091–2010). The global climate models were driven by 2 separate trajectories of GHG concentrations, Representative Concentration Pathways RCP4.5 and RCP8.5 representing the moderate and extreme GHG concentration trajectories adopted by the United Nations Inter-governmental Panel on Climate Change (IPCC) for its 5th Assessment Report (Moss et al. Citation2008, Meinshausen et al. Citation2011, Stocker Citation2014). These RCPs correspond respectively to the stabilization of radiative forcing after the 21st century at 4.5 W m−2 or a rising radiative forcing crossing 8.5 W m−2 by the end of the 21st century.
Lake modeling
The 1D process-based lake model MyLake (Saloranta and Andersen Citation2007) was used to simulate time series of daily water temperature, light penetration, and DO at 1 m vertical resolution for each study lake as well as daily ice cover thickness. The model focuses on in-lake physical processes (diurnal vertical distribution of thermal energy, surface heat balances, shortwave radiation penetration, wind mixing, ice formation, and break-up) and a simplified oxygen module comprising water column and sediment oxygen demand equations (Stefan and Fang Citation1994, Kiuru et al. Citation2018), with the latter replacing the sediment diagenesis module (Couture et al. Citation2015). This version of the model uses meteorological and hydrological input variables () and simulates time series of the following state variables: water temperature, ice and snow thickness, DO and DOC concentrations, and photosynthetically active radiation (PAR) irradiance (400–700 nm).
Table 2. Daily input variables used by the MyLake model.
Calibration of the focus lakes
The calibration procedure allowed the following model parameters to vary from their initial values: PAR light attenuation coefficient (SWAb1, 1 m−1), non-PAR light attenuation coefficient (SWAb0, 2.5 m−1), wind sheltering coefficient (c_shelter, 0.7), and oxygen demand coupled with the decomposition rate of organic carbon in the water column (kBOD, 0.1 d−1) and in the sediment (kSOD, 500 mg m−2 d−1) (equations pertaining to sensitive parameters in Supplemental Material). Further, the inflow scalers were also allowed to vary to approximate inflow volume (IscV, 1), temperature (IscT, 0), DO (IscO, 1), and DOC (IscDOC, 1). Note that values for the diffusion parameter ak during open water and ice cover periods, which is used with the stratification stability to estimate the vertical diffusion coefficient (K; m2 d−1), were not obtained from the calibration but calculated from the lake surface area using the optional default parameterisations included with MyLake.
The model calibration, or determination of the model parameter values, for the 12 focus lakes was done using the genetic algorithm (GA) tool provided by the Matlab global optimization toolbox (MATLAB Citation2019) with a population size of 48 parallel model runs and a maximum of 60 generations, leading to 2880 model runs per lake. The parameter values were initialized with values from the previously calibrated MyLake application at the boreal lake Langtjern (De Wit et al. Citation2018) and then allowed to vary with the automated calibration procedure. Calibration was done using the historical period 2001–2010, with the normalized RMSE used as a performance metric (Los and Blaas Citation2010). Performance against ice observations was considered for validation only using the additional historical period of 1971–1980. This calibration method provided the values for the parameters mentioned earlier ().
Table 3. Relations between parameters and predictors: the equations used for each parameter with R2 and p-value.
Derivation of model parameters for the data-poor lakes
The parameter values for the lakes with no or sparse data were determined using the following procedure. First, we determined relationships between lake and catchment characteristics (), which included the predictors and the values of the optimized parameters of the 12 focus lakes (i.e., the response variable). To select the potential predictor candidates, the variance inflation factor (VIF) was used to evaluate the possible collinearity between predictors. Six of the 10 parameters listed earlier were considered potential predictors with a threshold of VIF < 7. A stepwise regression approach (SR) was done by using the step function in base R (R Core Team Citation2020) to generate generalized linear models. Predictors were either log-transformed, taken as a square root (for areas), or left as-is when needed for normalization and to reduce heteroscedasticity (). We ranked relationships using the Akaike information criterion (AIC) and the Bayesian information criterion (BIC), metrics developed to provide an objective estimate of the model error (lower values are better model fit). In addition to R2 and RMSE, the AIC and BIC criteria include a penalty that increases the error when including additional terms in the regression. Thus, they help choose the more parsimonious model, which is a sensible approach (Hastie et al. Citation2018). According to the handbook of statistics (Narisetty Citation2020), AIC is a function of residual sums of square of the corresponding model and of a term that penalizes the model having a higher complexity. BIC builds on AIC but imposes a larger penalty for higher complexity models (formulation details in Narisetty (Citation2020). For each parameter, we selected the models that ranked first according to both criteria, as calculated in R. The final equation for each parameter was finally selected considering the RMSE, p-value, and R2 for each comparison. This exercise yielded equations relating lake and catchment characteristics with model parameters obtained for the 12 focus lakes. Except for the predictor of c_shelter, none of the models had strong indications of violating the standard assumptions of linearity (Supplemental Fig. S1). We then used those equations, with catchment characteristics as input parameters, to generate lake-specific values for the chosen model parameters ().
Simulations
Using the parameter values obtained from the SR equations, state variables for the 210 lakes were simulated at a daily time step for 5 decades (2 of which were historical periods and 3 were future periods) and the 2 climate scenarios RCP 4.5 and RCP 8.5 using projections of the 6 CORDEX climate models (Supplemental Table S1). The calculations led to 10 080 decadal simulations comprising the following outputs: vector of ice cover presence or absence, matrices (day, depth) of water temperature (°C), DO concentrations (mg L−1), and total photon flux (µmol m−2 s−1). Prescribed initial conditions were set to isothermal water temperature and DO at saturation (Golub et al. Citation2022).
Results
Water temperature, DO concentration, and SD in the 12 focus lakes
In all 12 focus lakes, water temperature followed a dimictic seasonal pattern, with the warmer temperature at the bottom of the lake during winter, an isothermal profile during seasonal mixing, and warmer temperatures at the surface during summer. Smaller lakes had larger seasonal variations in bottom temperature than deeper lakes. Field data from lake #2 () show that over the whole dataset, lake temperatures varied from 3.7 °C at the bottom to 22.9 °C at the surface in summer and from 4.2 °C at the bottom to 0.2 °C at the surface in winter. Overall, DO concentration was higher in winter than summer (), varying between 4.37 and 12.62 mg L−1 in summer and 5.35 and 15.13 mg L−1 in winter. The 12 focus lakes encompass both clear and coloured lakes, with the deepest SD at 17.5 m in lake #12 and the shallowest at 0.5 m in lake #1.
Figure 2. Observed daily temperature above (red squares) and below (blue squares) the thermocline at lake #2, along with simulated daily temperature using parameters determined by the genetic algorithm (GA; solid line) or estimated by stepwise regression (SR; dashed lines). The grey zones represent simulated periods of ice cover.

Figure 3. Observed daily DO concentrations above (red circles) and below (blue circles) the thermocline at lake #2, along with simulated daily DO using parameters determined by the genetic algorithm (GA; solid line) or estimated by stepwise regression (SR; dashed lines). The grey zones represent simulated periods of ice cover.

Calibration performance
Calibration performance for temperature, DO, and light (SD) () shows that the model performs better with more data, such as lake #2 ( and ). For all lakes and calibrations, the best fit for temperature was lake #12 (RMSETemp = 1.72 °C), for DO was lake #9 (RMSEDO = 0.76 mg L−1), and for SD was lake #1 (RMSESD = 0.45 m). Conversely, the worst performance for temperature was lake #1, for DO was lake #4, and for SD was lake #8 (). The SR approach found significant relationships (p < 0.05) between the parameters and the lake characteristics (i.e., the predictors), which varied in terms of predictive power, with R2 values ranging from 0.57 for SWAb1 to 0.89 for the inflow scalers IscV and IscDOC (slopes of estimated relationships in and summary in ; performance of GA and SR summary in and 6)
Figure 4. Relationships between parameter values estimated with genetic algorithm (GA) and optimized using linear regression models obtain with stepwise regression (SR) approach (). Parameters influencing water temperature are identified with squares and those influencing DO with circles. SWAb1 = PAR light attenuation coefficient, SWAb0 = non-PAR light attenuation coefficient, c_shelter = wind sheltering coefficient, IscV = inflow volume, IscT = inflow temperature, IscO = inflow DO, IscDOC = inflow DOC, kSOD = sediment oxygen demand, kBOD = water column oxygen demand.

Figure 5. Target diagrams summarizing model performance for temperature (squares, upper panel) and oxygen (circles, lower panel) using parameters constrained by the genetic algorithm (solid symbols) or estimated by stepwise regression (open symbols) for the 12 focus lakes. The blue squares and red circles show the performance for Lake #2. The diagrams used the normalized bias and normalized centered RMSE between the value observed and simulated from 2001 to 2010 for all depths measured.

Table 4. Model performance summary for temperature, dissolved oxygen (DO), and Secchi depth (SD) for the focus lakes with RMSE, normalized RMSE, and R2 of the accepted parameter set for genetic algorithm (GA) and stepwise regression (SR).
Simulated versus observed ice cover durations were plotted to validate the ice module, with ice cover data available for 47 of the 210 lakes. The comparison considers both parameterization methods (i.e., GA for the 12 focus lakes and SR for the other lake; ). The results show that the 2 methods present a similar duration of the ice cover, with an overestimation for the lakes with the shortest ice cover duration.
Figure 6. Simulated (y-axis) versus observed (x-axis) Secchi depth range, water temperature (square), and oxygen (circle) in the 12 focus lakes. Simulations were performed using parameters determined with genetic algorithm (left panels, solid symbols) or estimated using stepwise regression (right panels, open symbols). Sampling depths for observations are represented by the colour scale from shallow (red) to deep (blue), with relative depth ranging from 0 (Surface) to 1 (zmax). The dashed line shows the 1:1 line and the solid line a linear regression, and the light grey zones encompass 95% of the simulations.

Figure 7. Observed versus simulated annual average ice cover duration for 1971–1980 for 47 lakes with available ice observations. Simulations were performed using parameters determined with genetic algorithm (black diamonds) or estimated using stepwise regression (white diamonds). The dashed line shows the 1:1 relationship.

Projected changes over a 120-year period
The 210 lakes present a large range of future surface temperatures, ice cover duration, and DO concentration (). Within the limitations of the current model performance during calibration on the 12 focus lakes, and neglecting the possible evolution of phosphorus loads and thus of primary production over the next decades, such a framework at the regional scale can be used to explore the response of those 210 lakes to ongoing climate change. Under the Danish Meteorological Institute (DMI) model climate projections, when comparing past (1971–1980) and future (2091–2100) decadal periods, the model predicts on average (1) an increase of 0.75 °C (RCP 4.5) or 2.1 °C (RCP 8.5) for the surface temperatures, (2) a decrease of ice cover duration of 12.6 d (RCP 4.5) or 48.4 d (RCP 8.5), and (3) and a decrease of 0.31 mg L−1 in bottom water DO under RCP 4.5 and, conversely, an increase of 0.12 mg L−1 under RCP 8.5. The density plots also reveal a unimodal distribution for the surface temperatures and multimodal distribution for the ice-cover period, with larger lakes changing less than the smaller lakes. DO concentration at the bottom of the lakes presents a unimodal distribution, with slight changes in most lakes. Results generated from the RCP 8.5 scenario show a much more pronounced change than from the RCP 4.5 scenario, especially for temperature and ice cover duration.
Figure 8. Density curves showing the distribution of change in simulated surface temperature, bottom DO concentration, and ice cover duration between past (1971–1980) and future (2091–2100) decadal periods. Change is calculated as the difference between daily averages simulated for the historical 1971–1980 and future 2091–2100 period, using either the RCP 4.5 (grey line) or the RCP 8.5 (coloured line) scenario. The change in ice cover is the difference between the average ice cover duration of each period divided by the number of decades between past and future periods. Surface temperatures were calculated using simulations of the water layer at 0.5 m while bottom temperatures used the simulations of the last water layer before maximum depth.

Discussion
Performance of the model and comparison with previous studies
The model performance reported here is lower than in previous studies. RMSE for temperature and DO are at the higher end of values reported previously. In particular, we found that DO is less well predicted at depth (Markelov et al. Citation2019, Pilla and Couture Citation2021). MyLake has historically performed well when applied to single lakes. For example, low RMSE ranging from 0.02 to 1.20 °C for temperature and 1.67 to 1.70 mg L−1 for DO concentration were reported for the modeling of Lake Langtjern, Norway (Couture et al. Citation2015, Citation2018). Applications of the lake version of the General Ocean Turbulence Model (GOTM-Lake) at Lake Langtjern (Clayer et al. Citation2021) reported RMSE values of 1.33 °C and 1.9 mg L−1 for water temperature and DO concentration, respectively, in line with the values obtained using MyLake at the same site. Low RMSE for temperatures ranging from 0.63 to 1.00 °C were also reported using MyLake at Lake Kuivajarvi, Finland (Kiuru et al. Citation2018). For 1D lake models (Golub et al. Citation2022), performance has been satisfactory in multi-model studies using LakeEnsembleR, which included the MyLake model among others, with RMSE ranging from 0.5 to 1.3 °C depending on the model evaluated (Moore et al. Citation2021).
Past modeling experiments suggest, however, that the calibration performance tends to decrease when an increasing number of lakes are calibrated together. For instance, in a multi-lake study of 26 Minnesota lakes, the MINLAKE2012 model yielded RMSE values of 1.57 °C and 1.72 mg L−1 for temperature and DO, respectively (Jiang and Fang Citation2016). An RMSE of 2.79 °C was reported for the analysis of 10 774 lakes using uncalibrated iterations of the GLM model (Winslow et al. (Citation2017). Both studies reported RMSE higher than reported for single-lake applications but lower than those reported here. The lack of lateral homogeneity among the lakes selected here may play a role in the reported model performance. Nevertheless, despite high RMSE values of the GA on the 12 focus lakes, simulations using parameters from SR for those 12 lakes yield RMSE values close to those derived from GA. The fit indicates that the parameter inference technique works as intended.
Relationships between parameters and lake morphometry
The SR analysis yielded coherent results, in line with relationships previously observed in boreal lakes at a large scale (Hastie et al. Citation2018). All parameters were derived in relation to 2 or more predictors (). The target graph () shows that calibrating the model based on SR does not decrease performance when compared to a GA calibration except for light, where GA yields better results (). While this relationship is not a strict validation of the model for the data-poor lakes, it tends to support the idea that the determination of the parameters from morphometric characteristics is reasonable. The model shows a cold, low-DO bias for most lakes regardless of the calibration method, with normalized RMSE (i.e., with no units) varying between 0.1 and 0.21 for the temperature and 0.15 and 1.24 for DO concentration.
Projections for data-poor lakes
On average, surface water temperature increases faster in shallow lakes than in deep lakes, consistent with shallow lakes being more responsive to air temperature and therefore more sensitive to climate (De Stasio et al. Citation1996, Adrian et al. Citation2009, Fang and Stefan Citation2009, Dibike et al. Citation2011, Missaghi et al. Citation2017). Ice cover diminution is also in line with trends observed in Sweden from historical data (Hallerbäck et al. Citation2021) and forecasted by previous studies (Guzzo and Blanchfield Citation2017, Woolway et al. Citation2020). Our results project a reduction of ice cover duration of ∼4.03 (median 5.1) days per decade between 1971 and 2100 under the RCP 8.5 scenario (), consistent with previous estimates (Bartosiewicz et al. Citation2016) and within the range of 4–12 days per decade projected for subarctic lakes for 2040–2079 (Dibike et al. Citation2011). Our projected decrease of DO under RCP 4.5 () is in agreement with that observed globally (De Stasio et al. Citation1996, Jane et al. Citation2021). Conversely, RCP 8.5 leads to a slight increase in DO concentrations, reflecting a combined response to both disappearing ice cover and enhanced summer metabolism.
Sources of uncertainty in our projections arise from the lack of future projections for DOC loads in the lakes. Changing DOC would affect all 3 key state variables (Toming et al. Citation2020). Uncertainties also arise from the dataset we used for calibration (Fang and Stefan Citation2009, Missaghi et al. Citation2017). Further work is needed to quantify the data frequency that maximizes model performance (Jackson-Blake and Starrfelt Citation2015) and to quantify the amount of focus lakes necessary for large-scale studies. Specifically, the equations used to estimate our parameters are only based on the variability within a small sample of lakes that cannot exhibit all the variability in characteristics that continental-scale studies have achieved. Building on the presented method, robust relationships could be fitted between characteristics and parameters drawing on a larger lake dataset. Finally, because DO modeling is more complex than modeling water temperature only, it bears more parameter uncertainty.
Conclusions
The amount and nature of data available for each lake are likely to vary widely, ranging from minimal, such as morphology and sparse chemistry data (Jacobson et al. Citation2010, Van Zuiden et al. Citation2016), to comprehensive, such as locations with intensive time-series monitoring stations (Fang and Stefan Citation2009, Fang et al. Citation2012, Couture et al. Citation2015). Real-world datasets likely to be encountered by lake managers and governmental agencies are expected to comprise both lakes with sparse data and lakes with extensive time series. We used a technique in which the parameters for a group of data-poor lakes could be inferred from the parameters of a smaller group of data-rich lakes and morphometric properties (predictors) of these lakes. This technique provided the values of the parameters suitable to correctly simulate water temperature, DO concentration, ice cover duration, and light conditions in 198 data-poor lakes based on the parameters obtained from the calibrations of 12 data-rich lakes.
Supplemental Material
Download MS Word (1.4 MB)Supplemental Material - Fig. S1
Download TIFF Image (20.1 MB)Supplemental Material - Table S1
Download MS Word (19.7 KB)Supplemental Material - Table S2
Download MS Word (21.9 KB)Acknowledgements
We thank Koji Tominaga (Nanyang Technological University, Singapore) and Benjamin Laken (Cervest Inc., London, United-Kingdom) for the retrieval and preparation of the climate data.
Disclosure statement
No potential conflict of interest was reported by the author(s).
Additional information
Funding
References
- Adrian R, O’Reilly CM, Zagarese H, Baines SB, Hessen DO, Keller W, Livingstone DM, Sommaruga R, Straile D, Van Donk E, et al. 2009. Lakes as sentinels of climate change. Limnol Oceanogr. 54:2283–2297.
- Bartosiewicz M, Laurion I, Clayer F, Maranger R. 2016. Heat-wave effects on oxygen, nutrients, and phytoplankton can alter global warming potential of gases emitted from a small shallow lake. Environ Sci Technol. 50(12):6267–6275.
- Bartosiewicz M, Przytulska A, Lapierre JF, Laurion I, Lehmann MF, Maranger R. 2019. Hot tops, cold bottoms: synergistic climate warming and shielding effects increase carbon burial in lakes. Limnol Oceanogr Lett. 4(5):132–144.
- Bartosiewicz M, Ptak M, Iestyn Woolway R, Sojka M. 2020. On thinning ice: effects of atmospheric warming, changes in wind speed and rainfall on ice conditions in temperate lakes (Northern Poland). J Hydrol. 597:125724.
- Beier CM, Stella JC, Dovčiak M, Mcnulty SA. 2012. Local climatic drivers of changes in phenology at a boreal-temperate ecotone in eastern North America. Clim Change. 115(2):399–417.
- Bouffard D, Zdorovennova G, Bogdanov S, Efremova T, Lavanchy S, Palshin N, Terzhevik A, Vinnå LR, Volkov S, Wüest A, et al. 2019. Under-ice convection dynamics in a boreal lake. Inland Waters. 9(2):142–161.
- Clayer F, Thrane JE, Brandt U, Dörsch P, Wit HA. 2021. Boreal headwater catchment as hot spot of carbon processing from headwater to fjord. J Geophys Res Biogeosci. 126:e2021JG006359.
- Couture R-M, De Wit HA, Tominaga K, Kiuru P, Markelov I. 2015. Oxygen dynamics in a boreal lake responds to long-term changes in climate, ice phenology, and DOC inputs. J Geophys Res Biogeosci. 120(11):2441–2456.
- Couture R-M, Moe SJ, Lin Y, Kaste Ø, Haande S, Lyche Solheim A. 2018. Simulating water quality and ecological status of Lake Vansjø, Norway, under land-use and climate change by linking process-oriented models with a Bayesian network. Sci Total Environ. 621:713–724.
- De Stasio BT, Hill DK, Kleinhans JM, Nibbelink NP, Magnuson JJ. 1996. Potential effects of global climate change on small north-temperate lakes: physics, fish, and plankton. Limnol Oceanogr. 41(5):1136–1149.
- De Wit HA, Couture R-M, Jackson-Blake L, Futter MN, Valinia S, Austnes K, Guerrero J-L, Lin Y. 2018. Pipes or chimneys? For carbon cycling in small boreal lakes, precipitation matters most. Limnol Oceanogr Lett. 3(3):275–284.
- Dibike Y, Prowse T, Saloranta T, Ahmed R. 2011. Response of Northern Hemisphere lake-ice cover and lake-water thermal structure patterns to a changing climate. Hydrol Processes. 25(19):2942–2953.
- Dodds WK, Perkin JS, Gerken JE. 2013. Human impact on freshwater ecosystem services: a global perspective. Environ Sci Technol. 47(16):9061–9068.
- Fang X, Alam SR, Stefan HG, Jiang L, Jacobson PC, Pereira DL. 2012. Simulations of water quality and oxythermal cisco habitat in Minnesota lakes under past and future climate scenarios. Water Qual Res J. 47(3–4):375–388.
- Fang X, Stefan HG. 2009. Simulations of climate effects on water temperature, dissolved oxygen, and ice and snow covers in lakes of the contiguous U.S. under past and future climate scenarios. Limnol Oceanogr. 54(6 part 2):2359–2370.
- Golub M, Thiery W, Marcé R, Pierson D, Vanderkelen I, Mercado D, Woolway RI, Grant L, Jennings E, Schewe J, et al. 2022. A framework for ensemble modelling of climate change impacts on lakes worldwide: the ISIMIP lake sector. Geosci Model Dev Discuss. 15(11):4597–4623.
- Guzzo MM, Blanchfield PJ. 2017. Climate change alters the quantity and phenology of habitat for lake trout (Salvelinus namaycush) in small boreal shield lakes. Can J Fish Aquat Sci. 74(6):871–884.
- Hallerbäck S, Huning LS, Love C, Persson M, Stensen K, Gustafsson D, Aghakouchak A. 2021. Warming climate shortens ice durations and alters freeze and breakup patterns in Swedish water bodies. Göttingen (Germany): Copernicus GmbH.
- Hastie A, Lauerwald R, Weyhenmeyer G, Sobek S, Verpoorter C, Regnier P. 2018. CO2 evasion from boreal lakes: revised estimate, drivers of spatial variability, and future projections. Glob Change Biol. 24(2):711–728.
- Holubová M, Hejzlar J, Čech M, Vašek M, Blabolil P, Peterka J. 2021. Fluctuations in pelagic fish density linked to ambient conditions. J Fish Biol. 98(3):756–767.
- Jackson-Blake LA, Starrfelt J. 2015. Do higher data frequency and Bayesian auto-calibration lead to better model calibration? Insights from an application of INCA-P, a process-based river phosphorus model. J Hydrol. 527:641–655.
- Jacob D, Petersen J, Eggert B, Alias A, Christensen OB, Bouwer LM, Braun A, Colette A, Déqué M, Georgievski G, et al. 2014. EURO-CORDEX: new high-resolution climate change projections for European impact research. Reg Environ Change. 14(2):563–578.
- Jacobson PC, Stefan HG, Pereira DL. 2010. Coldwater fish oxythermal habitat in Minnesota lakes: influence of total phosphorus, July air temperature, and relative depth. Can J Fish Aquat Sci. 67(12):2002–2013.
- Jane SF, Hansen GJA, Kraemer BM, Leavitt PR, Mincer JL, North RL, Pilla RM, Stetler JT, Williamson CE, Woolway RI, et al. 2021. Widespread deoxygenation of temperate lakes. Nature. 594(7861):66–70.
- Jiang LP, Fang X. 2016. Simulation and validation of cisco lethal conditions in Minnesota lakes under past and future climate scenarios using constant survival limits. Water. 8(7):279.
- Karlsson J, Byström P, Ask J, Ask P, Persson L, Jansson M. 2009. Light limitation of nutrient-poor lake ecosystems. Nature. 460(7254):506–509.
- Karlsson K-GR. 2003. A 10 year cloud climatology over Scandinavia derived from NOAA Advanced Very High Resolution Radiometer imagery. Int J Climatol. 23(9):1023–1044.
- Keyler TD, Matthias BG, Hrabik TR. 2019. Siscowet lake charr (Salvelinus namaycush siscowet) visual foraging habitat in relation to daily and seasonal light cycles. Hydrobiologia. 840(1):63–76.
- Kiuru P, Ojala A, Mammarella I, Heiskanen J, Kämäräinen M, Vesala T, Huttula T. 2018. Effects of climate change on CO2 concentration and efflux in a humic boreal lake: a modeling study. J Geophys Res Biogeosci. 123(7):2212–2233.
- Knoll LB, Williamson CE, Pilla RM, Leach TH, Brentrup JA, Fisher TJ. 2018. Browning-related oxygen depletion in an oligotrophic lake. Inland Waters. 8(3):255–263.
- Kraemer BM, Anneville O, Chandra S, Dix M, Kuusisto E, Livingstone DM, Rimmer A, Schladow SG, Silow E, Sitoki LM, et al. 2015. Morphometry and average temperature affect lake stratification responses to climate change. Geophys Res Lett. 42(12):4981–4988.
- Kraemer BM, Pilla RM, Woolway RI, Anneville O, Ban S, Colom-Montero W, Devlin SP, Dokulil MT, Gaiser EE, Hambright KD, et al. 2021. Climate change drives widespread shifts in lake thermal habitat. Nat Clim Change. 11(6):521–529.
- Lester NP, Dextrase AJ, Kushneriuk RS, Rawson MR, Ryan PA. 2004. Light and temperature: key factors affecting walleye abundance and production. Trans Am Fish Soc. 133(3):588–605.
- Los FJ, Blaas M. 2010. Complexity, accuracy and practical applicability of different biogeochemical model versions. J Marine Syst. 81(1–2):44–74.
- Magee MR, McIntyre PB, Wu CH. 2018. Modeling oxythermal stress for cool-water fishes in lakes using a cumulative dosage approach. Can J Fish Aquat Sci. 75(8):1303–1312.
- Magnuson JJ, Webster KE, Assel RA, Bowser CJ, Dillon PJ, Eaton JG, Evans HE, Fee EJ, Hall RI, Mortsch LR, et al. 1997. Potential effects of climate changes on aquatic systems: Laurentian Great Lakes and Precambrian Shield region. Hydrol Processes. 11(8):825–871.
- Markelov I, Couture R-M, Fischer R, Haande S, Van Cappellen P. 2019. Coupling water column and sediment biogeochemical dynamics: modeling internal phosphorus loading, climate change responses, and mitigation measures in Lake Vansjø, Norway. J Geophys Res Biogeosci. 124(12):3847–3866.
- MATLAB V. 2019. 9.6. 0.1072779 (R2019a). Natick (MA): The MathWorks Inc.
- Meinshausen M, Smith SJ, Calvin K, Daniel JS, Kainuma MLT, Lamarque JF, Matsumoto K, Montzka SA, Raper SCB, Riahi K, et al. 2011. The RCP greenhouse gas concentrations and their extensions from 1765 to 2300. Clim Change. 109(1–2):213–241.
- Missaghi S, Hondzo M, Herb W. 2017. Prediction of lake water temperature, dissolved oxygen, and fish habitat under changing climate. Clim Change. 141(4):747–757.
- Moore TN, Mesman JP, Ladwig R, Feldbauer J, Olsson F, Pilla RM, Shatwell T, Venkiteswaran JJ, Delany AD, Dugan H, et al. 2021. Lakeensemblr: an R package that facilitates ensemble modelling of lakes. Environ Model Softw. 143:105101.
- Moss R, Babiker M, Brinkman S, et al. 2008. Towards new scenarios for analysis of emissions, climate change, impacts, and response strategies. Geneva (Switzerland): UN Intergovernmental Panel on Climate Change.
- Narisetty NN. 2020. Chapter 4 – Bayesian model selection for high-dimensional data. In: Srinivasa Rao ASR, Rao CR, editors. Handbook of statistics. Amsterdam (Netherlands): Elsevier; p. 207–248.
- Nürnberg GK. 1995. Quantifying anoxia in lakes. Limnol Oceanogr. 40(6):1100–1111.
- O’Reilly CM, Sharma S, Gray DK, Hampton SE, Read JS, Rowley RJ, Schneider P, Lenters JD, Mcintyre PB, Kraemer BM, et al. 2015. Rapid and highly variable warming of lake surface waters around the globe. Geophys Res Lett. 42(24):10773–10781.
- Pilla RM, Couture RM. 2021. Attenuation of photosynthetically active radiation and ultraviolet radiation in response to changing dissolved organic carbon in browning lakes: modeling and parametrization. Limnol Oceanogr. 66(6):2278–2289.
- Pilla RM, Williamson CE, Adamovich BV, Adrian R, Anneville O, Chandra S, Colom-Montero W, Devlin SP, Dix MA, Dokulil MT, et al. 2020. Deeper waters are changing less consistently than surface waters in a global analysis of 102 lakes. Sci Rep. 10(1):20514.
- Pilla RM, Williamson CE. 2021. Earlier ice breakup induces changepoint responses in duration and variability of spring mixing and summer stratification in dimictic lakes. Limnol Oceanogr. 67(S1):S173–S183.
- R Core Team. 2020. R: a language and environment for statistical computing. Vienna (Austria): R Foundation for Statistical Computing.
- Richardson D, Melles S, Pilla R, Hetherington A, Knoll L, Williamson C, Kraemer B, Jackson J, Long E, Moore K, et al. 2017. Transparency, geomorphology and mixing regime explain variability in trends in lake temperature and stratification across northeastern North America (1975–2014). Water. 9(6):442.
- Robson BJ. 2014. When do aquatic systems models provide useful predictions, what is changing, and what is next? Environ Model Softw. 61:287–296.
- Saloranta TM, Andersen T. 2007. Mylake – a multi-year lake simulation model code suitable for uncertainty and sensitivity analysis simulations. Ecol Modell. 207(1):45–60.
- Schindler DW. 2009. Lakes as sentinels and integrators for the effects of climate change on watersheds, airsheds, and landscapes. Limnol Oceanogr. 54(6 part 2):2349–2358.
- SMHI. 2017. Modell data per område [Modell data per area] [accessed June 2017]. https://vattenwebb.smhi.se/modelarea/
- SMHI. 2019. Is på sjöar och vattendrag [Ice on lakes and waterways] [accessed August 31, 2019]. https://www.smhi.se/data/hydrologi/is-pa-sjoar-och-vattendrag
- Solomon CT, Jones SE, Weidel BC, Buffam I, Fork ML, Karlsson J, Larsen S, Lennon JT, Read JS, Sadro S, et al. 2015. Ecosystem consequences of changing inputs of terrestrial dissolved organic matter to lakes: current knowledge and future challenges. Ecosystems. 18(3):376–389.
- Stasko AD, Gunn JM, Johnston TA. 2012. Role of ambient light in structuring north-temperate fish communities: potential effects of increasing dissolved organic carbon concentration with a changing climate. Environ Rev. 20(3):173–190.
- Stefan HG, Fang X. 1994. Dissolved oxygen model for regional lake analysis. Ecol Modell. 71(1-3):37–68.
- Stefan HG, Fang X, Eaton JG. 2001. Simulated fish habitat changes in North American lakes in response to projected climate warming. Trans Am Fish Soc. 130(3):459–477.
- Stefan HG, Hondzo M, Eaton JG, McCormick JH. 1995. Validation of a fish habitat model for lakes. Ecol Modell. 82(3):211–224.
- Stocker TF, editor. 2014. Climate Change 2013: the physical science basis. Working Group 1 (WG1) contribution to the Intergovernmental Panel on Climate Change (IPCC) 5th assessment report (AR5). Cambridge (UK): Cambridge University Press.
- Thrane J-E, Hessen DO, Andersen T. 2014. The absorption of light in lakes: negative impact of dissolved organic carbon on primary productivity. Ecosystems. 17(6):1040–1052.
- Toming K, Kotta J, Uuemaa E, Sobek S, Kutser T, Tranvik LJ. 2020. Predicting lake dissolved organic carbon at a global scale. Sci Rep. 10(1):8471.
- Van Dorst RM, Gårdmark A, Svanbäck R, Huss M. 2020. Does browning-induced light limitation reduce fish body growth through shifts in prey composition or reduced foraging rates? Freshw Biol. 65(5):947–959.
- Van Zuiden TM, Chen MM, Stefanoff S, Lopez L, Sharma S. 2016. Projected impacts of climate change on three freshwater fishes and potential novel competitive interactions. Divers Distrib. 22(5):603–614.
- Vasconcelos FR, Diehl S, Rodríguez P, Hedström P, Karlsson J, Byström P. 2019. Bottom-up and top-down effects of browning and warming on shallow lake food webs. Glob Change Biol. 25(2):504–521.
- Weidel BC, Baglini K, Jones SE, Kelly PT, Solomon CT, Zwart JA. 2017. Light climate and dissolved organic carbon concentration influence species-specific changes in fish zooplanktivory. Inland Waters. 7(2):210–217.
- Winslow LA, Hansen GJA, Read JS, Notaro M. 2017. Large-scale modeled contemporary and future water temperature estimates for 10774 Midwestern U.S. lakes. Sci Data. 4(1):170053.
- Woolway RI, Kraemer BM, Lenters JD, Merchant CJ, O’Reilly CM, Sharma S. 2020. Global lake responses to climate change. Nat Rev Earth Environ. 1(8):388–403.
- Zentner DL, Cross TK, Raabe JK, Jacobson PC. 2019. Using GIS to predict habitat in lakes: an example using nearshore substrate categories. Limnol Oceanogr. 17(1):1–16.