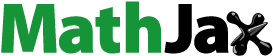
ABSTRACT
Stochastic weather generators create time series that reproduce key weather dynamics present in long-term observations. The dataset detailed herein is a large-scale gridded parameterization for CLImate GENerator (CLIGEN) that fills spatial gaps in the coverage of existing regional CLIGEN parameterizations, thereby obtaining near-global availability of combined coverages. This dataset primarily covers countries north of 40° latitude with 0.25° spatial resolution. Various CLIGEN parameters were estimated based on 20-year records from four popular global climate products. Precipitation parameters were statistically downscaled to estimate point-scale values, while point-scale temperature and solar radiation parameters were approximated by direct calculation from high-resolution datasets. Surrogate parameter values were used in some cases, such as with wind parameters. Cross-validation was done to assess the downscaling approach for six precipitation parameters using known point-scale values from ground-based CLIGEN parameterizations. These parameter values were derived from daily accumulation records at 7,281 stations and high temporal resolution records at 609 stations. Two sensitive parameters, monthly average storm accumulation and maximum 30-minute intensity, were shown have RMSE values of 1.48 mm and 4.67 mm hr−1, respectively. Cumulative precipitation and the annual number of days with precipitation occurrence were both within 5% of ground-based parameterizations, effectively improving climate data availability.
1. Introduction
Stochastic weather generators like CLImate GENerator (CLIGEN) can be used as a climate driver for various applications (Chen et al., Citation2018; Sparks et al., Citation2018). CLIGEN generates records of specified length that reproduce dynamics such as daily precipitation intensity, inter-annual variability, and long-term climate norms. With additional assumptions, sub-daily precipitation time series may also be generated for any time step. The synthetic time series can play important roles in various contexts such as risk assessments and long-term model simulations; moreover, they may have a larger role in the study of ungauged basins. Aside from being used in modelling, the synthetic time series may assist in disaggregation of long-term forecasts and climate projections. CLIGEN output is comprised of daily time series of precipitation amount, maximum and minimum air temperature, average dewpoint temperature, solar radiation, wind speed, and wind direction.
Most commonly, CLIGEN time series are used to drive soil erosion and agricultural models such as the Water Erosion Prediction Project (WEPP), Rangeland Hydrology and Erosion Model (RHEM), and Agricultural Policy Environmental eXtender (APEX), with precipitation often being the focus (Feng et al., Citation2019; Hernandez et al., Citation2017; Srivastava et al., Citation2019). Directly related to precipitation time series, rainfall erosivity is an index of water erosion potential and an important proxy for runoff. It is used as a factor in the family of models that are based on the Universal Soil Loss Equation (USLE), and a recommended minimum record length for calculation of annual erosivity is 20 years, which may be readily generated by CLIGEN (Wischmeier & Smith, Citation1965; Yin et al., Citation2017). Though further research is needed, CLIGEN time series appear to reproduce rainfall erosivity with reasonable accuracy, such as in Flanagan et al. (Citation2020) where an average erosivity value across tested locations was within 7% of the measured average. Studies have also explored the use of CLIGEN for watershed assessment, benefiting from having a long-term record that contains a range of climate conditions to test for a given location (Zhao et al., Citation2021).
At the global level, the availability of observed point-scale precipitation records is limited by the distribution of ground networks. Alternatives to observations may include point-scale precipitation records downscaled from gridded global climate products wherein grid-scale precipitation evenly distributed across the area of a grid cell is downscaled to point-scale, accounting for the spatial scale of weather dynamics acting within the grid resolution. The present paper uses a statistical downscaling approach to estimate point-scale CLIGEN precipitation parameters from grid-scale data. This ultimately enables the end user to generate point-scale weather time series.
Existing large-scale gridded CLIGEN parameterizations vary in several respects, including their spatial resolutions, the reference periods used to derive parameters, and the ways in which statistical downscaling of parameters is performed. The existing CLIGEN grids currently known to the authors are shown in . Covering the contiguous U.S., the PRISM CLIGEN (or PRISM CLIgrid) dataset is a spatial interpolation at 4 km resolution of a network of observed CLIGEN parameters that were derived from a minimum of 40-year records (PRISM climate group, Citation2023). Co-variates involved in the interpolation come from the gridded PRISM climate product. The dataset of Wang et al. (Citation2021) covering China at 10 km resolution is similarly based on a spatial interpolation of ground networks using co-variate geospatial information with ground-based parameters from 70-year records. The datasets created using the approach of Fullhart et al. (Citation2022) cover remaining global land masses south of ~ 40°N (excluding Antarctica) at 0.25° resolution (~28 km at the equator). In this approach, precipitation parameters were downscaled from grid-scale using machine learning regressions. The grid-scale climate data was derived from 20-year records, and the downscaling regressions were informed by global ground networks consisting of a mixture of long-term reference periods. Due to the relatively coarse resolution used in this approach, higher errors are possible in locations that have strong spatial climate gradients, as is often the case in mountainous regions and other scenarios; while in most places, the 0.25° spatial resolution is adequate to capture local gradients, such as in agricultural basins, for example.
Table 1. Coverages of known gridded CLIGEN parameterizations. The combined coverage includes most global land mass excluding Antarctica.
The objectives of this paper are to characterize a new CLIGEN parameter grid that fills remaining gaps in coverage in the northern hemisphere, encompassing countries north of roughly 40°N at 0.25° resolution and resulting in near-global terrestrial coverage, excluding Antarctica. The approach is slightly modified from the methodology of Fullhart et al. (Citation2022), mainly to include a different subset of predictor variables for statistical downscaling and to take average monthly temperatures and solar radiation from a high-resolution monthly dataset. To assess the accuracy of statistical downscaling models for precipitation parameters that were developed and applied to the coverage area, two forms of cross-validation are done. Potential sources of errors in precipitation parameters are assessed, and additionally, properties of the dataset are discussed in terms of the pros and cons of applying CLIGEN as a climate driver.
2. Materials and methods
2.1. Gridded CLIGEN parameterization
The new CLIGEN parameterization compatible with version 5.3 (Agricultural Research Service, Citation2023) covers areas in the northern hemisphere indicated in at 0.25° resolution. The data is formatted to produce CLIGEN parameter files constituting 114,150 files in total, corresponding to the number of grid points in the coverage. The coverage includes areas in North America, Europe, and Asia where there is no existing coverage, encompassing countries north of 40°N with the exceptions of the U.S. and China. shows attributes of the global climate grids and ground networks used as inputs. Additionally, the JAXA ALOS digital elevation model at 30 m resolution was used (Tadono et al., Citation2014). The precipitation parameterization approach makes use of historical gridded climate data products with resolutions ranging from 0.04° to 0.25°. Most information was taken from the ERA5-Daily climate reanalysis with 0.25° resolution (Hersbach et al., Citation2020), and as such, center coordinates of ERA5 grid cells were used as the grid point locations for the CLIGEN grid. As much as possible, the approach attempts to approximate parameters at the exact grid points. The climate products with finer resolutions (TerraClimate and GPM-IMERG v06 Final; Abatzoglou et al., Citation2018; Huffman et al., Citation2015) were sampled only at corresponding grid cells that cover grid point locations, meaning that not all grid cells were sampled within the coverage area for these products. This assumes that there is no dependency on neighbouring grid cells at the grid point location regardless of the proximity. The climate grids and other geospatial data were accessed using the Google Earth Engine cloud computing platform (Gorelick et al., Citation2017). The international CLIGEN ground network (based on NOAA GHCN-Daily) and a high temporal resolution ground network at U.S. airports, NOAA ASOS, were used to develop supervised datasets (maps shown in , respectively). These stations encompass areas in the northern hemisphere within the latitudinal range of the gridded coverage area. While the ASOS network is entirely outside of the coverage area, it is still representative of climate types present in the coverage area.
Figure 1. Distribution of the ground network CLIGEN parameterization used to inform downscaling of MEAN P, SDEV P, SKEW P, and RATIO (international CLIGEN network/NOAA GHCN-Daily, n = 7,281).

Figure 2. Distribution of the high temporal resolution ground network used to inform downscaling of MX.5P (NOAA ASOS). The total number of stations is 609, most with 14-year gap-filled record lengths.

Table 2. Datasets used to derive the gridded CLIGEN parameterization include gridded global climate products and ground network CLIGEN parameterizations.
Differing data availability meant that there were some inconsistencies in the starting and ending dates of the reference periods in both the historical gridded data and ground networks. The lengths of ground records used to calculate CLIGEN parameters were in the range of 10–30 years, with most stations representing 30-year records. Climate non-stationarity and extreme interannual variability during these periods have the potential to be sources of uncertainty when comparing to the 20-year reference periods that were used from the gridded datasets, being exclusively within the 21st century. The ASOS network was used to validate estimations of CLIGEN’s maximum 30-minute intensity parameter with 1-min, gap-filled 14-year records, which are particularly long record lengths for high temporal resolution data. Temporal gap-filling of missing data was performed using WEPPCLIFF v1.3 (McGehee et al., Citation2020), and a breakpoint conversion procedure was used to address intensity overestimation of the raw 1-minute, fixed-interval data. As discussed later, spatial clustering of the ground stations in certain climate types was addressed using a weighting scheme in which samples in the supervised dataset corresponding to overrepresented climate types were given smaller weighting values. Also discussed later, missing data affects the parameterization coverage in some areas in extreme northern latitudes, primarily within the Artic.
2.2. Precipitation parameterization from daily time series
CLIGEN precipitation parameters include monthly aggregate precipitation statistics calculated from observed daily and sub-daily time series. In the downscaling approach, daily time series taken from ERA5-Daily were used to calculate grid-scale parameter values, followed by application of downscaling models to ultimately produce estimations of point-scale parameter values given by Equations 1–5. The mean, standard deviation, and skewness of event accumulation are given by MEAN P, SDEV P, and SKEW P, respectively, and are directly estimated with downscaling models. In Equations 1–3, p is a precipitation event, and n is the total number of events during the considered month throughout the whole record (a maximum of one precipitation event per day is assumed by CLIGEN). The length unit is U.S. customary inches, which is converted to units of mm for reporting. In Equations 4 and 5, the monthly probability of precipitation occurrence following a “wet” day and following a “dry” day are given by the P(W|W) and P(W|D) conditional probabilities, respectively. Nww is the number of wet days following a wet day, Nwd is the number of wet days following a dry day, and so on. As described in the following, the parameters defined by Equations 4 and 5 are estimated indirectly.
Estimation of point-scale MEAN P is critical because of its further involvement in a parameter adjustment scheme wherein the monthly number of wet days are estimated, along with P(W|W) and P(W|D). In this scheme, monthly accumulation (ACCUM) values at each grid point are taken from 20-year average values from TerraClimate. The value of ACCUM relates to CLIGEN parameters according to Equation 6. In Equation 6, the term in brackets is equivalent to the fraction of wet days to total days in the month, i.e. P(W). This fraction is multiplied by the total number of days in the month (ndays) to yield the monthly number of wet days. In the parameter adjustment scheme, the value of ACCUM is divided by the estimated point-scale MEAN P to yield the number of wet days. Given TerraClimate’s high resolution, this scheme has the effect of providing an approximation of point-scale ACCUM and the number of wet days at each grid point.
At this stage in the parameter adjustment, there is not enough information to determine P(W|W) and P(W|D) individually without more information, such as the ratio of the two probabilities. The ratio of the two probabilities effectively controls the sequencing of wet and dry days given the total number of wet days. Therefore, their ratio is introduced as a new parameter in Equation 7 and is similarly estimated with a downscaling model.
Finally, given an estimate of both the number of wet days and RATIO, a script was created to solve the individual probabilities. This can be either done algebraically or by iteratively adjusting the two probabilities by equal proportions while respecting the RATIO value.
2.3. Precipitation parameterization from sub-daily time series
Two required CLIGEN parameters are derived from observed sub-daily records, being the mean monthly maximum 30-minute intensity (MX.5P) and the probability distribution for time-to-peak-intensity (TimePk). In this approach, grid-scale values of MX.5P calculated from GPM-IMERG 30-minute time series are downscaled to estimate point-scale MX.5P. The definition of MX.5P is given in Equation 8 where max is maximum 30-minute intensity for the considered month in a given year and k is equal to the number of years in the record:
The TimePk parameter is also calculated from high temporal resolution ground records. Precipitation intensity distributions in CLIGEN are largely insensitive to TimePk (Wang et al., Citation2018), and therefore, it was deemed acceptable to use surrogate ground observations on the basis of climate type. For a given location, TimePk represents empirically derived probabilities at 12 equal fractions of event duration between 1/12 and 1, where each probability quantifies the chance of an event having peak intensity at or before the respective fraction of event duration. A climate classification was used to group TimePk records by their climate type, and it was found that variance was small within the extents of each climate. Therefore, the average TimePk distribution is a reasonable approximation of TimePk for a location within a given climate classification. The climate classification used was according to the Köppen-Geiger classification mapped by Beck et al. (Citation2018). These average TimePk distributions were determined only within the U.S., and for some sub-classifications not present, it was necessary to assume TimePk distributions from within the same major classification.
2.4. Temperature parameterization
There are five required temperature parameters in CLIGEN that were calculated directly from 20-year time series from gridded products. Two of these could be calculated from the high-resolution TerraClimate dataset, while three were calculated from the coarser ERA5-Daily dataset. Monthly average maximum and minimum daily temperatures (TMAX AV and TMIN AV, respectively) represent two of the parameters that were taken from the TerraClimate high-resolution monthly dataset. The monthly standard deviation of daily maximum and minimum temperatures (SD TMAX and SD TMIN, respectively) are often not reported by monthly climate datasets. Similarly, monthly average dewpoint (TDEW) was not available at high resolution. These parameters were calculated from the ERA5-Daily dataset using the same 20-year window. All units are converted to U.S. customary Fahrenheit.
2.5. Solar radiation parameterization
As with other variables, incoming solar radiation parameterization requires both a daily mean and standard deviation parameter (SOL.RAD and SD SOL, respectively). In this case, SOL.RAD is set according directly to the monthly average incoming solar radiation taken from the high-resolution TerraClimate dataset, while SD SOL is calculated from the coarser GLDAS 3-hr dataset (Rodell et al., Citation2004) by finding daily average solar radiation and calculating the standard deviation for each month. The units are in U.S. customary Langley (1 Langley = 41,840 J m−2).
2.6. Wind parameterization
Monthly wind parameters in CLIGEN have high data requirements, and in fact, the majority of CLIGEN parameters are for wind. There are four parameters per each of sixteen cardinal directions. From each considered direction, the parameters include the percentage of time that wind comes from the considered direction, the mean windspeed, the standard deviation of windspeed, and the skewness of windspeed.
Due to data limitations, surrogate wind parameters were assigned from the U.S. CLIGEN ground network on the basis of similarities to non-wind parameters. For this, the most appropriate surrogate wind parameters were found using the “International Conversion Program” (Agricultural Research Service, Citation2023). This program finds a station within the U.S. CLIGEN ground network that most closely matches a target station based on its daily-derived precipitation parameters and daily-derived max/min temperature parameters. Each CLIGEN parameter file contains metadata about the location that the surrogate wind parameters were taken from and the statistical degree of similarity to the location.
2.7. Downscaling of precipitation parameters with gradient boosting
CLIGEN’s precipitation parameters were downscaled to account for the significant differences between grid-scale and point-scale precipitation records. This involved the development of five statistical downscaling models for MEAN P, SDEV P, SKEW P, MX.5P, and RATIO using a machine learning regression algorithm called gradient boosting. For each of the five parameters, grid-scale parameter values and other geospatial data were used as inputs for downscaling models, while the outputs of the models were point-scale parameter estimates. Data was organized into corresponding supervised datasets that included all monthly values and sampled locations pooled into single data tables. The downscaling models were not informed with the calendar month corresponding to each sample (i.e. the models were used to estimate corresponding parameters for all months and locations within the coverage area). Information included in the supervised datasets are shown in . The data table sizes reflect the number of ground stations that provide monthly target values with outliers and missing data cases removed. A total of 7,281 stations were used from the international CLIGEN network, and with omitted data, this brought the total number of monthly data table entries for corresponding data tables to 85,106 (down from 87,360). For the data table corresponding to the MX.5P model that took target values from the ASOS ground network, a total of 609 stations were used, and removal of outliers from the total number of data table entries resulted in 7,144 data points (down from 7,308). Outliers occurred for several possible reasons, including extreme storms, measurement error, and missing data. The most obvious outliers with the largest deviations occurred for MEAN P and MX.5P values greater than 3 inches and 4 inches hr−1 (76.2 mm and 101 mm hr−1), respectively. Data table entries were removed for all variables corresponding to the monthly value considered an outlier.
Table 3. Covariates and target variables for the gradient boosting models used for downscaling.
The Python Sci-Kit Learn library v1.0.2 implementation of gradient boosting (GB) was used for downscaling (Pedregosa et al., Citation2011). The GB regression model represents an ensemble method that has the objective of minimizing bias error. GB additively builds a regression ensemble by reducing the largest prediction errors with updates to the ensemble. GB is capable of handling non-linearity and does not assume a statistical distribution of the population of sampled data. Collinearity between predictor variables is generally less of a concern in GB compared to other regressions because of its framework. Moreover, unlike more basic statistical methods, GB regression has parameters that require tuning. A more thorough handling of GB is given by Friedman (Citation2002). The technique often outperforms other regression techniques in similar applications, e.g. when using GB for gap-filling meteorological time series (Körner et al., Citation2018). However, in a study that predicted monthly climate factors from gridded data, it was shown that performance differences between regression techniques may be statistically insignificant and that more basic statistical regressions such as multiple linear regression may actually perform better in some cases (Hussein et al., Citation2021). In the present methodology, GB is preferred for its overall flexibility and because assumptions of normally distributed data are likely violated by the supervised dataset due to the sampling design and other factors. As will be discussed, the present approach tunes only the learning rate hyperparameter, which sets the amount of error reduction that is allowed for each additive model, while the maximum number of regressions in the ensemble is held constant. As a hyperparameter, the learning rate has influence over the training process, and the GB skill and degree of fitting (over-fitting versus under-fitting) is highly sensitive to the learning rate.
The predictor variables shown in represent both climate and geospatial information corresponding to the locations of ground stations. Models that estimate point-scale factors calculated from daily time series had their point-scale target values taken from GHCN-Daily, while the downscaling model for MX.5P had its target values taken from the sub-daily ASOS ground network. In , a total of 23 predictor variables are shown that were used in each downscaling model, including grid-scale values of the target variables of each model, which were labelled as TARGET. Collinearity between predictor variables was assessed using the Variance Inflation Factor (VIF) that is based on Pearson Correlation. Threshold values for VIF have ranged from 4 to 10 in the literature, such that excessive collinearity is indicated when VIF is greater than the threshold value (Salmerón et al., Citation2018). The average VIF value of correlations between predictor variables for the daily-based parameters was 0.87, and 3 correlations were found with higher VIF values. These variables were retained because of the potential for scenarios where a combination of the variables could provide unique information. One pair of variables showing collinearity was TMAX AV and TMIN AV, which in combination, provide the range of diurnal temperature variation. A second pair was TMAX AV and TDEW. In terms of characterizing topography in the target grid cell, the third pair of collinear variables was ELEV and ELEV AVG (units of m), which provide the elevational difference between the grid point and average elevation of the 0.25° grid cell. The information given by this pair of variables could potentially provide useful information about the relative position of a grid point in mountainous terrain. The average slope (labelled SLOPE AVG, units of degrees) and surface area (labelled AREA, units of km2) of each grid cell were also used as geospatial predictors. The geospatial predictors were among others that are essentially constant for all monthly target values at a given station. These included constant statistics related to grid-scale parameter values and monthly accumulations for a given grid point. For the MX.5P supervised dataset, the average VIF value for collinearity of predictor variables was 1.01 with the same three variable pairs having higher VIF as the other models. Additionally, the ANNUAL and TARGET AVG, and TARGET AVG and TARGET MIN variable pairs had higher VIF. The ANNUAL and SDEV ACCUM predictor variables are equivalent to annual accumulation and the standard deviation of monthly accumulation, respectively.
2.8. Cross-validation with ground measurements
Validation of the precipitation downscaling approach is based on cross-validation in which a portion of the supervised dataset is reserved for testing only. Fitted model error is of little importance compared to error found by cross-validation, because in GB, predictions made from training data are likely to be overfitted, leading to an artificially small error; hence, all candidate and accepted models were tested with reserved subsets. Two forms of cross-validation are done: (1) K-folds during the model selection phase, and (2) leave-one-out for the accepted models. The RMSE statistic was considered for comparison of model performance and model selection using the ground data as known target values. K-folds with 5 folds (K = 5) was used to test each learning rate value during model selection. In this, a fraction of 1/5 of the supervised dataset was reserved for testing, and 5 model fittings were done with differing testing folds. Training 5 models for each tested learning rate provided a check for internal heterogeneity within the dataset, and as was expected, the 5 reserved folds gave similar RMSE. After model selection, leave-one-out cross-validation (LCV) was done as a second form of cross-validation. In this case, LCV used all training data except data from a single ground station that was reserved for error assessment, and therefore, LCV used the most similar training dataset to the accepted models (the accepted models were trained on the entire supervised dataset when used in predictive fashion to produce the gridded parameterization). Initially, a selection of random stations used for LCV consisted of 100 stations for all models, wherein 1 model is trained per station. However, for the international CLIGEN network, 15 of the randomly selected stations were removed due to missing data issues and suspected errors in ground measurements, which brought the total number of considered international CLIGEN stations to 85, while all 100 randomly selected U.S. stations were used for the MX.5P cross-validation.
2.9. Downscaling model selection
Model selection for each downscaling model was done by varying the learning rate hyperparameter across the following range of values: 1 × 10−5 < learning rate ≤ 1. Within this range, a total of 45 hyperparameter values were defined by dividing each order of magnitude into 9 equal intervals. The number of estimators in the GB ensemble was kept constant at 5,000 and default values suggested by the Sci-kit Learn library were accepted for other hyperparameters. For each candidate model, K-folds cross-validation was done using 5 splits, bringing the number of models to 225 (5 × 45). Then, the tuning process involving K-folds was repeated for the 5 downscaled parameters, bringing the total number of individually tested models in the model selection process to 1,125 (5 × 225). The models with the lowest corresponding average RMSE of reserved folds were accepted. During model training, the supervised dataset included weighting coefficients based on the global climate type present at each ground station. Sample weighting was done to account for the uneven distribution of training samples across different climate types, wherein weighting was assigned to a station based on its climate type that gave greater weight to stations less represented by ground network distributions. In this case, the Köppen-Geiger climate classification was used according to the global maps of Beck et al. (Citation2018). In addition to the proportion of ground stations in each climate type, the proportion of each climate type present in the gridded coverage was determined. The applied sample weight for a given climate type was the ratio of the latter quantity over the former quantity. Sample weighting was not applied to training data for the MX.5P model because the corresponding ground data had fewer stations and coverage only within the U.S., outside of the gridded coverage area.
3. Results
3.1. Accepted precipitation parameter downscaling models
The selected downscaling models for MEAN P, SDEV P, SKEW P, MX.5P, and RATIO had learning rate values of 1 × 10−1, 6 × 10−2, 7 × 10−3, 2 × 10−2, and 9 × 10−4, respectively. Accepted model outcomes represented by all reserved datapoints from K-folds are shown in . For comparison purposes, calculated RMSE based on fitted training datapoints (not shown) suggested that each model was over-fitted to a minimal degree, with the exception of the MX.5P model, which showed significant over-fitting. Also barring the MX.5P model, the slope coefficients for regressions of predictions versus observations, similar to those in , did not approach a value of 1 in any case within the range of tested learning rates. Furthermore, reserved data error was within 5% of training data error for models other than for MX.5P, which had 49% smaller error in predictions for fitted training data, reflecting over-fitting despite the model having a similar regression slope coefficient as other accepted models. There were notable tendencies in the outcomes of candidate models in the selection process that were consistent with these findings. With increasing learning rate, there were three tendencies given in the following: (1) models went from showing either slight under-fitting, or essentially no over-fitting, to varying degrees of over-fitting for the greatest learning rate; (2) variance (and RMSE) decreased quickly before an inflection point, and then slowly increased, meaning that the range of tested learning rates contained an apparent minimum RMSE for each model; and (3) slope coefficients for linear regressions against predicted data increased quickly at first, and then slowed without approaching a slope of 1, except for in the MX.5P model case. Coefficients less than 1 for the accepted models reflected the fact that the largest errors tended to be underestimations, particularly for the RATIO model. The GB model for RATIO also had the highest overall prediction error. Prediction of RATIO is challenged by a degree of ambiguity in values where different dry/wet transition probabilities can yield the same value of RATIO. It is evident in that many of the observations for RATIO, which were recorded to precision of hundredths, had the exact same values for this reason. However, as previously noted, errors in RATIO only affected the sequencing of wet and dry days, and not the total number of wet days.
Figure 3. Results of K-folds for the accepted downscaling models based on all reserved splits. The color scale represents a kernel density value for the density of datapoints.

Figure 4. Results of leave-one-out cross-validation (black X’s are ground-based parameter values, and red circles are predicted parameter values). Note that the Y-axis is truncated and does not always start at the origin. Also note that for the MX.5P results, the tested stations are at U.S. airports. These are outside of the coverage area but within the same latitudinal range.

3.2. Leave-one-out cross-validation of precipitation parameters
The leave-one-out cross-validation (LCV) trained each model with all data in the supervised datasets except for the data from a single station used only for testing, and this was repeated for a selection of random stations. LCV is suited to assessment of error at individual stations and produces models that are most representative of the accepted models, which used the entirety of the supervised datasets for training. Average error metrics of predictions based on all reserved data are shown in , including RMSE. The percent bias (PBIAS) is minimal for all estimates, while percent error (PERROR), which is an indicator of relative error, suggests that errors are particularly high for the RATIO model (error metric definitions were according to Moriasi et al. (Citation2007)). The LCV results compared to the K-folds cross-validation results shown in are improved. This is potentially a consequence of there being more training data in LCV, and furthermore, there is a potential for the selection of LCV stations to have smaller measurement error than others in the supervised datasets. In , results are shown for 10 individual stations that were considered in LCV. As may be expected judging from the regressions of , RATIO has the largest prediction errors for individual stations. Moreover, it is also consistent with that the largest errors for SKEW P coincide with the greatest observations at each station, such that there appears to be underestimation bias for larger SKEW P estimations. In contrast, the models for MEAN P, SDEV P, and MX.5P provided reasonable predictions in most cases.
Table 4. Leave-one-out cross-validation results determined based on a pool of all reserved data.
The LCV outcomes were also used to investigate sources of error. For this, all LCV predictions from reserved data were grouped into a single subset to facilitate analysis of error as a function of three factors: (1) the magnitude of observed values, (2) the spatial density of the corresponding ground network, and (3) the spatial variability of climate within the grid resolution, which further considered the possibility of topographically controlled climate gradients by analysing the average terrain slope within the grid resolution. These sources of error were investigated only for the MEAN P model, and combined error effects are considered later.
The correlation between prediction error and the magnitude of parameter values was checked for the MEAN P model to ascertain whether larger observed values had greater corresponding prediction errors. The potential for this source of error is apparent in the fact that there are non-heterogeneous residuals and outliers in some of the models in . The impact of the largest outliers on overall error was investigated by checking if a linear relationship exists between absolute error (units of mm) and the parameter value magnitude for corresponding observed values. A linear regression of absolute error versus observed values resulted in a weak correlation with r-squared of 0.327 and slope and intercept coefficients of 0.20 and −0.26, respectively. However, the relationship was statistically significant, with the T-test value for the slope coefficient corresponding to p < 0.01. This suggests that major outliers occurred often enough to have a significant impact on error, and the positive slope suggests that to some degree, absolute error increases with the magnitude of parameter values. This source of error is likely to affect some models more than others, particularly the RATIO model.
The second source of error was the effect of spatial density of the ground network and the potential for areas that are less densely represented by the ground network to show greater error. This was investigated by calculating point density at each station using a circular search area with a radius of 100 km. Binning the data according to spatial density and determining the average percent error for each bin showed that percent error had no relationship with spatial density of the ground network. There was no discernible increase in error associated with decreasing point density, and each bin tended to have a percent error close to the overall average percent error, unlike what would be expected if sparsely covered areas were affected by increased error. In fact, stations used for LCV from the densest coverage areas tended to show higher errors, granted that relatively few stations from the densest clusters were analyzed. The densest coverage areas of the network corresponded, in part, to mountainous regions with strong spatial climate gradients, potentially explaining this result. There is also a potential for clusters of ground stations to be affected by similar measurement errors that are part of local networks. In general, this error analysis suggests that the uneven distribution of the ground network had little impact on the success of regionalization of the downscaling models, and that over-fitting of predicted point-scale values due to spatially correlated training data was not an issue.
The third source of error is strong spatial climate variability within the grid resolution, which also partly relates to the presence of topographically controlled climate gradients. Again, this source of error was investigated only for the MEAN P model. For the MX.5P model, which considers more data at 0.1° resolution, the problem of local climate gradients is less of an issue for making point-scale predictions. The first investigation of this source of error was based on an indicator to represent spatial climate variability within the 0.25° grid resolution, being the standard deviation of grid values of annual precipitation taken from the higher resolution TerraClimate dataset within each grid cell (2000–2020 annual precipitation was considered). As before, the standard deviation values were binned, and the average percent error for each bin was determined. There was no apparent increase in percent error with increasing standard deviation, and the greatest average percent error values occurred for mid-ranged standard deviations. This analysis has the potential of being biased by spatial averaging, even with the relatively high resolution of TerraClimate. A second approach that specifically analysed the impact of complex terrain on climate gradients was more conclusive in showing increased error as a result of strong local climate gradients. Shown in , an upward trend in error with average terrain slope is evident, suggesting that the issue of strong climate gradients is primarily associated with complex terrain, at least for the stations selected for LCV. As is the case for many ground networks, ground stations in areas with higher average slope are less represented, and therefore there is potential for unrepresentative sampling design to influence the error analysis. is generally useful for identifying cases where error may be higher, and for the greatest average slopes, error is dramatically higher. This suggests that the use of gridded CLIGEN data at 0.25° spatial resolution may be inappropriate in certain cases.
3.3. Effect of combined errors on CLIGEN time series output
Also, using LCV data, error analysis was done for generated precipitation time series in comparison to those generated from the international CLIGEN ground network. By considering CLIGEN output, combined error effects associated with error in multiple CLIGEN input parameters could be quantified. Firstly, precipitation intensity for daily time frames were compared to those of the ground-derived outputs. In , the frequency distribution of combined daily intensities from all LCV stations are shown for the gridded coverage and ground network. The distributions were virtually identical with only small deviations being visually apparent, suggesting that errors in CLIGEN input parameters had minimal negative impact on the overall accuracy of daily intensities. This is partly because the downscaling models had low PBIAS, and subsequently, the sizable relative errors in individual input parameters had a cancelling effect. However, differences were identified in cumulative precipitation and the number of wet days. The LCV data showed 2.9% more cumulative precipitation than corresponding ground-based time series. Furthermore, this apparently overestimated cumulative precipitation was distributed over 3.9% more wet days. The relative accuracy of cumulative precipitation and daily intensities has the benefit of ensuring that precipitation is distributed over the correct number of days. This also reflects the accuracy of TerraClimate monthly accumulation and estimated point-scale MEAN P, which control the number of days with precipitation > 0 based on the parameter adjustment scheme.
Figure 6. Frequency distributions of daily intensity from all leave-one-out cross-validation reserved splits compared against the international CLIGEN network.

Secondly, a single metric is considered that is sensitive to the precipitation-time profile of storm patterns and both daily and sub-daily intensities, being annual rainfall erosivity. This metric is an index of the erosive power and the vigour of rainfall. Erosivity is exponentially greater for a given storm when compared to a storm with the same accumulation but lower intensity. Subsequently, the MX.5P input parameter and resulting sub-daily precipitation patterns have major influence on the resulting erosivity. The annual rainfall erosivity was calculated from 20-year CLIGEN time series according to Revised Universal Soil Loss EquationEquation (2)(2)
(2) (RUSLE2) guidelines. There are notable caveats, being that LCV ground-based parameterizations using NOAA GHCN-Daily time series required estimates of MX.5P, while other precipitation parameters could be directly calculated. Estimation error of MX.5P for the ground network parameterization was similar to the present study. As such, true values of MX.5P at corresponding international ground stations used in LCV were not known. Furthermore, air temperature is a factor in the calculation of annual rainfall erosivity in cold climates in order to omit frozen precipitation from consideration. This can result in uncertainties when only information on daily maximum and minimum temperatures are given. The plotted error given by the erosivity comparison is shown in . A cluster of data points is apparent with low erosivity that correspond partly to locations with large proportions of annual precipitation represented by snowfall. Larger erosivity values are reasonably estimated, though some heterogeneity of variance propagated into CLIGEN output. Overall, output from the gridded CLIGEN parameterization provides a close approximation of those derived from ground records and is useful for calculating rainfall erosivity and other factors.
4. Discussion
This gridded CLIGEN parameterization contributes to existing coverages that when combined, attain near-global coverage. Based on 21st-century, 20-year gridded climate records, stochastic point-scale time series can be readily produced for the majority of global land mass. In the present dataset, daily and sub-daily intensities are well represented, and monthly accumulation and temperatures are set according to the high-resolution TerraClimate dataset. Generated point-scale precipitation time series have major advantages over many available grid-scale climate products when being used for applications that expect point-scale precipitation. In comparison, even high-resolution daily historical datasets that exist for regions of the globe, some with resolutions of 4 km, often have distributions of daily intensity and number of wet days that are less representative of observed point-scale records as CLIGEN when properly parameterized. This has implications on the success of CLIGEN over other sources of climate data for applications relating to rainfall-runoff, rainfall erosivity, and long-term climate norms.
However, various issues led to higher error in some situations, with different sources of error having differing effects on resulting parameter estimates and generated time series, each with specific implications for potential applications of the data. While some of these uncertainties could be quantified, the spatial distribution of ground networks was a limiting factor that influenced the outcome of validations. Given this limitation, several suspected situations could be identified where the dataset may be less reliable. Due to the coarser spatial resolution of the CLIGEN grid, error may be higher where there are strong climate gradients within the resolution of the grid, including in grid cells where topography has control on climate. Ideally, time series should be generated in areas with uniform climate such as large agricultural basins or other broad landforms, or locations with relatively uniform weather patterns. Secondly, analysis of extreme rainfall based on generated time series may be particularly affected by higher error due to the error that was apparent in the SKEW P model, which has controls over the likelihood of extreme events. Moreover, when using CLIGEN for extreme event frequency analysis, there are known biases for sub-daily intensity windows, though daily intensity windows are generally well predicted (Wang et al., Citation2018). This potential source of error requires more investigation in the context of frequency analysis. Related to missing data and other issues, there is also a greater potential for error at extreme northern latitudes. In these locations, several issues lead to less reliable data in the original datasets, including in the GPM-IMERG dataset, which has spatial and temporal gaps in coverage. Grid-scale MX.5P from GPM-IMERG was used as a covariate in each downscaling model but was unavailable for much of the artic and sub-artic regions, leading to higher error. Above a latitude of 60°, a total of 55% of grid-scale MX.5P values were missing, with the prevalence of missing values being greater with latitude. The MX.5P downscaling model is likely to be most affected by this error. However, it is also likely that daily intensities and cumulative precipitation will still be reasonably approximated because they are largely controlled by other parameters.
Lastly, there are unquantified sources of error related to the objectivity of the cross-validation analyses involved in the downscaling approach. In general, the outcomes of the LCV better represent these potential errors compared to the K-folds outcomes. As is necessary for ground validation, the unique spatial distributions of each network had some impact on error, and the LCV closely represented the actual ground records of the accepted models. Furthermore, Hussein et al. (Citation2021) point out that random selection of stations and data shuffling (as done in the K-folds analysis) can lead to over-reporting of model accuracy due to data leakage, whereby information used for training is not available to the model when being used in predictive fashion. Considering this, it is the case that in the present K-folds analysis, monthly values for all calendar months and all stations were pooled to create the supervised datasets. As such, predictions made for monthly parameter values using models trained with data that included other monthly parameter values from the same station can lead to the potential for autocorrelation and artificially lowered error (granted, models were not informed with the locations of stations or any other unique identifier for individual stations). In contrast, this form of data leakage could not occur in the LCV methodology where all monthly parameters except for those from a single station were reserved for validation. However, since only K-folds was used for model selection, the potential for autocorrelation to be reflected in model selection represents a limitation of the downscaling approach.
For a given location, verification of the accuracy of monthly wet days can provide a check on the parameter adjustment scheme. In this scheme, the monthly number of wet days is essentially set equal to monthly accumulation according to TerraClimate divided by the estimated point-scale MEAN P value. The annual number of wet days is shown in to assess the scheme on an annual level and identify areas affected by higher error. Overall, comparison of the number of wet days according to the CLIGEN grid against long-term climate norms gives reasonable results, but some suspect areas exist. Particularly in extreme northern latitudes, the map shows some processing artifacts that are apparent along latitudinal and longitudinal lines, or where distortion occurs in the surface area of a square degree as area decreases approaching the northern pole. Related to this, there are suspect areas in Greenland and other places where extremely sharp gradients occur. Future CLIGEN parameterizations may potentially benefit from having access to high-resolution climate data that provides the monthly number of wet days rather than attempting to estimate this variable.
There are several potential applications for the gridded dataset. Most commonly, CLIGEN is used as the climate driver for soil erosion simulations at small scales, e.g. plot-scale or hillslope-scale. The validation of annual rainfall erosivity suggests that the generated precipitation time series are suitable for soil erosion modelling and can reproduce key dynamics that erosion models are sensitive to. In the case of the Universal Soil Loss Equation, annual rainfall erosivity is used as a factor directly rather than a continuous precipitation time series, illustrating the fact that CLIGEN output may be used in a variety of ways. While variance in the annual erosivity predictions is considerable, variance was primarily an issue for large values. A trade-off is that systematic bias of the estimated erosivity is minimal compared to other data sources from which rainfall erosivity may be calculated. Use of other data sources, such as ground records with coarse temporal resolutions, often require significant bias adjustments made to factors involved in the calculation, and when high-temporal resolution data with short record lengths are used, it is questionable what effect interannual variability has on annual averages. Another important potential application is hydrological and erosional simulations in ungauged basins where CLIGEN time series have the potential to be an integral component.
It is important to note that with further assumptions, CLIGEN time series may be disaggregated to any time step required by a hydrological model (Yu, Citation2002). However, since CLIGEN produces point-scale precipitation, it should generally not be applied to larger catchments or watersheds without some form of area weighting. Another consideration is that CLIGEN will not produce spatially coherent time series, meaning that if time series are generated for two nearby CLIGEN stations, the time series will not have the expected degree of spatial correlation due to the stochastic nature of the time series. Stochastic weather generators exist that are able to produce spatially coherent time series, but these require additional information to parameterize and are generally more computationally intensive (Zhao et al., Citation2019). It is also important to note that interannual variability of precipitation and other factors may not have realistic sequencing. While annual precipitation in CLIGEN can often be successfully fitted to probability distributions such as the Generalized Extreme Value distribution, dynamics like drought and pluvial cycles are not well represented. Lastly, a limitation of CLIGEN is that standard normal deviates and random variates are sampled from distributions that represent only single variables, and therefore, each variable is sampled independently, such that daily maximum temperature is not dependent on daily minimum temperature, etc. Applications that are sensitive to daily interactions between two or more variables may be affected.
Potential applications also include study of climate change projections and forecasts, which can both be done with similar approaches in CLIGEN. Further processing is necessary to represent such time series based on stochastically generated time series because the generated time series represent a stationary climate and have other differences. Two scenarios are discussed in the following, in which climate projections and forecasts are ingested into CLIGEN by disaggregating forecasted time series to finer temporal resolutions (additionally, observed time series may also be disaggregated in this way). The first scenario uses a built-in feature of CLIGEN, which requires both a parameterization for a given location and a forecasted daily precipitation time series for the same location. In this scenario, CLIGEN disaggregates a daily-scale precipitation record and produces corresponding point-scale storm profiles based on daily accumulations. The second scenario involves disaggregation of monthly time series from climate projections and long-term forecasts (note, the former data are currently available globally at high-resolution). This can be done by simply matching the projected monthly accumulations or average temperatures with the most similar 1-month blocks in CLIGEN time series. Both scenarios essentially represent the creation of a semi-stochastic dataset. For long-range climate projections, it is best to update CLIGEN parameterizations to reflect changes in the climate regime caused by climate change. Tools exist for this, such as WEPPCAT, which manipulates baseline CLIGEN parameters to reflect climate change scenarios (Bayley et al., Citation2010).
5. Conclusion
In conclusion, the CLIGEN parameterization greatly increases the availability of climate time series for several applications and brings the combined global coverages of CLIGEN parameterizations to all land mass excluding Antarctica. CLIGEN time series are capable of reproducing point-scale precipitation intensity distributions and other weather dynamics. The generated time series may have an integral role in the study of ungauged basins or other locations where data limitations (e.g. short or incomplete ground records) preclude climate-related studies. Additionally, challenges associated with spatially continuous global climate data are addressed by this dataset. The issue of incompatible spatial or temporal scales of global climate data for a given application is potentially resolved by use of this dataset. As such, stochastic point-scale time series may be useful as a climate driver for numerous models in the context of risk-assessment and other goals.
Open Scholarship
This article has earned the Center for Open Science badge for Open Data. The data are openly accessible at https://doi.org/10.15482/USDA.ADC/1529311
Acknowledgements
This research was a contribution from the Long-Term Agroecosystem Research (LTAR) network. LTAR is supported by the United States Department of Agriculture. Thank you to those who make climate data publicly available for research.
Disclosure statement
No potential conflict of interest was reported by the author(s).
Data availability statement
The CLIGEN parameter files for each grid point are freely available for download at the National Agricultural Library data repository, Ag Data Commons, with the following link: https://doi.org/10.15482/USDA.ADC/1529311. To generate climate time series from the parameter files, CLIGEN may be downloaded from the following website: https://www.ars.usda.gov/midwest-area/west-lafayette-in/national-soil-erosion-research/docs/wepp/cligen/. The CLIGEN software runs efficiently on essentially all computers.
Additional information
Notes on contributors
Andrew T. Fullhart
Andrew Fullhart is currently a post-doctoral researcher at the USDA-ARS Southwest Watershed Research Center in Tucson, Arizona, U.S. Andrew earned a B.Sc. in Geoscience from the University of Iowa, a M.Sc. in Environmental Science, and a Ph.D in Hydrologic Science. Andrew’s primary research interests are in hydrological modelling and hydrometeorology. Andrew has been involved in international soil erosion research projects and creating large-scale geospatial datasets.
References
- Abatzoglou, J. T., Dobrowski, S. Z., Parks, S. A., & Hegewisch, K. C. (2018). TerraClimate, a high-resolution global dataset of monthly climate and climatic water balance from 1958–2015. Scientific Data, 5(170191), 1–12. https://doi.org/10.1038/sdata.2017.191
- Agricultural Research Service. (2023). CLIGEN [Software]. https://www.ars.usda.gov/midwest-area/west-lafayette-in/national-soil-erosion-research/docs/wepp/cligen/
- Bayley, T., Elliot, W., Nearing, M. A., Guertin, D. P., Johnson, T., Goodrich, D., & Flanagan, D. (2010). Modeling erosion under future climates with the WEPP model. In Proceedings of the 2nd Joint Federal Interagency Conference on Sedimentation and Hydrologic Modeling, Las Vegas, NV, 27 June - 1 July, (pp. 1–12).
- Beck, H. E., Zimmermann, N. E., McVicar, T. R., Vergopolan, N., Berg, A., & Wood, E. F. (2018). Present and future Köppen-Geiger climate classification maps at 1-km resolution. Scientific Data, 5(1), 180214. https://doi.org/10.1038/sdata.2018.214
- Chen, J., Chen, H., & Guo, S. (2018). Multi-site precipitation downscaling using a stochastic weather generator. Climate Dynamics, 50(5–6), 1975–1992. https://doi.org/10.1007/s00382-017-3731-9
- Diamond, H. J., Karl, T. R., Palecki, M. A., Baker, C. B., Bell, J. E., Leeper, R. D., Easterling, D. R., Lawrimore, J. H., Meyers, T. P., Helfert, M. R., Goodge, G., & Thorne, P. W. (2013). US climate reference network after one decade of operations: Status and assessment. Bulletin of the American Meteorological Society, 94(4), 485–498. https://doi.org/10.1175/BAMS-D-12-00170.1
- Feng, Q., Engel, B. A., Flanagan, D. C., Huang, C. H., Yen, H., & Yang, L. (2019). Design and development of a web-based interface for the agricultural policy environmental eXtender (APEX) model. Environmental Modelling & Software, 111, 368–374. https://doi.org/10.1016/j.envsoft.2018.09.011
- Flanagan, D. C., McGehee, R. P., & Srivastava, A. (2020). Evaluation of different precipitation inputs to WEPP, ASABE Annual International Virtual Meeting, St. Joseph, Michigan, U.S.A., July 12-15, 2020, https://doi.org/10.13031/aim.202000740
- Friedman, J. H. (2002). Stochastic gradient boosting. Computational Statistics & Data Analysis, 38(4), 367–378. https://doi.org/10.1016/S0167-9473(01)00065-2
- Fullhart, A. T., Nearing, M. A., Armendariz, G., & Weltz, M. A. (2021). Climate benchmarks and input parameters representing locations in 68 countries for a stochastic weather generator, CLIGEN. Earth System Science Data, 13(2), 435–446. https://doi.org/10.5194/essd-13-435-2021
- Fullhart, A., Nearing, M., & Weltz, M. (2021). Temporally downscaling precipitation intensity factors for Köppen climate regions in the United States. Journal of Soil and Water Conservation, 76(1), 39–51. https://doi.org/10.2489/jswc.2021.00156
- Fullhart, A., Ponce-Campos, G. E., Meles, M. B., McGehee, R. P., Armendariz, G., Oliveira, P. T., Almeida, C. N., de Araújo, J. C., Nel, W., & Goodrich, D. C. (2022). Gridded 20-year climate parameterization of Africa and south America for a stochastic weather generator (CLIGEN). Big Earth Data, 7(2), 1–26. https://doi.org/10.1080/20964471.2022.2136610
- Gorelick, N., Hancher, M., Dixon, M., Ilyushchenko, S., Thau, D., & Moore, R. (2017). Google Earth Engine: Planetary-scale geospatial analysis for everyone. Remote Sensing of Environment, 202, 18–27. https://doi.org/10.1016/j.rse.2017.06.031
- Hernandez, M., Nearing, M. A., Al-Hamdan, O. Z., Pierson, F. B., Armendariz, G., Weltz, M. A., Spaeth, K., Williams, J. C., Nouwakpo, S. K., Goodrich, D. C., Unkrich, C. L., Nichols, M. H., & Holifield-Collins, C. D. (2017). The rangeland hydrology an erosion model: A dynamic approach for predictiong soil loss on rangelands. Water Resources Research, 53(11), 9368–9391. https://doi.org/10.1002/2017WR020651
- Hersbach, H., Bell, B., Berrisford, P., Hirahara, S., Horányi, A., Muñoz‐Sabater, J., Nicolas, J., Peubey, C., Radu, R., Schepers, D., Simmons, A., Soci, C., Abdalla, S., Abellan, X., Balsamo, G., Bechtold, P., Biavati, G., Bidlot, J., Bonavita, M., & Villaume, S.… Thépaut, J. N. (2020). The ERA5 global reanalysis. Quarterly Journal of the Royal Meteorological Society, 146(730), 1999–2049. https://doi.org/10.1002/qj.3803
- Huffman, G. J., Bolvin, D. T., Nelkin, E. J., & Tan, J. (2015). Integrated multi-satellite retrievals for GPM (IMERG) technical documentation. NASA/GSFC Code.
- Hussein, E. A., Ghaziasgar, M., Thron, C., Vaccari, M., & Bagula, A. (2021). Basic statistical estimation outperforms machine learning in monthly prediction of seasonal climatic parameters. Atmosphere, 12(5), 539. https://doi.org/10.3390/atmos12050539
- Körner, P., Kronenberg, R., Genzel, S., & Bernhofer, C. (2018). Introducing gradient boosting as a universal gap filling tool for meteorological time series. Meteorologische Zeitschrift, 27(5), 369. https://doi.org/10.1127/metz/2018/0908
- McGehee, R. P., Flanagan, D. C., & Srivastava, P. (2020). WEPPCLIFF: A command-line tool to process climate inputs for soil loss models. Journal of Open Source Software, 5(49), 2029. https://doi.org/10.21105/joss.02029
- Moriasi, D. N., Arnold, J. G., Van Liew, M. W., Bingner, R. L., Harmel, R. D., & Veith, T. L. (2007). Model evaluation guidelines for systematic quantification of accuracy in watershed simulations. Transactions of the ASABE, 50(3), 885–900. https://doi.org/10.13031/2013.23153
- Pedregosa, F., Varoquaux, G., Gramfort, A., Michel, V., Thirion, B., Grisel, O., Blondel, M., Prettenhofer, P., Weiss, R., Dubourg, V., Vanderplas, J., Passos, A., Cournapeau, D., Brucher, M., Perrot, M., & Duchesnay, E. (2011). Scikit-learn: Machine learning in Python. Journal of Machine Learning Research, 12, 2825–2830.
- PRISM Climate Group. (2023). PRISM (parameter-elevation regressions on Independent Slopes model) climate group. Oregon State University. http://www.prism.oregonstate.edu
- Rodell, M., Houser, P. R., Jambor, U. E. A., Gottschalck, J., Mitchell, K., Meng, C. J., Arsenault, K., Cosgrove, B., Radakovich, J., Bosilovich, M., Entin, J. K., Walker, J. P., Lohmann, D., & Toll, D. (2004). The global land data assimilation system. Bulletin of the American Meteorological Society, 85(3), 381–394. https://doi.org/10.1175/BAMS-85-3-381
- Rui, H., Beaudoing, H., & Loeser, C. (2021). README document for NASA GLDAS version 2 data products, Goddard Earth Sciences data and information Services center (GES DISC). NASA Goddard Space Flight Center.
- Salmerón, R., García, C. B., & García, J. (2018). Variance inflation factor and condition number in multiple linear regression. Journal of Statistical Computation and Simulation, 88(12), 2365–2384. https://doi.org/10.1080/00949655.2018.1463376
- Sparks, N. J., Hardwick, S. R., Schmid, M., & Toumi, R. (2018). IMAGE: A multivariate multi-site stochastic weather generator for European weather and climate. Stochastic Environmental Research and Risk Assessment, 32(3), 771–784. https://doi.org/10.1007/s00477-017-1433-9
- Srivastava, A., Flanagan, D. C., Frankenberger, J. R., & Engel, B. A. (2019). Updated climate database and impacts on WEPP model predictions. Journal of Soil and Water Conservation, 74(4), 334–349. https://doi.org/10.2489/jswc.74.4.334
- Tadono, T., Ishida, H., Oda, F., Naito, S., Minakawa, K., & Iwamoto, H. (2014). Precise global DEM generation by ALOS PRISM. ISPRS Annals of the Photogrammetry, Remote Sensing & Spatial Information Sciences, 2, 71–76. https://doi.org/10.5194/isprsannals-II-4-71-2014
- Wang, W., Flanagan, D. C., Yin, S., & Yu, B. (2018). Assessment of CLIGEN precipitation and storm pattern generation in China. Catena, 169, 96–106. https://doi.org/10.1016/j.catena.2018.05.024
- Wang, W., Yin, S., Flanagan, D. C., & Yu, B. (2018). Comparing CLIGEN-generated storm patterns with 1-minute and hourly precipitation data from China. Journal of Applied Meteorology and Climatology, 57(9), 2005–2017. https://doi.org/10.1175/JAMC-D-18-0079.1
- Wang, W., Yin, S., Yu, B., & Wang, S. (2021). CLIGEN parameter regionalization for China. Earth System Science Data, 13(6), 2945–2962. https://doi.org/10.5194/essd-13-2945-2021
- Wischmeier, W. H., & Smith, D. D. (1965). Predicting rainfall erosion losses in the Eastern U.S.: A guide to conservation planning. In Agricultural Research Service (p. 47). US Gov. Print Office.
- Yin, S., Nearing, M. A., Borrelli, P., & Xue, X. (2017). Rainfall erosivity: An overview of methodologies and applications. Vadose Zone Journal, 16(12), 1–16. https://doi.org/10.2136/vzj2017.06.0131
- Yu, B. (2002). Using CLIGEN to generate RUSLE climate inputs. Transactions of the ASAE, 45(4), 993. https://doi.org/10.13031/2013.9952
- Zhao, Y., Nearing, M. A., & Guertin, D. P. (2019). A daily spatially explicit stochastic rainfall generator for a semi-arid climate. Canadian Journal of Fisheries and Aquatic Sciences, 574, 181–192. https://doi.org/10.1016/j.jhydrol.2019.04.006
- Zhao, Y., Nearing, M. A., & Guertin, D. P. (2021). Modeling hydrologic responses using multi-site and single-site rainfall generators in a semi-arid watershed. International Soil & Water Conservation Research, 10(2), 177–187. https://doi.org/10.1016/j.iswcr.2021.09.003