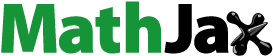
ABSTRACT
Urbanization comes with the price of the negative complexity of crime. It is a reflection of the socio-demographic and permeability factors. This paper aims to integrate socio-demographic crime factors with street permeability to find the association with residential burglary spots. We first find the residential burglary hotspots area related to socio-demographic factors of the study area, then identify potential residential burglary risk areas based on the factors of street permeability and find the association of residential burglary with socio-demographics and street permeability factors in Akure. The methodology employed includes the Inverse Distance Weight factor analysis, space syntax, and the Poisson regression analysis. The findings showed hotspots of burglary within neighbourhoods, confirming the relationship between the factors. Issues identified herein denote some logical starting points for criminological engagement with the Sustainable Development Goals. In the conclusion, we discussed the implications for the statistical output.
1. Introduction
Rapid urbanization comes with the price of the negative complexity of crime and the fear of crime, a key concern in cities. Safety is a vital feature of what is considered a high-quality sustainable environment. The notions of crime in design and planning practice imply broad articulation of property crime and urban form.
The crime dimension of sustainable urban form has only recently been subject to widespread scrutiny (Rudlin & Falk, Citation1999; P. Cozens, Citation2007; Glasson & Cozens, Citation2011; Hipp and Kim (Citation2019), and Kim and Wo (Citation2021). This is reported to be exacerbated by the recent advocation of the new urbanism. It is argued that a planned sustainable urban form should be the case where the residents ‘should not have cause for fear for their personal safety and the safety of possessions’ (Du Plessis, Citation1999; Newman, Citation1996). The United Nations sustainable urban development agenda, ‘Agenda 2030’, especially related to Goal 11 reiterated cities’ Sustainability and emphasized the concern of controlling crime and building safe and just societies (United Nations, Citation2015).
The high population densities generally promoted by planners (New Urbanism) generate more people on the streets. In theory, this seems appropriate for sustainable development since it entails more pedestrian activity and encourages ‘eyes on the street’ posited by Jacobs (Citation1961). In similar contrast is the defensible space theory of Newman (Citation1972), which advocates territorial control to deter strangers from entering spaces. These theories are established to have a relation with the patterns of crime in neighbourhoods (Newman, Citation1996; Frith et al., Citation2017; Hipp & Kim, Citation2019). This is because the number and people’s movement patterns in a given space and time can influence crime patterns, largely determined by street networks and permeability.
The prevailing theories of the spatial location of crime are predicated on the theory of social disorganization theory and opportunity theory (Weisburd & Zastrow, Citation2022). The spatial location of crime hinges on the theory of routine activity. Meanwhile, emerging theories on sustainable urban form all suggest the cognizance of the influence of street permeability and the variety of land uses near the streets (P. L. Brantingham & And, Citation2008).
Therefore, planning for a crime-free sustainable city must put into cognizance the form of the city in terms of the influence of street permeability, and characteristics of the street segment in correlation with the social and demographic characters of neighbourhoods. The influence of these mentioned parameters on city form has been explained to determine crime risk areas (Johnson & Bowers, Citation2010). In this context, permeability is described as the street network’s influence on pedestrian movement, which in turn, affects the occurrence of crime (Silva & Li, Citation2020; Wu et al., Citation2015).
Studies carried out by Bevis et al. (Citation1977), examined the relationship between burglary rates in Minneapolis and street network permeability measured at the census tract level. Using variations in socio-economic factors, they found a positive correlation between permeability measured at the census tract level and burglary risk. White (Citation1990) found that street permeability influences neighbourhood burglary rates when other factors are controlled (i.e. economic factors and structural density). Armitage (Citation2010) examined the risk of burglary and total crime using data from households in housing estates in West Yorkshire, UK, and found that an increase in street permeability would increase the risk of residential burglary. Later, Johnson and Bowers (Citation2010) analyzed a police district in Merseyside, UK, and concluded that cul-de-sacs and sinuous streets are a less probability of residential burglary compared to permeable roads. Succinctly put, early methodologies considered only socio-demographic factors. But recently researchers have also suggested that the studies of crime risk need to consider the effects of urban form in terms of street network configurations on crime. They show relatively less attention paid to the relationship with socio-demographic factors of crime (Frith et al., Citation2017).
However, related research by Legewie (Citation2018) attempt to explain the relationship between social boundaries and crime spot, while accounting for physical effects. He concluded that race and socio-economy could determine the area of crime. Similarly, Hipp and Kim (Citation2020) attempted to relate physical boundaries and social characteristics of the sides of a street segment and found that traditional physical boundaries, such as roads, exhibit a positive relationship with crime and that street segments with social boundaries consistently have a high rate of violent and property crime. These outcomes indicate that control variations in socio-economic factors show a positive correlation between permeability and burglary risk. Hence, it can be deduced that permeability has a significant influence on neighbourhood burglary rates, socio-demographic factors being equal.
The current study considers the small areal unit of the city core. It attempts to demonstrate that crime is localized in one area. This is congruent with the ‘law of crime concentration at place’. Weisburd (Citation2015) predicts a very small proportion of city locales will account for a significant proportion of crime incidents. Crime tends to cluster in small areas, like street segments or buildings at micro-places (Bernasco & Block, Citation2011; Malleson et al., Citation2019). Hence, small areal units should be best able to capture the spatial patterns of crime, being less likely to conceal the actual locale of crime hotspots or combine various hotspots that are very distinct (Carmona et al., Citation2003; Oberwittler & Wikstrom, Citation2009). Even though smaller areal units will largely contain fewer crimes, this will suffice for this research since we are dealing with only incidences of burglaries.
The research seeks how urban form in terms of street network configuration and socio-demographic factors can explain the risk of crime in street segments. It aims to integrate socio-demographic factors and street permeability to find the association with residential burglary spots. It will be carried out through the objectives of finding residential burglary hotspots area related to socio-demographic factors of the study area; identifying potential residential burglary risk areas based on the factors of street permeability; finding the association of residential burglary with socio-demographics and street permeability factors in the study area.
It is hypothesized that there is an association between socio-demographic factors and pedestrian movement patterns with crime hot spots.
1.1. Theoretical framework and literature review
Offences committed on properties without force is property crime (P. J. Brantingham & Brantingham, Citation1993). In line with this, Soh (Citation2012) defined property crimes as those offences involving the loss of property during which there is no use of violence by the perpetrators (including burglary, arson, larceny, theft, wreck, pilfering, stealing, house and store breaking, and vehicle theft), those with violence include robbery, snatch theft, pilfering, pickpocketing, vandalization, and others (Dambazau, Citation2007; Song et al., Citation2013). Urban form describes all elements that make up the city like buildings, streets, and squares. It is the outcome of a determining process, which could be geographical or man-made (Hipp et al., Citation2020).
Many works in the the literature looking at the spatial patterns of crime have identified relationships between socio-demographic factors (i.e. age, gender, poverty, income, education, etc.). Such factors have either been theorized or shown to be associated with crime across various geographic units (Boessen & Hipp, Citation2015; Kim & Wo, Citation2021). While the literature looking at the spatial patterns of crime identified the relationships between structural characteristics (i.e. poverty, residential instability, racial heterogeneity, economic inequality, etc.) of street segments and crime; these studies usually employ social disorganization theory as the theoretical frame (Hipp & Kim, Citation2019). Although scholars of criminology of place have somewhat neglected the importance of the structural characteristics in empirical studies, Weisburd et al. (Citation2012) suggested that when the street segment is seen as a type of ‘micro-community’, then social disorganization theory would have direct relevance to the understanding of the criminology of place. This implies the necessity of testing the effects of structural characteristics on crime. However, in this research for the use of space syntax theory, it is pertinent to consider the theory of natural movement. It explains movement along specific, planned pathways, which connect the origin and destination points (Boivin & Felson, Citation2017). As urban structure shapes patterns of movement, so too does it shape patterns of criminal activity (Silva & Li, Citation2020; Wuschke & Bryan, Citation2018).
Understanding the influence of urban form on crime has been the focus of researchers in both design and social fields. In social sciences, the prevailing theory of spatial location of crime is the social disorganization theory (Sampson & Groves, Citation1989). On the other hand, the theory of the spatial location of the crime is the theory of routine activity, which claims that criminal events are caused by attraction to targets, the time and space of crime (Cohen & Felson, Citation1979). The opportunity theory explains the pattern and ecology of environmental crime, including the physical environment of the urban area and that criminals are motivated by knowledge about the environment. While the opportunities for crime and their awareness of spaces largely depend upon their routine activity patterns (P. L. Brantingham & And, Citation2008). Cozens et al. (Citation2019) and Carr (Citation2020) toed the line of environmental determinism, explaining that the immediate environment potentially precipitates criminal behaviour, i.e the environment’s attribute can prompt criminal behaviour and pattern of crime. The pattern of streets in a city is related to the pattern of crime because the roads determine the accessibility to potential crime spots in a city. Cities built on gridiron patterns are known to have higher crime rates when compared to cities with naturally developed street layouts (Silva & Li, Citation2020).
The issue of street permeability has become central to considering how to deliver less crime and more sustainable urban design. There are two distinct perspectives on this topic: the ‘encounter’ model and the ‘enclosure’ model (Dovey, Citation1998). The ‘encounter model’, explains that permeable streets are safer because they encourage walking, and social contact, which enhances levels of ‘eyes on the street’ (Jacobs, Citation1961). However, proponents of the ‘enclosure model’ argue that permeability is limited, by controlling access to strangers (Newman, Citation1972). Residents can more readily distinguish between strangers and potentially criminal behaviour, thereby reducing opportunities for crime. Dempsey (Citation2008) observes how a well-connected and permeable built environment is considered a feature of high quality in the form of small street blocks. Small blocks provide more varied routes for pedestrians and allow easier movement (Carmona et al., Citation2003). Gehl (Citation2001) assumes that well-connected pedestrian routes become well used and are preferred over deserted or low-volume routes. Therefore, the assumption is that permeable streets are safer. Hillier and Shu (Citation2000) explicate that incidents of burglary are higher on more isolated properties on cul-de-sacs.
1.2. Permeability and crime
It is well established that crime and permeability are correlated. Previous approaches to identifying crime hotspots required creating maps showing the distribution of crime but have been found inadequate. This is because it is a manual process that neither provides accurate nor reliable comprehensive data. This does not help in trend prediction and decision support, which results in a low accuracy rate (Alabi, Citation2021; Jones & Fanek, Citation1997; Nubani, Citation2003).
Several metrics have been developed to determine permeability, such as ‘integration’, which measures how close (or easy to access) a given location is to all others in terms of paths through the network. Applying these methods to crime and analyzing data from London, UK, shows that crime is positively related to road connectivity, but negatively related to integration (Hillier, Citation2004). This leads to the conclusion that permeable designs are advantageous, but only in situations when redundant connectivity is present (that which does not increase integration). In Davies and Johnson (Citation2014) street network metrics model, permeability was measured using explanatory factors such as indicators of opportunity and a few numbers of socio-demographic descriptors. While Wu et al. (Citation2014) analyzed the variation in street network configuration and socio-economic factors to determine areas of permeability. Kim and Wo (Citation2021) studied the connection and interaction between street networks by applying a measure of centrality (Betweenness centrality). They found that betweenness exerted a criminogenic impact, which suggests that the permeability and accessibility of a segment (based on the street network) are more likely to weaken social control and capable guardianship. Whereas some studies have found that streets with higher integration, connectivity, and choice have a higher risk of crime. Other studies have found that less accessible streets have more crime when controlling for the length of street segments (Armitage 2007; Armitage, et al Citation2010; Alabi, Citation2021; Hillier & Iida, Citation2005).
The foregoing all depict the big role the street network plays in urban processes. It is the main foundation of ‘space syntax’. This rests on the fundamental observation that individual street segments cannot be well understood alone but must be considered in the context of the rest of the network and their position within it.
Three main measurable elements of space syntax are as follows: (1) integration, (2) connectivity, and (3) choice. Integration measures accessibility or closeness centrality that indicates how close a street segment is to all other surrounding segments within a given distance threshold. It is defined as the inverse of the cumulative distance required from the street segment to all other segments that fall within the search radius along the shortest paths. Connectivity measures the number of street segments connected to a street segment. Choice is defined as the fraction of the shortest paths between pairs of other street segments in the network that pass by a street segment. Several studies empirically tested the relationship of space syntax measures with crime and their findings are mixed.
Other studies show high associations between street integration, permeability, and the presence of pedestrians. The street design determines the pattern of pedestrian movement, which indirectly influences spots of crime. Jones and Fanek (Citation1997) and Nubani (Citation2003) conducted crime research using street connectivity in space syntax, and they found that streets with higher integration values were associated with higher crime rates, and lower connectivity values were also associated with lower crime rates. Other urban design researchers have also employed space syntax techniques to analyze the geographic distribution of crime with promising results (Wu et al., Citation2015). Hence, the measure of space syntax is useful in further clarifying the importance of street design since it deals with both spatial and functional aspects of urban form (Koohsari et al., Citation2013; Koohsari et al., Citation2014).
2. Study area
Akure is a traditional city of Yoruba origin in the southwestern part of Nigeria. It lies approximately between 7°1112411 and 7°1713811 North of the Equator and between 5°615011 and 5°161111 East of the Greenwich meridian. It is a medium-sized urban area, the capital city of Ondo province, and Akure local government council headquarters in 1976. The city has experienced a population growth rate of approximately 3.01% increase from 235,000 in 1991 to 360,268 in 2006 and 486,300 in 2016. Its Population is estimated to be 719,393 (Population Stat, Citation2022). Akure is located about 420-km southwest of Abuja, the Federal capital of Nigeria (). The area of research is limited to the Akure core and immediate surroundings. This consists of Araromi, Cathedral, Erekesan, Eruoba, Ijomu, Imuagun, Isolo, Obanla, Odo-Ijoka, Odo-Ikoyi, and the Stadium area. The area is crossed by a major road like Oba Adesida Road ().
3. Methodology
The dependent variables of the current study are the number of incidents of property crime (burglary) in street segments. The street segments are streets between two intersections, following buildings on street segments within a 500-m buffer in the centre. Data were collected from buildings on both sides of the streets and each line was segmented based on its intersection with another road using the open street map (). The study area covered 11 neighbourhoods. It is worth noting that the areal units often used in analyses at the meso-level such as this in the crime and place literature are administrative boundaries that encapsulate highly diverse populations and urban characteristics (Andresen and Malleson Citation2011).
The Police considered these parameters in the reported incidents of property crime (burglary) at the 114 street segment, where 321 burglaries were reported. This was inputted to produce a crime hotspot map, using Inverse Distance Weight analysis (IDW). The acquired crime spots were geocoded for each neighbourhood separately to latitude – longitude point locations using ArcGIS 10.2 and subsequently aggregated to street segments. This helped to achieve the first objective.
(i) Determination of crime hot Spot Maps through the GIS Interpolation method (IDW).
The interpolation of the data is done by using the inverse distance weighting method. This method is used to find the unknown value of a particular point by taking the average weight of surrounding known points. The values of cells at locations that lack sampled points are used to predict crime points. This depends on the guideline of spatial autocorrelation or spatial reliance, which estimates the level of relationship and reliance among close and far-off items. The Inverse Distance Weighted (IDW) function is used for a set of points that is dense enough to capture the extent of local surface variation needed for analysis. Interpolation is done for victimization points with known values and proposed values at alternate unknown points. It is meant for the prediction of the new data point, which is missing from the dataset with the help of known discrete data points.
The IDW as a predictor is a weighted average observation given by:
Where,v
for x/∈ {x1, …, xn} and vk(xi) = 1 if i = k and 0 otherwise. Thus, by definition, ˆy(x) interpolates the data. The weighting function wk(x) is chosen so that the prediction at x is influenced more by the nearby points than the distant points.
To achieve the second objective, we searched for the socio-demographic characteristics of the neighbourhoods’ road segments, obtained with the use of a structured questionnaire. The sample size of 321 buildings was determined from the police report. We locate the buildings within street segments. The total population of buildings counted from Google Maps was 2,180. All neighbourhoods were allotted 29 copies of the questionnaire, except the Ijomu (market area) with high population and density, which was allotted 31 copies of the questionnaire. All copies of the questionnaire were duly returned (321) amounting to a 100% response rate.
Inquiries were made on the age, gender, education level, and employment status of residents (socio-demographics) similar to prior studies linking crime (Kim, Citation2018; Wo, Citation2019; Haberman & Kelsay, Citation2020; Hipp et al., Citation2020).
Crime hotspots were further spatially analyzed and illustrated using the space syntax technique, where the dependent variable in the study is the count of residential burglaries in each street segment. Factors considered influencing the distribution of burglaries along each street segment in the study include factors such as permeability (ease of movement).
(ii) Applying the space syntax technique.
The application of the space syntax technique utilizes factors of permeability. These factors quantitatively describe street networks such as connectivity, control, local integration, and local depth. The road type is the hierarchical street layout in city planning in the study, it includes major arterial streets, collector streets, and local streets (2.7–3.6 m). Arterial streets (width 30–4.0 m) are supplementary transporting paths between arterial roads. Collector streets (width 4.0–4.6 m) connect to larger neighbourhoods. Cul-de-sacs are defined as street segments with only one inlet or one outlet and include ‘dead-end’ streets.
In the analysis of the street network, the space syntax methodology of Hillier (Citation2009) was applied to measure permeability. This measure refers to the number of lines directly connected to a given line in an axial map. The axial map is created from the open street map processed by the DepthMap-X 8.0 software.
The street integration is calculated for each street segment. The integration (I) of the axial line i is a function of its depth in relation to all the other axial lines. The latter is ‘calculated by assigning a depth value to each space considering the number of spaces away from the original space. The summation of these values is divided by the number of spaces in the system less one’ (Hillier & Hanson, Citation1984).
The formula for integration (I) is given as:
where n is the number of axial lines in the street considered, and dij is the shortest distance (least number of steps) between two axial lines i and j.
Lines with high connectivity values are deemed to be more utilized by pedestrians than other lines. The ‘depth’ concept was introduced for understanding the structure of the graph. The depth of an axial line is the number of neighbouring lines within a given number of steps. The connectivity and depth are defined from a topological point of view (Van Nes & Yamu, Citation2021). Combining the various space syntax measurements into one map demonstrates simultaneously the spatial potential of local neighbourhood centres and the degree of vitality of the main routes. The highest value of axial lines inside the grid determines the value of each grid cell. All measurements were categorized based on the value allocated, and a histogram was produced showing variation. The blue-coloured lines represent low values, the green represents the medium values, while the red represents high values (see, for example, Hillier and Shahbaz (Citation2005); Van Ness and López (Citation2010).
Control is a parameter that expresses the degree of choice each axial line represents for its directly linked neighbour lines. Control is a parameter that expresses the degree of choice each axial line represents for its directly linked neighbour lines.
The axial-line-based graph and corresponding connectivity and depth for a line can be calculated as follows: Connectivity = 3 as it has three immediate neighbour lines 2, 3, and 4 connecting with it (Baran et al., Citation2008). The local mean depth is the mean distance of axial lines within several steps from the studied axial line. The streets with the lowest Depth Distance values are said to be the nearest to all other streets. The search radius can be limited to any distance. Accordingly, the mean depth is the mean depth value of the studied axial line to all other axial lines. The local mean depth, on the other hand, is the mean distance of axial lines within several steps from the studied axial line.
Local integration represents accessibility and connectedness within a part of the entire spatial framework. This value is figured in just several shapes of a specific space, as a during three in depth. Axial local integration is portrayed as blend estimations of axial lines at radius 3 (root notwithstanding two topological steps from the root), which can be used to show a concentrated picture of integration. The red colour represents the highest values and then the green after the blue colour represents the least values.
(iii). Poisson regression analysis
To accomplish the third objective, the Poisson regression analysis was applied. Poisson regression models are generalized linear models with the logarithm as the link function, and the Poisson distribution functions as the assumed response probability distribution. The distribution of ln(λi) follows a Poisson distribution with the mean response related to the covariates via:
EquationEquation 1(1)
(1) is a regression equation relating the natural logarithm of the mean or expected number of events for case i, ln(λi), to the sum of the products of each explanatory variable, xik, multiplied by a regression coefficient, βk (where β0 is a constant multiplied by 1 for each case). EquationEquation 2
(2)
(2) indicates that the probability of yi, the observed outcome for this case, follows the Poisson distribution (the right-hand side of the equation) for the mean count from Eq. (1), λi. Thus, the expected distribution of crime counts, and the corresponding distribution of regression residuals depend on the fitted mean count, λi
Poisson distribution is characterized by its mean equal to its variance. Therefore, as the mean count increases, the probability of observing any specific number of events declines, and a broader range of values have meaningful probabilities of being observed. Occasionally the observed variance may be larger than the mean, which is known as overdispersion, and this would render the model inappropriate.
4. Data analysis and results
4.1. Residential burglary hotspots and socio-demographic factors
A total of 321 residential burglaries were recorded in the Akure core area, according to the police report, between October 2021 and October 2022, with a mean of 6.2927 and a standard deviation of 3.65714. Each record in the dataset includes the XY coordinates of the crime location (using the World Geodetic System (1984). This was further used to prepare a map of crime levels by location based on socio-demographic factors in .
shows the IDW (Inverse Distance Weight) results using socio-demographic and location factors. It shows the high intensity of crime along the major transport route cutting through the Akure core. Crime occurrence is found very high at the inner core around the Odo-Ikoyi, Wema bank area, and Boro Street but moderates around the Arakale road, Alafiatayo street, while very low crime is reported around Imuagun street, Salvation Army Primary School area, Saint David’s Anglican church area. Other areas of high crime around the core include Odundun street, Eleye street, Odo-Ikoyi Street, Isolo street, Oke-Ijebu street, and Eru-oba street (Appendix i).
4.2. Residential burglary risk areas and pedestrian movement factors
Potential residential burglary risk areas based on the pedestrian movement factors (permeability) are identified using space syntax techniques. Based on the axial line graphs, space syntax analysis focuses on how streets are connected, and a series of accessibility measures are calculated for the graphs. Since the accessibility measures were initially determined for axial lines, an axial-line-based graph is used as an instance for illustrating some useful measures in space syntax in the following paragraph ().
Figure 4. Streets accessibility of the study area: (a) Connectivity (b) Control (c) local mean depth (d)local integration.

The red-coloured lines (streets) depict the more active movement of pedestrians with more street integration. They are the most important streets in the city core, they are areas of mixed-use with residential and commercial activities. Since these streets are located in a more integrated and less separated area, they form potential areas for criminal activities like theft and burglary. While streets that represent green and blue are less integrated streets and more secession and some streets have closed ends. is the result of the analysis of the maps in showing the spatial level of accessibility of street segments, where streets with connectivity line values are in the range of 7.6–9.0 constituting 35% of the streets, while 3.6–7.5 constitutes 50%, and 1.0–3.5 constitutes 15%. The street control also shows lines with a colour range of high control at the range of 3.0–5.06 constituting 60%, while 1.0–1.3.0 constitutes 25%, and 0.01–1.0 constitutes 15%. The depth of the streets ranges from 1.8 to 1.9 constituting 25%, while 1.0–1.8 constitutes 60%, and 0 > 1.0 also constitutes 10%. The local integration depicts the range of 4.0–8.0 constituting 79.8%, while 2.0–4.0 constitutes 36.8% and 0–2.0 constitutes 2.63% ()
Table 1. Spatial level of accessibility of street segments.
4.3. Association of socio-demographic and permeability factors with residential burglary occurrences
is the outcome of the superimposition of the crime hot spot map generated from socio-demographic factors and that generated from permeability factors (integration). The outcome illustrates the same area of crime spots, which is consistent with the effectiveness of the space syntax methodology as a complementary method of determining crime sports.
The association of socio-demographic and permeability factors with residential burglary is determined using the Poisson regression analysis. The results from the goodness-of-fit show a ratio of values/df less than 1 (.845, .771), which indicates under-dispersion, hence the analysis can continue. If this had been otherwise where the value/df is more than 1, it would have been described as over-dispersion and problematic; which could have required moving from Poisson regression to a negative binomial regression model. Also, since we used police reports of only buildings that experienced burglary, there were no zero experienced buildings or else there will have been zero buildings, which will prompt us to use a Zero-Inflated Negative Binomial (ZINB) regression model to fit the dataset, which will account for both overdispersion and excess zero problems (Osborne, Citation2017), (Appendix-ii).
shows parameter estimates of the occurrence of residential burglary cases and their association with socio-demographic variables. Where the predicted log count of gender compares male and female occurrences in the crime. The output shows no difference between male and female participation in crime. However, the predicted log count of age (26–35) increases by.098. The incidence ratio indicates that for every unit of age (26–35) increase, a 10.3% increase in residential burglary will occur, ceteris paribus (b = .098, S. E = .2205, p < .058).
Table 2. Parameter estimates.
The predicted log count of employment level (artisans) was negative and significant (b = −.058, S. E = .0861, p < .098) indicating that for every one unit increase in the employment level of this category, the rate of residential burglary will reduce by 5.7%.
The occurrence of residential burglary cases is also found to be associated with the factors of space syntax. Where the predicted log count of connectivity was positive and significant (b = .283, S. E = .3322, p < .095) indicating that for every unit increase in connectivity, the rate of residential burglary will increase by 32.7%. However, the predicted log count of median control was negative and significant (b = −.023, S.E. = .0767, p < .066) indicating that for every unit increase in median control, the rate of residential burglary will decrease by 2.3%, ceteris paribus.
The predicted log count of local mean depth (medium) was positive and significant (b = .139, S.E. = .0886, p < .007) indicating that for any one unit increase in local mean depth (medium), the rate of residential burglary will increase by 15%, ceteris paribus.
The predicted log count of local integration (medium) was positive and significant (b = .151, = 0959, p < .016) indicating that for any unit increase in local integration (medium), the rate of residential burglary will increase by 16.3%, ceteris paribus.
also shows that the occurrence of residential burglary cases is associated with the type of road segment. Where the predicted log count of the local street was positive and significant (b = .064, S.E. = .0997, p < .023) indicating that for any one unit increase in a local street, the rate of residential burglary will increase by 6.0%, ceteris paribus.
The predicted log count of the arterial road was negative and significant (b = −.2.44, S.E. = .1330, p < .067) indicating that for any one unit increase in the arterial road, the rate of residential burglary will decrease by 21.6%. Similarly, the predicted log count of the cul-de-sac was negative and non-significant (b = −.164, S.E. = 2.332, p < .309) indicating that for any one unit increase in a cul-de-sac, the rate of residential burglary will decrease by 15.1%, ceteris paribus.
5. Discussion
A plethora of the literature largely engrossed on the consequences of the built environment on crime. While they offer crucial knowledge for understanding different causes of burglary across places, very few studies have investigated the association between socio-demographic (age, gender, education level, and employment) and permeability factors (street connectivity, local control, local mean depth, and integration) on spatial crime patterns. The findings of this research showed that gender does not have an association with burglary, though in most literature burglary is associated with males. The age category of 26–35 has been found to be associated with crime with 10.3% per unit change. This is to say that the higher the percentage of youth concentration, the more negative the relationship between connectivity and burglary, with other factors remaining equal. This is consistent with the findings of Kim and Wo (Citation2021).
Street connectivity was found to be highly associated with overall burglary counts followed by median local control, local mean depth, and integration; this increases permeability, i.e. free movement. That is to say, neighbourhoods that offered highly accessible routes to pedestrians also offered criminals easy routes of escape. To elaborate on this finding, street spaces that had low integration values were safer (where more eyes can be on the street). High connectivity was also found associated with high burglary areas, as it allows free movement for strange fellows that cannot be identified and hence high crime potential, this is in agreement with Jacobs (Citation1961); Hipp and Kim (Citation2019), and Kim and Wo (Citation2021).
The model features other factors such as road segment type, local street, arterial road, and cul-de-sacs. The model shows that every unit increase in the local street increases the rate of burglary due to the small width and high connectivity to other roads, but an increased unit of the arterial road will reduce the burglary rate. This could be due to the widening size of the road and a smaller number of buildings compared to the former, perhaps these results can be related to the effects of ‘eyes on the street’. This is similar to the findings of previous studies by Hillier and Shu (Citation2000); Van Nes and Yamu (Citation2021). The foregoing confirms the hypotheses, that there is a relationship between socio-demographic factors and pedestrian movement patterns with crime hot spots within the city core of the study area. The results also confirm the existence of a relationship between street permeability factors and burglary, suggesting that the use of space syntax has considerable potential.
6. Conclusion
The study has shown that small areal units can capture the spatial patterns of crime, with less obscurence of the locations of crime hotspots, showing the distinct reality of hotspots. The study outcome showed the patterns and locations of hotspots of burglary within the neighbourhoods. The results of this research are considered a potential contribution of criminological research to the 2030 Agenda for Sustainable Development. The issues identified herein merely denote some logical starting points for criminological engagement with Sustainable Development Goals (SDGs). The findings of crime spots could be prioritized by concentrating security measures at crime spots to curb crime and discourage crime attempts to secure the city neighbourhoods and allay the fear of crime in the cities for sustainable development.
Ethical approval
All procedures performed in studies involving human participants were in accordance with the ethical standards of the institutional and/or national research committee and with the 1964 Helsinki declaration and its later amendments or comparable ethical standards.
Informed consent
Informed consent was obtained from all individual participants included in the study.
Disclosure statement
No potential conflict of interest was reported by the authors.
Additional information
Funding
References
- Alabi, M. O. (2021). Space syntax: Evaluating the influence of urban form and socio-economy on walking behaviour in neighbourhoods of Akure, Nigeria. Urban, Planning, and Transport Research, 9(1), 581–21. Publisher Taylor and Francis group www.tandfonline.com https://doi.org/10.1080/21650020.2021.2003234
- Ándresen, M. A., & Malleson, N. (2011). Testing the stability of Crime paaterns : Implications for theory and policy. J. Res Delinq, 48(1), 58–82.
- Armitage, R., Monchuk, L., & Rogerson, M. (2010). It looks good, but what is it like to live there? Exploring the impact of innovative housing design on crime. European Journal on Criminal Policy Research, 17(1), 1–26. https://doi.org/10.1007/s10610-010-9133-8
- Baran, P. K., Rodríguez, D. A., & Khattak, A. J. (2008). Space syntax and walking in new urbanist and suburban neighbourhoods. Journal of Urban Design, 13, 5–28. https://doi.org/10.1080/13574800701803498
- Bernasco, W., & Block, R. (2011). Robberies in Chicago: A block-level analysis of the influence of crime generators, crime attractors, and offender anchor points. The Journal of Research in Crime and Delinquency, 48(1), 33–57. https://doi.org/10.1177/0022427810384135
- Bevis, C., Nutter, J. B., & Center, M. C. P. (1977). Changing street layouts to reduce residential burglary. Minnesota Crime Prevention Center.
- Boessen, A., & Hipp, J. R. (2015). Close-ups and the scale of ecology: Land use and the geography of social context and crime. Criminology, 53(3), 399–426. https://doi.org/10.1111/1745-9125.12074
- Boivin, R., & Felson, M. (2017). Crimes by visitors versus crimes by residents: the influence of visitor inflows. In Journal of Quantitative Criminology (Vol. 34(2), 465–480). doi:10.1007/s10940-017-931-1.
- Brantingham, P. L., & And, P. J. B. (2008). The rules of crime pattern theory. In R. Wortley & L. Mazerolle (Eds.), Environmental criminology and crime analysis (pp. 5–25). Devon. U.K: Willan Publishing.
- Brantingham, P. J., & Brantingham, P. L. (1993). Environment, routine, and situation: towards a pattern theory crime. In R. V. Clarke & M. Felson (Eds.), Routine activity and rational choice. advances in criminological theory (Vol. 5, pp. 87–100). Transactions Books.
- Carmona, M., Heath, T., Oc, T., & Tiesdell, S. (2003). Public places urban spaces: The dimensions of urban design. Architectural Press).
- Carr, M. M. (2020). Urban Hostility: CPTED, Hostile Architecture and the Erasure of Democratic Public Space. An unpublished B.Sc. Architecture Thesis submitted to School of Architecture, Portland State University,
- Cohen, L. E., & Felson, M. (1979). Social change and crime rate trends: a routine activity approach. American Sociological Review, 44, 588–608. https://doi.org/10.2307/2094589
- Cozens, P. (2007). Planning, crime, and urban sustainability”, in A. Kungolas, C. Brebbia, & E. Beriatos (Eds.) Sustainable Development, and Planning III, Vol. I. WIT Transaction on Ecology and the Environment, 102 , 187–196. Southampton WITPress.
- Cozens, P. M., Love, T., & Davern, B. (2019). Geographical juxtaposition: A new direction in CPTED. Journal of Social Sciences, 8(252), 2–22. https://doi.org/10.3390/socsci8090252
- Dambazau, A. B. (2007). The Nigerian police and crime prevention: Criminology and criminal justice Nigerian, 5 Kaduna. Defence Academy Press.
- Davies, T., & Johnson, S. D. (2014). Examining the relationship between road structure and burglary risk via quantitative network analysis. Journal of Quantitative Criminology, 31(2015), 481–507. https://doi.org/10.1007/s10940-014-9235-4
- Dempsey, N. (2008). Quality of the built environment in urban neighbourhoods. Planning Practice and Research, 23(2), 249–264. https://doi.org/10.1080/02697450802327198
- Dovey, K. (1998) Safety and danger in urban design. Paper presented at the conference Safer Communities: Strategic Directions in Urban Planning, Australian Institute of Criminology and Victorian Community Council Against Violence, Melbourne, 10–11 September.
- Du Plessis, C. (1999). The links between crime prevention and sustainable development. Open House International, 24(1), 33–40.
- Frith, M. J., Johnson, S. D., & Fry, H. M. (2017). Role of the street network in burglars’ spatial decision-making. Criminology, 55(2), 344–376. https://doi.org/10.1111/1745-9125.12133
- Gehl, J. (2001). Life between buildings: Using public space. Arkitektens Forlag).
- Glasson, J., & Cozens, P. (2011). Making communities safer from crime: An undervalued element in contemporary environmental assessment (EA) practice. Environmental Impact Assessment Review, 31(1), 25–35. https://doi.org/10.1016/j.eiar.2010.03.007
- Haberman, C. P., & Kelsay, J. D. (2020). The topography of robbery: Does slope matter? Journal of Quantitative Criminology, 37, 625–645. https://doi.org/10.1007/s10940-020-09451-z
- Hillier, B. (2004). Can streets be made safe? Urban Design International, 9(1), 31–45. https://doi.org/10.1057/palgrave.udi.9000079
- Hillier, B. (2009) “The now and the future of space syntax: From structures and models to the theory”, keynote speech, 9th International Space Syntax Symposium Seoul, Sejong University, South Korea.
- Hillier, B., & Hanson, J. (1984). The sociallogic of space. Cambridge University Press.
- Hillier, B., & Iida, S. (2005) Network and psychological effects in urban movement. In: A. J. Cohn & D. M. Mark () () () (Eds.), Proceedings of Spatial Information Theory: International Conference, COSIT 2005, Ellicotts ville, N.Y ., U.S.A, Springer, Berlin (pp. 475–490).
- Hillier, B., & Shahbaz, O. (2005) “High-resolution analysis of crime patterns in urban street networks: An initial statistical sketch from an ongoing study of a London borough”, proceedings, 5th International Space Syntax Symposium Delft, (pp. 451–478). London.
- Hillier, B., & Shu, S. (2000). Crime and urban layout: The need for evidence. (S. Ballintyne, K. Pease, & V. McLaren, Eds.). Institute of Public Policy Research).
- Hipp, J. R., & Kim, Y. -A. (2019). Explaining the temporal and spatial dimensions of robbery: Differences across measures of the physical and social environment. Journal of Criminal Justice, 60, 1–12. https://doi.org/10.1016/j.jcrimjus.2018.10.005
- Hipp, J. R., & Kim, Y -A. (2020). Both sides of the street: Introducing measures of physical and social boundaries based on differences across sides of the street, and consequences for crime. Journal of Quantitative Criminology. https://doi.org/10.1007/s10940-020-09484-4
- Jacobs, J. (1961). The death and life of great American cities. Jonathon Cope).
- Johnson, S., & Bowers, K. (2010). Permeability and burglary risk: Are cul-de-sacs safer? Journal of Quantitative Criminology, 26(1), 89–111. https://doi.org/10.1007/s10940-009-9084-8
- Jones, M., & Fanek, M. (1997). Crime in the Urban environment. Proceedings of Space Syntax First International Symposium, London.
- Kim, Y. -A. (2018). Examining the relationship between the structural characteristics of place and crime by imputing census block data in street segments: Is the pain worth the gain? Journal of Quant Criminal, 34(1), 67–110. https://doi.org/10.1007/s10940-016-9323-8
- Kim, Y. -A., & Wo, J. C. (2021). Topography and crime in place: The effects of elevation, slope, and betweenness in San Francisco street segments. Journal of Urban Affairs. https://doi.org/10.1080/07352166.2021.1901591
- Koohsari, M. J., Badland, H., & Giles-Corti, B. (2013). (Re)designing the built environment to support physical activity: Bringing public health back into urban design and planning. Cities, 35, 294–298. https://doi.org/10.1016/j.cities.2013.07.001
- Koohsari, M. J., Sugiyama, T., Lamb, K. E., Villanueva, K., & Owen, N. (2014). Street connectivity and walking for transport: Role of neighborhood destinations. Preventive Medicine, 66, 118–122. https://doi.org/10.1016/j.ypmed.2014.06.019
- Legewie, J. (2018). Living on the edge: Neighborhood boundaries and the spatial dynamics of violent crime. Demography, 55(5), 1957–1977. https://doi.org/10.1007/s13524-018-0708-1
- Malleson, N., Steenbeek, W., & Andresen, M. A. (2019). Identifying the appropriate spatial resolution for the analysis of crime patterns. PLoS One, 14(6), e0218324. https://doi.org/10.1371/journal.pone.0218324
- Newman, O. (1972). Defensible Space. Macmillan.
- Newman, O. (1996). Creating defensible space, center for Urban policy research. Rutgers University, U.S, Department of Housing and Urban Development.
- Nubani, L. (2003) The role of space syntax in describing the effect of urban configuration on pedestrian movement. Paper presented at EDRA 34, Minneapolis, Minnesota.
- Oberwittler, D., & Wikstrom, H. (2009). Why small is better: Advancing the study of the role of behavioural contexts in crime causation. In D. Weisburd, W. Bernasco, & G. Bruinsma (Eds.), Putting crime in its place: Units of analysis in spatial crime research (pp. 35–59). Springer.
- Osborne, J. W. (2017). Regression and linear modeling: Best practices and modern methods. Sage.
- Population Stat. (2022, August 3) World Statistical Data. https://populationstat.com/nigeria/akure
- Rudlin, D., & Falk, N. (1999). Building the 21st century home: The sustainable urban neighbourhood. Architectural Press.
- Sampson, R. J., & Groves, W. B. (1989). Community structure and crime: testing social- disorganization theory. The American Journal of Sociology, 94. (4, 774–802. https://doi.org/10.1086/229068
- Silva, P., & Li, L. (2020). Urban crime occurrences in association with built environment characteristics: An African case with implications for Urban design. Journal of Sustainability, 12(3056), 1–22. https://doi.org/10.3390/su12073056
- Soh, M. B. C. (2012). Crime and urbanization: Revisited Malaysian case. Procedia – Social and Behavioral Sciences, 42, 291–299. https://doi.org/10.1016/j.sbspro.2012.04.193
- Song, J., Spicer, V., Brantingham, P., & Frank, R. (2013, August 12-14). Crime ridges: Exploring the relationship between crime attractors and offender movement. In IEEE European intelligence and security informatics conference (EISIC), Uppsala, Sweden, pp. 75–82. doi: 10.1109/EISIC.2013.18
- United Nations. (2015) ‘Draft doha declaration on integrating crime prevention and criminal justice into the wider United Nations Agenda to address social and economic challenges and to promote the rule of law at the national and international levels, and public participation, A/CONF.222/L.6, https://www.unodc.org/documents/congress/Accessed 15 August. 2017
- Van Ness, A., & López, M. (2010). Macro and micro-scale spatial variables and the distribution of residential burglaries and theft from cars: An investigation of space and crime in the Dutch cities of Alkmaar and Gouda. Journal of Space Syntax, 2(1), 296–314.
- Van Nes, A., & Yamu, C. (2021). Introduction to space syntax in Urban studies. 1–250. Springer.
- Weisburd, D. (2015). The law of crime concentration and the criminology of place. Criminology, 53(2), 133–157. https://doi.org/10.1111/1745-9125.12070
- Weisburd, D., Groff, E. R., & Yang, S. -M. (2012). The criminology of place: Street segments and our understanding of the crime problem. Oxford University Press.
- Weisburd, D., & Zastrow, T. (2022). Does a large crime decline mean that hot spots of crime are no longer ‘hot’?: Evidence from a study of New York city street segments. Policing: A Journal of Policy and Practice, 16(4, December 2022), 591–601. https://doi.org/10.1093/police/paab080
- White, G. F. (1990). Neighbourhood permeability and burglary rates. Justice Quarterly, 7(1), 57e67. https://doi.org/10.1080/07418829000090471
- Wo, J. C. (2019). Mixed land use and neighborhood crime. Social Science Research, 78, 170–186.
- Wu, L., Liu, X., Ye, X., Leipnik, M., Lee, J., & Zhu, Z. (2015). Permeability, space syntax, and the patterning of residential burglaries in urban China. Applied Geography, 60(2015). ELSEVIER. https://doi.org/10.1016/j.apgeog.2014.12.001.
- Wuschke, K. E., Bryan, J. K. et al. (2018). Built environment, land use and crime. In G. J. N. Bruinsma & S. D. Johnson (Eds.), Oxford handbook of environmental criminology (pp. 1–30). Alexandrine press.
- Wu, L., Xu, X., Ye, X., & Zhu, X. (2014). Repeat and near-repeat burglaries and offender involvement in a large Chinese city. Cartography and Geographic Information Science, 42(2), 178–189. https://doi.org/10.1080/15230406.2014.991426