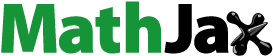
ABSTRACT
The rise in automobiles within rapidly developing nations in Sub-Saharan Africa necessitates the exploration of sustainable transportation alternatives, especially in urban regions. Walking and cycling are essential active modes of transport that can improve city residents’ well-being and economic vitality. However, their adoption as mobility choices depends on infrastructure limitations and residents’ perception of their safety. This study investigates the impact of socio-demographic and neighborhood characteristics on the safety perception of active mobility within the Accra metropolis of Ghana. The study utilized survey data on residents’ mobility habits and willingness to use active transport modes for daily commutes. Most of the respondents are permanent residents of Accra, and 51% are between 18–35 years old. The developed random parameter ordered logit model reveals that the perception of safety varies across the population and is influenced by respondents’ demographic characteristics and neighborhood configuration. Notably, factors related to walking duration to bus stops, income level, and household size, unplanned neighborhoods, among others, decrease residents’ safety perception of active mobility. The study offers valuable insights that can aid in planning future urban active mobility systems in Accra to establish safer and more sustainable transportation choices for the city’s residents.
1. Introduction
The rising vehicle population in the low- and middle-income countries (LMIC) of Sub-Saharan Africa requires the exploration of sustainable alternatives, especially in urban areas, to accelerate the socio-economic development that will make these areas livable. Today, 55% of the global population lives in urban areas, projected to increase to 68% by 2050, according to the United Nations (United Nations, Citation2018). Parallel to this statistic, a report by Essel and Spadaro (Citation2020) noted that Accra, Ghana, is one of the fastest-growing cities in Africa, with an annual population increase of 2%. Additionally, the report indicated that the population is expected to grow from 4.5 million to 9.6 million by 2050, stoking an increase in transport demand. With such a rise in population in urban areas like Accra comes the need to promote a road environment that is less carbon-dependent and traffic-congested while also decreasing the associated negative impacts that typically accompany these urban roadways.
LMICs face many challenges regarding mobility in urban environments due to transportation systems that are expensive, unreliable, and inefficient. In LMICs, a significant percentage of the populace relies on non-motorized transportation and paratransit since owning a private vehicle is generally too expensive (Godefrooij & Schepel, Citation2010). Active transport is typically the mode used to travel by the lower-income population in LMICs (Godefrooij & Schepel, Citation2010; Lucas, Citation2011; Oyeyemi & Larouche, Citation2018). However, private vehicle ownership in Ghana is rapidly increasing, and with it comes rising air pollution, traffic congestion, noise, and depletion of the vegetative cover through road construction (Ajayi et al., Citation2023; Amegah & Agyei-Mensah, Citation2017; Armah et al., Citation2010; Garcia et al., Citation2021; Obeng-Odoom, Citation2009). According to projections, in Ghana, the proportion of passenger kilometers accounted for by active modes of transportation such as walking is expected to decrease from 37.6% to 35.3% between 2015 and 2030, while cycling is predicted to remain unchanged at a mere 0.1% during the same period (Essel & Spadaro, Citation2020). However, by examining the factors influencing bicycle use/ownership in Tamale, Ghana’s Metropolis, Timpabi et al. (Citation2023) found a latent desire to shift modes that exist among 85% of bicycle riders. Perhaps the decreasing trend in active mobility patronage can be attributed to the users’ safety perceptions of the modes.
Conversely, the modal share by passenger-kilometers is projected to increase from 13.7% in 2015 to 21.2% in 2030 for cars, taxis, and motorcycles. It should be noted that urban buses and electric mass transit are also experiencing a decreasing trend (Essel & Spadaro, Citation2020). Further, the trends Ghana is experiencing are expected to extend to 2050. This and Accra’s expected population growth through 2050 pose many mobility, environmental, and economic challenges. With the rising population comes the need to create an environment that encourages sustainable mobility. Active transport can help mitigate the adverse effects of motorized transport in urban areas while providing many benefits. Aside from the socio-economic and environmental importance of sustainable transport, active mobility is associated with health benefits such as the reduced risk of chronic ailments like diabetes, cancer, or heart disease, improved sleep, and reduced anxiety (CDC, Citation2021).
Many studies have been conducted to better understand active mobility as a transportation mode choice (Hackl et al., Citation2019; Koszowski et al., Citation2019; Markvica et al., Citation2020; Pisoni et al., Citation2022). Some have shown that safety is critical when deciding to use active modes over short distances and that safety concerns may be the most significant barrier to higher use of active mobility.
A study by Rojas López and Wong (Citation2017) investigated users’ attitudes toward active mobility in Singapore and found that safety concerns about walking tend to increase among adult and elderly pedestrians when heavy traffic is on the adjacent roadway. Additionally, it was noted that pedestrians were worried for their safety along narrow shared facilities. Similar results were yielded from a study by Aziz et al. (Citation2018) on the impact of various factors on active transport mode choice in New York City using a random parameter model. The authors also indicated that reducing the number of traffic crashes involving pedestrians and bicyclists would increase the safety perception and the likelihood of taking active modes.
On the other hand, Fishman et al. (Citation2012) suggested that regarding the fear of bicycle riding as a mode in Australia, a person’s fear was more plausibly related to the (more frequently occurring) near collision than actual collisions, while Heinen et al. (Citation2011) found that a person’s perception of safety only impacted their bicycle usage as a mode over short distances when studying the role of attitudes toward bicycle commuting over various distances. Later, when analyzing cycling for transport, Fishman (Citation2016) suggested the role of reallocating road space to overcome perceived and actual safety fears of bicycling as a mode. Hidayati et al. (Citation2020) explored the gender differences and safety perceptions of urban mobility in Southeast Asia and found that safety was based on spatial and sociocultural features. It was also observed that women generally had a negative perception of safety for walking in urban environments.
A study by Jamal and Mohiuddin (Citation2020) on the indicators of active transport in Rajshahi, Bangladesh, noted that safety is a crucial component for determining the cycling rate of a locality. Indeed, it was observed that locations with high cycling rates were also related to less traffic and people’s improved safety feeling in those areas. Additionally, people’s safety perception of active modes was generally lower on main roadways, which experience higher traffic, and on local streets and in educational areas, people’s safety perception was generally high for both walking and bicycling.
While Jamal and Mohiuddin (Citation2020) created a baseline for which safety perception of active modes in developing countries like Bangladesh can be analyzed, to the best of the authors’ knowledge, at the time of conducting this study, there are no existing studies that have attempted to capture the effects of socio-demographic factors on safety perception of active mobility in Sub-Saharan Africa.
In Ghana, pedestrian fatalities are increasing (Building & Road Research Institute, Citation2023). This provides an impetus for a study to understand active mobility users’ characteristics and safety perceptions. Thus, the primary objective of this study is to capture the effects of socio-demographic and neighborhood factors on the safety perception of active mobility in Accra, Ghana. This is accomplished by developing a random parameter ordered logit (RPOL) model to analyze survey data collected from Accra residents between January and June 2021 on their mobility habits and willingness to use active transport modes. By extension, this study aims to offer valuable insights that can aid in planning future urban active mobility systems in Accra to establish safer and more sustainable transportation choices for the city’s residents.
2. Data and method
2.1 Data description
The study data were collected by surveying Accra residents’ mobility habits and willingness to use active transport modes via an online platform. The survey was conducted between January and June 2021. In the survey, the respondents were asked to provide their perception of active mobility safety in their immediate neighborhoods. One of the main focuses of the survey examined in this study was to understand the influence of the socio-demographic factors and neighborhood attributes on residents’ safety perception of active mobility. The respondents were asked to rank their safety perception of active mobility based on the 4-Likert scale, where 1 = unsafe, 2 = somewhat unsafe, 3 = somewhat safe, and 4 = very safe. The data were cleaned to remove incomplete responses before conducting a preliminary analysis, including descriptive statistics. The final analysis was based on 1273 complete responses. The analysis data comprised 48.9% female, and 51.1% of the respondents are 18 to 35 years old. Also, 44% of the respondents are employed, and 26.4% are students. Those who usually walk for less than 15 minutes to the nearest bus stop made up 43.4% of the data, and 61.0% of the respondents are from well-planned neighborhoods. Regarding safety perception of active mobility, 81.7% of the respondents perceived active modes (i.e. walking and cycling) to be either somewhat safe or very safe. describes the variables relevant to the study, especially those included in the model.
Table 1. Descriptive statistics of the model variables.
shows the distribution of respondents across the four-safety perception category according to gender. The male and female respondents expressed similar perceptions of active mobility across the four categories. A higher proportion of females perceive active modes to be ‘very safe’ compared to their male counterparts, with a difference of 1.3%. The ‘somewhat safe’ perception has a higher proportion of male respondents.
shows the distribution of safety perception based on the respondents’ age group and walking duration to the nearest bus stop, respectively. From , most respondents under 18 years or above 50 feel somewhat safe about active mobility. Forty-four percent of those between 35–50 years old perceived walking and cycling to be very safe. About 45% of the respondents walk to the nearest bus stop for less than 15 minutes.
Figure 2. (a) safety perception by age group, (b) safety perception by walking duration to the bus stop.

showed a proportional decreasing trend in the respondents’ perception of active mobility as very safe. Some 46% of the employed respondents perceived active mobility as very safe followed by 36%, 34%, and 33% for the unemployed, students, and the retired, respectively. From , 51% of those who earn a monthly net income of 101–1000 Cedis perceived active mobility to be very safe followed by those whose income is between 1001–5000 Cedis at 30%. The lowest income earners, i.e. those that earn less than 100 Cedis have the most proportional perception of active mobility as unsafe at 6%.
2.2 Statistical modeling
The choice of a modeling approach primarily depends on the nature of the data and the study objectives or research questions. Different studies have used statistical modeling techniques to examine the relationships between a response variable and a set of independent variables. For discrete choice problems, researchers have often considered the response variable as either nominal (ordered) or categorical (unordered). For instance, the mode choice (e.g. cars, transit, bike, and walking) as a response variable is considered a categorical variable. On the other hand, customer service ratings ranging from poor to excellent can be regarded as ordered. If a service rating is measured on a 5-Likert scale, the marginal difference between consecutive numbers is assumed to be uniform.
This study considered the respondents’ safety perception of active mobility as ordered, increasing from unsafe to very safe. To estimate the factors contributing to the increasing or decreasing order of the response variable (i.e. safety perception), we developed an RPOL model. The RPOL model captures the dependent variable’s increasing order and accounts for observations’ heterogeneity through random coefficients (Azimi et al., Citation2020). The response variable, safety perception, is ordered as follows: 1 = unsafe, 2 = somewhat unsafe, 3 = somewhat safe, and 4 = very safe. The model is estimated by building a relationship between latent continuous function, that determines safety perception
; discrete safety perception categories; where 1 = unsafe, 2= somewhat unsafe, 3= somewhat safe and 4 = very safe) through a given threshold value denoted as
. The model’s response variable (i.e., safety perception) is intrinsically ordered while allowing the explanatory coefficients,
to vary across observations following a logit distribution. Accordingly, for each observation, the relationship between the dependent variable and the determinants is expressed in Eq. (1).
where is the latent function associated with safety perception across the observations,
is the vector of explanatory variables,
is the vector of estimable random coefficients, and
is the distributed error term.
By assuming that the random parameter follows a normal distribution, the probability of a response belonging to a safety perception category (j = 1,2,3,4) can be expressed as follows.
Average pseudo-elasticities of the independent variable were computed to supplement the model results in explaining the impact of explanatory variables on safety perception. A positive average pseudo-elasticity indicates a higher relational probability between a variable and the corresponding safety perception. On the other hand, a negative average pseudo-elasticity indicates a lower relational probability between a variable and the corresponding safety perception. It gives the percentage change in the probability of a choice (i.e., safety perception) when the value of a dummy variable changes from zero to one (Ulfarsson & Mannering, Citation2004). For a given response , the pseudo-elasticity is defined as expressed in Eq. (6).
where is the
indicator variable from response
, and
is the probability of response
having safety perception
. The average pseudo-elasticity is calculated as the mean of pseudo-elasticities estimated for all observations. A Halton draw (Halton, Citation1960) of 500 was implemented for the model maximum likelihood estimation. Studies have shown that the Halton draw approach generates more accurate approximations with fewer draws than regular random draws (Bhat, Citation2003; Train, Citation2003).
3. Results
The model estimates, including the average pseudo-elasticities, are presented in . Only variables whose t-value corresponded to a minimum of 0.1 significance level on a two-tailed t-test were included in the model specification. In total, 17 variables significantly influenced the respondents’ safety perception of active mobility. The significant variables about the respondents’ socio-demographic status include age (35–50 years old), level of education (secondary), employment status (employed), net monthly income (1001–5000 & 5001 to 10,000 Cedis), household size (4–7 & 8–12), living with children (yes), walking duration to the nearest bus stop (30–45 minutes & 45–60 minutes) and residence duration in Accra (5–10 years). The significant variables about the neighborhood are building proximity (not close) and road traffic rating (not too much traffic & some traffic during the day). Employment status (employed) and neighborhood traffic (some daily traffic) were estimated as random parameters. The random parameters were assumed to follow a normal distribution (Mannering et al., Citation2016). As random parameters, the standard deviations of both parameters were significant at a minimum of 0.1 significance level. The variables with positive estimates increase the respondents’ safety perception of active mobility. This implies that the respondents are more likely to feel very safe about active mobility in the presence of such variables. The results are further interpreted in the following sub-sections.
3.1 Socio-demographic factors
The variable employment status (employed) was estimated as a random parameter with a mean of 0.441 and a standard deviation of 0.884. These values imply that the probability of increasing safety perception of active mobility is high for 69.1% of the employed respondents. Whereas for the remaining 30.9% of the employed respondents, the probability of increasing safety perception is low. The results showed that walking for longer minutes to the nearest bus stop decreases the safety perception of active mobility. Walking for 31–45 minutes or 46–60 minutes to the nearest bus stop reduces the safety perception of active mobility. The estimates showed that walking for 46–60 minutes negatively impacts safety perception more than walking for 31–45 minutes. The estimated pseudo elasticities indicated that those who walk for 31–45 minutes and 46–60 minutes are 57.6% and 77.2% more likely to feel unsafe about active mobility, respectively.
In terms of households, a household of 4–7 people increases the safety perception of active mobility, whereas a household of 8–12 people decreases the safety perception of active mobility. However, living with children increases the respondents’ safety perception of active mobility.
Other variables that increased the safety perception of active mobility include age (35–50 years old) and education level (secondary). The pseudo elasticities showed that respondents between 35–50 years old and those with secondary school education are more likely to perceive active mobility to be very safe in their neighborhood, with a probability of 20.3% and 14.1%, respectively. Those who earn a net monthly income of 1001–5000 Cedis and 5001–10,000 Cedis are less likely to perceive active mobility as very safe, with a probability of −36.6% and −41.1%, respectively. further shows the pseudo elasticities of selected explanatory variables and their contributions to increasing or decreasing the probability of ‘very safe’ perception of active mobility.
Table 2. Model estimates.
3.2 Neighborhood factors
The neighborhood factor, road traffic (not too much traffic), was estimated as a random parameter with a mean of 0.764 and a standard deviation of 0.262. This means that the probability of increasing safety perception of active mobility is high for 99.8% of the respondents who rated the neighborhood traffic as not too much. Also, the variable neighborhood traffic (some daily traffic) increases the probability of safety perception. The variable unplanned neighborhood decreases the safety perception of active mobility. The pseudo elasticity shows that respondents from unplanned neighborhoods are 32.4% more likely to perceive active mobility as unsafe. On the other hand, housing proximity (not close) increased the safety perception of active mobility. The pseudo elasticity showed that respondents from neighborhoods where the houses are spaced out are 14.1% more likely to perceive active mobility as very safe.
4. Discussion
The increasing number of automobiles in rapidly developing nations in sub-Saharan Africa presents challenges for active mobility safety. Nevertheless, active mobility, such as walking and cycling, play a crucial role in enhancing the well-being of city dwellers and mitigating the impacts of traffic congestion and emissions while promoting sustainable development. Understanding the safety perception of active mobility among city residents and identifying the factors influencing such perceptions can facilitate the formulation and implementation of policies to promote such modes’ attractiveness. This study uses online survey data to examine Accra residents’ perception of active mobility safety in their neighborhoods.
An initial descriptive analysis of the data revealed that a higher proportion of female respondents perceived active mobility (walking or cycling) as unsafe compared to their male counterparts. A similar study also found that women generally held negative perceptions of walking in urban environments (Hidayati et al., Citation2020). This observation could be attributed to women’s vulnerability or susceptibility to threats, particularly in isolated or poorly lit areas. Also, research has shown that female pedestrians in developing nations tend to manifest greater levels of fear and insecurity in public spaces (Dameria & Fuad, Citation2021). A higher proportion of older people (i.e. those above 50 years) perceived active mobility as unsafe compared to other age groups. The literature has previously established that older pedestrians are more concerned about safety, especially along heavy traffic roadways (Rojas López & Wong, Citation2017). Compared to other income groups, a higher proportion of those who earn less than 100 Cedis monthly perceived active mobility as unsafe. This demographic is more likely to rely heavily on active modes of transportation due to their income constraints and potentially limited access to personal vehicles. Therefore, improving the safety of the active mobility environment would be critical in keeping this group from seeking non-sustainable alternative modes and potentially attracting non-users.
Further, a random parameter ordered logit model was developed to identify the significant socio-demographic and neighborhood factors increasing or decreasing the respondents’ safety perception of active mobility. The modeling results revealed some interesting findings that are consistent with the literature. For instance, it was found that longer walking duration to the nearest bus stop decreases the safety perception of active mobility. In other words, those who walk to the nearest bus stop for 31–60 minutes are more likely to perceive active mobility as unsafe. Longer walking duration could expose pedestrians to more potential traffic conflicts, especially in areas of certain cities in sub-Saharan Africa with inadequate pedestrian facilities and traffic enforcement. Previous studies have established that long-distance walking increases pedestrian exposure to traffic crash risks (Quistberg et al., Citation2017; Sukhai et al., Citation2021). As such, locating bus stops with shorter walking duration can encourage more people to walk and use public transit more frequently, especially low-income residents. Household size was another variable that impacted the safety perception of active mobility. For instance, the result showed that respondents from households of size 8–12 expressed a negative perception of active mobility compared to those of size 4–7. Perhaps the large households are composed of parents and ‘more children’ who rely primarily on active transport options for daily mobility.
Regarding neighborhood characteristics, the finding revealed that the safety perception of active mobility increases with a decrease in vehicular traffic. This finding aligns with a previous study by Jamal and Mohiuddin (Jamal & Mohiuddin, Citation2020). Their study in a similar context of a developing country found that safety perceptions of active mobility are usually lower on roadways with high vehicular traffic. They further noted that cycling rates are higher on roads with low vehicular traffic. Also, the findings showed that unplanned neighborhoods have a negative impact on respondents’ safety perception of active mobility. Respondents from the unplanned neighborhoods are 32.4% more likely to feel unsafe about active mobility. This observation shows that planning plays a critical role in active mobility safety. There are possibly no pedestrian walkways and cycling lanes in an unplanned neighborhood. In most instances, there is usually no separation between vehicular traffic and vulnerable road users in time and space. Separating vehicular and vulnerable road users’ traffic spatiotemporally and providing adequate green spaces within the residential areas can enhance residents’ safety perception of active mobility.
5. Conclusions
This present study investigated the impact of socio-demographic and neighborhood factors on residents’ perception of active mobility safety in Accra, Ghana. An online survey conducted between January and June 2021 collected information from the residents on their mobility habits and willingness to use active transport modes for their daily commutes. The final analysis was based on 1,273 completed responses. The study findings provide the basis for understanding the underlying factors impacting active mobility in Accra and, by extension, similar contexts within Sub-Saharan Africa. These findings offer valuable insights that can aid in planning future urban active transport systems in Accra to establish safer and more sustainable transportation choices for city dwellers. Some of the key findings are highlighted as follows:
Low vehicular traffic improves the safety perception of active mobility.
Longer walking duration to the nearest bus stops increases the safety concerns of active modes.
Unplanned neighborhoods and housing proximity create a poor safety perception of active modes.
Female respondents generally expressed poor safety perception of active mobility compared to their male counterparts.
Low-income earners expressed more concern about active modes’ safety than their counterparts.
These findings necessitate adopting appropriate measures to combat the poor safety perception of active mobility. For instance, Complete Streets – an approach to planning, designing, building, operating, and maintaining streets that facilitate safe access for all road users, should be considered in existing and future roadway designs (Smart Growth America, Citation2023). This approach would ensure that bike lanes and pedestrian facilities are separated from the main roadway or are protected to improve the perception of active mode safety on roads that experience high vehicular traffic. Complete Streets would also ensure that bus stops are within short walking distance to enhance the safety perception of active mobility.
Since unplanned neighborhoods were shown to decrease the safety perception of individuals, the perceived safety of active modes might be enhanced by implementing improved land management and control policy, accompanied by better future neighborhood design based on community and stakeholder engagement. These measures to improve neighborhood design would further increase housing unit spacing, which, based on this study’s findings, would also enhance the perceived safety of active modes. Despite expressing a poor perception of active mobility safety, low-income earners and female residents are more likely to use these modes for daily commuting or first and last-mile trips to public transit stops. As such, improving the safety of active mobility would significantly benefit these groups of users.
Governing road agencies in Ghana and similarly positioned countries should consider this study’s significant findings and recommendations. By implementing these recommendations, individuals are expected to perceive active modes as safer and thus increase their willingness to use them.
This present study offers valuable insights that can aid in planning future urban active mobility systems in Ghana and in similar contexts of sub-Saharan Africa. However, future studies can expand on this study’s findings by investigating additional contextual variables, especially those related to the built environment. The survey data scaling should be more detailed regarding the respondents’ profiles, focusing on abilities possessed by each category, including their physical limitations and their impact on the perception of active mobility safety. With a larger data size, further study can also examine the differences that might exist in the active mobility safety perception across the sub-region of the Accra metropolis to provide more customized countermeasures.
Disclosure statement
No potential conflict of interest was reported by the author(s).
Data availability statement
The study data and relevant survey questions will be made available upon reasonable request from the corresponding author.
Additional information
Funding
References
- Ajayi, S. A., Adams, C. A., Dumedah, G., Adebanji, O. A., Ababio-Donkor, A., Ackaah, W., & Kehinde, A. (2023). Public perceptions of vehicular traffic emissions on health risk in Lagos metropolis Nigeria: A critical survey. Heliyon, 9(5), e15712. https://doi.org/10.1016/j.heliyon.2023.e15712
- Amegah, A. K., & Agyei-Mensah, S. (2017). Urban air pollution in Sub-Saharan Africa: Time for action. Environmental Pollution, 220, 738–15. https://doi.org/10.1016/j.envpol.2016.09.042
- Armah, F., Yawson, D., & Pappoe, A. A. N. M. (2010). A systems dynamics approach to explore traffic congestion and air pollution link in the city of Accra, Ghana. Sustainability, 2(1), 252–265. https://doi.org/10.3390/su2010252
- Azimi, G., Rahimi, A., Asgari, H., & Jin, X. (2020). Severity analysis for large truck rollover crashes using a random parameter ordered logit model. Accident Analysis & Prevention, 135, 105355. https://doi.org/10.1016/j.aap.2019.105355
- Aziz, H. M. A., Nagle, N. N., Morton, A. M., Hilliard, M. R., White, D. A., & Stewart, R. N. (2018). Exploring the impact of walk–bike infrastructure, safety perception, and built-environment on active transportation mode choice: A random parameter model using New York City commuter data. Transportation, 45(5), 1207–1229. https://doi.org/10.1007/s11116-017-9760-8
- Bhat, C. R. (2003). Simulation estimation of mixed discrete choice models using randomized and scrambled Halton sequences. Transportation Research Part B: Methodological, 37(9), 837–855. https://doi.org/10.1016/S0191-2615(02)00090-5
- Building & Road Research Institute. (2023). Road traffic crashes in Ghana, statistics 2021. The Building & Road Research Institute.
- CDC. (2021). Physical activity - walking. Center for Disease Control and Prevention. https://www.cdc.gov/physicalactivity/walking/index.htm
- Dameria, C., & Fuad, A. H. (2021). Enhancing female pedestrians’ safety perceptions through the permeability of building frontages (case study: Blok M area). IOP Conference Series: Earth and Environmental Science, 673(1), 012040. https://doi.org/10.1088/1755-1315/673/1/012040
- Essel, D., & Spadaro, J. (2020). Health and economic impacts of transport interventions in Accra, Ghana.
- Fishman, E. (2016). Cycling as transport. Transport Reviews, 36(1), 1–8. https://doi.org/10.1080/01441647.2015.1114271
- Fishman, E., Washington, S., & Haworth, N. (2012). Understanding the fear of bicycle riding in Australia. Journal of the Australasian College of Road Safety, 23(3), 19–27. https://doi.org/10.3316/informit.772959788443259
- Garcia, L., Johnson, R., Johnson, A., Abbas, A., Goel, R., Tatah, L., Damsere-Derry, J., Kyere-Gyeabour, E., Tainio, M., de Sá, T. H., & Woodcock, J. (2021). Health impacts of changes in travel patterns in Greater Accra Metropolitan Area, Ghana. Environment International, 155, 106680. https://doi.org/10.1016/j.envint.2021.106680
- Godefrooij, T., & Schepel, S. (2010). Co-Benefits of Cycling-Inclusive: Planning and Promotion. CID: 20.500.12592/t7ps2w. Retrieved December 20, 2022, from https://policycommons.net/artifacts/1505993/co-benefits-of-cycling-inclusive/2169777/on.
- Hackl, R., Raffler, C., Friesenecker, M., Kramar, H., Kalasek, R., Soteropoulos, A., Wolf-Eberl, S., Posch, P., & Tomschy, R. (2019). Promoting active mobility: Evidence-based decision-making using statistical models. Journal of Transport Geography, 80, 102541. https://doi.org/10.1016/j.jtrangeo.2019.102541
- Halton, J. H. (1960). On the efficiency of certain quasi-random sequences of points in evaluating multi-dimensional integrals. Numerische Mathematik, 2(1), 84–90. https://doi.org/10.1007/BF01386213
- Heinen, E., Maat, K., & van Wee, B. (2011). The role of attitudes toward characteristics of bicycle commuting on the choice to cycle to work over various distances. Transportation Research, Part D: Transport & Environment, 16(2), 102–109. https://doi.org/10.1016/j.trd.2010.08.010
- Hidayati, I., Tan, W., & Yamu, C. (2020). How gender differences and perceptions of safety shape urban mobility in Southeast Asia. Transportation Research Part F: Traffic Psychology and Behaviour, 73, 155–173. https://doi.org/10.1016/j.trf.2020.06.014
- Jamal, S., & Mohiuddin, H. (2020). Active transportation indicators and establishing baseline in a developing country context: A study of Rajshahi, Bangladesh. Growth and Change, 51(4), 1894–1920. https://doi.org/10.1111/grow.12420
- Koszowski, C., Gerike, R., Hubrich, S., Götschi, T., Pohle, M., & Wittwer, R. (2019). Active mobility: Bringing together transport planning, urban planning, and public health. https://doi.org/10.1007/978-3-319-99756-8_11
- Lucas, K. (2011). Making the connections between transport disadvantage and the social exclusion of low income populations in the Tshwane region of South Africa. Journal of Transport Geography, 19(6), 1320–1334. https://doi.org/10.1016/j.jtrangeo.2011.02.007
- Mannering, F. L., Shankar, V., & Bhat, C. R. (2016). Unobserved heterogeneity and the statistical analysis of highway accident data. Analytic Methods in Accident Research, 11, 1–16. https://doi.org/10.1016/j.amar.2016.04.001
- Markvica, K., Millonig, A., Haufe, N., & Leodolter, M. (2020). Promoting active mobility behavior by addressing information target groups: The case of Austria. Journal of Transport Geography, 83, 102664. https://doi.org/10.1016/j.jtrangeo.2020.102664
- Obeng-Odoom, F. (2009). Drive left, look right: The political economy of urban transport in Ghana. International Journal of Urban Sustainable Development, 1(1–2), 33–48. https://doi.org/10.1080/19463130903561475
- Oyeyemi, A. L., & Larouche, R. (2018). Prevalence and correlates of active transportation in developing countries. In Children’s active transportation (pp. 173–191). Elsevier. https://doi.org/10.1016/B978-0-12-811931-0.00012-0
- Pisoni, E., Christidis, P., & Navajas Cawood, E. (2022). Active mobility versus motorized transport? User choices and benefits for the society. Science of the Total Environment, 806, 150627. https://doi.org/10.1016/j.scitotenv.2021.150627
- Quistberg, D. A., Howard, E. J., Hurvitz, P. M., Moudon, A. V., Ebel, B. E., Rivara, F. P., & Saelens, B. E. (2017). The relationship between objectively measured walking and risk of pedestrian–motor vehicle collision. American Journal of Epidemiology, 185(9), 810–821. https://doi.org/10.1093/aje/kwx020
- Rojas López, M. C., & Wong, Y. D. (2017). Attitudes towards active mobility in Singapore: A qualitative study. Case Studies on Transport Policy, 5(4), 662–670. https://doi.org/10.1016/j.cstp.2017.07.002
- Smart Growth America. (2023). Complete streets. https://smartgrowthamerica.org/what-are-complete-streets/
- Sukhai, A., Govender, R., van Niekerk, A., & Tu, W. (2021). Fatality risk and issues of inequity among vulnerable road users in South Africa. PLOS ONE, 16(12), e0261182. https://doi.org/10.1371/journal.pone.0261182
- Timpabi, A. P., Adams, C. A., & Osei, K. K. (2023). The role of infrastructure and route type choices for walking and cycling in some cities in Ghana. Urban, Planning and Transport Research, 11(1). https://doi.org/10.1080/21650020.2023.2222838
- Train, K. (2003). Discrete choice methods with simulation. Cambridge Univeristy Press. https://www.researchgate.net/profile/Kenneth_Train/publication/5206179_Discrete_Choice_Method_With_Simulation/links/560f62e408ae48337517b980/Discrete-Choice-Method-With-Simulation.pdf
- Ulfarsson, G. F., & Mannering, F. L. (2004). Differences in male and female injury severities in sport-utility vehicle, minivan, pickup and passenger car accidents. Accident Analysis & Prevention, 36(2), 135–147. https://doi.org/10.1016/S0001-4575(02)00135-5
- United Nations. (2018, May, 16). 68% of the world population projected to live in urban areas by 2050, says UN. United Nations Department of Economic and Social Affairs.