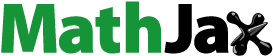
ABSTRACT
Public bike sharing is an increasingly popular mode of transportation in India, and understanding the factors influencing the intention to use bike share is crucial. This study employs a comprehensive approach by integrating the theory of planned behaviour (TPB), theory of interpersonal behaviour (TIB), and the technology acceptance model (TAM). Additionally, variables such as policy support, habits, external factors, and perceived bicycling conditions are also considered. A sample of 450 respondents from Delhi, the capital city of India, was collected to assess their intention to use bike sharing. The study employed Structural Equation Modelling, to examine the relationships among variables. The findings demonstrate that the research model effectively explains residents’ intention to use bike share. Factors such as perceived ease of use, behavioural attitude, subjective norms, and habits have direct and positive effects on the intention to use bike share. Notably, perceived ease of use, behavioural attitude, and policy support have the most substantial impact on the intention to use bike share. Conversely, perceived bicycling conditions and external factors exert negative effects on bike share usage intentions. This study provides valuable insights into the acceptance of bike sharing and offers a strategic direction for promoting bike sharing in Indian cities.
1. Introduction
Bike share schemes have gained significant attention as a sustainable and affordable mode of transportation in urban areas worldwide. In recent years, India also witnessed the emergence and growth of bike share systems, aiming to address transportation challenges and promote eco-friendly mobility options. The introduction of bike share schemes in India can be traced back to the early 2010s when several cities, including Delhi, Mumbai, and Pune, initiated pilot projects to promote non-motorized transportation. These early efforts were primarily driven by government initiatives, aiming to alleviate traffic congestion, reduce pollution, and provide last-mile connectivity. Over time, bike share schemes in India have evolved with the involvement of various stakeholders, including municipal corporations, private operators, non-profitable organisations, and technology providers. Presently, bike share schemes have expanded to numerous cities across India, including major metropolises like Bengaluru, Chennai, and Hyderabad, as well as smaller cities and towns. As of now, more than 48 bike share schemes are operational with a fleet size of excess 21,000 bicycles across 31 Indian cities (Machavarapu & Ram, Citation2022a). These schemes offer a variety of models, such as docked and dockless systems, E bikes and conventional bikes allowing users to rent bicycles for short-duration trips at affordable rates. Additionally, digital platforms and mobile applications have been integrated into bike share operations, enabling seamless booking, payment, and tracking functionalities. Despite of rapid growth of bike share schemes, various challenges impeding the successful implementation and operation of bike share schemes in Indian cities. Studies conducted by (Dhingra & Kodukula, Citation2010; Patel & Patel, Citation2020) have identified the lack of adequate cycling infrastructure as a key barrier to successful bike share scheme operations and usage in Indian cities. Insufficient cycling lanes, poor road conditions, and a lack of parking facilities make cycling less convenient and safe for users. Furthermore, safety concerns, such as excessive traffic volumes, insufficient road design, and a lack of understanding about sharing the road with bicycles, contribute to a perceived risk of accidents and injuries, hindering the adoption of bike share schemes. The risk of theft and vandalism reduces users’ confidence in the system and discourages bike share usage. In addition, Cultural perceptions, and attitudes towards cycling, especially when viewed as a mode of transport for the lower socio-economic strata, create a stigma that deters bike share scheme Sharmeen et al. (Citation2021). The limited integration of bike share schemes with other modes of transport, such as public transit, poses a barrier due to the inconvenience of inadequate last-mile connectivity. Limited user awareness and education about bike share schemes and their benefits result in bike share schemes low utilization rates in India. Additionally, due to limited funding, operational costs, and income generation, bike sharing schemes suffer financial viability and sustainability issues. Furthermore (Patel & Patel, Citation2020; Machavarapu & Ram, Citation2022b), emphasized how lack of understanding on bike share user behaviour, intents, and preferences led to poor bike share usage and the termination of these schemes in some of the Indian cities. Overall, addressing these challenges can contribute to the success and long-term viability of bike share schemes in Indian cities.
However, the present study focuses primarily on addressing the most significant difficulty associated with bike share user behaviour and intentions. What aspects are important to urban residents, and what factors influence their behaviour to use bike share? The underlying phenomenon is worth investigating. When we have a greater grasp of the public’s purpose to utilise bike share, operators can improve the cycling experience in a more targeted manner, local governments may propose appropriate laws, and citizens can realise low-carbon green travel more effectively. Further, it allows operators to optimize system operations, improve user satisfaction, and tailor the system to meet user needs. It also promotes sustainable transportation by identifying factors that influence mode choice and usage patterns. Insights from user behaviour studies inform policy and planning decisions, ensuring effective integration of bike share schemes into the transportation network. Additionally, studying user behaviour helps identify barriers and develop targeted strategies to enhance adoption and usage.
From the earlier literature studies, several constructs are considered to understand individuals’ motivations and intentions to use bike share systems. Attitudes play a significant role, with positive evaluations of bike share associated with higher intentions Shaheen et al. (Citation2010); Fan, Wang & Yang (Citation2021). Subjective norms, representing social influence, also influence adoption intentions (Chataway et al., Citation2014; Fishman et al., Citation2012; Kaplan & Prato, Citation2014). Perceived behavioural control, reflecting individuals’ beliefs about their ability to overcome barriers, is associated with stronger intentions. Perceived usefulness, encompassing beliefs about the benefits of bike share, and perceived ease of use, reflecting perceptions of effort required, both contribute to higher intentions (Hazen et al., Citation2015; Shaheen et al., Citation2011). In addition, Fishman et al. (Citation2014) conducted a comprehensive literature review that examines various factors influencing bike share usage intentions, including perceived bicycling conditions, external factors, and policy support. Ma, Zhang & Wang (Citation2019); Dill, Mohr & Ma (Citation2014) explored the factors influencing bike share user destination choice, including external factors, and policy support, using regression models. Shaheen and Chan (Citation2016) examined the factors influencing bike share usage intention in North America based on operator and user perspectives, encompassing perceived bicycling conditions, and policy support. These constructs draw from established theories such as the Theory of Planned Behaviour (TPB), Technology Acceptance Model (TAM), Diffusion of Innovations (DI), and, Theory of Interpersonal Behaviour (TIB), providing a comprehensive framework to understand bike share adoption intentions. Previous studies on the intention to use bike sharing have primarily used single model analysis, such as eighter TPB, TAM, or TIB, and the interpretation rate of these models has been low, making it difficult to understand the residents’ desire to use bike share systems. Therefore, the current study utilising TPB, TAM, TIB, and other behaviour models will incorporate additional important factors such as policy support, perceived bicycling conditions, and external factors to investigate residents’ intention to use bike share schemes and test the applicability of the proposed theoretical model for studying the intention to use bike share.
The structure of this study is outlined as follows: Section 2 provides a comprehensive review of the relevant literature pertaining to travel behaviour theories and their application in bike share research, as well as the utilization of Structural Equation Modelling (SEM) models in the study of bike share user behaviour. Section 3 presents the research framework and the development of hypotheses based on the literature review. In Section 4, the research methodology employed in this study is described in detail. Section 5 provides a brief overview of the case study conducted. The questionnaire design and data collection procedures are discussed in Section 6. Section 7 focuses on the data analysis methods employed and presents the results of the study. Section 8 comprises a thorough discussion of the findings, providing explanations and interpretations of the results within the context of the research objectives. Finally, Section 9 summarizes the research findings and conclusion.
2. Literature review
The section on literature review is divided into three subsections. 1) A review of several travel behaviour theories and their key constructs for understanding the complexities of NMT users’ behaviour and the factors influencing their mode choice. 2) Studies focusing on the application of travel behaviour theories to bike sharing research. 3) Existing study on the use of SEM techniques in bike share research.
2.1. Review of travel behavioural theories explaining NMT user behaviour
In this section, we aim to provide a comprehensive overview of different travel behaviour theories and the constructs associated with them. By reviewing relevant literature, we delve into the complexities of non-motorized transportation (NMT) users’ behaviour. Understanding the theoretical foundations and constructs of these theories is essential for unravelling the intricacies of individuals’ travel choices and guiding the development of effective interventions to promote sustainable transportation practices.
The Theory of Planned Behaviour (TPB) stands as one of the most widely used theories in travel behaviour research. TPB posits that an individual’s behaviour is determined by their attitudes, subjective norms, and perceived behavioural control (Ajzen, Citation1991). TPB has been effectively applied to analyse various travel behaviours, including electric vehicle adoption, public transit use, and car-sharing behaviour (Chatterjee et al., Citation2013; Steg & Vlek, Citation2009).
Building upon TPB, the Theory of Interpersonal Behaviour (TIB) incorporates social norms and interpersonal communication to further understand travel behaviour (Fazio, Citation1990). TIB has been utilized to explore aspects such as public transit use, bicycle commuting behaviour, and carpooling behaviour (Habibian et al., Citation2017; Rasouli & Tsotsos, Citation2020).
Another influential theory in travel behaviour research is the Theory of Reasoned Action (TRA), which suggests that behaviour is determined by an individual’s attitudes and subjective norms (Fishbein & Ajzen, Citation1975). TRA has been employed to examine various travel behaviours, including public transit use and bicycle commuting behaviour (Bamberg et al., Citation2003; Fujii & Gärling, Citation2003).
The Social Cognitive Theory (SCT) is another prominent theory that has been applied to the study of travel behaviour. SCT proposes that behaviour is influenced by individual factors, environmental factors, and behavioural factors (Bandura & National Inst of Mental Health, Citation1986). SCT has been effectively used to examine travel behaviours such as active transportation and vehicle use (Gatersleben et al., Citation2014).
Furthermore, the Technology Acceptance Model (TAM) has been widely utilized to study technology adoption, including in the transportation sector (Davis, Citation1989). TAM suggests that the adoption of technology is influenced by perceived usefulness and ease of use. TAM has been applied to investigate the adoption of various transportation technologies, including electric vehicles and intelligent transportation systems (Geurs & Van Wee, Citation2004; Hu et al., Citation2015).
also provides a summary of these travel behaviour theories, including important constructs, focus, and applicability. In conclusion, this section has provided a comprehensive overview of various travel behaviour theories and their associated constructs. By reviewing the relevant literature, we have explored the complexities of non-motorized transportation (NMT) users’ behaviour and highlighted the significance of understanding the theoretical foundations and constructs of these theories.
Table 1. List of travel behaviour theories on NMT user behaviour.
2.2. Application of behavioural theories on bike sharing research
2.2.1. Theory of Planned Behaviour (TPB)
The Theory of Planned Behaviour (TPB) has been extensively used in bike share studies to understand the factors that influence bike share usage behaviour. TPB is a social cognitive theory that posits that people’s attitudes, subjective norms, and perceived behavioural control predict their intentions to engage in a particular behaviour, which in turn predicts their actual behaviour. This literature review summarizes the existing research on the use of TPB in bike share studies.
The TPB is a well-established theoretical framework that explains how individual attitudes, subjective norms, and perceived behavioural control influence behaviour. The TPB suggests that a positive attitude toward the behaviour, social pressure or subjective norms, and perceived behavioural control over the behaviour can lead to increased likelihood of performing the behaviour.
Several studies have applied the TPB to understand bike share adoption and usage. For instance, a study conducted in Beijing, China, found that positive attitudes towards bike sharing were associated with increased usage frequency (Sun et al., Citation2019). The study also found that subjective norms, including social influence and social pressure, positively predicted bike share adoption and use. Another study conducted in New York City found that attitudes towards bike share, subjective norms, and perceived behavioural control were significant predictors of intention to use the system among potential users (Reilly et al., Citation2021).
A recent study conducted in Singapore also applied TPB to understand the adoption and usage of bike share systems (Zhang et al., Citation2021). The study found that perceived behavioural control had a significant positive effect on bike share usage. The authors suggested that enhancing user experiences through improved bike availability, ease of use, and maintenance could enhance perceived behavioural control and encourage bike sharing behaviour.
In addition to TPB, other theoretical frameworks such as the Technology Acceptance Model (TAM) have been applied in bike share studies. TAM emphasizes the importance of perceived usefulness and perceived ease of use in shaping individual behaviour. A study conducted in Beijing found that perceived ease of use and perceived usefulness positively influenced bike share adoption and usage (Yang et al., Citation2018).
In summary, the TPB and TAM have been applied to understand the factors that influence bike share adoption and usage. These theoretical frameworks can provide insights into the attitudes, subjective norms, and perceived behavioural control and ease of use and usefulness that influence bike share behaviour. However, further research is needed to explore the moderating effects of contextual and demographic factors on the relationship between TPB constructs and bike share usage behaviour. Targeted interventions can be developed based on these insights to encourage bike sharing and promote the development of sustainable transportation systems.
2.2.2. Theory of Interpersonal Behaviour (TIB)
The Theory of Interpersonal Behaviour (TIB) is an extension of the Theory of Planned Behaviour (TPB) that emphasizes the role of social norms and interpersonal communication in shaping individual behaviour. In the context of bike share studies, TIB has been used to understand factors that influence individuals’ decisions to adopt and use bike share systems. Several studies have applied TIB to investigate bike share usage behaviour. This literature review provides an overview of the use of TIB in bike share studies.
One study that applied TIB to bike share behaviour found that social norms played a significant role in bike share adoption and use (Xu et al., Citation2018). The study surveyed bike share users in Guangzhou, China and found that social influence from friends and family positively influenced bike share adoption and use. Another study conducted in China found that interpersonal communication was a significant predictor of bike share use (Yang et al., Citation2018Citation). The study found that individuals who received positive feedback and encouragement from friends and family were more likely to use bike share systems.
TIB has also been used to understand bike share behaviour in the context of university campuses. A study conducted in the United States found that social norms and interpersonal communication were significant predictors of bike share use among college students (Zhang et al., Citation2021). The study found that students who perceived positive social norms regarding bike share use and had more frequent communication about bike share with friends were more likely to use the system.
Another study applied TIB to understand bike share behaviour among tourists in Taiwan (Li et al., Citation2023). The study found that perceived social norms and interpersonal communication were significant predictors of bike share use among tourists. The study suggests that bike share systems can benefit from promoting positive social norms around bike use and encouraging tourists to communicate with each other about bike share options.
Overall, TIB has been shown to be a useful framework for understanding the social and interpersonal factors that influence bike share behaviour. By accounting for social norms and interpersonal communication, TIB can help identify strategies for promoting bike share adoption and use.
2.2.3. Technology Acceptance Model (TAM)
The Technology Acceptance Model (TAM) has been widely used in the field of transportation research, specifically in bike share studies, to understand user acceptance and adoption of technology-based systems. Several studies have applied TAM to bike share systems to determine the factors that influence user acceptance and adoption of bike sharing technology. TAM is a useful tool for bike share system operators and researchers to identify factors that may impact user adoption and to tailor marketing strategies to enhance system uptake.
One study found that the perceived usefulness and ease of use of bike share technology significantly influenced user intention to use the system Liu and Yang (Citation2018). Another study found that perceived ease of use, perceived usefulness, and subjective norm significantly influenced user intention to use bike share systems Guo et al. (Citation2021). These findings highlight the importance of understanding user perceptions and attitudes towards bike share technology in order to promote its adoption.
Furthermore, some studies have extended TAM to include additional factors that may impact user adoption of bike share technology. For example, one study found that perceived risk significantly influenced user acceptance of bike sharing technology Wang et al. (Citation2015). Another study identified the role of social influence and trust in user acceptance of bike share technology Li et al. (Citation2013).
Overall, TAM has proven to be a useful framework for understanding user acceptance and adoption of bike sharing technology. By identifying factors that influence user behaviour, bike share system operators and researchers can develop strategies to increase adoption and usage of bike sharing systems, which can have positive impacts on transportation sustainability and public health.
2.3. Structural Equation Modelling (SEM)
2.3.1. Application of SEM in transportation research
Structural equation modeling (SEM) is a statistical technique that can be used to test complex models of relationships between variables. SEM has been applied in a variety of research areas such as Psychology, Education, Marketing, Public health, Economics etc each with its own unique benefits. SEM has many benefits over traditional statistical methods, including the ability to model latent variables, Examining mediating and moderating effects, and account for measurement error. As a result of multiple benefits over traditional Structural equation modeling (SEM) has also become an increasingly popular statistical technique in transportation research over the past few decades. The current study presents a brief literature review on the use of SEM in various streams of transportation research.
Identifying the factors that influence travel behaviour: SEM can be used to identify the factors that influence travel behaviour, such as mode choice and travel demand. For example, a study by Litman (Citation2019) used SEM to examine the factors that influence mode choice in urban areas. The study found that travel time, cost, and convenience were the most important factors in mode choice, and that demographic characteristics, land use patterns, and attitudes towards transportation also played a significant role.
Understanding the effects of transportation policies and interventions: SEM can be used to model the effects of transportation policies and interventions on travel behaviour. For example, a study by Hu et al. (Citation2015) used SEM to examine the effects of transportation policies on travel demand in China. The study found that policies aimed at reducing car use, such as parking pricing and congestion charges, had a significant impact on travel demand.
Examining the relationships between transportation and the built environment: SEM can be used to examine the relationships between transportation and the built environment, such as land use patterns and urban design. For example, a study by Johansson et al. (Citation2022) used SEM to explore the factors that influence car use in Sweden. The study found that attitudes towards car use, perceived behavioural control, and social norms were the most important factors in predicting car use, and that demographic characteristics and the built environment also had significant effects.
Modelling the impacts of transportation on the environment and public health: SEM can be used to model the impacts of transportation on the environment and public health. For example, a study by Yang et al. (Citation2018) used SEM to examine the relationship between transportation emissions and air quality in urban areas. The study found that reducing transportation emissions could have significant benefits for air quality and public health.
Overall, SEM is a powerful statistical tool for understanding the complex relationships between different factors that influence travel behaviour, and for modelling the effects of transportation policies and interventions on travel behaviour, the built environment, and public health. By using SEM, transportation researchers and policymakers can gain valuable insights into how to design more sustainable and efficient transportation systems.
2.3.2. Application of SEM on bike share studies
Several studies have employed Structural Equation Modelling (SEM) to investigate the determinants of bike share usage and satisfaction. Yuhan et al. (Citation2021) used SEM to examine the relationship between perceived service quality, perceived value, and customer satisfaction in a bike share program in Shanghai, finding that both service quality and value positively influenced satisfaction, with value mediating the relationship. Pan et al. (Citation2022) used SEM to explore the factors affecting the intention to use bike share systems in China, revealing that perceived usefulness, ease of use, social influence, and perceived risk all significantly influenced intention, with usefulness being the most important predictor. Shaheen et al. (Citation2014) utilized SEM to study the impact of bike share systems on travel behaviour in the United States, revealing that bike share use was associated with increased walking, decreased car use, and mediated by perceived convenience, environmental attitudes, and subjective norms. Pan et al. (Citation2022) applied SEM to examine pricing strategies in Beijing, finding that a flat-rate pricing strategy led to higher ridership compared to distance-based pricing, mediated by perceived price fairness. These studies highlight the value of SEM in understanding the multifaceted relationships influencing bike share usage and satisfaction, while also suggesting avenues for future research, such as exploring additional variables like infrastructure, weather, and cultural factors using SEM.
Despite the extensive literature, several major research gaps exist in the application of structural equation modelling (SEM) to the study of bike sharing user behaviour. First, it remains difficult to prove causation and the direction of influence between distinct components. For accuracy, it is needed to develop more sophisticated SEM models adapted to the specific context of bike share behaviour. In addition, the integration of external elements such as urban infrastructure and policy influences, as well as the research of unique behaviour patterns across different user groups, are frequently inadequate. Understanding how user behaviour evolves over time and incorporating temporal dynamics, enhancing the reliability and validity of measurement tools, and taking contextual factors such as weather conditions and cultural influences into account are topics that require additional focus. In addition, examining the connections between bike sharing and other means of transportation and incorporating user comments and qualitative data can provide a more complete picture. In order to determine the generalizability of SEM findings in diverse urban environments, cross-cultural research is required. Addressing these deficiencies will result in a more thorough and nuanced understanding of bike share user behaviour, hence influencing the improvement and promotion of bike sharing systems in a variety of urban environments.
3. Research framework and development of hypotheses
The Conceptual Model is represented graphically in . This conceptual framework has been influenced by numerous theories on behaviour in general and travel behaviour on NMT mode choice as discussed in the previous literature section. These theories include Ajzen and Fishbein’s Theory of Reasoned Action (TRA) (Fishbein & Ajzen, Citation1975) and the Theory of Planned Behaviour (TPB) (Ajzen, Citation1991; Ajzen & Fishbein, Citation1970). The model was then enhanced by incorporating additional elements influencing travel behaviour, such as habitual reactions, situational restrictions, and circumstances derived from the Theory of Interpersonal Behaviour by Roger Fisher (Triandis, Citation1977). During the process of developing the conceptual model, other behavioural theories that explain individual decision making, such as Schwartz’s (Citation1977) Norm-Activation Theory (NAT) and Stern’s (Citation2000) Value-Belief-Norm Theory (VBN), were also investigated. These theories provide an explanation for why people make the decisions that they do. For the most part, the conceptual model is built on the constructs of TPB, TIB and TAM models with policy support, external factors added to examine people’s intentions towards the usage of bike share. In TIB, intention is motivated not just by personal cognition (subjective norms and attitude), but also by emotions (affective constructions), social norms, and self-identity (social constructs), habitual responses, situational constraints and conditions. Triandis (Citation1977) contends that automatic performance of a behaviour reduces the level of conscious control over such behaviour. In the TIB, the presence of objective external restrictions on the performance of a behaviour has a direct effect, whereas the TPB implies a subjective representation of those elements influencing intention. In the TAM model, perceived usefulness and perceived ease of use are the two most important and basic constructs, and they collectively affect the user’s attitude. Based on the relationship between variables and structures of the three theoretical models, we propose the hypothesis relationship as shown in . The variables in the three theoretical methods are separated by dashed lines. The conceptual model includes a total of ten boxes, each of which represents one of the ten different features of the variables. User attributes of rectangular Box shows the individual and household characteristics of users of bike share programmes (which include socio economic, demographic characteristics, trip characteristics). As was said before, the socio demographic parameters that influence the mode choice behaviour are considered in the user characteristics box. These categories include age, income, gender, education level, and ownership of a car, among others. In addition to the observed variables, such as User characteristics, nine latent variables shown in ellipse boxes, such as the perceived quality of the bike share service, behavioural attitude, habits, policies, and subjective norms were also taken into consideration to gain a better understanding of the bike share usage intentions and behaviour.
Latent features, on the other hand, are unable to be quantified directly, in contrast to the data on users’ individual, household, and trip characteristics. In order to quantify these latent features, about 39 latent variable indicators were selected and constructed to collect information that would examine bike share users’ behavioural intentions. Further, the list of the latent variables and their constructs were discussed in Appendix . The selection and design of these indicators was based on the objective of this study. In the following section, we will go through the specifics of the indicators of these latent variables, as well as their interactions with one another. Further, in the conceptual model the one-way arrows indicate the direction of dependence between these latent variables. Individual/household, perceived bike share quality, habits, behavioural attitude, subjective norm, perceived biking conditions, external factors, and policy support are all predicted to have a direct/indirect effect on behavioural intents and bike sharing behaviour.
The hypotheses for the relationships within the model are summarized in the . By incorporating these theoretical frameworks and considering a range of variables, this conceptual model provides a comprehensive understanding of the factors influencing bike share intentions and behaviour.
Table 2. List of the hypotheses.
4. Methodology
To explore the behaviour of bike sharing users, Structural equation modelling (SEM) was used in the study. In recent years, Structural equation modelling (SEM) has become a popular statistical technique in travel behaviour studies due to its ability to analyse complex relationships among latent constructs and observed variables. Over the years, the application of SEM in travel behaviour research has evolved, and some notable trends can be observed. In the early years, SEM was mainly used to test the validity of hypothesized relationships among travel-related constructs, such as attitudes, perceptions, and intentions. Researchers primarily used confirmatory factor analysis (CFA) to assess the dimensionality and construct validity of the underlying constructs. Later, SEM was applied to investigate the relationships among these constructs, such as the influence of attitudes on travel behaviour. With the advent of the theory of planned behaviour and the reasoned action approach, SEM was used to test complex theoretical models that explain travel behaviour. In recent years, SEM has been applied to examine the causal relationships among multiple constructs simultaneously. In addition, SEM has been used to test dynamic models of travel behaviour that account for the temporal dynamics of travel decision-making. As the current study also consists of multiple latent variables affecting bike share intentions to use and their observed indicators, the (SEM) technique was used to identify the complex relationships for understanding the underlying mechanisms of behaviour. The research followed a step-by-step methodology to develop SEM model. The process consists of mainly five phases as shown in .
The study’s first phase included a pilot study to investigate the numerous latent factors influencing existing bike share user behaviour in Delhi and to analyse the impact of these latent characteristics on bike share users’ attitudes and intentions to utilise the service in the future. The questionnaire was carefully designed, with questions drawn from past studies and other bike share travel behaviour survey instruments. The pilot survey was undertaken to determine the amount of time required to complete the questionnaire and the level of difficulty of the questions asked of respondents. Following the pilot study, the questionnaire was revised by eliminating and changing several elements based on input from respondent bike sharing users.
Second phase, the bike share user travel behaviour survey was conducted using modified questionnaire. Volunteer & opportunity sampling method is used in main survey and samples are collected from different bike Sharing age groups. Voluntary sampling occurs when researchers seek volunteers to participate in studies. Volunteers can be solicited in person, over the internet, via public postings, and a variety of other methods. Opportunity sampling is a sampling technique used to select participants from a target group to take part in a research study. It consists of the researcher selecting anyone who is available and willing to take part in the study. As the respondents were not willing to give face-to-face interview immediately after Covid − 19 Pandemic, the survey was performed through both online and offline modes. A total of 450 complete questionnaire samples were collected, out of which (85%) samples were collected through online and remaining (15%) samples were collected through offline mode.
In the third phase of the study, an Exploratory Factor Analysis (EFA) is performed to identify the variables that influence bike share user intentions. To determine the initial factor structure, we utilised a factor extraction method such as principal component analysis. To improve interpretability, we used a factor rotation technique (e.g. Varimax rotation) to generate a more understandable and concise factor solution. We evaluated the factor loadings and the theoretical consistency of each item’s contribution to each component. This procedure enables us to discover and categorise the underlying characteristics that influence the behaviour of bike share users. Further in phase 4, We provided a hypothesised factor structure for the Confirmatory Factor Analysis based on the EFA results (CFA). This required defining the connections between the observable variables and their related latent factors. CFA enabled us to validate the underlying components that explain bike share user behaviour by confirming the factor structure discovered by EFA. To refine the model, we analysed the factor loadings, standard residuals, and modification indices. A Cronbach’s alpha value of 0.70 or greater indicates adequate dependability (Gliem & Gliem, Citation2003). It evaluates the precision with which a set of questions measures a unidimensional factor. Using the Kaiser – Meyer–Olkin (KMO) test, sufficiency was established. KMO values between 0.80 and 1 indicate adequate sample (Gliem & Gliem, Citation2003).
In the fifth phase, SEM or path analysis was utilised to assess the hypothesised correlations between constructs of the proposed model developed using NMT travel behaviour theories such as TPB, TIB, and TAM. To estimate a system of structural equations, the ML method is utilised. Using SEM, the structural coefficients and fit indices are analysed. Several indices are used to evaluate the quality of fit of structural models, with at least one index from each model fit category required (Hair et al., Citation2013; Holmes-Smith et al., Citation2006; Hooper et al., Citation2008). Iterating the model until appropriate fit indices are reached yields the final model.
5. Study area
The current study focuses on Delhi as a case city to investigate the user behaviour of bike share schemes. Delhi, the capital and one of India’s largest metropolitan cities, spans an area of 1484 sq. km and has a population of 20.5 million. The city boasts a well-established public transport network, with 31% of the population relying on it. However, only 4% of the population uses bicycles despite non-motorized transportation (NMT) accounting for 41% of the modal share. Historically, bicycles were the primary mode of transport in Delhi, with one-third of trips made by bicycle. However, factors such as urban sprawl, increased per capita income, and rapid motorization have led to a significant decline in the bicycle modal share, plummeting from 36% in 1961 to 4% in 2011 (Tiwari & Jain, Citation2008). To address this decline and promote sustainable transportation, plans were implemented to enhance NMT infrastructure, integrate the transport network, and establish a cycle network. As part of the efforts to revive bicycling culture and promote sustainable transport, bike share schemes were introduced in Delhi on a pilot basis in 2008 and later expanded throughout the city. Presently, Delhi boasts one of India’s premier bike share networks, with over 180 bike stations and approximately 3200 bikes. Alongside Pune, Delhi was among the first cities in India to launch bike share schemes in 2008. Despite these initiatives, bike share schemes in Delhi have not achieved the same level of success and popularity as similar schemes in other developed and developing countries. Machavarapu and Ram (2022a) highlighted the low performance metrics of bike share schemes in the city, particularly in terms of ridership and fleet utilization. Furthermore, Machavarapu and Ram (2022b) identified various factors influencing the usage and performance of bike share schemes in Delhi. However, their study solely relied on app-based bike share data analysis, underscoring the need for a bike share user behaviour study to comprehensively examine the factors impacting users’ behavioural intentions to use bike share in the city. To address these gaps, the present study focuses on Delhi as a case city and conducts a bike share user behaviour study to identify the factors influencing users’ bike share usage intentions.
6. Questionnaire design and data collection
The questionnaire used in this study was developed by drawing upon relevant literature, as discussed in the literature section. The survey was conducted using a Volunteer & Opportunity sampling method. To ensure that the respondents have experience using bike share services, they were initially asked if they have used bike share before proceeding to fill out the questionnaire. If the respondents answered negatively, the questionnaire was concluded.
Previous research has demonstrated the strong applicability of the Theory of Planned Behaviour (TPB), Theory of Interpersonal Behaviour (TIB), and Technology Acceptance Model (TAM) in various intention research domains. Building upon these models, this study incorporates additional variables such as policy support, habits, external factors, and perceived bicycling conditions, with the aim of enhancing the predictive capability of the model. The questionnaire consisted of two sections: demographic information and a combined TPB, TIB, and TAM scale. The demographic information section included variables such as age, gender, occupation, education, monthly income, and vehicle ownership. The combined TPB, TIB, and TAM scale encompassed eight variables: Perceived Ease of Bike Share Service (PE), Policy Support (PS), Perceived Bicycling Conditions (PBC), Behavioural Attitude (BA), Subjective Norms (SN), Perceived Behavioural Control (BC), External Factors (EF), and Intention to Use (IU). Through consultations with experts and preliminary testing, the initial questionnaire was refined to its final version. The specific items are shown in .
The sample size was initially calculated using the formula given (Johnson & Wichern, Citation1992). According to statistics provided by bike share operators in Delhi, a total of 400,000 residents enrolled through bike share apps, which is used to calculate sample size. Furthermore, a confidence level of 95%, a margin of error of 5%, and a population proportion of 50% were considered.
Table
In accordance with the prior calculations, the minimum sample estimate is 384. However, the study collected a total of 450 samples, of which 34 were discarded due to insufficient response. The data of the remaining 416 bike share respondents were subsequently incorporated into the analysis.
7. Data analysis and results
7.1. Demographic profile
The demographic information of the sample population is presented in . Out of the 416 respondents, 66.8% were male, while 33.2% were female. The age distribution of the participants indicates that 66.9% of the sample falls within the 18–40 years age range, which is a significant portion for the study. In terms of qualification, 19.4% of the participants had an Intermediate/Level education, 46% were graduates, and 30.6% held a master’s degree. Looking at occupation, 36.9% of the sample consisted of students, while 27.5% were employed. Regarding monthly household income, the majority of respondents fell within the ranges of Rs 50,000–Rs 1,00,000 (33.5%) and Rs 50,000 - Rs 1,00,000 (35.4%). When considering household vehicle ownership, 53.7% of the respondents had at least one bicycle, while 78.1% had at least one two-wheeler (motor). Finally, in terms of car ownership, 75% of the respondents did not have a car in their household. These demographic details provide insights into the characteristics of the sample population for the study.
Table 3. Demographics and relevant frequency statistics of bike share user respondents.
7.2. Descriptive statistics
7.2.1. Behavioural Attitude (BA)
Understanding bike share behaviour is dependent on the concept of behavioural attitude, which encompasses individuals’ attitudes, opinions, judgments, and emotions towards bike sharing. This construct reflects their underlying psychological state, which is not directly observable. Extensive research, supported by studies on the Technology Acceptance Model (TAM) and the Theory of Planned Behaviour (TPB), has consistently shown the significant impact of behavioural attitude on both the intention to engage in bike share behaviour and the actual adoption and usage of bike share systems. Zhang et al. (Citation2021) found that a positive behavioural attitude towards bike sharing significantly predicted users’ intention to adopt bike share systems, while Chen et al. (Citation2019) demonstrated that favorable behavioural attitudes positively influenced actual usage of bike share services. These findings underscore the importance of assessing and understanding individuals’ behavioural attitudes as a predictor of their engagement with bike share systems.
To encourage positive attitudes and promote bike share usage, incorporating behavioural attitude as a focal point in bike share interventions and strategies is crucial. Bike share operators and policymakers can enhance the adoption and usage of bike sharing as a sustainable mode of transportation by addressing individuals’ concerns, improving their perceptions, and fostering positive attitudes towards bike share systems through effective communication and marketing campaigns. In this study, the researchers specifically measured the behavioural attitude of bike share users using six observational items or indicators. Participants responded on a 5-point Likert scale ranging from strongly disagree to strongly agree. Descriptive statistics for the Behavioural Attitude (BA) construct are presented in , where item 7, ‘Bike share is comfortable to use in the city’, received the lowest mean score (M = 2.72, SD = 1.142), indicating relatively less positive attitudes towards bike share comfort. Conversely, item 1, ‘Bike share is economical to use’, obtained the highest mean score (M = 3.79, SD = 1.257), suggesting that participants perceived bike share as a cost-effective transportation option.
Table 4. Mean scores, standard Deviation values for behavioural attitude (BA) scale.
7.2.2. Perceived ease of use bike share service (PE)
Perceived Ease of Use is a crucial factor that significantly impacts users’ attitudes and behavioural intentions towards bike share systems. It encompasses individuals’ perception of the level of ease or difficulty associated with using the bike share system as a mode of transportation. This concept aligns with the Technology Acceptance Model (TAM), which emphasizes users’ perceptions of ease when adopting and utilizing a specific system (Davis, Citation1989).
When users evaluate bike share systems, they prioritize the perceived ease of use as a key criterion in their decision-making process. They actively seek bike share technology that requires minimal effort and provides convenience in terms of accessing and using the bikes. The perceived ease of use plays a critical role in users’ assessment of bike share systems as it influences their perceptions of utility and efficiency compared to alternative transportation options. Numerous studies have consistently shown the positive impact of perceived ease of use on users’ attitudes and behavioural intentions towards bike share systems. For instance, Fishbein and Ajzen (Citation2010) demonstrated that perceived ease of use significantly influenced individuals’ attitudes and intentions to use bike share systems, while Ma et al. (Citation2018) revealed a positive relationship between perceived ease of use and users’ adoption and continued use of bike share services. These studies underscore the importance of designing bike share systems that are user-friendly, intuitive, and accessible. By enhancing the perceived ease of use through features such as user-friendly mobile applications, convenient bike docking stations, and simplified registration processes, bike share operators can positively influence users’ attitudes and intentions to embrace bike share systems as a sustainable mode of transportation.
Descriptive statistics for the Perceived Ease of Use Bike Share Service (PE) construct are presented in . Notably, item 4, “I am happy with the ‘Bike sharing network coverage (availability of bike stations at desired places) in the city”, received the lowest mean score (M = 2.54, SD = 1.356), indicating users’ relative dissatisfaction with the bike share network coverage in the city. On the other hand, item 5, ‘I am happy with the “Convenience of using bike sharing app”’, obtained the highest mean score (M = 3.79, SD = 1.257), suggesting that users perceive the bike share app as highly user-friendly and easy to use.
Table 5. Mean scores, standard Deviation values for perceived ease of use (PE) scale.
7.2.3. Habits (H)
Habits play a significant role in activating travel behaviour, including bike share usage, despite their non-psycho-social nature. From a cognitive perspective, once a behaviour becomes habitual, it is no longer perceived as a conscious choice but rather as a routine action guided by an established script based on past experiences. This cognitive process aims to reduce cognitive effort by relying on automated responses. The strength of a habit can be measured by the speed of a person’s reaction to a specific situation. Research has demonstrated that as behaviours become more automatic and habitual, individuals rely less on actively seeking information to make conscious decisions.
Studies in the field of travel behaviour have highlighted the influence of habits on bike share usage. For example, a study conducted by Böcker et al. (Citation2020); Gutiérrez etal. (Citation2020) examined the role of habits in bike share adoption and found that habitual behaviours played a significant role in individuals’ decision to use bike sharing systems. Similarly, a study by Fishbein and Ajzen (Citation2010) emphasized the impact of habits on travel behaviour, suggesting that habitual actions are less dependent on conscious decision-making and more influenced by automatic responses.
Understanding the role of habits in bike share behaviour is essential for effective intervention strategies and policy development. By acknowledging the power of habits in shaping behaviour, bike share operators and policymakers can implement interventions that target habit formation and facilitate the integration of bike sharing into individuals’ daily routines. Encouraging the development of positive habits through repeated exposure and reinforcement can contribute to sustained bike share usage and the promotion of sustainable transportation options. Current study also considered Habits as one of the important constructs to understand the users bike share intentions and the descriptive statistics of Habits scale items are shown in .
Table 6. Mean scores, standard Deviation values for habits scale.
7.2.4. Subjective Norms (SN)
Norms can have a significant impact on individual behaviour, whether they are individual norms or social norms. Social norms are learned and internalized through the process of social identity formation, becoming personal norms. When individuals perceive themselves as part of a group, they are more likely to conform to the norms of that group. Other customs and norms may remain unfamiliar, but they still exert influence on our behaviour. Subjective norms, a subset of social influence, refer to the social pressure individuals experience when deciding whether to engage in a particular behaviour and can be used to predict behavioural intentions. They represent the influence of other individuals or organizations on an individual’s decision-making process.
In the current study, the researchers used five observational items/indicators to measure the subjective norm (SN) of bike share users. A 5-point Likert scale ranging from strongly disagree to strongly agree was used to capture participants’ responses. Additionally, descriptive statistics for the subjective norm (SN) construct are presented in . The mean scores for the items ranged from 3.12 to 3.72. Item 1, ‘My family members can influence my choice to use bikes share’, received the lowest mean score (M = 3.12, SD = 1.260), suggesting that participants may be less inclined to conform to the behaviour of their social circle. On the other hand, item 5, ‘Awareness information on bike share through social media can influence me to use bike share’, obtained the highest mean score (M = 3.72, SD = 1.213), indicating that participants perceive social media as a potential influencer in their decision to use bike share systems.
Table 7. Mean scores, standard Deviation values for subjective norms (SN) scale.
Table 8. Mean scores, standard Deviation values for policy support (PC) scale.
7.2.5. Policy Support/Change (PC)
Governments have a range of policy instruments at their disposal to influence behaviour and promote behaviour change. These instruments include monetary incentives, prohibitions and regulations, public awareness campaigns, and more recently, nudges. Policy instruments provide tangible ways for governments to encourage behaviour change. However, it is crucial for governments to avoid being manipulative and instead ensure that their policy instruments have widespread support. Building a conceptual framework for effective and supported behaviour change can help governments ask important questions when studying behaviour change. Collaborative efforts between practitioners and scholars, along with the development of new theories, can contribute to a better understanding of how to achieve effective and supported behaviour change.
In the context of bike share user behaviour, the study also assessed the impact of policy instruments by evaluating five observational items related to policy support. Participants’ support for policy changes was measured using a 5-point Likert scale, ranging from strongly disagree to strongly agree. Descriptive data for the Behavioural Attitude (BA) construct are presented in . The mean scores for the items ranged from 2.81 to 4.01. Item 2, ‘Government restrictions on private vehicles would encourage me to shift to bike share’, received the lowest mean score (M = 2.81, SD = 1.297), suggesting that participants may not perceive government restrictions on private vehicles as a strong incentive to use bike share. On the other hand, item 5, ‘Government incentives for bike share trips would encourage me to use bike share more’, obtained the highest mean score (M = 4.01, SD = 1.145), indicating that participants perceive government incentives as an effective way to encourage greater bike share usage.
7.2.6. Perceived Bicycling Conditions (PBC)
Perceived bicycling conditions have a significant influence on individuals’ intentions to use bike share systems. When people perceive the conditions to be safe, convenient, comfortable, and accessible, they are more likely to engage in bike share usage. Several studies have investigated the impact of perceived bicycling conditions on bike share behaviour, shedding light on the factors that shape individuals’ decisions. For example, research conducted by Fishman et al. (Citation2013) in Melbourne and Brisbane emphasized the importance of safety, convenience, and comfort as determinants of bike share usage. Jahanshahi et al. (Citation2020) examined factors influencing the usage of Capital Bikeshare in Arlington, Virginia, including the influence of perceived conditions. Similarly, Chen and Hsu (Citation2020); Cheng et al. (Citation2020) focused on motivations for using public bicycle-sharing schemes in Taipei and highlighted the role of perceived conditions. Li et al. (Citation2023) analyzed public bicycle usage in Xiamen, China, and identified perceived conditions as a significant determinant. These studies collectively demonstrate that factors such as safety, convenience, comfort, and accessibility, which are encompassed in perceived bicycling conditions, play a crucial role in shaping bike share usage behaviour.
To assess how bike share users perceive bicycling conditions and its impact on their usage intentions, the study employed four observational items/constructs related to the safety, convenience, and comfort of bicycling. Participants responded to a 5-point Likert scale, ranging from strongly disagree to strongly agree. Descriptive statistics for the Behavioural Attitude (BA) construct are presented in . The study measured participants’ perceptions of these conditions to understand their influence on bike share usage intentions, providing valuable insights into the factors that can encourage or discourage individuals from using bike share systems.
Table 9. Mean scores, standard Deviation values for Perceived Bicycling Conditions (PBC) scale.
7.3. Exploratory Factor Analysis (EFA)
In this study, we followed a systematic procedure to conduct Exploratory Factor Analysis (EFA) for determining the factors influencing bike share intentions. Firstly, we collected the necessary data on bike share user behaviour, including survey responses capturing various aspects of bike share intentions. Subsequently, the collected data was appropriately formatted and prepared for analysis. As a part of EFA, we utilized principal component analysis (PCA) as a factor extraction technique to identify the initial factor structure. This technique helped us uncover the underlying factors that explain the shared variance among the observed variables. PCA allowed us to determine the linear combinations of variables that accounted for the most significant amount of variation in the data.
Following the extraction of initial factors, we employed Varimax rotation, a factor rotation method, to achieve a more meaningful and interpretable factor solution. This rotation process aimed to simplify the factor structure by maximizing the variance of loadings within factors and minimizing the cross-loadings across factors, thus enhancing the interpretability of the results. Subsequently, we examined the factor loadings, which represented the correlations between the observed variables and the underlying factors. Higher loadings indicated stronger relationships between variables and factors.
Based on the pattern of loadings, we interpreted the factors and assigned meaningful labels to each factor, reflecting the underlying constructs that drive bike share intentions. To ensure theoretical consistency, we assessed the alignment of items within each factor with the conceptual framework and logical sense. Consequently, we eliminated items such as PE 6, PE 7, PE 8, BA 6, and BA 7 that did not align with the intended factor.
The outcomes of the EFA provided us with a hypothesized factor structure, which illustrated the relationships between the observed variables and their respective latent factors as shown in . This hypothesized structure serves as the foundation for further analysis and validation in Confirmatory Factor Analysis (CFA).
7.4. Confirmatory Factor Analysis (CFA)
Based on the outcomes of the Exploratory Factor Analysis (EFA), a hypothesized factor structure was constructed to illustrate the relationships between the observed variables and their corresponding latent factors. Subsequently, a Structural Equation Model (SEM) was developed, specifying the paths and associations between the indicators and factors, drawing upon theoretical foundations and prior research. To estimate the parameters of the Confirmatory Factor Analysis (CFA) model, we employed AMOS 23.0 statistical software. This estimation process involved determining factor loadings, which depict the relationships between the observed variables and latent factors, as well as error variances and covariances. The primary objective was to evaluate the adequacy of the specified model in representing the observed data. A visual representation of the CFA model’s path diagram is provided in , while the corresponding validity and reliability values, along with the results of the CFA, are presented in . Further, a reliability analysis was conducted to assess the reliability of the items used in the latent factor constructs. Cronbach’s alpha, a widely recognized reliability index, was utilized for this purpose. The resulting Cronbach’s alpha values for each variable exceeded 0.7, indicating high reliability of the questionnaire. Additionally, the measurement items displayed standard factor loadings exceeding 0.5, which indicates good internal consistency of the scale. Convergence validity and discriminant validity were examined to evaluate the questionnaire’s validity. As presented in , all variables exhibited AVE (average variance extracted) values greater than 0.50, along with CR (compound reliability) values above 0.7. These findings indicate the questionnaire’s high reliability and convergent validity. Furthermore, the goodness of fit of the CFA model was evaluated using various fit indices, including chi-square, Comparative Fit Index (CFI), goodness-of-fit index (GFI), and Root Mean Square Error of Approximation (RMSEA). The results of CFA indicated a good fit of the model as shown in .
Table 10. Results of confirmatory factor analysis.
Table 11. Goodness of model fit statistics of Structural model.
7.5. Path analysis and hypothesis testing (structural model)
Based on the results of CFA and the theoretical framework, we specified the paths and relationships between the latent variables and observed variables in the structural model as shown in . These paths represent the hypothesized causal relationships among the constructs. Next, the model is estimated using statistical software, AMOS 23.0, to obtain parameter estimates, standard errors, and test statistics.
7.5.1. Model goodness-of-fit testing
Six specific indexes were used to assess the model’s goodness of fit: chi-square freedom degree ratio (c2/df), root-mean-square-error of approximation (RMSEA), standardised root-mean-square residual (SRMR), goodness-of-fit index (GFI), adjusted goodness of fit index (AGFI), comparative fit index (CFI), and normed fit index (NFI). AMOS 23.0 is used to compute the indices. AMOS is a structural equation model processing software created by IBM in the United States (SEM). As indicated in , the model’s fitting indexes are all in the ideal range, indicating that the model’s fitting degree is high and the model adequately represents the relationships among the variables.
Further in the hypothesis, we examined the statistical significance of specific paths in the model to determine the support for our hypotheses. The significance of each path was assessed using p-values, with a predetermined significance level of p < 0.05. Our analysis revealed that all hypothesis-specific paths, except for H3, H5, H10, and H11, were found to be statistically significant, providing evidence in support of the hypothesized relationships as shown in .
Table 12. Path coefficients of the structural model.
In addition, we conducted a comprehensive study of the model’s direct and indirect effects by analysing the relevance of the pathways connecting the variables. The standard direct, indirect, and total impacts of the various variables on behavioural intention are presented in . Except for Behavioural Attitude, all the other variables have direct and indirect influence on bike sharing intentions. To examine the relative influence of these variables on the intention to use the bike sharing service, we ranked their contributions as follows:
Perceived Ease of Use (0.529): This factor has the highest importance, indicating that individuals’ perceptions of how easy it is to use the bike share system strongly influence their intention to utilize it.
Perceived Bicycling Conditions (−0.453): The quality of the bicycling conditions negatively affects the intention to use the bike share service. This suggests that if individuals perceive the bicycling conditions to be unfavorable, they are less likely to have an intention to use the service.
Policy Support/Change (0.340): This variable positively contributes to the intention to use the bike share system. It implies that policy support and favorable changes in regulations or infrastructure can increase individuals’ willingness to use bike share services.
Behavioural Attitude (0.421): Although this variable does not have significant indirect effects, it still holds considerable importance. Individuals’ personal attitudes toward using bike share services contribute positively to their intention to utilize them.
External Factors (−0.324): External factors, such as external barriers or constraints, negatively impact individuals’ intention to use the bike share service. This suggests that overcoming external obstacles can help promote the adoption of bike sharing.
Subjective Norms (0.188): Social norms or perceived social pressure exert a positive influence on individuals’ intention to use bike share systems. This implies that if individuals believe that their peers or society in general favours using bike share services, they are more likely to adopt them. However, this result is not significant as (p > 0.05)
Habits (0.155): The habitual behaviour of individuals also plays a role in their intention to use bike share services. If individuals have established habits related to biking or using alternative modes of transportation, it positively affects their intention to utilize bike sharing. However, this result is not significant as (p > 0.05).
Table 13. Effects of different variables on the intention to use bike share.
These results provide valuable insights into the significance and contribution of each variable in explaining the relationships and influencing behavioural intention in the context of bike sharing.
8. Discussions on model results and recommendations
8.1. Key findings
On the basis of bike share user survey, we examined various factors that may influence the adoption of bicycle sharing. Here, we summarize and discuss the quantitative and qualitative findings of the study as well as the relevant strategies can be implemented to promote the adoption of bicycle sharing.
8.2. Behavioural attitude
The study attempted to investigate the impact of six latent components on behaviour attitudes as part of the theoretical conceptual model. Direct paths were built as part of the model between the six latent variables and behavioural attitude, assuming that the six latent factors influence behavioural attitude. Following an assessment of the model, the results suggest that just four pathways are supported. Constructs like perceived quality of bike share service and external influences have a strong influence on behavioural attitude. Subjective norms and perceived bicycling conditions were also found to have a moderate effect on behavioural attitude. Additionally, among these, perceived bike sharing service quality and subjective norm have a favourable influence on behaviour, whereas external factors and perceived cycling conditions have a negative effect. Further, habits and policy support also have a negligible impact on behavioural attitude; nevertheless, these relationships are invalid due to the importance of these components (P > 0.05).
8.3. Behavioural intention on bike share use
8.3.1. Perceived ease of bike share service (PE)
The findings of the study reveal a strong positive relationship between residents’ perceived ease of use and their behavioural intention to use bike share services. This implies that when residents perceive bike share services as easy to use, it significantly influences their intention to use them in the future. These results align with prior research in the field, supporting the importance of perceived ease of use in the adoption and usage of bike share systems. Previous research by Fishman et al. (Citation2013) on bike share membership in Melbourne and Brisbane, as well as a study by Shaheen et al. (Citation2010) on bike sharing system membership decisions in Washington, D.C., also support the importance of perceived ease of use in influencing individuals’ intention to adopt and use bike share systems.
To support these findings, bike share scheme operators should prioritize proposing appropriate solutions to simplify and reduce the difficulties associated with using bike share services. This can involve initiatives such as implementing user-friendly mobile applications or intuitive bike rental and return processes, ensuring bike availability at convenient locations, and providing clear instructions for users. By focusing on enhancing the ease of use of bike share services, operators can facilitate residents’ adoption and encourage regular usage, ultimately contributing to the success and sustainability of bike sharing as a viable transportation option.
8.4. External factors (EF)
The study findings indicate that external factors have a moderating negative effect on bike share intentions. Individuals who prioritize external factors such as weather conditions, traffic conditions, geographic conditions (e.g. terrain), and the availability of bicycle-friendly infrastructure when choosing their mode of transportation tend to have lower intentions to use bike share. This suggests that the perceived barriers associated with external factors influence individuals’ decision to adopt bike share services.
Supporting this notion, research by Buehler and Pucher (Citation2012) examined the impact of various external factors on cycling behaviour in different cities worldwide. The study highlighted how external factors such as weather, traffic safety, topography, and infrastructure played a crucial role in determining individuals’ cycling choices. Similarly, a study by Sylwra et al. (Citation2021); Piatkowski et al. (Citation2016) investigated the influence of environmental factors on travel behaviour, emphasizing the importance of external factors in shaping individuals’ mode selection.
To overcome these barriers, bike share operators and policymakers should address the concerns related to external factors. This can involve initiatives such as improving cycling infrastructure, enhancing safety measures, providing real-time weather updates to users, and implementing strategies to mitigate traffic congestion. By proactively addressing external factors and creating a conducive environment for bike share usage, operators can increase individuals’ intentions to use bike share services.
8.5. Behavioural attitudes (BA)
The study findings highlight that behavioural attitudes toward bike share have a substantial positive impact on behavioural intention. When individuals perceive bike share as affordable, convenient, comfortable, and intriguing, they develop a positive behavioural attitude toward bike share, which strongly influences their intention to use it. These results are consistent with previous research in the field, emphasizing the importance of these characteristics in shaping individuals’ perceptions and attitudes toward bike sharing. A study conducted by Delbosc and Currie (Citation2011) investigated the factors influencing public bicycle scheme use in Melbourne, Australia. The study found that perceived convenience, comfort, and affordability significantly influenced individuals’ behavioural attitudes toward bike share. Similarly, a study by Shaheen et al. (Citation2010) explored the impacts of various factors on bike sharing system membership decisions in Washington, D.C. The study highlighted that perceived convenience and affordability positively influenced users’ behavioural attitudes toward bike share.
To enhance users’ perceptions and behavioural attitudes toward bike sharing, bike share operators, city officials, and policymakers should prioritize these characteristics. Strategies can include ensuring affordability through flexible pricing schemes, improving the convenience of bike share systems through expanding station networks and implementing user-friendly mobile applications, and enhancing the comfort of bike share bicycles through regular maintenance and upgrades. By focusing on these aspects, stakeholders can cultivate positive behavioural attitudes among users, ultimately fostering higher intentions to use bike sharing.
8.6. Subjective norms (SN)
The study findings suggest that subjective norms have a moderate positive impact on influencing behavioural intention in bike sharing. This implies that promotional and awareness campaigns through social media, as well as the opinions of people in their social circle, can influence individuals’ decision to use bike share. It is common for individuals to align their behavioural objectives with those around them or to avoid appearing discordant with them. Due to social acceptance and peer influence, individuals are more likely to develop favourable attitudes toward bike share when they believe their friends and peers perceive bike share usage favourably. In addition, a sense of social obligation and normative attitudes might strengthen their intents to utilise public bike sharing, as individuals want to adhere to what is expected within their social circles, ultimately influencing behaviour and adoption of these sustainable transportation solutions. Therefore, bike share operators should focus on enhancing favourable publicity about bike sharing to create a positive influence on potential users. Supporting these findings, a study by Chen and Hsu (Citation2020) examined the factors influencing bike sharing adoption in Colombia. The study emphasized the role of subjective norms and social influence in individuals’ decision to use bike share. Similarly, a study conducted by Fishman et al. (Citation2013) investigated bike share schemes in Melbourne and Brisbane, highlighting the importance of social influence in shaping individuals’ perceptions and intentions toward bike share.
To improve the radiation effect of subjective norms on potential users, bike share operators should implement effective promotional strategies and engage in targeted social media campaigns. By leveraging social influence and creating positive perceptions within individuals’ social circles, operators can encourage more people to adopt bike sharing as a preferred mode of transportation.
8.7. Policy support (PC) and habits (H)
The study findings reveal that both policy support has a significant impact on bike sharing behavioural intentions. Government policies and initiatives that promote bicycling, such as investing in non-motorized transportation (NMT) infrastructure development, providing incentives for bike share schemes, and prioritizing NMT movement, have a positive influence on individuals’ intentions to use bike sharing. This underscores the importance of supportive policy measures in encouraging the adoption and usage of bike sharing systems.
The study finding reveal that Personal habits have negligible impact on bike sharing behavioural intentions. However, personal habits play a moderate role in shaping behavioural intentions towards bike sharing. Individuals who regularly use public transportation, engage in physical activities, and exhibit a passion for new items and technologies are more likely to have positive intentions regarding bike sharing usage. These personal habits indicate a predisposition towards sustainable transportation modes and a willingness to embrace innovative solutions. The literature supports these findings. For example, a study by An, Lin, and Wang (Citation2013) examined the impact of policy support on bike sharing adoption in China. The research demonstrated that government policies, such as subsidies and infrastructure development, positively influenced individuals’ intentions to adopt bike sharing. Furthermore, a study by Kamargianni, Li, and Matyas (Citation2018) investigated the role of personal habits in bike sharing adoption. The findings indicated that individuals with a regular habit of using public transportation and engaging in physical activities were more likely to adopt bike sharing.
To promote positive bike sharing behavioural intentions, policymakers should focus on implementing supportive policies that promote bicycling and NMT infrastructure development. Additionally, targeting individuals with existing habits of using public transportation and participating in physical activities through awareness campaigns can help emphasize the benefits and convenience of bike sharing. Regular use promotes familiarity, convenience, and environmental awareness, which results in positive sentiments. Automaticity-driven habitual action reduces cognitive effort, reinforces aims, and generates habit triggers. This cycle encourages a commitment to bike share services and, when widely embraced, can impact community norms, encouraging a favourable collective attitude and increased intents to utilise this sustainable mode of transportation. By addressing policy support and personal habits, bike share operators and policymakers can create an environment conducive to increased bike sharing adoption and usage.
8.8. Perceived bicycle conditions (PBC)
The study findings indicate that perceived bicycle conditions in the city have a moderate influence on behavioural intentions, but interestingly, they have a negative impact on intentions to use shared bikes. Respondents were asked to evaluate their perceptions of bicycling in the city, and it was revealed that those who reported poor experiences regarding safety, comfort, interactions with motorized transport users, and other factors had lower intentions to use bike share in the future.
This result aligns with previous research highlighting the significance of perceived bicycle conditions on bike sharing intentions. For instance, a study conducted by Yang (Citation2018) explored the factors influencing bike sharing adoption in Singapore. The findings indicated that concerns about safety, including traffic conditions and interactions with other road users, were significant barriers to bike sharing usage. Similarly, a study by Fishman et al. (Citation2013) examined bike share perceptions in Melbourne and Brisbane. The research identified perceived safety as a key factor affecting individuals’ willingness to use bike share.
To address this issue and promote positive behavioural intentions towards bike sharing, it is crucial for bike share operators and city officials to improve perceived bicycle conditions in the city. Strategies may involve enhancing cycling infrastructure, implementing traffic safety measures, and raising awareness about shared bike programs’ safety features and benefits. By addressing these concerns and improving the overall perception of bicycle conditions, stakeholders can mitigate barriers and encourage greater adoption and usage of bike sharing systems.
Overall, the findings of our study offer valuable insights for governments and policymakers seeking to promote the adoption of bike share programs. By prioritizing the construct-based strategies discussed in our research, stakeholders can effectively shape a positive perception of bicycle conditions and address the barriers that discourage potential users from embracing bike sharing. This concerted effort can lead to greater acceptance and usage of bike share systems, thereby fostering sustainable and efficient urban transportation options while promoting active and healthy lifestyles.
It is crucial for governments and policymakers to recognize the significance of investing in cycling infrastructure, improving road safety measures, and implementing awareness campaigns to highlight the benefits and safety features of bike share programs. Additionally, collaborating with local authorities to develop and implement supportive policies and incentives can further encourage the public’s intention to adopt bike sharing. By aligning efforts and focusing on these strategies, stakeholders can create an environment that not only facilitates the adoption of bike share but also contributes to the overall well-being and sustainability of urban communities.
9. Conclusion
This study represents a pioneering effort to investigate user behaviour related to the adoption of bike sharing in India by utilizing the Structural Equation Modelling (SEM) based constructs from travel behavioural theories such as TPB, TIB and TAM along with other important constructs such as policy support, habits, external factors, perceived bicycling conditions. The findings highlight that perceived ease of use, behavioural attitude, and perceived bicycling conditions have the greatest influence on the intention to use public bike share, while habits and subjective norms have the least impact. This study contributes to the literature by extending the application of the combined TPB, TIB, and TAM model to the bike share context for the first time. Furthermore, the study demonstrates that the combined model has a high explanatory power (R2 = 75.1%) compared to individual use of these models, indicating its effectiveness in explaining residents’ intention to use shared e-bikes. The study also provides practical suggestions for practitioners and policymakers to develop marketing strategies that facilitate the adoption of public bike share schemes in a developing economy like India. While the study makes significant contributions, several limitations should be acknowledged. Future research should consider incorporating additional demographic information in the SEM analysis to enable a more comprehensive understanding of user behaviour. Additionally, it is important to investigate the generalizability of the findings by including Tier 2 and Tier 3 cities in India. Furthermore, future studies should explore the behaviour and factors influencing non-bike share users to identify barriers and devise strategies for their engagement with bike sharing. Overall, this study enhances our understanding of the key drivers influencing the adoption of bike sharing in India and provides valuable insights for practitioners and policymakers. Addressing the identified limitations through future research will lead to a more comprehensive understanding of bike share usage patterns and factors influencing user behaviour in different contexts.
Disclosure statement
No potential conflict of interest was reported by the author(s).
References
- Ajzen, I. (1991). The theory of planned behavior. Organizational Behavior and Human Decision Processes, 50(2), 179–38. https://doi.org/10.1016/0749-5978(91)90020-T
- Ajzen, I., & Fishbein, M. (1970). The prediction of behavior from attitudinal and normative variables. Journal of Experimental Social Psychology, 6(4), 466–487. https://doi.org/10.1016/0022-1031(70)90057-
- Ajzen, I., & Fishbein, M. (2005). The influence of attitudes on behavior. In D. Albarracín, B. T. Johnson, & M. P. Zanna (Eds.), The handbook of attitudes (pp. 173–221). Lawrence Erlbaum Associates Publishers.
- Bamberg, S., Ajzen, I., & Schmidt, P. (2003). Choice of travel mode in the Theory of Planned behavior: The roles of past behavior, habit, and Reasoned action. In Basic and applied social psychology, 25, 175–188. https://doi.org/10.1207/S15324834BASP2503_01
- Bandura, A., & National Inst of Mental Health. (1986). Social foundations of thought and action: A social cognitive theory. Prentice-Hall, Inc.
- Böcker, L., Anderson, E., Uteng, T. P., & Throndsen, T. (2020). Bike sharing use in conjunction to public transport: Exploring spatiotemporal, age and gender dimensions in Oslo, Norway. Transportation Research Part A: Policy and Practice, 138, 389–401. https://doi.org/10.1016/j.tra.2020.06.009
- Buehler, R., & Pucher, J. (2012). Cycling to work in 90 large American cities: New evidence on the role of bike paths and lanes. Transportation, 39(2), 409–432. https://doi.org/10.1007/s11116-011-9355-8
- Chataway, E. S., Kaplan, S., Nielsen, T. S. A., & Prato, C. G. (2014). Safety perceptions and behavior related to cycling in mixed traffic: A comparison between Brisbane and Copenhagen. Transportation Research Part F: Traffic Psychology and Behav- Iour, 23, 32e43. https://doi.org/10.1016/j.trf.2013.12.021
- Chatterjee, K., Sherwin, H., Jain, J., Christensen, J., & Marsh, S. (2013). Conceptual model to explain turning points in travel behavior: Application to bicycle use. Transportation Research Record, 2322(1), 82–90. https://doi.org/10.3141/2322-09
- Cheng, C.-F., Chen, Y.-X., Mu, P.-J., & Yu, Y.-H. 2020. Beyond adoption of shared bike: A case study on antecedents and consequences of brand attachment in Taiwan. Research in Transportation Business & Management, 40, 100574. Retrieved October 1, 2020, from https://doi.org/10.1016/j.rtbm.2020.100574
- Chen, L. T., & Hsu, Y. W. (October 20, 2020). Socio-ecological predictors of frequent bike share trips: Do purposes matter? International Journal of Environmental Research and Public Health, 17(20), 7640. https://doi.org/10.3390/ijerph17207640. PMID: 33092087; PMCID: PMC7589542.
- Chen, J., Zhang, Y., Zhang, R., Cheng, X., & Yan, F. (2019). Analyzing users’ attitudes and behavior of free-floating bike sharing: An investigating of Nanjing. Transportation Research Procedia, 39, 634–645. https://doi.org/10.1016/j.trpro.2019.06.065
- Davis, F. D. (1989). Perceived usefulness, perceived ease of use, and user acceptance of information technology. MIS Quarterly, 13(3), 319–340. https://doi.org/10.2307/249008
- Delbosc, A., & Currie, G. (2011). Using Lorenz curves to assess public transport equity. Journal of Transport Geography, 19(6), 1252–1259. https://doi.org/10.1016/j.jtrangeo.2011.02.008
- Dhingra, C., & Kodukula, S. (2010). Public bicycle schemes: applying the concept in developing cities. GTZ Sustainable Urban Project. Retrieved August 38, from http://www.sutp.org/files/contents/documents/resources/B_Technical-Documents/GIZ_SUTP_TD3_Public-Bicycle-Schemes_EN.pdf.
- Dill, J., Mohr, C., & Ma, L. (2014). How can psychological Theory help cities increase walking and bicycling? Journal of the American Planning Association, 80(1), 36–51. https://doi.org/10.1080/01944363.2014.934651
- Fan, Y., Wang, W., & Yang, X. (2021). Factors influencing the adoption of shared bicycle: A mixed-methods approach. Transportation Research Part A: Policy and Practice, 145, 161–180. https://doi.org/10.1016/j.tra.2020.10.012
- Fazio, R. H. (1990). A practical guide to the use of response latency in social psychological research. In C. Hendrick & M. S. Clark (Eds.), Research methods in personality and social psychology (Vol. 11, pp. 74–97). Sage.
- Fishbein, M., & Ajzen, I. (1975). Belief, attitude, intention and behaviour: An introduction to Theory and research. Addison-Wesley.
- Fishbein, M., & Ajzen, I. (2010). Predicting and changing behavior: The Reasoned action approach. Psychology Press (Taylor & Francis), New York.
- Fishman, E., Washington, S., & Haworth, N. (2012). Barriers and facilitators to public bicycle scheme use: a qualitative approach. Transportation Research Part F: Traffic Psychology and Behaviour, 15(6), 686–698. https://doi.org/10.1016/j.trf.2012.08.002
- Fishman, E., Washington, S., & Haworth, N. (2013). Bike share: A synthesis of the literature. Transport Reviews, 33(2), 148–165. https://doi.org/10.1080/01441647.2012.751194
- Fishman, E., Washington, S., & Haworth, N. (2014). Bike share’s impact on car use: Evidence from the United States, Great Britain, and Australia. Transportation Research, Part D: Transport & Environment, 31, 13e20. https://doi.org/10.1016/j.trd.2014.05.013
- Fishman, E., Washington, S., Haworth, N., & Watson, A. (2013). Factors influencing bike share membership: An analysis of Melbourne and Brisbane. Journal of Transport Geography, 30, 63–70. https://doi.org/10.1016/j.tra.2014.10.021
- Fujii, S., & Gärling, T. (2003). Development of script-based travel mode choice after forced change. Transportation Research Part F: Traffic Psychology and Behaviour, 6(2), 117–124. https://doi.org/10.1016/S1369-8478(03)00019-6
- Gatersleben, B., Murtagh, N., & Wokje Abrahamse. (2014). Values, identity and pro-environmental behaviour. Contemporary Social Science, 9(4), 374–392. https://doi.org/10.1080/21582041.2012.682086
- Geurs, K. T., & Van Wee, B. (2004). Accessibility evaluation of land-use and Transport strategies: Review and research directions. Journal of Transport Geography, 12(2), 127–140. https://doi.org/10.1016/j.jtrangeo.2003.10.005
- Gliem, J. A., & Gliem, R. R. (2003). Calculating, interpreting, and reporting Cronbach’s alpha reliability Coefficient for Likert-type scales. 2003 Midwest Research to Practice Conference in Adult, Continuing, and Community Education, Columbus, 82–88.
- Guo, X., Chengpeng, L., Sun, D., Gao, Y., & Xue, B. (2021). Comparison of usage and influencing factors between Governmental public bicycles and dockless bicycles in Linfen city, China. Sustainability, 13(12), 6890. https://doi.org/10.3390/su13126890
- Gutiérrez, M., Hurtubia, R., Ortúzar, J., & de, D. (2020). The role of habit and the built environment in the willingness to commute by bicycle. Travel Behaviour and Society, 20, 62–73. https://doi.org/10.1016/j.tbs.2020.02.007
- Habibian, M., Hamouni, P., & Haghshenas, P. (2017). Determining the best Path for bicycle lane construction using sustainable transportation approach (case study: District 1 of shiraz). Amirkabir Journal of Civil Engineering, 49(3), 593–602. https://doi.org/10.22060/ceej.2016.683
- Hair, J. F., Ringle, C. M., & Sarstedt, M. (2013). Partial least squares structural equation modeling: Rigorous applications, better results and higher acceptance. Long Range Planning, 46(1–2), 1–12. https://doi.org/10.1016/j.lrp.2013.01.001
- Hazen, B. T., Overstreet, R. E., & Wang, Y. (2015). Predicting public bicycle adoption using the technology acceptance model. Sustainability, 7(11), 14558–14573. https://doi.org/10.3390/su71114558
- Holmes-Smith, P., Coote, L., & Cunningham, E. (2006). Structural equation Modelling: From the fundamentals to advanced topics. SREAMS.
- Hooper, D., Coughlan, J., & Mullen, M. R. (2008). Structural equation Modelling: Guidelines for determining model fit. Electronic Journal of Business Research Methods, 6, 53–60. https://doi.org/10.21427/D7CF7R
- Hu, T., Zhang, D., & Wang, J. (2015). A meta-analysis of the trait resilience and mental health. Personality and Individual Differences, 76, 18–27. https://doi.org/10.1016/j.paid.2014.11.039
- Jahanshahi, D., Tabibi, Z., & van Wee, B. (2020). Factors influencing the acceptance and use of a bicycle sharing system: Applying an extended unified Theory of acceptance and use of technology (UTAUT). Case Studies on Transport Policy, 8(4), 1212–1223. https://doi.org/10.1016/j.cstp.2020.08.002
- Johansson, L., Al-Attar, M., Adi, D., Johansson, L., Al-Attar, M., Adi Tutor, D., & Wilde Björling, M. (2022). An exploration of the factors influencing EV adoption in Sweden title: An exploration of the factors influencing EV adoption in Sweden: How consumer perceptions are impacted upon adoption of EV. May.
- Johnson, R. A., & Wichern, D. W. (1992). Applied multivariate statistical analysis. Prentice Hall.
- Kamargianni, M., Matyas, M., Li, W., & Muscat, J. (2018). Londoners’ attitudes towards car-ownership and Mobility-as-a-Service: Impact assessment and opportunities that lie ahead. MaaSLab - ( UCL Energy Institute Report). Prepared for Transport for London.
- Kaplan, S., & Prato, C. G. (2014). “Them or us”: Perceptions, cognitions, emotions, and overt behavior associated with cyclists and motorists sharing the road. International Journal of Sustainable Transportation, 10(3), 193–200. https://doi.org/10.1080/15568318.2014.885621
- Kumar Machavarapu, P., & Ram, S. (2022a). Infrastructure evaluation, role of public bike sharing systems. Global and Indian Context, Case Studies on Transport Policy, 10(3), 1841–1854. ( ISSN 2213-624X). https://doi.org/10.1016/j.cstp.2022.07.013
- Kumar Machavarapu, P., & Ram, S. (2022b). Review on public bike share schemes in large developing cities: A case study of Delhi. India, Case Studies on Transport Policy, 10(4), 2075–2091. ( ISSN2213-624X). https://doi.org/10.1016/j.cstp.2022.09.009
- Litman, T. (2019). Transportation cost and benefit analysis guidebook: Techniques, estimates and implications, VTPI. Retrieved March 2, 2022, from www.vtpi.org
- Liu, Y., & Yang, Y. (2018). Empirical examination of users’ adoption of the sharing economy in China using an expanded technology acceptance model. Sustainability, 10(4), 1262. https://doi.org/10.3390/su10041262
- Li, Z., Wang, W., Yang, C., & Ragland, D. R. (2013). Bicycle commuting market analysis using attitudinal market segmentation approach. Transportation Research Part A: Policy and Practice, 47, 56–68. https://doi.org/10.1016/j.tra.2012.10.017
- Li, S., Zhuang, C., Guo, E., Liang, K., & Liang, S. (2023). Research on bike-sharing travel behavior: A review of the Chinese language literature. Transactions in Urban Data, Science, and Technology, 2(2–3), 100–122. https://doi.org/10.1177/27541231231179397
- Ma, L., Zhang, X., Ding, X., & Wang, G. (2018). Bike sharing and users’ subjective well-being: An empirical study in China. Transportation Research, 118, 14–24. https://doi.org/10.1016/j.tra.2018.08.040
- Ma, Z., Zhang, J., & Wang, Y. (2019). Exploring key determinants of public bicycle usage behaviour in China: Evidence from Nanjing. Sustainability, 11(8), 2392.
- Pan, L., Xia, Y., Xing, L., Song, Z., & Yunbao, X. (2022). Exploring use acceptance of electric bicycle-sharing systems: An empirical study based on PLS-SEM analysis. Sensors, 22(18), 7057. https://doi.org/10.3390/s22187057
- Patel, S. J., & Patel, C. R. (2020). A stakeholders perspective on improving barriers in implementation of public bicycle sharing system (PBSS). Transportation Research Part A: Policy and Practice, 138, 353–366. (September 2019). https://doi.org/10.1016/j.tra.2020.06.007
- Piatkowski, D., Böcker, L., & Gruber, J. (2016). The influence of weather conditions on bike-sharing usage: A case study in Cologne, Germany. Transportation Research Part A: Policy and Practice, 94, 363–378. https://doi.org/10.1016/j.tra.2016.09.009
- Prochaska, J. O., & Velicer, W. F. (1997). The transtheoretical model of health behavior change. American Journal of Health Promotion, 12(1), 38–48. https://doi.org/10.4278/0890-1171-12.1.38
- Rasouli, A., & Tsotsos, J. K. (2020). Autonomous vehicles that interact with pedestrians: A survey of theory and practice. IEEE Transactions on Intelligent Transportation Systems, 21(3), 900–918. https://doi.org/10.1109/TITS.2019.2901817
- Reilly, K. H., Wang, S. M., Gould, L. H., Baquero, M., & Crossa, A. (2021). Characteristics of the social network of bike share members in New York City. Journal of Transport & Health, 22, 101128. https://doi.org/10.1016/j.jth.2021.101128
- Schwartz, S. H. (1977). Normative influence on altruism. In L. Berkowitz (Ed.), Advances in experimental social psychology (Vol. 10, pp. 221–279). Academic Press. https://doi.org/10.1016/s0065-2601(08)60358-5
- Shaheen, S., Camel, M., & Velu, M. (2011). California Department of Transportation, district 4Em ployee bikesharing pilot program evaluation. 0003(February), 44. http://76.12.4.249/artman2/uploads/1/Caltrans_District_4_Employee_Bikesharing_Pilot_Program_Final_Report_2.pdf
- Shaheen, S., & Chan, N. (2016). Mobility and the sharing economy: Potential to facilitate the first-and last-mile public transit connections. Built Environment, 42(4), 573–588.
- Shaheen, S. A., Guzman, S., & Zhang, H. (2010). Bikesharing in Europe, the Americas, and Asia past, present, and future. https://doi.org/10.3141/2143-20
- Shaheen, S. A., Martin, E. W., Chan, N. D., Cohen, A. P., & Pogodzinski, M. (2014). Public bikesharing in North America during a period of rapid expansion: Understanding business models, industry trends and user impacts. Mineta Transportation Institute ([Online] http://transweb.sjsu.edu/PDFs/research/1131-public-bikesharingbusiness-models-trends-impacts.pdf [Accessed 09/01/2015]).
- Sharmeen, F., Ghosh, B., & Mateo-Babiano, I. (2021). Policy, users and discourses: Examples from bikeshare programs in (Kolkata) India and (Manila) Philippines. Journal of Transport Geography, 90, 102898. https://doi.org/10.1016/j.jtrangeo.2020.102898
- Steg, L., & Vlek, C. (2009). Encouraging pro-environmental behaviour: An integrative review and research agenda. Journal of Environmental Psychology, 29(3), 309–317. https://doi.org/10.1016/j.jenvp.2008.10.004
- Stern, P. C. (2000). Toward a coherent theory of environmentally significant behavior. Journal of Social Issues, 56(3), 407–424. https://doi.org/10.1111/0022-4537.00175
- Sun, L., Zhou, X., & Sun, Z. (2019). Improving cycling behaviors of dockless bike-sharing users based on an extended Theory of Planned behavior and credit-based supervision policies in China. Frontiers in Psychology, 10, 2189. https://doi.org/10.3389/fpsyg.2019.02189
- Sylwra, P., Marusz, K., & D’Agostino, C. (2021, April). Impact of environment on bicycle travel demand—Assessment using bikeshare system data. Sustainable Cities and Society, 67(1), 1–8. https://doi.org/10.1016/j.scs.2021.102724
- Tiwari, G., & Jain, H. (2008). ‘bicycles in urban India’ in position papers on bicycling in Asia, interface for cycling expertise. Interface for Cycling Expertise, 3–20.
- Triandis, H. C. (1977). Interpersonal behavior. https://doi.org/10.1111/j.1749-6632.1977.tb29370.x
- Wang, X., Lindsey, G., Schoner, J., & Harrison, A. (2015). Modeling bike share station activity: Effects of nearby businesses and jobs on trips to and from stations. Journal of Urban Planning and Development, 142(1), 04015001. https://doi.org/10.1061/(ASCE)UP.1943-5444.0000273
- Xu, X., Ye, Z., Li, J., & Xu, M. (2018). Understanding the Usage Patterns of Bicycle-Sharing Systems to Predict Users’ Demand: A Case Study in Wenzhou, China. Computational Intelligence and Neuroscience, 9892134. https://doi.org/10.1155/2018/9892134
- Yang, W., Wang, S., & Zhao, X. (2018). Measuring the direct and indirect effects of neighborhood-built environments on travel-related CO2 emissions: A Structural equation modeling approach. Sustainability, 10(5), 1372. https://doi.org/10.3390/su10051372
- Yuhan, G., Yuan, Q., Wang, Y., & Park, K. (2021). The Structural relationship among perceived service quality, perceived value, and customer satisfaction-focused on starbucks reserve coffee shops in Shanghai, China. Sustainability, 13(15), 8633. https://doi.org/10.3390/su13158633
- Zhang, X., Shen, Y., & Zhao, J. (2021). The mobility pattern of dockless bike sharing: A four-month study in Singapore. Transportation Research, Part D: Transport & Environment, 98, 102961. https://doi.org/10.1016/j.trd.2021.102961
- Zhang, X., Wang, J., Long, X., Li, W., & Dragan, D. (2021 Dec 2). Understanding the intention to use bike-sharing system: A case study in Xi’an, China. PLoS One, 16(12), e0258790. PMID: 34855753; PMCID: PMC8638983. https://doi.org/10.1371/journal.pone.0258790
Appendix
Table A1. Description of latent variables used SEM model.