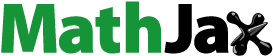
ABSTRACT
Though much research has been conducted on students’ travel behavior around the world, not much is known about university students in Sierra Leone. This study investigates the most common transport modes amongst public university students, the factors influencing their preference, and the impact of their choice on the environment. Through a Revealed Preference survey, using the traditional paper-and-pencil method combined with a smartphone-assisted interviewing technique, 632 students were sampled. Discrete travel choice models were developed and estimated, and the methodology was demonstrated for the three campuses under the University of Sierra Leone. The study found that more than one-third of students preferred the paratransit mode, ‘podapoda’, followed by taxi, two and three-wheelers. According to the mode choice model results and exploratory analysis, sociodemographics, travel time, cost, and trip distance are the main factors considered by students’ for selecting a transport mode. Students varied widely in their assessment of how their mode choice affects the environment. These findings highlight the need for a sustainable transport system for university trips. Acquiring appropriate transport modes, promoting active travel, and developing student residences with shorter commutes will ensure long-term land management. This will promote the sociocultural, economic, educational, and ecological aspects of campus growth.
1. Introduction
Since mode choice modeling influences both resource allocation and overall travel performance, it is possibly one of the most important components of transportation planning (De Ortúzar & Willumsen, Citation2002). Mode choice models use a discrete modeling technique to assess and forecast the probability that an individual will select a particular mode of transportation for a journey, taking into account both individual and trip characteristics as well as the modes that are available (Golshani et al., Citation2018). Ben-Aakiva and Lerman (Citation1985) found out that when users are presented with multiple modal options, they choose the one that best maximizes their utility. Discrete choice models have shown to be particularly effective in providing a thorough, theoretically grounded depiction of the various facets of transportation demand. These models seem to represent people’s travel behavior when they are given a variety of options to choose from. Since universities are frequently among the largest employers in the majority of growing cities, they attract a high concentration of trips during certain hours. When compared to the general public, university students use public and non-motorized transport more often (Bonham & Koth, Citation2010; Danaf et al., Citation2014a; Lundberg & Weber, Citation2014; Nguyen-Phuoc et al., Citation2018a). Cities with large student populations use public transportation and other alternative modes, like walking, biking, and motorcycles, more often than private vehicles (Etminani-Ghasrodashti et al., Citation2018; Santos et al., Citation2013). In the case study area Freetown, there is a dearth of research on mode preference modeling for large trip generators and attractors, like schools, colleges, shopping centers, business and work trips. Owing to the rapid growth of student population, government and university authorities were faced with the need to provide suitable accommodation for its students on campus. The absence of this led to disparities in demand for travel and an observed increase in patronage of public transport services dominated by low capacity but flexible paratransit modes like podapoda (minibus), taxi, keke (three-wheeler) and motorbike (two-wheeler). Historically, these modes, which are owned and operated by private individuals have been competing for the same road infrastructure, causing a lot of traffic problems, such as congestion, long queues (delay), pollution, and reduction of road capacity and efficiency. Commute for lectures by students has been dependent on these modes especially when most universities in the country were operating on day-shift, amidst the low volume of student buses available for camp ride. It has been argued that the choice of mode is one of the most important and fundamental factors that influences transportation demand in an urban setting as it directly determines the temporal distribution of traffic flow in any given transportation strip. Thus, it is important to understand the link between mode choice decisions of university students and to develop mode choice models that can mimic the travel behavior of student trips originating from different demographic areas within and outside the city. Therefore, to contribute to the existing literature with insights from a developing city, this study has investigated the most prevalent means of transportation used by public university students in Freetown, as well as the important factors that influence their choice of mode. It also explored how students perceived the impact of their choice on the environment and transportation infrastructure. The study significantly broadens the boundaries of the research field, and as far as the researchers are aware, it is the first to examine University of Sierra Leone students’ mode-choice travel behavior in the case of an unindustrialized country where paratransit modes; minibuses, taxis, two and three-wheelers have dominated the public transportation sector. This paper has addressed the following research questions in a bid to achieve the broader research objective:
Which modes of transportation are most frequently used by public university students in Freetown?
What are the most important factors that influence students’ travel choice decision?
How do students perceive the impact of their mode choice on transport infrastructure and the environment?
The paper is arranged into five sections; the first two sections introduce the study and present review of relevant literature. The third section presents a description of the study area and research approach. Section four presents the results and discussion and section five concludes the study with policy applicability and direction for future research.
2. Literature review
In different countries, the factors that influence university students’ mode choice decision encompass a wide range of natural environmental factors, as well as trip and mode characteristics. Even though there may be some overlaps between industrialized and developing nations, the factors and their effects may not entirely be the same. In the Philippines, De Guzman and Diaz (Citation2005) studied the ways in which students at Miriam College and the University of Ateneo de Manila chose their modes of transport. Based on their research, the three main factors that influence students’ preference for a particular mode are comfort, travel time, and cost. At Virginia Commonwealth University (VCU), Chen (Citation2012) performed a statistical analysis of student travel patterns. According to their findings, university students’ travel habits differ from those of the general public. Undergraduate students are likely to make more daily trips than graduate students. They revealed that home and academic activities are the most common student activities; and that student group categories have almost no influence on daily activity profiles, though activity types do have a significant impact. In McMaster university in Hamilton, Canada, Whalen et al. (Citation2013) discovered that cost, individual attitudes, and environmental factors such as street and sidewalk density influence modal choices. They also found that, the utility of car and bicycle is positively impacted by travel time, although this effect diminishes with increase in travel time. In Xanthi, Greece, Kotoula et al. (Citation2018) assessed a number of travel-related factors, including travel time, comfort, safety, comfort level, and travel distance. Additionally, because the university moved from an urban to a more rural location, they provided a better understanding of how students travel in two different environments. The results supported earlier theories, showing that in both scenarios, time and distance were the most important factors. In the United States, Zhou et al. (Citation2018) investigated the factors that influence college town students’ mode choice. In contrast to their peers at urban universities, they found that students are more likely to choose more environmentally friendly (non-driving-alone) modes of transportation, such as walking, for their commutes. In a more recent study, Nasrin (Citation2020) explored the mode choice behavior of private university students traveling to universities using Dhaka as a case study. Results showed that the primary determinants of the various modes of transportation that private university students choose for their educational trips are cost, comfort, time, and availability. They also showed that, in comparison to travel time and cost, the magnitude of the coefficient of attribute ‘comfort’ is substantially higher. Additionally, in terms of non-motorized modes, Henning et al. (Citation2020) recently analyzed the factors that affect students’ decision to use active modes of transportation at a university campus in Joinville, Brazil. Findings show that, travel time, university, income, and marital status all affect modal choices.
Despite these interesting findings, some studies in developing countries revealed significant differences in socio-economic factors like gender, age, and university degree. Such factors would normally have a large impact on mode choice and other types of travel behavior in developed countries (Faghih-Imani et al., Citation2017). Studies in developing cities revealed that users are more concerned about travel cost and time, which varies across income levels, as observed by (Akar et al., Citation2013; Hasnine & Habib, Citation2018; Srinivasan et al., Citation2007). Travel time to campus, travel cost, trip distance, and bus stop waiting time are all factors that influence whether a student walks, rides a motorbike, or takes the student bus to campus. Similar findings were also reported by (Nkegbe, Citation2012). Santos et al. (Citation2013) found that cities with higher student populations are inclined to using public transportation and other modes, like walking, biking, and motorbikes, especially given the high percentage of motorbike usage in developing nations. To underscore the influence of socio-demographics on students’ modal choice, Nguyen-Phuoc et al. (Citation2018b) investigated university students’ travel mode preferences in a developing world where motorcycles have long dominated traffic systems. The key findings show that student’s age, gender, and income have a major effect on their mode selection decision. Walking as a mode of transportation to school was found to have a strong negative impact on travel time from home to school. Students who commute to school on motorcycles are often willing to turn to public transportation if an accessible and effective public transportation system is available. In Aizawl, a rapidly expanding hilly city in northeast India, Saitluanga and Hmangaihzela (Citation2022) conducted a more recent study to examine the travel habits of non-local, off-campus college students. Their investigation revealed that students’ preference for a particular mode of transport is impacted by a number of interconnected factors, such as housing location, socioeconomic status, demography, and the availability of travel modes. Of the college students surveyed, it was also discovered that walking and public transportation are the most popular ways to get around. The likelihood of walking is higher among female students who rent houses close to their colleges compared to their male peers. Male students from wealthy families, on the other hand, typically use private vehicles from their homes, which are situated somewhat distant from the university.
From the literature, it is obvious that students from both developed and developing countries consider different factors (with some commonalities) when making their modal choice for university trips. However, to the best of the researchers’ knowledge, no research on mode choice decision of Sierra Leonean university students, both public and private, was uncovered. This was addressed by the current study by exploring travel diary data and understanding the result from the perspective of mode choice model of public university students in Sierra Leone. This contributes to the existing literature on university students travel pattern in other countries. Some aspects of this study may also help to control and authorize the privately owned and operated paratransit industry, that offers the majority of public transport services to students for a safer, more dependable, reliable, and affordable travel experience.
3. Methodology
A simple random sampling with revealed preference (RP) questionnaire survey was adopted in this study. The overall research design and flow process is illustrated in . In addition to the literature review presented in section 2, each design process is discussed in the sub-sections that follow.
3.1. Characteristics of the study area
The study was conducted at the University of Sierra Leone which is situated in Freetown, on the Western region of Sierra Leone. The university constitutes of three colleges; Fourah Bay College (FBC) which is located in the neighbourhood of Mount Aureol in Freetown, Institute of Public Administration and Management (IPAM) located in central Freetown and College of Medicine and Allied Health Sciences (COMAHS) whose main campus is around the peninsular in the Western Rural side of the city (). These campuses were chosen for the survey as they attract considerable number of students every academic year. Students from Fourah Bay College and the Institute of Public Administration and Management frequently contribute to traffic congestion, particularly during rush hour. Comparatively, COMAHS students have relatively low effect on traffic congestion. What is even more alarming is that, because FBC is situated within a hilly or mountainous environment without adequate traffic control systems, students attending the college are extremely vulnerable to traffic accidents.
Generally, the country’s capital and largest emerging urban centre and chief port, Freetown has historically been plagued by extreme traffic congestion and urban air pollution as a result of high public car dependence, coupled with long distance travel by various categories of road users. It has an approximate land area of 82 km2 and a population of around 1.5 million people (both urban and rural areas) (Census, Citation2015). Many universities have been established in this city in recent years, and some traditional ones were planning to expand in order to accommodate the growing number of student population. These campuses would become major trip production and attraction centers, necessitating better planning and coordination.
3.2. Survey design and sampling
The research was designed with mixed data collection method using revealed preference (RP) survey which provided the data for the models described in this work. The questionnaire was made up of closed-ended questions, which were accompanied by answer options to explore the students’ responses. This was done in an effort to gather data on how university students behave when traveling for academic purposes. Students were asked to answer three sections of an RP questionnaire as part of the survey. The first section addressed trip-related characteristics. The second section focused on attitudinal and behavioral statements with respect to transportation infrastructure and the environment. Ten statements that impact the environment, service satisfaction, and mode of transportation were presented to be ranked in order of significance by the respondents. Like most transport surveys, a 5-point Likert scale was adopted (Basbas et al., Citation2021; Campisi et al., Citation2020; Malhotra & Dash, Citation2007; Rodriguez-Valencia et al., Citation2019; Taherdoost, Citation2019). Sociodemographic data (sex, age, education level, average household monthly income, and family size) are included in the third section of the survey instrument. Since the total student population size for the three campuses was not available as at the time of the survey, a more reliable estimate of the sample size was computed using a relationship formulated by Cochran (Citation1977), given in Equationeq. (1)(1)
(1) . This formula is based on proportions and allows easy estimation of an optimal sample size given a desired level of precision, desired confidence level, and the estimated proportion of the attribute present in the population. It is ideal in circumstances where the population size
is predicted to be large, such as the three campuses combined.
Where;
is Cochran’s recommended sample size
is the
-value at 95% confidence level (
1.96)
is the desired level of precision (i.e. the margin of error
5%),
is the (estimated) proportion of the student population which has the attributes in question = 50% (maximum variability). Variability denotes the degree to which the characteristics measured in the questions are distributed throughout the population. Higher degree of variability ensures:
distribution of the attributes to be within the target audience and
larger sample size in order to obtain the same level of precision
We have selected a conservative number of 50% to establish a balance between the two. With this information in Equationequation 1(1)
(1) , a minimum sample size was estimated as 384. However, we adopted a sample size of 632 in order to account for model calibration and validation, potential omissions, and uncertainties in data sampling and collection.
3.3. Survey method and implementation
In this study, traditional paper and pencil-based questionnaire method harmonized with online survey using smartphone assisted self-interviewing technique was adopted. With this approach, sufficient data were collected within limited time and budget. Prior to survey administration, potential enumerators who were fresh graduates from the University of Sierra Leone were recruited and trained. This was done to ensure quality data collection especially when the enumerators are familiar with the study area and sample population. During the survey, all qualified participants were informed about the objective of the study and were assured of data protection and privacy of their responses. The paper and pencil-based questionnaires were distributed to students in their lecture theatres, peace, society or faculty gardens, library, laboratory, cafeteria and hospitals (especially for students from College of Medicine and Allied Health Sciences who were assigned to various hospitals across Freetown on clinical posting). All questionnaires were completed instantaneously with the guidance of the enumerators and none were left in the hands of students to be completed at a later time. Additionally, for the online survey, smartphones were equipped with a web page of the questionnaire in Google Form. The Google link was enabled to allow multiple responses from different students using the same smartphone. The enumerators remained available for instruction and to offer any assistance related to the questionnaire. Once the students submit their responses, the data were stored in the Google Form account of the researcher, which were then downloaded for analysis. In both methods, students were cautioned to submit their responses once and not to share the questionnaires with colleagues from other universities. Overall, the average questionnaire completion time was 15 minutes. The survey was administered in early June, 2021 and was completed in early July the same year. This was a little over one year when the first case of COVID-19 was reported in Sierra Leone (MoHS, Citation2021). It was also a period when students were preparing for their first semester exams. The target sample reflects respondents who were registered as fulltime students at the three university campuses for 2020/2021 academic year. All categories of students pursuing different programs at undergraduate and postgraduate levels were surveyed. The survey was conducted in day time, 10 am − 5 pm on college days, Monday – Friday. Despite the participants were randomly selected from the student population, and the unequal ratio of males to females, the implementation phase was very sensitive to gender. A total of 449 (71%) paper-based and 183 (29%) online valid responses were received.
3.4. Model estimation and goodness of fit assessment
About 80% of the overall sample representing 509 cases was randomly chosen from the sample data and utilized for model estimation, while the remaining 20% was used for model validation. With the estimation dataset, a Multinomial Logistic Regression model that constitutes of sociodemographics, service satisfaction, trip and travelers’ related attributes () was developed and estimated using BIOGEME, an open-source python package.
Table 1. Model estimation parameters.
Maximum likelihood, a classification technique that applies logistic regression to multi-class problems with more than two possible discrete outcomes, was used to estimate the model parameters (Lagani et al., Citation2013; Samuel et al., Citation2020; Yin et al., Citation2018). Based on descriptive data analysis, students’ modal preference was modeled using five modes: podapoda (minibus), motorbike (two-wheeler), taxi, keke (three-wheeler), and NMT (walking and cycling).
The deterministic share of the utility is formulated as:
Where;
is the net utility function for mode
, for individual
is the alternative specific constant for mode
…
are
number of attributes of mode
for individual
and;
…
are
number of coefficients of mode
which need to be estimated from the survey data
More specifically, the utility for each travel mode is specified as:
Since the students did not consider private car, and student buse as viable choices, they were not included in the model structure. Podapoda (minibus) was used as the reference alternative while various specifications were tested to determine which one best replicate the data for education trips. The utility function parameters were compared and more potential variables were tested using a trial-and-error method. Until an optimal fit was obtained, the fundamental functions were adjusted. To assess the model’s goodness of fit, fitting parameters such as Akaike Information Criterion (AIC), Bayesian Information Criterion (BIC) and the asymptotic Rho-Squared were considered. These indices are mathematical ways for assessing how well a model fits the data from which it was derived. They are used to compare different models in order to determine which one fits the data best. AIC and BIC assess the plausibility of the model by focusing on minimizing information loss rather than evaluating improvements in the model’s ability to explain data responses (Hauber et al., Citation2016). Lower AIC and BIC values indicate better fit (Aho et al., Citation2014; Lin et al., Citation2017). The asymptotic Rho-Squared on the other hand measures the fraction of an initial likelihood value explained by the model and it returns values between 0 and 1, with 0 indicating a poor fit and 1 indicating a perfect fit. Values of 0.4 and above, according to Costanzo et al. (Citation1982) maybe considered as excellent fits.
4. Results and discussion
4.1. Results of the descriptive analysis and discussion of key sociodemographics and trip characteristics
The descriptive statistics for the sample data are presented in . The national age pyramid in Sierra Leone has 11 categories with a relatively broad base (Census, Citation2015). It is skewed towards children between the ages of 0–9 years who are younger than university age. Given that the current study was intended for university students, age segmentation that is appropriate for this target sample was necessary (). It was found that, the majority of university students (93.8%) are between the ages of 18 and 35, thus constituting a relatively young to mid-age demographic group. Their early college enrollment following high school graduation placed them in this competitive group. Of the 632 respondents, 289 were female (46%) and 343 were male (54%).
Table 2. Attributes of respondents.
Just roughly 12% of the students were in their final years of study (years 4 and 5), while the majority of the students (67%) were in their preparatory years (years 1 and 2). It was found that first-year students were significantly more likely to use public transportation to get to college than second, third, qualifying, or final-year students. When designing a public transportation system, a university campus with large influx of first-year students should be considered a major attraction center. Final-year students typically have fewer contact hours on campus and are more likely to be involved in internships and research, which is reflected in the low number of final-year students who use motorized means of public transport. On the other hand, only 5% of the students were in ‘other’ levels. This is essentially a representation of COMAHS since it has both ‘year’ and ‘Pre-Med’ as strata.
In terms of day and campus scholars, approximately 64.2% of students live more than 5 km from the three campuses, and they spend a little over 40 minutes to get to college using different means of transport. These students spend at least 50,000 SLL ($5) a week and 200,000 SLL ($20) a month on transport. Since the average household monthly revenue of these students is approximately 750,000 SLL ($75), it can be inferred that, in the absence of any other source of income, transportation accounts for approximately 27% of their household monthly revenue. Only about 4% of the student population lived within a 1-kilometer radius of each campus and most of them attend COMAHS. This might be because of its geographic location as well as the fact that major government hospitals in Freetown have provisions for clinical posting of COMAHS students.
In terms of mode choice and travel distance, the study demonstrates a strong case against using NMT for long-distance travel. Since majority of students reside more than 5 km from campus, it is highly likely that they will not use NMT. This finding is consistent with that of (Babey et al., Citation2009; Martin et al., Citation2007; McDonald, Citation2008; McDonald et al., Citation2011; McMillan, Citation2002) who revealed that a household’s likelihood of walking or biking to school only increases when it is less than a mile away. Many students in the sample began to use motorized forms of transportation at distances of 2-5 km. Walking and cycling are great, but they are not appropriate for long distance travel, particularly for students who are carrying a backpack and other items. The findings verified that students are probably going to walk or ride their bikes (where possible) for shorter distances (up to 1 km). When the distance surpasses the allowable limit, motorized forms of transportation become their most preferred means, especially for students who reside farther than a 5-kilometer radius.
In terms of public transport mode choice, findings revealed that, a little less than half of the students opted for the paratransit mode, podapoda (minibus) as their preferred mode of transportation to campus (). At 6% of all trips, walking and cycling were the least popular means of transport among college students. In the absence of electric bikes in the country, these modes were combined to a single transport mode; ‘Non-Motorized Transport’ (NMT). Given that motorbikes are the primary means of transportation in the majority of developing nations, it is not surprising that both male and female university students cited them as their second most popular mode of transportation. The findings of this study mirror Freetown’s current modal split (). However, this research has also revealed the modal share of other travel modes for both motorized and non-motorized transport (Serena, which is a variant of podapoda/minibus and NMT). Overall, there is a connection between students’ preference and the general travel pattern in the city in terms of public transport use (. The results are comparable except for taxi and bus whose modal usage showed a significant decline in students’ preference. Although this comparison may not be entirely ideal (since Freetown’s modal split is for various trip purposes), it does provide insights on the limited number of public transport taxis and student buses available at the three campuses. Similar to kekes’, motorbikes’, and podapodas’, the majority of taxis’ in Sierra Leone are privately owned and operated. Traditionally, they have served as flexible public transportation modes under the demand-responsive paratransit sector which constitutes about 70% of motorized transport as seen in the current modal split (). This sector still controls the majority of the city’s public transportation system, particularly in the absence of on-demand services like Bolt, Uber, inDriver and others. If students prefer to use the bus, the decline in taxi usage will be positive since the number of cars on the road will be reduced, resulting in less traffic congestion, which will eventually preserve the urban transport space.
Figure 3. (a) Distribution of students’ modal share (Source: Authors’ field data) (b) Current motorized transport modal split in Freetown (Source: Statistics Sierra Leone, 2015; 2017).

Figure 4. (a) Graphical representation of transport modes (b) Distribution between university campus and preferred mode (Source: Authors’ field data).

By modal share per campus, FBC students are accustomed to taxi (55.3%), serena (a variant of minibus) (71.4%) and two-wheeler (43.2%), given that the college is located on a mountainous environment that can be relatively easily accessed by these modes (). Most students that engaged in active travel to get to campus are from COMAHS. This is because the largest fraction of students at the school reside less than one kilometer away. Particularly for long distance trips, motorbike and keke are the country’s comparatively expensive means of transportation. In Sierra Leone, the cost of transportation varies depending on the mode, with motorbikes, kekes and taxis, having the highest fare, in contrast to podapoda, which is almost always less expensive regardless of distance. However, the cost of transportation for these modes increases depending on the journey hour, as operators and their assistants (apprentices) routinely increase transportation fares during rush period.
In terms of private car use, only about 1% of students drive to campus. Historically, the use of private car has not been prevalent in Sierra Leone. As one of the least developed countries in the world in terms of GDP per Capita, people can barely live on $5 per day (Unctad, Citation2023). Due to the COVID-19 pandemic and the ensuing economic downturn, 4.8 million people (about 58.4% of the country’s population) were living on less than $1.90 per day in 2021 (Randy Aikins, Citation2023). The majority of people in the country live in multidimensional poverty, with about 25% of them unable to afford a basic meal. Given these prevailing circumstances, most college students and their households cannot afford a private car for travel to campus. They therefore rely on the services offered by the paratransit sector for various trip purposes. For the few students whose families can afford private cars for campus trips, they may have been deterred from driving due to time spent stuck in traffic, high auto maintenance costs, and higher fuel usage which may influence their decision, since majority of them are highly dependent on their family’s income. Based on the distribution of students with and without a driver’s license, the majority of students, about 89% () do not have a driver’s license. This could be another reason for the very low percentage of students who drive to campus. However, because a higher percentage of the students were over the age of 18, they are qualified to earn a driver’s license under the country’s eligibility requirements.
In terms of the impact of students’ mode choice decision on the environment and transportation infrastructure, shows that students exhibit a highly diverse range of behavior. In general, they are more cognizant of the need for campus buses to have Wi-Fi, as well as the need to reduce travel times, deal with traffic congestion, and ensure environmental safety. Additionally, they expressed little interest in driving private cars to campus. This suggests that, they are less supportive to the notion of commuting by private automobile as being safer, more economical, and more reliable than taking public transportation. This finding supports the call to reduce private car dependency in order to achieve sustainable urban environment in congested cities (Adbelhamid et al., Citation2018).
4.2. Results of model estimation and discussion of key factors
During model estimation, the utility functions’ parameters; Equationeqs. (3)(3)
(3) -(Equation7
(7)
(7) ) were compared and potential variables were tested using a trial-and-error method. Until the best fit was obtained, the fundamental functions were adjusted. Based on model fitting parameters like the asymptotic Rho-Squared, the Akaike Information Criterion (AIC), the Bayesian Information Criterion (BIC), the Likelihood Ratio Tests, and test statistics, the better performing model was selected after multiple iterations, indicating a higher level of explanatory ability than its counterparts. This model shows the least AIC and BIC values, along with reasonable asymptotic Rho-Squared and Likelihood Ratio values. The results revealed that this model’s likelihood ratio when combined with additional policy variables is correspondingly higher, and it outperformed all other models in terms of reasonable prediction during model validation. The researchers’ ability to identify the variables elucidating students’ mode choices and describing their distinct mobility behaviors was made possible by the minimum model’s estimation results. reports the p-value, t-statistics, standard error (SE), and logistic coefficient for each predictor.
Table 3. Model estimation output.
4.2.1. Model results for the choice of podapoda (minibus)
According to the findings, there are three factors that influence students’ choice of minibus as presented in Equationeq. (3)(3)
(3) . This mode is the most preferred among the five and across distribution by campus as it is highly favoured by 38.6% of IPAM and 34.2% of COMAHS students (). According to the model validation results, out of 53 students who chose this mode, 51 were correctly predicted representing 96.2%, revealing the highest prediction accuracy for this mode (). This indicates that the majority of observations choosing podapoda are not misclassified as other modes. Also, as performance indicators, the model predicted the mode choice distribution of this mode with reasonable accuracy at the coverage level with a relatively good coverage rate.
Table 4. Model prediction results.
The estimated coefficient of travel cost for this mode is negative (−1.45) as expected in theoretical modelling. This variable’s absolute t-statistics value is 5.10, which is higher than the critical value of 1.96 at 95% confidence level (). This suggests that when travel expenses increase, students’ patronage for this mode declines. They may therefore make less long-distance journeys to college with this mode. Findings also revealed that students are more sensitive to travel cost than journey time since travel time was found to be statistically insignificant. This implies that a unit increase in travel cost reduces the utility of this mode for more students. This could be because the majority of the students are relatively young and mid-age people between the ages of 18 and 35, who do not have a sustainable source of income. Students in this group are more adaptable to discomfort and have more time flexibility than they do with their money. As a result, they prefer to take public transportation when traveling to campus. These findings are similar to those of Yu (Citation2018), in their investigation into the variables influencing University of Waterloo students’ decisions about which intercity mode to choose as well as the relative weights of the variables that were found to have an impact. The demand for this mode appears to be extremely elastic, with a direct travel cost elasticity of −4.86 (). This suggests that for every 1% increase in travel cost, the patronage of this mode decreases by 4.86%.
Table 5. Aggregate elasticity of public transport modes.
In the literature, the trip variables that are most frequently studied are trip length and in-vehicle travel time. These attributes are closely related to one another and are often viewed as being interchangeable (Ton et al., Citation2019). In this study, the estimated coefficient of trip distance for minibus is 1.55. This parameter’s absolute t-statistics value is 5.91, which is higher than the critical value of 1.96 at 95% confidence level. This implies that, students are more likely to patronize the services of this mode as their trip length to campus increases. Because the majority of students live more than 5 km from campus, this high level of certainty strongly supports the use of podapoda. This outcome is comparable to that of (Ewing et al., Citation2004; Yeung et al., Citation2008) on the importance of trip distance in mode choice preference. In terms of comfort, the model demonstrates that students prefer this mode despite the fact that it is not as comfortable as driving a private automobile with an estimated coefficient of 0.29. This parameter’s absolute t-statistics value is approximately 3.16, which is higher than the critical value of 1.96 at 95% confidence level. Students may have felt at ease with the comparatively low transit costs connected with this modality and that travelling with their peers to school offers them comfort. They may have also felt comfortable listening to pleasant music, radio and watching a movie on their way to school as most drivers of this mode do provide. However, students may less likely depend on podapoda seats for comfort as most of them are not as comfortable as those of student bus and other modes.
4.2.2. Model results for the choice of motorbike and taxi
Findings revealed that, there are four common factors that influence students’ choice of motorbike and taxi as presented in Equationeqs. (4)(4)
(4) and (Equation5
(5)
(5) ), a fifth factor, trip hour was only found to influence taxi users. These modes are respectively the second and third most preferred among the five. Across distribution by campus, motorbike was highly favoured by 43.2% of FBC and 40.8% of IPAM students, whilst 55.3% of FBC and 31.5% of COMAHS students are accustomed to taxi (). The results also show that 18 out of 34 students who chose motorbike (52.9%), and 3 out of 10 students who chose Taxi (30%) were correctly predicted (). The percentage of correct prediction for motorbike offered the third highest prediction accuracy after NMT. Slightly more than half of the observations choosing this mode are not misclassified. Also, as performance indicators, the model predicted the mode choice distribution of the two modes with reasonable accuracy at the coverage level with a relatively good coverage rate.
For these modes, the estimated coefficients of travel time and cost are negative as the hypothetical model predicts. Motorbike usage will decline if travel costs rise proportionately to distance traveled, according to an estimated coefficient of −1.02. This parameter’s absolute t-statistics value is approximately 3.68, which is higher than the critical value of 1.96 at 95% confidence level (). Low patronage of this mode is linked to poor safety, air pollution, and hot, muggy weather, which is especially difficult for long-kilometer travel (Bray & Holyoak, Citation2015; Ferreira et al., Citation2022). Accordingly, the more time it takes for students to travel, the less useful these modes become. The estimated travel time coefficients show that students prioritize travel time over travel cost, with the exception of three-wheeler (keke), whose users place more premium to the latter. These modes (two-wheeler and taxi) are faster than three-wheeler and the reference minibus mode, which can also be explained by their higher t-values for travel time. Despite the high cost of this mode of transportation (up to two times the transport fare for a taxi or student bus), students can travel to their desired destination with greater flexibility when using motorbike. Overall, cross time elasticities estimated for taxi, and three-wheeler differ, with taxi having the highest, implying that taxi users are more sensitive to changes in their travel duration than users of two and three-wheelers (). This finding is also supported by the estimated coefficient of travel time for taxi (−1.15) which is higher than that of two and three-wheelers; −0.96 and −0.69 respectively. Similarly, when compared with two-wheelers, the cross and direct cost elasticities for three-wheelers are quite higher, suggesting that users of three-wheelers are more concerned with changes in trip cost. The researchers’ however believed that public transportation, particularly motorbikes, do not equal the desirable characteristics of podapoda, keke, and taxi, and was not sufficiently compelling to overcome the appealing features of these modes. Taxi and motorbike’s estimated bus stop waiting time factor is generic and have a negative sign (−0.30). This parameter has an absolute t-statistic of approximately 2.81, indicating statistical significance at 95% confidence level. In comparison to podapoda users, students who utilize taxis and motorbikes spend less time waiting at bus stops. Students who use active transport to college do not have to wait at bus stops.
In terms of comfort, the model demonstrates that students prefer taxi with an estimated coefficient of 0.26, and absolute t-statistics of 2.74, which is above the threshold of 1.96 at 95% confidence level. On the other hand, the coefficient of this variable is negative for motorbike with an estimated parameter of −0.24, implying that students are less dependent on this mode for comfort as compared to podapoda. This may be due to the finding that majority of students resided outside a 5-kilometer radius from campus, and that commuting with motorbike for such a distance could expose them to health risks and traffic-related problems. Of the percentage of students who travel to school during the morning rush hour, only about 15.3% are using motorbikes. This relatively low percentage is due to the annoyance and discomfort associated with this mode in the early hours of the morning. The level of risk involved in the use of motorbike, particularly for long-distance travel during rush hours, was found to have negatively influenced students’ decision to use that mode of transport. This finding supports numerous studies, including those of (Hoang & Okamura, Citation2020; Kudebong et al., Citation2011).
4.2.3. Model results for the choice of Keke (three-wheeler)
For this mode, there are four factors that influence students’ choice as presented in Equationeq. (6)(6)
(6) . This mode is the most recent public transport mode in the country and across distribution by campus, as it is highly favoured by 42.9% of IPAM and 47.6% of COMAHS students (). Model validation results also revealed that 1 out of 14 students who chose this mode were correctly predicted, representing 7.1%, offering the least prediction accuracy. This indicate that the majority of observations choosing this mode were misclassified. Also, as performance indicators, the model predicted the mode choice distribution of keke with very low accuracy and coverage rate.
Accordingly, the estimated coefficients of travel time and cost for this mode are negative as expected in hypothetical modelling (−0.69 and −1.42, respectively). These variables absolute t-statistics values are approximately 2.85 and 4.92 in that order, which are all higher than the critical value of 1.96 at 95% confidence level (). This suggests that when travel time and cost increase, students’ utility for this mode will decrease.
In contrast to two-wheeler, taxi and NMT whose users are time sensitive, users of three-wheelers place more premium on travel cost. The demand for this mode appears to be extremely elastic, with a direct fare elasticity of −5.61 (), implying that for every 1% increase in travel cost, the market shares of keke decreases by 5.61%. In contrast, the demand for this mode is quite inelastic with a very low cross-elasticity of 0.77, implying that for every 1% increase in the motorbike travel fare, the usage for this mode will rise by 0.77%. When this happens, more students are likely to patronize three-wheelers than two-wheelers. However, because the elasticity assesses the percentage change in the modal share from the base share, the growth in keke users is not as large as the elasticity estimate suggests.
With an absolute t-statistics value of 3.61 and a positive coefficient of 0.73, the age variable for three-wheeler (keke) is higher than the critical value of 1.96 at the 95% confidence level (). As a result, relatively older students are less likely to engage in active travel and more likely to use motorized transport. This result supports the findings of (Mackett & Ahern, Citation2000), but inconsistent to some respect with those of Dias et al. (Citation2022), who found out in a study carried out in Kandy, Sri Lanka that, older students are more likely than younger students to walk, take the school bus, and use public transportation. Conversely, the gender variable showed a negative sign in the utility function for three-wheeler, suggesting that male students are more likely than females to use this mode. This finding should be better explored by sampling more categories of three-wheeler users, since this is the country’s most recent travel mode. What is more clear is that, student demographics have a significant impact on keke modal preference, often functioning as considerably stronger predictors of this mode, as they have been shown to be highly sensitive to age and gender. Many of the findings in previous research such as those of (Danaf et al., Citation2014b; Das et al., Citation2016; De Guzman & Diaz, Citation2005; Khattak et al., Citation2011; Mahlawat et al., Citation2007; Nguyen-Phuoc et al., Citation2018b) were confirmed, particularly in terms of the effects of socio-demographic and trip-related characteristics like age, and gender.
4.2.4. Model results for the choice of Non-Motorized Transport (NMT)
This research revealed six factors that influence students’ to engage in active travel as presented in Equationeq. (7)(7)
(7) . This mode is the least preferred among the five and across distribution by campus, though highly favoured by 45.7% of COMAHS students (). This was expected since this campus has the highest percentage of students who live within a 1 km radius. The results also show that 3 out of 4 students who chose Non-Motorised Transport were correctly predicted (75%); offering a good prediction accuracy for this mode. This indicates that the majority of observations choosing this mode are not misclassified. Also, as performance indicators, the model predicted the mode choice distribution of NMT with reasonable accuracy at the coverage level with a relatively good coverage rate.
The results demonstrate that, the estimated travel time coefficient for NMT is negative (−2.15) as expected, having an absolute t-statistics value of about 4.73 () much over the decisive t – statistics at 95% confidence level. This shows that the usefulness of active modes decreases with trip time, corroborating the idea that travel time is a significant factor in students’ choice of mode of transportation. Thus, students may make less long-distance trips as walking and cycling become slower. Also, the travel distance parameter yielded a negative sign (−1.62). This variable’s absolute t-statistics value is approximately 3.54, which is higher than the critical value of 1.96 at 95% confidence level. This implies that students who travel longer kilometers are less likely than those who travel shorter distances to engage in active travel, which is in line with the findings of (McMillan, Citation2002; Simons et al., Citation2017; Song et al., Citation2013). The elasticity results in also support this finding. For these modes, travel distance is the most important factor in deciding whether or not to cycle, with greater distances being linked with these modes negatively (Heinen et al., Citation2010; Ton et al., Citation2019). When the distance surpasses the 1.5–2 km allowable walking threshold, motorized modes of transport will become their most preferred means (Kelly & Fu, Citation2014; Mammen et al., Citation2014). Students that live within a 1 km radius from their campus prefer to use active modes of transportation. Many students in the sample began to use motorized forms of transportation at distances of 2–5 km. According to the model’s findings, a sense of safety and proximity to bicycle lanes and pedestrian routes may boost the likelihood of walking or biking to campus. Additionally, non-motorized transportation can provide a safe and convenient alternative to driving, lowering traffic congestion and emissions from mobile sources. Through greater possibilities for student contact, this approach can contribute to enhanced societal health, serve as a recreational outlet, and promote a socially active community.
Because Non-Motorized travel entails the expenditure of physical energy, an individual’s age is likely to influence his or her decision to choose active modes of transportation. With an estimated coefficient of −1.90, having an absolute t-statistics value of approximately 2.87, the socio-economic variable age indicates that older students are less likely to walk or cycle to campus for lectures than younger students, and that these students prefer to use motorized modes of transportation, comparable to the findings of (Mackett & Ahern, Citation2000). In comparison to podapoda mode, this group of older students were more likely to use keke. Notably, gender showed up with a positive sign in the NMT utility function (parameter estimate of 1.76 with an approximate t-statistics of 2.62), suggesting that men are more likely than women to use active modes. This finding corroborates that of McMillan et al. (Citation2006) and is compatible with the Sierra Leonean culture. Additionally, the degree program of students was observed to have a substantial influence on mode selection (parameter estimate of 1.18 with an approximate t-statistics of 2.74). Students pursuing higher degree programs were found to use active modes more frequently, which is consistent with the conclusion of (Atasoy et al., Citation2013). Individuals with higher educational qualifications are more likely to select an active or environmentally friendly mode of transportation. Students in this group are more aware of the environmental consequences of their actions, which is thought to be the basis for this observation.
4.3. Results of value of travel time (VoT) reduction
One of the widely studied concept relevant to travel preference is the trade-off between time and cost. presents an overview of the key factors influencing students’ choices for each of the examined PT modes. Users of all modes clearly prioritize travel time, with the exception of minibus, for which this parameter was statistically non-significant. Given that it is the most important factor for three of the five modes, it is even more important than cost. Students view their time spent traveling to college positively. Only in the podapoda and keke modes – where students gave significant consideration to distance and travel cost respectively, was the primacy of time challenged.
In order to better understand students’ preference between time and cost, we estimated the maximum amount of money that they are willing to give up in order to save one unit of their travel time – the value of travel time (VoT) – as long as all other trip-related factors stay the same. The ratio of parameter estimates for travel time and travel cost is used to estimate this value (). It is important that both travel time and travel cost be statistically significant in order to compute VoT; otherwise, no meaningful estimate can be made. This value was only estimated for taxi, motorbike, and keke because travel time for minibuse was found to be statistically insignificant, and NMT users are presumed not to pay any direct transportation fare. According to the findings, students who take a taxi or a motorbike have a high value for travel time, as they are willing to pay an additional SLL56.5/hr and SLL58.0/hr respectively, while keke users are only willing to pay an extra SLL29.2/hr. This further confirms the supremacy of cost over time for keke users. Students’ relatively low value of travel time was not surprising, given that the majority of them come from low-income families. Reducing travel time allows students to make better use of their extra time by being more productive with their studies and this is similar to the findings of (Banister et al., Citation2019; Wadud & Huda, Citation2019). Another benefit of reduced travel time is to inform decision-makers about the merits of implementing sustainable transport options for campus trips. Reaction by students using various transport modes to a change in the transport network depends on how much value they place on travel time. More generally, the estimated value of travel time reductions serves as a stand-in for the ultimate financial return on investment from investing in a transportation project. This return is derived from lower transportation costs to industry, better job access, and increased competition (Tsolakis et al., Citation2011).
5. Conclusion and policy applicability
We have examined students’ travel pattern for three public colleges. The findings highlight the importance and viability of modeling mode choices for short and long-distance travel from both rural and urban areas, as well as the important role of trip distance, built environment, personal attitudes, and sociodemographic factors in university students’ mode choice decision. Overall, podapoda, motorbike, taxi and keke are highly patronized by college students and that their preferences vary according to their sociodemographic, trip characteristics and features of the built environment. Among the public transport modal share, IPAM students heavily depend on kekes’, motorbikes’, and podapodas’, while FBC students are more accustomed to taxis’ and motorbikes’. Students at COMAHS, however, rely on taxis, podapodas, and kekes. The findings also show that the closer a student lives to the university campus, the more likely s/he will choose active modes of transport. Even among students who live somewhat far from school, providing the infrastructure of bike paths and sidewalks can help to increase the number of active commuters. Students’ positive attitude towards the time it takes to commute to college is evident in their preference for travel time over cost. However, the value for time was only undermined in the minibus and three-wheeler, where travel distance and cost were significantly considered by students in that order. University students were found to be quite heterogeneous when it comes to evaluating the potential influence of mode choice on the environment and their travel behavior. What is even more clear is that, student demographics have a significant impact on the choice of three-wheeler, often functioning as considerably stronger predictors of this mode, as its preference is significantly influenced by age and gender.
The multinomial logit model performance estimation indicates that, the survey dataset is appropriate for this travel pattern, particularly when the findings align with Freetown’s traditional modal split. If comparable survey datasets are available for other regions of the country, they could also be suitable for the development of similar models. However, as compared to the contemporary modal split of Freetown, there was an observed decline in terms of students’ patronage for taxis and buses. Although this comparison may not be entirely representative, given that Freetown’s modal split accounts for different trip purposes, it does shed light on the limited number of student buses and taxis serving the three campuses. Despite its low utilization, if student buses are made available and affordable for students at the three campuses, the decline in taxi usage will be beneficial since the number of cars on the road network will be reduced, resulting in less traffic congestion. In most cities, universities frequently strive to build sustainable campuses for their students because they are important trip destinations. Students’ selection of modes of transport influences both the general sustainability of urban transportation and the sustainability of campus growth. Therefore, reducing the use of private vehicles to encourage students to switch to more efficient and sustainable public transport or non-motorized modes of transportation should be a top priority for university administrators. In this study, private car use was not alarming as only about 1% of students drive to campus. A set of potential policies that will improve a sustainable campus experience should be designed in light of the estimation results. Assessment of these policies should take into account the sociodemographic, characteristics of the current public transportation system, and the infrastructure, which does not offer sufficient facilities like bus stops, shelters, or active transport infrastructure such as bike lanes, walkways, or crosswalks. The majority of public transportation services in the country are offered by private vehicle owners, and active travel is not supported by the current city structure. Due to the unique topography of FBC, provision of the infrastructure for active travel to campus will be difficult to execute and will come at a significant expense. Additionally, difficulties in navigating the campus’s terrain and its narrow streets will make it nearly impossible to implement dedicated cycle paths and bus lanes. Given their locations, IPAM and COMAHS, the other two campuses, might benefit from the implementation of dedicated bus lanes and cycle paths. Furthermore, because the majority of student-serving public transportation vehicles are privately owned and run, it is difficult to completely regulate their operations as these vehicles rarely adhere to set routes and schedules. In addition, there are enforcement-related concerns to deal with. In light of these constraints, it is recommended to provide public transportation suitable for each campus, such as student buses, shared taxis or shuttles. Students should be able to receive upscale service from these vehicles. These rules may be simple to administer and put into effect. Provision of student residences that will shorten commutes for better mobility should also be prioritized. This will guarantee long-term land management that will incorporate the sociocultural, ecological, and economic aspects of campus expansion. Among the contributions of this research are the modal preference of public university students and the identification of factors and measurement of their impact on university trips in Freetown.
Limitations and future research direction
This paper’s findings are limited to trips made by public university students in Freetown. Another limitation is that, students had difficulties recalling the average monthly income of their families. Consequently, discrepancies could exist between the data they submitted and their household’s actual financial status. This may have rendered the variable statistically irrelevant to the choice of mode made by the students. It is appropriate for future research to consolidate information from survey participants with national household demographic data, where available. Also, the model, fared poorly in predicting those who patronize the services of three-wheelers – keke (7.1%). The misclassification results imply that it cannot differentiate between podapoda and keke modes, such that; many observations under these two modes are mutually misclassified. This affirms that the two modes, which share only travel characteristics, have a higher level of homogeneity among the explanatory variables than other modes. The podapoda mode accounts for a considerable portion of the misclassified observations of the keke mode, implying some unobserved comparable preferences across students utilizing these modes. Inadequate observations and the method of random selection of the dataset may have also affected the prediction. Future research should look into sampling more university students (both private and public) in other regions of the country in order to address issues of sampling inadequacies which may have affected the model’s predicting power.
Ethical approval
The study was conducted in line with the procedures and requirements of the Committee on Human Research, Publication & Ethics of the Kwame Nkrumah University of Science and Technology, Kumasi, Ghana with certificate number CHRPE/AP/432/21.
Informed consent Declaration
Informed consent was received from all participants involved in the survey.
Acknowledgments
Many thanks to the Regional Transport Research and Education Centre Kumasi (TRECK) under the Department of Civil Engineering, Kwame Nkrumah University of Science and Technology (KNUST), Kumasi – Ghana for the funding support. Sincere appreciation to the authorities of the University of Sierra Leone especially Professor Kelleh-Gbawuru Mansaray, Deputy Vice Chancellor, Fourah Bay College and Rev. Ing. Obafemi B.A Davies, senior lecturer, Faculty of Engineering and Architecture, University of Sierra Leone for their continued support and mentorship. We also recognize the effort of the field enumerators throughout the data collection process.
Disclosure statement
No potential conflict of interest was reported by the author(s).
Data availability statement
The data presented in this study are available on request from the corresponding author.
Additional information
Funding
References
- Adbelhamid, M. M., Elfakharany, M. M., & Elfakharany, A. M. (2018, October 19–21). Reducing private cars dependency to achieve sustainable urban environment in congested cities. In 7th International Conference on Modern Research In Civil Engineering, Architectural & Urban Development, Munich, Germany (pp. 1–27).
- Aho, K., Derryberry, D., & Peterson, T. (2014). Model selection for ecologists: The worldviews of AIC and BIC. Ecology, 95(3), 631–636. https://doi.org/10.1890/13-1452.1
- Akar, G., Fischer, N., & Namgung, M. (2013). Bicycling choice and gender case study: The Ohio State University. International Journal of Sustainable Transportation, 7(5), 347–365. https://doi.org/10.1080/15568318.2012.673694
- Atasoy, B., Glerum, A., & Bierlaire, M. (2013). Attitudes towards mode choice in Switzerland. DisP - the Planning Review, 49(2), 101–117. https://doi.org/10.1080/02513625.2013.827518
- Babey, S. H., Hastert, T. A., Huang, W., & Brown, E. R. (2009). Sociodemographic, family, and environmental factors associated with active commuting to school among US adolescents. Journal of Public Health Policy, 30(S1), S203–S220. https://doi.org/10.1057/jphp.2008.61
- Banister, D., Cornet, Y., Givoni, M., & Lyons, G. (2019). Reasonable travel time–the traveller’s perspective. A Companion to Transport, space and equity, 197. Cheltenham, UK and Northampton, USA: Edward Elgar Publishing Limited.
- Basbas, S., Campisi, T., Georgiadis, G., Al-Rashid, M. A., & Tesoriere, G. (2021). COVID-19 and public transport demand trends in Sicily: Analyzing external factors and governmental recommendations. European Transport/Trasporti Europei, (83), 1–15. https://doi.org/10.48295/ET.2021.83.9
- Ben-Aakiva, M., & Lerman, S. R. (1985). discrete choice analysis: theory and application to travel demand.
- Bonham, J., & Koth, B. (2010). Universities and the cycling culture. Transportation Research, Part D: Transport & Environment, 15(2), 94–102. https://doi.org/10.1016/j.trd.2009.09.006
- Bray, D., & Holyoak, N. (2015). Motorcycles in developing Asian cities: A case study of Hanoi. In 37th Australasian Transport Research Forum, Unpublished Conference Paper, Sydney, Australia.
- Campisi, T., Torrisi, V., Ignaccolo, M., Inturri, G., & Tesoriere, G. (2020). University propensity assessment to car sharing services using mixed survey data: The Italian case study of enna city. Transportation Research Procedia, 47, 433–440. https://doi.org/10.1016/j.trpro.2020.03.155
- Census. (2015). Sierra Leone 2015 Population and Housing Census national analytical report.
- Chen, X. (2012). Statistical and activity-based modeling of university student travel behavior. Transportation Planning and Technology, 35(5), 591–610. https://doi.org/10.1080/03081060.2012.701818
- Cochran, W. G. (1977). Sampling techniques (3rd ed.). John Wiley & Sons, Inc.
- Costanzo, C. M., Halperin, W. C., Gale, N. D., & Richardson, G. D. (1982). An alternative method for assessing goodness-of-fit for logit models. Environment & Planning A: Economy & Space, 14(7), 963–971. https://doi.org/10.1068/a140963
- Danaf, M., Abou-Zeid, M., & Kaysi, I. (2014a). Modeling travel choices of students at a private, urban university: Insights and policy implications. Case Studies on Transport Policy, 2(3), 142–152. https://doi.org/10.1016/j.cstp.2014.08.006
- Danaf, M., Abou-Zeid, M., & Kaysi, I. (2014b). Modeling travel choices of students at a private, urban university: Insights and policy implications. Case Studies on Transport Policy, 2(3), 142–152. https://doi.org/10.1016/j.cstp.2014.08.006
- Das, R., Kumar, S. V., Prakash, B., & Subbarao, S. S. V. (2016). Analysis of university students travel behaviour: En route to sustainable campus. Indian Journal of Science & Technology, 9(30), 30. https://doi.org/10.17485/ijst/2016/v9i30/99246
- De Guzman, M. P., & Diaz, C. E. (2005). Analysis of mode choice behavior of students in exclusive schools in metro Manila: The case of Ateneo de Manila University & Miriam College. In Proceedings of the Eastern Asia Society for Transportation Studies. Citeseer, 5, 1116–1131.
- De Ortúzar, J. D., & Willumsen, L. G. (2002). Modelling transport. John Wiley & Sons. West Sussex.
- Dias, C., Abdullah, M., Lovreglio, R., Sachchithanantham, S., Rekatheeban, M., & Sathyaprasad, I. M. S. (2022). Exploring home-to-school trip mode choices in Kandy, Sri Lanka. Journal of Transport Geography, 99, 103279. https://doi.org/10.1016/j.jtrangeo.2022.103279
- Etminani-Ghasrodashti, R., Paydar, M., & Hamidi, S. (2018). University-related travel behavior: Young adults’ decision-making in Iran. Sustainable Cities and Society, 43, 495–508. https://doi.org/10.1016/j.scs.2018.09.011
- Ewing, R., Schroeer, W., & Greene, W. (2004). School location and student travel analysis of factors affecting mode choice. Transportation Research Record: Journal of the Transportation Research Board, 1895(1), 55–63. https://doi.org/10.3141/1895-08
- Faghih-Imani, A., Hampshire, R., Marla, L., & Eluru, N. (2017). An empirical analysis of bike sharing usage and rebalancing: Evidence from Barcelona and Seville. Transportation Research Part A: Policy and Practice, 97, 177–191. https://doi.org/10.1016/j.tra.2016.12.007
- Ferreira, M. C., Costa, P. D., Abrantes, D., Hora, J., Felício, S., Coimbra, M., & Dias, T. G. (2022). Identifying the determinants and understanding their effect on the perception of safety, security, and comfort by pedestrians and cyclists: A systematic review. Transportation Research: Part F, Traffic Psychology and Behaviour, 91, 136–163. https://doi.org/10.1016/j.trf.2022.10.004
- Golshani, N., Shabanpour, R., Mahmoudifard, S. M., Derrible, S., & Mohammadian, A. (2018). Modeling travel mode and timing decisions: Comparison of artificial neural networks and copula-based joint model. Travel Behaviour and Society, 10, 21–32. https://doi.org/10.1016/j.tbs.2017.09.003
- Hasnine, M. S., & Habib, K. N. (2018). What about the dynamics in daily travel mode choices? A dynamic discrete choice approach for tour-based mode choice modelling. (Oxf), 71, 70–80. https://doi.org/10.1016/j.tranpol.2018.07.011
- Hauber, A. B., González, J. M., Groothuis-Oudshoorn, C. G. M., Prior, T., Marshall, D. A., Cunningham, C., IJzerman, M. J., & Bridges, J. F. P. (2016). Statistical methods for the analysis of discrete choice experiments: A report of the ISPOR conjoint analysis good research practices task force. Value in Health, 19(4), 300–315. https://doi.org/10.1016/j.jval.2016.04.004
- Heinen, E., Van Wee, B., & Maat, K. (2010). Commuting by bicycle: An overview of the literature. Transport Reviews, 30(1), 59–96. https://doi.org/10.1080/01441640903187001
- Henning, E., Ferreira Schubert, T., & Ceccatto Maciel, A. (2020). Modelling of University Student Transport Mode Choice in Joinville: A binary logistic Model for active modes. Journal of Sustainable Development of Energy, Water and Environment Systems, 8(4), 678–691. https://doi.org/10.13044/j.sdewes.d7.0303
- Hoang, Q., & Okamura, T. (2020). Analyzing behavioral intentions in new residential developments of motorcycle dependent cities: The case of Ho Chi Minh City. Case Studies on Transport Policy, 8(1), 163–172. https://doi.org/10.1016/j.cstp.2018.09.007
- Kelly, J. A., & Fu, M. (2014). Sustainable school commuting – understanding choices and identifying opportunities. Journal of Transport Geography, 34, 221–230. https://doi.org/10.1016/j.jtrangeo.2013.12.010
- Khattak, A., Wang, X., Son, S., & Agnello, P. (2011). Travel by University Students in Virginia. Transportation Research Record: Journal of the Transportation Research Board, 2255(1), 137–145. https://doi.org/10.3141/2255-15
- Kotoula, K. M., Sialdas, A., Botzoris, G., Chaniotakis, E., & Grau, J. M. S. (2018). Exploring the effects of university campus decentralization to students’ mode choice. Periodica Polytechnica Transportation Engineering, 46, 207–214. https://doi.org/10.3311/PPtr.11641
- Kudebong, M., Wurapa, F., Nonvignon, J., Norman, I., Awoonor-Williams, J. K., & Aikins, M. (2011). Economic burden of motorcycle accidents in Northern Ghana. Ghana Medical Journal, 45(4), 135–142.
- Lagani, V., Kortas, G., & Tsamardinos, I. (2013). Biomarker signature identification in “omics” data with multi-class outcome. Computational and Structural Biotechnology Journal, 6(7), e201303004. https://doi.org/10.5936/csbj.201303004
- Lin, L.-C., Huang, P.-H., & Weng, L.-J. (2017). Selecting path models in SEM: A comparison of model selection criteria. Structural Equation Modeling: A Multidisciplinary Journal, 24(6), 855–869. https://doi.org/10.1080/10705511.2017.1363652
- Lundberg, B., & Weber, J. (2014). Non-motorized transport and university populations: An analysis of connectivity and network perceptions. Journal of Transport Geography, 39, 165–178. https://doi.org/10.1016/j.jtrangeo.2014.07.002
- Mackett, R. L., & Ahern, A. (2000). Centre for Transport Studies University College London.
- Mahlawat, M., Rayan, S., Kuchangi, S., Patil, S., & Burris, M. W. (2007). Examination of student travel mode choice.
- Malhotra, N. K. (2007). Marketing Research: An Applied Orientation. (pp. 134–171, 4th ed). Pearson Education.
- Mammen, G., Stone, M. R., Buliung, R., & Faulkner, G. (2014). School travel planning in Canada: Identifying child, family, and school-level characteristics associated with travel mode shift from driving to active school travel. Journal of Transport & Health, 1(4), 288–294. https://doi.org/10.1016/j.jth.2014.09.004
- Martin, S. L., Lee, S. M., & Lowry, R. (2007). National prevalence and correlates of walking and bicycling to school. American Journal of Preventive Medicine, 33(2), 98–105. https://doi.org/10.1016/j.amepre.2007.04.024
- McDonald, N. C. (2008). Critical factors for active transportation to school among low-income and minority students: Evidence from the 2001 national household travel survey. American Journal of Preventive Medicine, 34(4), 341–344. https://doi.org/10.1016/j.amepre.2008.01.004
- McDonald, N. C., Brown, A. L., Marchetti, L. M., & Pedroso, M. S. (2011). U.S. School travel, 2009. American Journal of Preventive Medicine, 41(2), 146–151. https://doi.org/10.1016/j.amepre.2011.04.006
- McMillan, T. E. (2002). The influence of urban form on a child’s trip to school. In Association of Collegiate Schools of Planning Annual Conference, Baltimore.
- McMillan, T., Day, K., Boarnet, M., Alfonzo, M., & Anderson, C. (2006). Johnny Walks to school—does Jane? Sex differences in Children’s Active Travel to school. Children, Youth and Environments, 16(1), 75–89. https://doi.org/10.1353/cye.2006.0038
- MoHS. (2021). Sierra Leone COVID-19 Emergency Preparedness and response project. Ministry of Health and Sanitation (MoHS), Government of Sierra Leone (GoSL).
- Nasrin, S. (2020). Private University Students’ Mode Choice Behaviour for Travel to University: Analysis in the context of Dhaka City. In T. V. Mathew, G. J. Joshi, N. R. Velaga, & S. Arkatkar (Eds.), Transportation Research (pp. 299–310). Springer Singapore.
- Nguyen-Phuoc, D. Q., Amoh-Gyimah, R., Tran, A. T. P., & Phan, C. T. (2018a). Mode choice among university students to school in Danang, Vietnam. Travel Behaviour and Society, 13, 1–10. https://doi.org/10.1016/j.tbs.2018.05.003
- Nguyen-Phuoc, D. Q., Amoh-Gyimah, R., Tran, A. T. P., & Phan, C. T. (2018b). Mode choice among university students to school in Danang, Vietnam. Travel Behaviour and Society, 13, 1–10. https://doi.org/10.1016/j.tbs.2018.05.003
- Nkegbe, P. K. (2012). Choice of transport mode by non-resident university students in Ghana. International Journal of Business and Social Science, 3(20), 136–142.
- Randy Aikins, E. (2023). Forecasting the future of Africa.
- Rodriguez-Valencia, A., Rosas-Satizabal, D., & Paris, D. (2019). Importance-performance analysis in public transportation: Methodological revision for practical implementation. Transportation Research Record: Journal of the Transportation Research Board, 2673(2), 710–723. https://doi.org/10.1177/0361198118825125
- Saitluanga, B. L., & Hmangaihzela, L. (2022). Transport mode choice among off-campus students in a hilly environment: The case of Aizawl, India. Transport Problems, 17(3), 179–188. https://doi.org/10.20858/tp.2022.17.3.14
- Samuel, J., Ali, G. G. M. N., Rahman, M. M., Esawi, E., & Samuel, Y. (2020). Covid-19 public sentiment insights and machine learning for tweets classification. Information, 11(6), 314. https://doi.org/10.3390/info11060314
- Santos, G., Maoh, H., Potoglou, D., & von Brunn, T. (2013). Factors influencing modal split of commuting journeys in medium-size European cities. Journal of Transport Geography, 30, 127–137. https://doi.org/10.1016/j.jtrangeo.2013.04.005
- Simons, D., De Bourdeaudhuij, I., Clarys, P., De Geus, B., Vandelanotte, C., Van Cauwenberg, J., & Deforche, B. (2017). Choice of transport mode in emerging adulthood: Differences between secondary school students, studying young adults and working young adults and relations with gender, SES and living environment. Transportation Research Part A: Policy and Practice, 103, 172–184. https://doi.org/10.1016/j.tra.2017.05.016
- Song, Y., Preston, J. M., & Brand, C. (2013). What explains active travel behaviour? Evidence from case studies in the UK. Environment & Planning A: Economy & Space, 45(12), 2980–2998. https://doi.org/10.1068/a4669
- Srinivasan, K. K., Pradhan, G. N., & Naidu, G. M. (2007). Commute mode choice in a developing country: Role of subjective factors and variations in responsiveness across captive, semicaptive, and choice segments. Transportation Research Record: Journal of the Transportation Research Board, 2038(1), 53–61. https://doi.org/10.3141/2038-07
- Taherdoost, H. (2019). What is the best response scale for survey and questionnaire design; review of different lengths of rating scale/attitude scale/Likert scale. International Journal of Academic Research in Management (IJARM), 8(1), 1–10.
- Ton, D., Duives, D. C., Cats, O., Hoogendoorn-Lanser, S., & Hoogendoorn, S. P. (2019). Cycling or walking? Determinants of mode choice in the Netherlands. Transportation Research Part A: Policy and Practice, 123, 7–23. https://doi.org/10.1016/j.tra.2018.08.023
- Tsolakis, D., Shackleton, J., & Makwasha, T. (2011). Small travel time savings: Treatment in project evaluations. Austroads Publication No. AP–R392-11.
- Unctad. (2023). The Least Developed Countries Report 2023.
- Wadud, Z., & Huda, F. Y. (2019). Fully automated vehicles: The use of travel time and its association with intention to use. In Proceedings of the Institution of Civil Engineers-Transport (pp. 127–141). Thomas Telford Ltd. https://doi.org/10.1680/jtran.18.00134
- Whalen, K. E., Páez, A., & Carrasco, J. A. (2013). Mode choice of university students commuting to school and the role of active travel. Journal of Transport Geography, 31, 132–142. https://doi.org/10.1016/j.jtrangeo.2013.06.008
- Yeung, J., Wearing, S., & Hills, A. P. (2008). Child transport practices and perceived barriers in active commuting to school. Transportation Research Part A: Policy and Practice, 42(6), 895–900. https://doi.org/10.1016/j.tra.2007.12.007
- Yin, M., Zeng, D., Gao, J., Wu, Z., & Xie, S. (2018). Robust multinomial logistic regression based on RPCA. IEEE Journal of Selected Topics in Signal Processing, 12(6), 1144–1154. https://doi.org/10.1109/JSTSP.2018.2872460
- Yu, Y. (2018). Understanding university students’ intercity transport mode choice: a case study of the university of waterloo students’ travel behavior between the region of waterloo and the greater Toronto and Hamilton area.
- Zhou, J., Wang, Y., & Wu, J. (2018). Mode choice of commuter students in a college town: An exploratory study from the United States. Sustainability (Switzerland), 10(9). https://doi.org/10.3390/su10093316