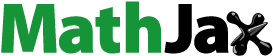
ABSTRACT
Bike-sharing is one of the world’s fastest-growing new modes of transportation, with new schemes appearing on a regular basis. This paper examines the usage trends in a bike-sharing scheme operating in Chandigarh since 2020. While many studies have been conducted on how bike-sharing schemes are changing mobility in cities worldwide, particularly in developed countries, few have examined the dynamics of these schemes in cities in developing countries like India. One of the reasons for looking at a city like Chandigarh is to see if medium and smaller cities in developing countries benefit from bikesharing schemes and if bike-sharing schemes can play a prominent role in these cities. The study conducted an exploratory analysis using app data to examine the impact of factors such as temporal, weather, travel characteristics, and bike type (conventional or E-bike) on bike share usage in the city. Some of the research findings on the impact of various factors on bicycle ride durations (short, medium, and long) can assist other cities in developing countries in planning new bike station networks. Furthermore, analysing mode selection (conventional and E-bike) in bike share provides insights into user preferences and how various influencing factors influence their mode choice behaviour in similar cities.
1. Introduction
Rapid population growth and motorisation have become major issues in developing countries such as India, resulting in numerous transportation challenges such as traffic congestion, road accidents, noise pollution, air pollution, and climate (Benedini et al., Citation2019). India developed the National Urban Transport Policy (NUTP) to counteract these negative externalities. One of the policy’s main goals was to promote sustainable transportation systems in Indian cities. The Ministry of Urban Development (MoUD) has launched several initiatives and programmes to include Non-Motorised Transport (NMT) as an essential component of an integrated urban transportation system. One such initiative was implementing bike-sharing systems to revitalise cycling culture in Indian cities. Bikesharing is a short-term access mode that provides users with an environmentally friendly mode of public transportation (S. A. Shaheen et al., Citation2010). Furthermore, it enables point-to-point active travel for short-distance trips without needing a bicycle (Mateo-Babiano, Citation2015). It also allows people to borrow a bike from one of the system’s ‘docks’ and return it to another dock Sustainable Urban Transport Project (SUTP). Furthermore, implementing bike share in cities provides numerous social, economic, and environmental benefits such as reduced congestion, lower emissions, health benefits, and lower capital transportation investment costs (DeMaio, Citation2009; S. A. Shaheen et al., Citation2010).
Though bike share schemes were first introduced in European countries, due to their success and popularity, these schemes have rapidly expanded to other parts of the world. According to (Meddin, Citation2020), approximately 2008 bike share systems were operating globally at the end of 2020. Many cities in India have recently implemented bike share schemes as part of the ‘Smart Cities Mission’ to promote sustainable transport systems. Further, shows a list of 27 cities operating bikesharing schemes in India. Given the increased popularity of bike sharing, it is crucial to assess how well operations are performing to increase the efficiency of the current bike-sharing programmes (Fishman et al., Citation2013). Furthermore, understanding the impact of various factors such as user behaviour, built environment, and topography on bike-share usage will aid in the operations of newly installed or modifying existing systems. While bike sharing has become popular in Western countries, it is still in its infancy in Asia, particularly in developing countries such as India. Moreover, little research has been conducted on bike share scheme operations in developing countries. Furthermore, a direct adaptation of western bike-share operation models may not yield adequate results in developing countries, particularly in the Asian region. Compared to developed countries, they confront different urban challenges, such as diverse land uses, relatively dense urban centres, traffic safety, road infrastructure, and law enforcement.
Table 1. List of cities with bike sharing schemes in India source: (primary survey by Author).
Given the scarcity of literature on bike share use outside of developed countries, this study examines bike share use in Chandigarh in the context of small and medium-sized cities in India to guide newly developed bike schemes. Further, most bike-sharing schemes in these cities are of a similar scale to Chandigarh and have similar geographical conditions. The study focuses on how weather conditions, the types of bikes available in the schemes, temporal changes such as the length of the week, and the time of day, and travel characteristics such as ride duration, OD patterns, and frequency influence the use of bike-share schemes in these cities.
The remainder of this paper is organised as follows. In Section 2, we present the extensive previous literature that forms the background of this study. Section 3 describes the data collection development and examines trends in bike share usage data. Further, Section 4 discusses the study’s methods and results, followed by conclusions in the final section.
2. Literature review
In Western countries, there has been a significant amount of research on bike-share topics such as user characteristics and preferences, business and operational models, network distribution and optimization techniques, the impact of bike-share on other modes of transportation and the environment, and evaluation of bike-share performance and usage. However, the article mainly focuses on usage patterns of bike share schemes in developing countries like India. Therefore, the article mainly discusses some existing literature on bike share usage patterns and the factors affecting them. (Gauthier et al., Citation2014; Goodman & Cheshire, Citation2014; O’Brien et al., Citation2014) investigated the impact of bike share attributes such as system size, membership, type of bike available, and location on bike share usage patterns. Furthermore (Fishman et al., Citation2013, Citation2015; Gauthier et al., Citation2014; O’Brien et al., Citation2014), examined the effect of the density and compactness of the station network on bike share usage patterns. Additionally, bicycle infrastructures such as bike lanes, tracks, and pathways influence bike share usage 13 (Castillo-Manzano & Sánchez-Braza, Citation2013; Mateo-Babiano et al., Citation2016). The usage of PBS Systems is also influenced by weather conditions such as temperature, humidity, wind speed, and rainfall. According to some studies, high humidity, rain, snow, and extreme temperature conditions result in low usage (Corcoran et al., Citation2014; Faghih-Imani et al., Citation2017; Hampshire & Marla, Citation2012). Further (Caulfield et al., Citation2017; Zhao et al., Citation2015), discussed the impact of spatial and temporal factors such as built environment (land use and Point of Interest), seasonal changes, and time of day and day of the week on bike share usage. Moreover (Caulfield et al., Citation2017; Faghih-Imani et al., Citation2017; Fishman et al., Citation2015; Hampshire & Marla, Citation2012), analyzed the user socio-demographic and travel preferences on bike share usage. However, most of these studies focused on developed countries. Very little research has been conducted in developing countries to investigate how these factors influence bike share usage.
Mainly in India, though bike-sharing was first introduced in 2008, little research has been conducted on various aspects of bike-share schemes. (Dhingra et al., Citation2010) conducted a study that examined the operations of various bike share schemes in Indian cities and proposed planning, financing, marketing, and promotion strategies. Furthermore (Patel & Patel, Citation2020), discussed the planning and design of bike-sharing systems in Indian cities using Surat as a case study to overcome challenges such as parking, congestion, and dense population growth. Furthermore, the study by (Patel & Patel, Citation2020) discussed the barriers to implement public bikesharing systems in Indian cities through stakeholders’ responses. In addition (Sharmeen et al., Citation2021), proposed a conceptual framework to understand bike-share stakeholder interactions in Kolkata using a case study. In addition (Machavarapu et al., Citation2022a), examined the impact of available bike-share infrastructure on the performance and usage of bike-share schemes in several Indian cities. Further (Machavarapu et al., Citation2022b), evaluated Delhi’s various bike share schemes operations and performance. Additionally, using structural equation modelling to analyse user survey data, Machavarapu et al. (Citation2023) investigated the variables influencing bike sharing users’ intents to use the service in Indian cities (SEM). A university campus bike sharing programme was conceived, designed, and analysed by Verma et al. (Citation2020) in an effort to lessen vehicle traffic and foster a more sustainable, safe environment. They looked into bike usage patterns using early usage data, highlighting data constraints and suggesting mode share modelling and the collection of demographic data on college campuses. However, most of these studies were conducted through expert surveys. None of the studies have looked at how the current cycling infrastructure, geographic location, climate, social economics, demographics, and policies affect real bike-share usage, whether through data mining from web sources or acquiring app-based trip data from the bike-sharing operator. These factors are important for planning and implementing system expansions or improvements, designing and adopting new systems, and managing operations and maintenance. Analysis of the database, for example, can disclose information on changes in bikesharing demand, the best locations for bike stations, and the required capacity of a bicycle station. It also gives information on travel patterns. To address some of these gaps, the study used the bikeshare app database to analyse how different factors affect bike-share usage in Indian conditions.
3. Case study profile – Chandigarh
The study area adopted in this paper is Chandigarh, the capital city of Punjab and Haryana states, India. Chandigarh is an important Tier 2 city in India, with a population of approximately 1.053 million people (per the 2011 census). Chandigarh city is located in the foothills of the Shivalik Range, which adds to its scenic environment. The city is distinguished by its flat, undulating topography and several natural attractions, notably Sukhna Lake. The planned metropolis is separated into sectors, each with a particular role. Chandigarh has a humid subtropical climate. Summers are hot, with temperatures exceeding 40°C (104°F), and winters are cold, with temperatures falling to around 5°C (41°F). Monsoons produce moderate to heavy rains in July and August. Chandigarh’s transportation system includes a large bus network maintained by the CTU, as well as auto and cycle rickshaws. Private vehicles, such as cars and motorbikes, are commonly used. The city encourages non-motorized transportation through bike tracks and pedestrian-friendly zones. Initiatives to implement a metro system are under consideration. As a part of promoting Non motorised transportation in the city administration launched Chandigarh’s public bike share system in December 2020 as part of the smart city project. The concessionaire Smart Bike Tech Pvt. Ltd., Hyderabad, has implemented the project on a Public-Private Partnership (PPP) basis for 10 years. The scheme covers the city servicing all the main trip attractors, including bus and rail stations, colleges, schools, parks and other tourist spots. Further, the spatial distribution of the bike share station in the city is shown in . Currently, the system operates with a fleet size of 1250 bicycles and 155 fixed docking stations and uses a Mobile app for booking and payment. It provides 24–7 bicycle-sharing services to the users in the city. The program provides users with two types of bikes: traditional pedal bikes and electric bikes, aiming to improve citizen health and provide a faster commuting service. To avail of the service, Users of this bicycle-sharing program pay Rs 10 (USD 0.12) for the first 30 minutes and the rest of the 30 Minutes, at the total price of Rs 15 (USD 0.18) per 30 Minutes.
3.1. Data
Smart Bike Mobility Pvt Ltd, the city’s bike-share operator, provided the data for the study. The data represents the entire operation of the bike-share scheme from 14 October 2021, to 31 October 2021. The original data set included around 28,000 trip records. Furthermore, during the data cleaning process, trips with ride durations of less than 2 minutes were removed, as they were assumed to be trips in which bikes were simply taken from the dock and returned without performing a ride. Moreover, the data set with ride duration above 300 minutes were also removed, as they were assumed to be trips in which bikes were not checked out properly while returning the bicycles at checkout stations. As a result, the 6% trip record was removed. Since the data used in the study was anonymous, the operator did not provide age or gender information. This is identified as one research limitation in understanding user socio-demographic profiles. However, the findings revealed in the following sections provide several exciting findings on the city’s bike-share operations.
3.2. Examining trends in the Bike share usage data
The results presented in this section describe the variables used in the following modelling sections and provide insight into the user behavior of the bike share scheme in the city.
describes the variables used in the Multinomial Logit (MNL) model. The first variable investigated is the vehicle type. The scheme offers two types of bikes: traditional bikes (pedal) and electric bikes. The results show that most trips (72.3 %) under the scheme are performed using E-bikes rather than conventional bikes (27.7 %). Further depict the mode-wise distribution of trips on different days and at different hours of the day. Another aspect of the scheme that was investigated is the travel time of bike trips, where bike trips performed under the scheme are classified into four groups to understand better the nature of the trip, such as very long (>35 Minutes), long (35 Minutes—20 Minutes), medium (9 Minutes—20 Minutes), and short trips (<9 Minutes). The results show that trip travel time is distributed evenly across the groups. Trips taken on both bikes have a similar travel time distribution, as shown in . However, the distance travelled by these bikes differs because the Electric bike assists the rider in reaching speeds of 25 kmph, which may not be possible on a traditional pedal bike.
Figure 2. Mode wise (conventional bike & E bike) distribution of trips on different days and hours of day.

Table 2. Description of variables used in the MNL model.
The other variables investigated in station usage are the Origin-Destination (OD) Patterns of Trips. According to the findings, only 10.6 % of all trips have the same OD pattern, while the remaining trips have different OD patterns. The final findings examine the time of day, day of week, and weather variables. According to the time-of-day data, 36.5 % of trips occur between 4 and 8 p.m. (Evening Peak). According to the findings on the duration of the week, more than 65 % of trips were recorded during the week and 34.3 % on weekends. also shows a detailed breakdown of the number of trips taken during the week and time of day. Further, the temperature variable was calculated by taking the average temperature in Chandigarh during October and estimating whether the trips occurred on days when the temperature was above or below the city’s minimum and maximum average temperatures. This weather data was obtained from the Meteorological Department of Chandigarh. More trips (66.6 %) took place during intermediate temperatures ranging from 18 to 30 degrees, as intended, rather than extreme maximum and minimum average temperature conditions. Moreover, they are divided into three demand-based categories to examine how busy the scheme’s bike stations are. Stations with more than 30 trips per day are classified as busy, stations with a demand of 30–15 trips per day as moderately busy, and stations with fewer than 15 trips per day as less busy. According to the findings, approximately 68 % of all trips begin at the scheme’s less busy stations.
Figure 4. Distribution of trips day of week (Weekend & Weekday) and hours of day (peak & off peak) an.

This section provides information on the scheme’s usage trends for conventional bikes and E-bikes.
depicts a detailed breakdown of the number of trips made on weekdays and weekends. The scheme’s weekend usage shows a morning and evening peak and consistent usage during the afternoon off-peak period. However, the scheme only observed one peak during the evening on weekday usage. depicts the hourly variations in demand between conventional and electric bike trips during the week and on weekends. Both bikes followed similar hourly demand patterns, but the observations revealed some significant differences. The scheme’s conventional and E-bikes are in higher demand on weekends than on weekday mornings. However, these differences are not observed in the afternoon and evening periods. In the case of conventional bikes, a drop in demand was observed during afternoon periods, whereas E bikes showed constant growth in demand during afternoon periods. This could be due to high afternoon temperatures, which can be more challenging for conventional bike users than E-bike users. also illustrates the differences in average ride durations of bike trips between weekdays and weekends. People use bike schemes for longer durations on weekends than on weekdays, which is understandable given that they have more free time on weekends. also shows the differences in average ride duration between conventional and E-bike trips. It demonstrates that people ride E-bikes for extended periods than traditional bikes. In the case of conventional bikes, the average ride duration of trips is high in the morning, gradually decreases in the afternoon, and peaks in the evening. However, people use Ebikes for longer durations in the afternoon than in the morning and evening.
4. Methods and results
4.1. Modelling approach
According to the literature review, several models, such as discrete choice models, machine learning techniques, spatial models, and time-series models, have been used to analyse bike share usage (Zhang et al., Citation2014). assess the performance of multiple discrete choice models in predicting bike-sharing demand, including Multinomial Logit (MNL), Mixed Logit, and Multinomial Probit (MNP). The results of the study show that the Mixed Logit model outperforms the MNL and MNP models in terms of capturing heterogeneity in user preferences and improving demand projections. Similarly (Schnieder, Citation2023), used Random Forests, a machine learning model, to analyse bike sharing demand by adding spatial context and station attributes. The results show that the Random Forests model makes good predictions and sheds light on the significance of geographical elements and station features in bike sharing utilisation. Furthermore (Qu et al., Citation2018), used a spatial regression model to examine the factors that influence bike-sharing demand in New York City. The study discovered strong spatial impacts and indicates the impact of variables including population density, transit accessibility, and land use on bike share usage patterns. (Widhalm et al., Citation2019) examined bike sharing utilisation patterns using data mining approaches such as decision trees and association rules. The findings revealed intriguing usage patterns, such as trip duration distributions and connections between bike sharing utilisation and variables like time of day and weather conditions. Each of these models has distinct strengths and insights that enable researchers to acquire a thorough understanding of bike sharing behaviour and make informed judgments about system planning and management. Machine learning algorithms, such as Random Forests, give accurate demand projections and forecasting. The current study, on the other hand, was primarily concerned with determining the interrelationships between various factors influencing bike share usage. Moreover, the study has only considered limited data set to work with it due to difficulties in accessing data. As a result, the current study evaluated the dataset’s correlations and trends using a Multinomial logistic regression model. When compared to other models, these models can handle restricted data sets well and provide appropriate interpretation. In addition, to capture the correlations between these variables and bike share usage decisions, the current study employed categorical variables such as trip characteristics and weather conditions, which are well handled by MNL models. The model used trip time as a dependent variable against several independent variables in the Multi nominal regression analysis. The model also employed the functional form described below.
where P is the probability that the event occurs. In this case, it is the probability that bicycle trip travel time falls into one of the quartiles examined. b1x1 is the set of trip characteristics (Vehicle type, time of the day, and day of the week). b2x2 represents the set of bike station characteristics (bike station usage, trip OD pattern, and frequency of origin and destination pair), and e represents a random error term.
4.2. Results of the multiple logistic regression and binary logistic regression models
The Multiple logistic regression model results are shown in . The model examines the factors that impact ride duration. The Nagelkerke R2 of 0.622 indicates a good model fit. Further, the model provides several discernments into the usage of bike-share systems in medium-sized cities in India. The first set of results shows that most conventional bike trips are likely to perform for longer durations than E-bike trips. This suggests that E bikes are faster in speed compared to conventional bikes, so E-bike users finish their trip comparatively early than conventional bike users to reach their destination. Further, the results of the duration of the week show that longer trips are likely to occur on weekends.
Table 3. Multinomial logistic regression model result for bike share ride duration.
The third set of variables examined the impact of the time of day travelled on trip duration. The findings show that longer-duration trips are performed in the morning peak period, and shorter trips are performed in the afternoon off-peak period. This suggests that in the morning, people prefer to ride bicycles for long durations for health and fitness purposes compared to the afternoon. Moreover, the rise in temperature during afternoon periods in Indian cities does not favour riding a bicycle for long durations. However, in the evening peak period, not many variations in the coefficient values are observed between trip duration groups, showing that a mix of shorter and longer duration trips are performed in the evening period. The next set of results related to the bike station shows that the shortest trips (less than 9 min) are likely to be round trips from the same bike station, with longer-duration trips likely to have a different OD. Further, the results for temperature show that shorter trips are more likely to perform on warm days. The final set of variables examines the impact of the usage of bike stations on trip duration. The findings show that shorter trips are more likely to occur at less busy stations than busy stations. This suggests that most busy stations are located in activity areas such as parks, tourist spots and markets, where people are likely to spend more time using bicycles compared to low-activity areas.
Further binary logistic regression analysis was performed to examine the factor’s impact on mode choice (Conventional Bike or E-bike) in the Bike share scheme. The results of the binary regression model are shown in . The Nagelkerke R2 of 0.563 indicates a good model fit. The model provides important findings on using conventional and E-bikes in bike share in Indian cities. The first set of results shows that people are more likely to use conventional bikes for longer durations than E-bikes, as discussed earlier. The next set of variables examined the impact of time of day on the mode selection in the bike share scheme. The findings show that people prefer to use the E-bike compared to the Conventional bike during morning and afternoon periods. This suggests that, in general, people in the city are more attracted to e-bikes than Conventional bikes due to their superiority in terms of convenience, comfort and speed. However, in the case of Conventional bike usage, the number of trips performed drastically decreases in the afternoon compared to the morning period of the day and vice versa. This may be because people prefer riding conventional bikes during morning durations as health/fitness trips. Further, subcontinental conditions in Indian cities, where temperatures are high in afternoon periods, do not favour peddling a conventional bike. As a result, people prefer to use E-bike in the afternoon periods. Further, the weekly duration results show that conventional bike and E-bike trips are higher on weekends than on weekdays. The fourth set of variables examined the impact of OD patterns on mode selection. The findings observed more different OD patterns using conventional bikes than E-bikes. Further, the temperature results on mode selection in bike share show that people prefer to use conventional bikes on days when the average temperatures are low. The average temperature increase shows a decreasing trend in conventional bike usage and an increasing trend in E-bike usage. The final set of variables examines the impact of the usage of bike stations on mode selection. The findings show that people prefer to use Conventional bikes at less busy stations than busy stations. This is understandable, as most of the busy stations are located at major activity centers in the city, where the traffic is high. From a safety point of view, people prefer to use conventional bikes at less busy stations to avoid collisions with traffic. However, the usage of E-bike is observed to be high at busy stations. This may be because E-bike provides more flexibility than other transport modes to beat traffic and reach destinations.
Table 4. Binary logistic regression model result for (bicycle type choice) in bike share.
5. Conclusions
As discussed in this paper, bike-sharing is one of the fastest-growing modes of shared mobility globally and is changing attitudes to cycling and shared transport systems. This paper details one of India’s most recently launched bike-sharing schemes in Chandigarh. The results show that even though Chandigarh is a city without a strong cycling culture, the scheme is being used frequently.
This paper mainly focused on usage patterns in the bike-sharing scheme. According to the findings, most users in the city preferred to use E-bikes compared to Conventional bikes. Further, temporal factors such as time of the day and day of the week were observed to significantly impact travel time and selection of mode (E-bike or Conventional bike) in the bike share scheme. Additionally, it was found that the weather affected bike share usage. During good weather, the number of trips and travel time were higher. However, these weather conditions significantly impacted traditional bike usage more than E-bikes. Furthermore, it was discovered that people prefer to use conventional bikes at less busy stations and E-bikes at busier stations, implying that when choosing a mode in a bike share scheme, users are more concerned with safety and traffic conditions.
When the findings of this study are compared to other schemes in developed countries, several similarities and dissimilarities appear. AM and PM peaks in bike share usage are observed in cities such as New York, Washington, Zhongshan and Chicago, but this differs significantly from systems in Montreal, and Cork, where the system tends to be most prevalent in the evenings, similar to Chandigarh (Caulfield et al., Citation2017; S. A. Shaheen et al., Citation2012; Zhao et al., Citation2015). Further, the presence of long travel duration trips (49% of trips with >20 Mins travel time) for conventional bikes in the city differs from shorter travel durations (75% of trips with <20 Mins travel time) observed in cities like Hangzhou, Ningbo, Paris, Lyon and Beijing (Gebhart & Noland, Citation2014; Noland et al., Citation2016; Zhao et al., Citation2015). Many factors, such as station network density, availability of bicycling supportive infrastructure, and user characteristics in the city, would affect the travel durations. The study’s findings show that city users prefer to use the conventional bike for health/fitness purposes rather than discretionary purposes, which is also the reason for longer travel durations. The presence of long-duration trips on weekends in the city is also examined in cities like Washington, Zhongshan and Chicago (O’Brien et al., Citation2014; S. A. Shaheen et al., Citation2012; Zhou & Chen, Citation2015). However, the study compared the analysis results to cities in developed countries due to limited literature on bike share in developing countries. Overall, there are many differences in bike share usage between Chandigarh and other developed cities. Furthermore, most bike share schemes in India operate on a smaller scale. More research is needed on these smaller schemes to understand how the dynamics of the schemes differ from those schemes in developed countries.
Overall, the study provides valuable insights into how a bike-sharing scheme works in cities in developing countries like India. The exploratory analysis performed in the study using app-based trip data would aid in understanding the impact of various factors such as temporal, weather, travel characteristics, and bike type (conventional or E-bike) on bike share scheme usage in the city. The findings regarding the impact of various factors on travel durations (short, medium, and long) can assist in planning new bike station networks in other cities. For example, findings on the impact of various factors on travel durations (short, medium, and long) can be useful for infrastructure planning, such as designing new bike station networks and prioritising the creation of supportive infrastructure, such as dedicated bike lanes or paths in potential city locations. Furthermore, the analysis of mode selection (conventional and E-bike) in bike share provides insights into user preferences and the influence of various factors on mode selection in the city. Similarly, the information pertaining to trip demand patterns at various times of day, including morning, afternoon, evening, and night (weekdays and weekends), can help administrators optimise system functions, like redistributing bikes among stations or modifying service levels in response to demand. For instance, if the models show that demand for bikes is highest at some stations at times of the day, managers might designate resources to guarantee that there is a sufficient supply of bikes at those stations during peak hours. However, the current study does not take into account the impact of other significant elements such as land use characteristics, socioeconomic profiles, transportation network, and infrastructural characteristics on bike sharing utilisation, which should be explored in future studies.
Acknowledgments
The authors would like to acknowledge Smart Bike Tech Pvt. Ltd., Hyderabad, for providing access to the Chandigarh city bike share dataset.
Disclosure statement
No potential conflict of interest was reported by the author(s).
References
- Benedini, D. J., Lavieri, P. S., & Strambi, O. (2019). Understanding the use of private and shared bicycles in large emerging cities : The case of BrazSao Pauloil Débora J. Benedini * The University of Texas at Austin.
- Castillo-Manzano, J. I., & Sánchez-Braza, A. (2013). Managing a smart bicycle system when demand outstrips supply: The case of the university community in Seville. Transportation, 40(2), 459–17. https://doi.org/10.1007/s11116-012-9424-7
- Caulfield, B., O’Mahony, M., Brazil, W., & Weldon, P. (2017). Examining usage patterns of a bike-sharing scheme in a medium-sized city. Transportation Research Part A: Policy and Practice, 100, 152–161. https://doi.org/10.1016/j.tra.2017.04.023
- Corcoran, J., Li, T., Rohde, D., Charles-Edwards, E., & Mateo-Babiano, D. (2014). Spatio-temporal patterns of a public bicycle sharing program: The effect of weather and calendar events. Journal of Transport Geography, 41, 292–305. https://doi.org/10.1016/j.jtrangeo.2014.09.003
- DeMaio, P. (2009). Bike-sharing: History, impacts, models of provision, and future. Journal of Public Transportation, 12(4), 41–56. https://doi.org/10.5038/2375-0901.12.4.3
- Dhingra, Kodukula, S., & C. (2010). Public bicycle schemes: Applying the concept in developing cities. GTZ Sustainable Urban Project. Retrieved from. August. 2010. http://www.sutp.org/files/contents/documents/resources/B_TechnicalDocuments/GIZ_SUTP_TD3_Public-Bicycle-Schemes_EN.pdf
- Faghih-Imani, A., Hampshire, R., Marla, L., & Eluru, N. (2017). An empirical analysis of bike-sharing usage and rebalancing: Evidence from Barcelona and Seville. Transportation Research Part A: Policy Practice, 97, 177–191. https://doi.org/10.1016/j.tra.2016.12.007
- Fishman, E., Washington, S., & Haworth, N. (2013). Bike share: A synthesis of the literature. Transport Reviews, 33(2), 148–165. https://doi.org/10.1080/01441647.2013.775612
- Fishman, E., Washington, S., Haworth, N., & Watson, A. (2015). Factors influencing bike-share membership: An analysis of Melbourne and Brisbane. Transportation Research Part A, 71, 17–30. https://doi.org/10.1016/j.tra.2014.10.021
- Gauthier, A., Hughes, C., Kost, C., Li, S., Linke, C., Lotshaw, S., & Treviño, X. (2014). The bike-share planning Guide. 152. Retrieved from https://go.itdp.org/display/live/The+Bike-Share+Planning+Guide
- Gebhart, K., & Noland, R. B. (2014). The impact of weather conditions on bikeshare trips in Washington, DC. Transportation, 41(6), 1205–1225. https://doi.org/10.1007/s11116-014-9540-7
- Goodman, A., & Cheshire, J. (2014). Inequalities in the London bicycle sharing system revisited: Impacts of extending the scheme to poorer areas but then doubling prices. Journal of Transport Geography, 41, 272–279. https://doi.org/10.1016/j.jtrangeo.2014.04.004
- Hampshire, R. C., & Marla, L. (2012). An analysis of bike sharing usage: Explaining trip generation and attraction from observed demand. Transportation Research Board.
- Kumar Machavarapu, P., & Ram, S. (2022a). Infrastructure evaluation, role of public bike sharing systems – global and Indian context. Global and Indian Context, Case Studies on Transport Policy, 10(3), 1841–1854. ISSN 2213-624X. https://doi.org/10.1016/j.cstp.2022.07.013
- Kumar Machavarapu, P., & Ram, S. (2022b). Review on public bike share schemes in large developing cities: A case study of Delhi, India. India, Case Studies on Transport Policy, 10(4), 2075–2091. Pages 20752091, ISSN2213-624X. https://doi.org/10.1016/j.cstp.2022.09.009
- Machavarapu, P. K., Ram, S., & Kant, P. (2023). Factors influencing bike share intentions of users in Indian cities: A structural equation modelling approach. Urban, Planning and Transport Research, 11(1). https://doi.org/10.1080/21650020.2023.2276405
- Mateo-Babiano, I. (2015). Public bicycle sharing in Asian cities. Journal of the Eastern Asia Society for Transportation Studies, 11, 60–74. December 2015 https://doi.org/10.11175/easts.11.60
- Mateo-Babiano, I., Bean, R., Corcoran, J., & Pojani, D. (2016). How does our natural and built environment affect the use of bicycle sharing? transp. Research Part A: Policy Pract, 94, 295–307. https://doi.org/10.1016/j.tra.2016.09.015
- Meddin, R. (2020). https://bikesharingworldmap.com/.
- Noland, R. B., Smart, M. J., & Guo, Z. (2016). Bikeshare trip generation in New York City. Transportation Research Part A: Policy and Practice, 94, 164–181. https://doi.org/10.1016/j.tra.2016.08.030
- O’Brien, O., Cheshire, J., & Batty, M. (2014). Mining bicycle sharing data for generating insights into sustainable transport systems. Journal of Transport Geography, 34, 262–273. https://doi.org/10.1016/j.jtrangeo.2013.06.007
- Patel, S. J., & Patel, C. R. (2020). A stakeholders perspective on improving barriers in implementation of public bicycle sharing system (PBSS). Transportation Research Part A: Policy and Practice, 138(September 2019), 353–366. https://doi.org/10.1016/j.tra.2020.06.007
- Patel, S. J., Patel, C. R., & Joshi, G. J. (2020). Planning of public bicycle (bike) sharing system (PBSS): A case study of Surat City. Transportation Research Procedia, 48, 2251–2261. https://doi.org/10.1016/j.trpro.2020.08.283
- Qu, C., Dong, J., Zhao, P., & Li, Z. (2018). Spatial analysis of factors influencing bike-sharing demand in New York City. Journal of Transport Geography, 71, 291–305.
- Schnieder, M. 2023. Ebike Sharing vs. Bike Sharing: Demand Prediction Using Deep Neural Networks and Random Forests. Sustainability, 15, 13898. https://doi.org/10.3390/su151813898
- Shaheen, S., Cohen, A. P., & Martin, E. W. (2013). Public bike-sharing in North America: Early operator understanding and emerging trends. Transportation Research Record: Journal of the Transportation Research Board, 2387(1), 83–92. https://doi.org/10.3141/2387-10
- Shaheen, S. A., Guzman, S., & Zhang, H. (2010). Bikesharing in Europe, the Americas, and Asia past. Transportation Research Record: Journal of the Transportation Research Board, 2143(1), 159–167. https://doi.org/10.3141/2143-20
- Shaheen, S. A., Martin, E., Cohen, A., & Finson, R. (2012). Public bikesharing in North America: Early Operator and user understanding.
- Sharmeen, F., Ghosh, B., & Mateo-Babiano, I. (2021). Policy, users and discourses: Examples from bikeshare programs in (Kolkata) India and (Manila) Philippines. Journal of Transport Geography, 90, 102898. https://doi.org/10.1016/j.jtrangeo.2020.102898
- Verma, A., Vajjarapu, H., & Thuluthiyil Manoj, M. (2020). Planning and Usage Analysis of Bike Sharing System in a University Campus BT - Recent advances in traffic engineering S. S. Arkatkar, S. Velmurugan, & A. Verma, (Eds.). Springer Singapore.
- Widhalm, P., Friesenbichler, K. S., & Zaffalon, M. (2019). Analysis of bike-sharing usage patterns using data mining techniques. Transportation Research Part C: Emerging Technologies, 106, 393–412.
- Zhang, Y., Hong, S., & Nasri, A. (2014). A comparative study of discrete choice models for predicting bike-sharing demand. Transportation Research Part C: Emerging Technologies, 38, 6–19.
- Zhao, J., Wang, J., & Deng, W. (2015). Exploring bike-sharing travel time and trip chain by gender and day of the week. Transportation Research Part C: Emerging Technologies, 58, 251–264. https://doi.org/10.1016/j.trc.2015.01.030
- Zhou, X., & Chen, Y. (2015). Understanding Spatiotemporal Patterns of Biking Behavior by Analyzing Massive Bike Sharing Data in Chicago. PloS ONE, 10(10), e0137922. https://doi.org/10.1371/journal.pone.0137922