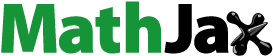
ABSTRACT
Pedestrian safety becoming a serious issue, especially in developing nations, wherein higher crash rates have been reported by the World Health Organization. Despite evidence suggesting higher pedestrian crash counts at signalised intersections in urban areas, there is a lack of in-depth analysis in most developing countries. Motivated by this need, this study aims to: 1) identify significant roadway environment characteristics and traffic volume factors influencing pedestrian – vehicle accidents at signalised intersections in Amman, Jordan, 2) elucidate relationships between pedestrian – vehicle accidents and these factors, and 3) discuss the limitations of pedestrian crash data and propose solutions for future research. We have analysed 166 accidents at 47 signalised intersections in Amman during the period of 2007–2019. The multilevel Generalised Linear Mixed Gamma regression model is the best fit for the data, indicating significant positive correlations between pedestrian crash frequencies and Annual Average Daily Traffic, pedestrian crossing volume, number of lanes, average lane width, and number of parking sides. Conversely, commercial land use and the presence of public transit facilities showed significant negative correlations with pedestrian crashes. This work presents a novel approach that will help developing countries to determine and explain pedestrian crash causes while considering various challenges in these contexts.
1. Introduction
Road accidents have become one of the most prominent transportation problems around the world, making road safety in the transportation sector a major global concern. The World Health Organization (WHO) found that pedestrians represent 23% of all road crash deaths around the world (WHO, Citation2018). Moreover, reported injuries for pedestrians are around twice those for motor vehicle users. At the same time, nobody can ignore the sharp increase of walking as a main mode of transport around the world due to its health and environmental benefits, thus, pedestrians can be considered the most important category of vulnerable road users. Therefore, there is a necessity to analyse pedestrian – vehicle crashes in order to investigate their causes, which helps in finding solutions to avoid or reduce these crashes that will improve pedestrian safety on roads.
According to the WHO, 90% of road traffic fatalities happen in low- and middle-income countries, though these countries have only 48% of the world’s registered vehicles (WHO, Citation2018). Despite this, it has been found that most pedestrian safety estimation studies have been conducted in developed countries, specifically the United States (US), with a small number branching out to developing nations, wherein a larger number of accidents occur. For instance, the pedestrian fatality rate in Jordan is on average about 6.0 and 12.5 times higher than that in the US and Great Britain (GB) respectively (DfT, Citation2020; NHTSA, Citation2020; PSD, Citation2020). This is a worrying trend that should be investigated carefully. That lack of studies in developing countries could be because road safety managers and researchers in developing nations face substantial obstacles. For example, despite the prevalence of pedestrian accidents at signalised intersections, developing pedestrian crash frequency models has been problematic due to a paucity of both traffic and pedestrian exposure data. This widespread scarcity of data has impeded finding the pedestrian crash reasons and assessing pedestrian safety situation. These challenges are shared by developing countries, where poor knowledge available about the relationship between pedestrian accidents, exposure factors, and roadway environment variables at signalised intersections. In addition to that, pedestrian safety research in Jordan is relatively rare because of the limited resources and the absence of funded lead agencies to guide the national road safety effort, which is a similar situation to those in other developing countries.
Many prior studies have indicated that the number of pedestrian trips is larger in urban areas, wherein the frequency of traffic crashes is concurrently higher (Elvik et al., Citation2013; Kraidi & Evdorides, Citation2020). For example, around 81% of pedestrian fatalities in the US occurred in urban areas in 2018 (NHTSA, Citation2020). According to a study conducted by the Federal Highway Administration, around 23.4% of pedestrian deaths from 1998–2007 happened at intersections in the US, with the number of potential pedestrian – vehicle conflicts at intersections being heightened due to mixed traffic in a shared area and a higher exposure rate of pedestrians to crash hazards there (Guo et al., Citation2017; Kraidi & Evdorides, Citation2020; Osama & Sayed, Citation2017; Wang et al., Citation2016). Furthermore, many studies have stated that the number of pedestrian crashes is higher at signalised intersections than at unsignalised ones (Strauss et al., Citation2014; Su & Sze, Citation2022). In Washington, for example, it was found that the pedestrian crash rate at signalised locations was twice that at locations with no traffic control Quistberg et al., (Citation2015).
Therefore, there is a necessity to develop and adopt many strategies and approaches so as to improve pedestrian safety at signalised intersections, specifically in urban areas of developing countries. The most important steps, undoubtedly, are: 1) finding the reasons for pedestrian accidents and 2) understanding the relationships between these reasons and crash frequencies and quantifying their influence on crashes. This means a deep understanding of such reasons and relationships could help in improving road safety, which is done by analysing the pedestrian crash data. In general, statistical analysis of crash data, which are obtained from police reports, is considered to be the most traditional method used to evaluate pedestrian safety around the world. Based on this methodology, many studies have found various contributing factors that significantly affect pedestrian safety, such as road user behaviour, traffic volume variables, and roadway environment characteristics (Al-Omari & Obaidat, Citation2013; Dumbaugh et al., Citation2013; Moradi et al., Citation2016; WHO, Citation2018). These studies indicate that the major contributing factors directly related to crash frequency are road user behaviour (e.g. crossing violations) and traffic volume variables (e.g. pedestrian volume, vehicular volume, speed). However, there is still a lack of sufficient studies that analyse and investigate the effect of roadway environment characteristics (e.g. land use, road width, presence of public transit facilities) on pedestrian safety; therefore, not all pedestrians’ surrounding spaces used for mobility on roads are equally safe. This highlights the need for more research on roadway environment characteristics and their influence on pedestrian safety.
This study aims to provide such an investigation using statistical modelling in an effort to: 1) find the most significant roadway environment characteristics that influence the frequency of pedestrian – vehicle accidents at signalised intersections in Amman, 2) examine how they may affect pedestrian crash frequencies, and 3) address limitations and issues of pedestrian crash data encountered in this study and suggest solutions for future research.
The significance of this study can be summarised in three major points: firstly, the results of this research are expected to help traffic authorities and planners in identifying serious pedestrian safety problems and making decisions regarding pedestrian safety improvement. Secondly, the study will explain and quantify the complex influence of significant roadway environment characteristics on pedestrian crash frequencies. Thirdly, a new vision will be presented for developing countries in dealing with limitations and challenges that usually arise while conducting actual crash studies as well as how to overcome them by mainly employing the suitable statistical techniques such as using the GLMMs in modelling pedestrian crash frequencies.
This study is divided into eight sections. Section 2 provides a literature review. Section 3 outlines the statistical modelling technique used in this study. Section 4 describes the site selection process and the data used in the modelling process. Section 5 clarifies the crash frequency modelling process and discusses the results in detail. Section 6 presents the study limitations, while section 7 displays the conclusions resulting from this study. Finally, section 8 offers recommendations and directions for future work.
2. Literature review
According to the Highway Safety Manual Data Needs Guide (NASEM, Citation2008), roadway environment factors include: 1) a built environment (surrounding public space), 2) a roadway geometric design, and 3) road surface condition factors, which are recommended by this guide to be included in analysis and modelling for any road safety study in addition to traffic volumes. This study is more focused on the environment and roadway geometric design factors in addition to traffic volumes and their vital role as exposure factors in accident risk estimation (Elvik & Bjørnskau, Citation2017; Zhu et al., Citation2022). Therefore, a broad review was conducted of previous studies that have investigated the effects of roadway environment characteristics on pedestrian safety and highlighted their significance. It was found that several studies reported a strong relationship between different roadway environment characteristics and the frequency of pedestrian – vehicle crashes, which could be due to the imbalance between the design and the use of roads Ukkusuri et al., (Citation2012). The following subsections present the most significant roadway environment characteristics which have been found to affect pedestrian crash frequencies, and explain their influence, in addition to traffic volume factors.
2.1 Land use
Many studies have investigated the relationship between land use and the frequency of pedestrian crashes and found a statistically significant relation between them. These studies have incorporated several indicators for land use, such as the presence of specific types of land use, areas of land use, and the proportion of specific land use types in the studied site/zone/location. Ukkusuri et al., (Citation2012), Rankavat & Tiwari, (Citation2015), Lizarazo & Valencia, (Citation2018) and Obinguar & Iryo-Asano, (Citation2021) concluded that commercial land use is significantly associated with more pedestrian crashes in comparison with residential land use, as it witnesses higher pedestrian activities, a matter that results in higher exposure to traffic conflicts with vehicles (Narayanamoorthy et al., Citation2013; Ukkusuri et al., Citation2011). However, Guo et al. (Citation2017) found that commercial land use was associated with fewer pedestrian crashes through a study conducted in Hong Kong, where the number of overpasses increases in commercial areas, in addition to more compliance of people with traffic rules by using these facilities in crossing roads.
On the other hand, Ding et al., (Citation2018) found that the impact of commercial land use on pedestrian safety fluctuates based on its proportion from the total studied zone; therefore, when the proportion of commercial land use value is up to 8%, the pedestrian crash frequency increases by around 1.2 accidents. Otherwise it has a negative impact on the number of pedestrian accidents. This is due to higher pedestrian volumes in larger commercial land use, which leads to an increment in drivers’ attention, leading them to reduce their driving speed. Ukkusuri et al., (Citation2012) and (Obinguar & Iryo-Asano, Citation2021) came to the conclusion that industrial land use is significantly associated with more pedestrian crashes, which may refer to the high-speed limits there in addition to loading and unloading manoeuvres, which are associated with a higher risk of pedestrian accidents. However, this finding does not agree with the significant negative association raised by Chen & Zhou, (Citation2016), Miranda-Moreno et al., (Citation2011) and Ukkusuri et al., (Citation2011).
2.2 Intersection configuration
In relation to intersection configuration, Quistberg et al., (Citation2015), Wang et al., (Citation2016) and Ding et al., (Citation2018) reported that intersections with four arms and more significantly witness more pedestrian crashes due to a larger number of conflicts between pedestrians and vehicles in comparison to three-arm intersections.
2.3 Number of lanes
Ukkusuri et al., (Citation2012), Rankavat & Tiwari, (Citation2015) and Congiu et al.,(Citation2019) concluded that a higher number of lanes is significantly associated with more pedestrian-involved crashes. It is widely demonstrated that a higher number of lanes may lead to an increase in vehicular speed and driving manoeuvres, whereby resulting in a higher risk of pedestrian collisions (Strasser et al., Citation2010).
2.4 Road/Lane width
Ukkusuri et al., Citation(2012), Quistberg et al., (Citation2015), Barón et al., (Citation2021), and Zhu et al., (Citation2022) summarised that wider roads/lanes require a longer time for pedestrians to cross, in addition to providing a more comforting feeling for drivers to speed as well as more space for complex manoeuvres, resulting in a significantly higher likelihood that pedestrian crashes will occur.
2.5 Public transit facilities
The association between the frequency of pedestrian – vehicle accidents and various public transit facility variables has been investigated and found to be statistically significant. Public transit facilities usually refer to properties, equipment, services or buildings that are built, provided or established for a public transit system. Thus, such studies have involved many indicators for public transit facilities, such as the presence of subway/tram/bus/light bus stations, the presence of bus stops, the number of transit stops, the number of buses, the bus density, the bus stop density, the number of bus stops, the number of real bus stoppings, the frequency of bus trips, the amount of daily bus passenger boardings and alighting, and the number of taxis in the studied site/zone/location. Ukkusuri et al., (Citation2012), Quistberg et al., (Citation2015), Strasser et al., (Citation2010), Kraidi & Evdorides, (Citation2020), Su & Sze, (Citation2022), Zhu et al., (Citation2022), Ashraf et al., (Citation2022) and Rahman et al., (Citation2022) concluded that public transit facilities are significantly associated with more pedestrian crashes. This is due to larger and more frequent pedestrian activities and crossings near public transit stations and bus stops, in addition to the obstructed vision limitations resulting from parked buses, trams and taxis, which increase the probability of pedestrian collisions (Pulugurtha & Srirangam, Citation2021; Sun et al., Citation2020; Ulak et al., Citation2021). Simultaneously, Lizarazo & Valencia, (Citation2018) found that an increase in the number of taxis in particular usually reduces pedestrian crashes, as the entire trip by a taxi is covered from the origin to the destination, a matter that decreases walking trips, which will ultimately reduce exposure and collision risk.
2.6 On-street parking
Congiu et al., (Citation2019), Kraidi & Evdorides, (Citation2020) and Zhu et al., (Citation2022) examined the relationship between the presence of on-street parking and pedestrian crashes, reporting that the presence of on-street parking in comparison with no on-street parking is three times more likely to result in more pedestrian crashes. This is due to the fact that parked vehicles entail visual obstruction for pedestrians and drivers, which increases pedestrian crashes. However, a study conducted in Portugal stated that on-street parking is significantly associated with fewer pedestrian accidents because of the reduction in vehicular speeds as a consequence of parking manoeuvres Barón et al., (Citation2021).
2.7 Vehicular volume
Strauss et al., (Citation2014) found that as traffic flow increases by 10%, an increase in pedestrian crashes of 5.6% can be observed at signalised intersections. The same significant relationship was found by Kraidi & Evdorides, (Citation2020), Tulu et al., (Citation2015) and Guerra et al., (Citation2020). In contrast, Nabavi Niaki et al., (Citation2016) and Barón et al., (Citation2021) stated that an increase in vehicle traffic flow is significantly associated with a smaller number of pedestrian crashes, as vehicles on congested roads proceed at lower speeds.
2.8 Pedestrian volume
Ukkusuri et al., (Citation2012), Strauss et al., (Citation2014), Tulu et al., (Citation2015), Nabavi Niaki et al., (Citation2016)and Zhu et al., (Citation2022) reported that the pedestrian volume is significantly associated with more pedestrian crashes, as a higher pedestrian volume creates higher pedestrian activities, which in turn can lead to a higher probability of conflicts with vehicles, a matter that may result in a higher rate of crashes. Nevertheless, Barón et al., (Citation2021) and Rankavat & Tiwari, (Citation2015) concluded that when the number of pedestrians increases, divers will proceed with a higher degree of attention (Elvik & Bjørnskau, Citation2017) and reduce their speed, which means that collisions with pedestrians will be less likely to occur. This supports the concept of ‘safety in numbers’ Jacobsen et al., (Citation2015).
Overall, it has been found that many roadway environment characteristics have statistically significant correlations with pedestrian crashes. This means that they may affect the likelihood of pedestrian – vehicle crash occurrences and will be considered in the statistical modelling process in our study. These include: 1) number of arms, 2) number of lanes, 3) number of permitted parking sides, 4) lane width, 5) land use, and 6) presence of public transit facilities, in addition to traffic volume factors.
3. Statistical modelling technique
Building on the purpose of this study, a statistical approach was used to investigate the effects of roadway environment characteristics (used as independent variables in the modelling process) on the frequency of pedestrian – vehicle crashes (dependent variable) at signalised intersections. Generalised Linear Mixed Models (GLMM) were used in this study due to their ability to: 1) deal with crash data, which are known as rare events (i.e. many locations in our study are with zero records, in addition to the small sample size of 166 pedestrian crashes), as well as non-normally distributed and non-negative data (count) (Lord & Geedipally, Citation2011) (i.e. the crash data in our study found to follow Gamma distribution), and 2) overcome common limitations usually found in actual crash studies such as comprehensiveness of crash data, time interval variations, small sample size, and lack of exposure variables counts as mentioned in Section 4.2. Thus, GLMMs were used in our study as they are able to:
Allow nonlinear links between the dependent variable (crash frequency) and the independent variables (roadway geometric variables and traffic volumes),
Fit non-normally distributed values,
Deal with hierarchical data in order to model the multiple effects of multiple variables, including a mixture of continuous, discrete, categorical and ordinal data,
Deal with a dependent variable that involves repeated measures, and
Consider random effects, which allow factors to vary across observations to account for unobserved heterogeneities within sites observed (spatial unobserved heterogeneities) across multiple years (temporal unobserved heterogeneities) (Coxe et al., Citation2009; Atkins et al., Citation2013; Bell et al., Citation2019; Bono et al., Citation2021).
Therefore, utilising the multilevel Generalised Linear Mixed Gamma regression model in our study leads to have a novel contribution to the existing pedestrian crash frequency modelling.
There are many estimators that can be utilised in developing GLMMs to model accident frequency, such as Poisson, negative binomial, and gamma regression (Kraidi & Evdorides, Citation2020; Osama & Sayed, Citation2017; Quistberg et al., Citation2015; Ukkusuri et al., Citation2012). Overall, this study developed many GLMMs and selected the best-fit model by evaluating each based on: 1) Goodness-of-Fit (GOF) measures, utilising Akaike Information Criterion (AIC) and Bayesian Information Criteria (BIC) measures (where lower values indicate a better fit (Washington, Citation2020), and 2) validity, through plotting the predicted crash frequencies against the observed ones to assess how well the resulting model fits the observed data (Kraidi & Evdorides, Citation2020) (where larger agreement indicates better validity).
4. Site selection and data description
This section covers the process of selecting the signalised intersections, data description, and general statistics of the crash data.
4.1 Site selection
The studied signalised intersections were selected based on the roadway environment characteristics considered and the availability of a broad range of residential, industrial and commercial areas, traffic volumes, pedestrian activities, traffic interactions, bus stops, and on-street parking. These signalised intersections were selected to satisfy these requirements of research criteria from different districts in Amman. For this reason, serious attention was paid to the properties of the selected intersections, which have traffic control signals and crosswalks to facilitate data collection and ensure their accuracy. Additionally, a precursory survey was conducted to find appropriate signalised intersections that met the criteria and could be included in this study.
In Amman, there are 170 installed traffic signals (at the time of data collection: December 2020 to February 2021). Eighty-four of them are installed at intersections and equipped with video cameras. Eleven (out of 84) were turned off during the data collection period due to construction works of the Bus Rapid Transit project. Accordingly, 47 signalised intersections met the aforementioned criteria and, therefore, were selected for the analysis. Appendix A shows the studied sample with general characteristics.
The data for each intersection were collected based on a buffer size of a 200 m diameter drawn around the centre of each intersection (Obinguar & Iryo-Asano, Citation2021; Rankavat & Tiwari, Citation2015). This was conducted using Geographic Information System (GIS) maps available in the Central Traffic Department (CTD) in Amman and, thus, the geometric design and the surrounding public space characteristics, land use types, and traffic volumes, in addition to pedestrian crash data were collected within these identified buffer areas.
4.2 Data description and processing
4.2.1 Crash data
This study used pedestrian crash data obtained from police reports managed by the CTD. A pedestrian crash is defined as one involving at least a pedestrian and a vehicle. Police records include information on every reported crash, such as the date, location, weather conditions, degree of street lighting, injury level, and age and gender of the pedestrian involved in the crash. The final analysis sample involved a total of 166 pedestrian – vehicle crashes that occurred at 47 signalised intersections in Amman between 2007 and 2019, which were used as a dependent variable in the modelling process. The crash data were restructured into longitudinal data to account for the years of accidents for each intersection as a vector, whereby resulting in a data matrix (equal to 47 intersections X 13 years = 611 intersection accident year record). Additionally, prior to applying the modelling, the count of accidents was adjusted through the addition of a constant value ( = 1) to alleviate the zero accident values in the dependent variable. Thereafter, the resultant count score was transformed with the Natural Logarithm (LN) before commencing the modelling process in order to aid in decreasing any dispersion found in the crash data.
Generally, pedestrian safety studies are largely challenged by the limited available crash data, which suffer from some limitations and issues that have been found over the years. The most common issue is the small sample size. The scarcity of crash data, the long time periods required to collect an adequate sample size, and the high cost of collecting crash data may produce a low sample mean issue in safety studies, especially pedestrian safety ones. This issue is also found in developed countries, with sample sizes usually being small. For example, a research conducted in Philadelphia, US (fifth biggest city in US regarding population) to study pedestrian crash frequency happened during 2010–2017 on the whole street network of the city (not only signalised intersections as in our study) Guerra et al., (Citation2020), it found that large number of street segments are with zero or only a few pedestrian crashes. Rahman et al., (Citation2022) found in their study that each road segment witnessed around 0.11 pedestrian crash, while Kraidi & Evdorides, (Citation2020) found that 2.82 crashes happened per site on average in their study. The same was found in our study where 166 pedestrian crashes occurred on 47 intersections along 13 years in Amman.
This issue of crash data and many other issues (e.g. Comprehensiveness and quality of crash data) could be a potential source of mistakes in modelling, which may result in wrong inferences and a blurry vision of crash causes if they are not appropriately addressed and considered in the study.
Based on the literature review, different approaches are proposed by safety researchers and statisticians to overcome such limitations, such as:
Utilising appropriate statistical models and distributions to fit crash data well.
Adopting a grouped random parameters approach (Lord & Bonneson, Citation2007; Abdulhafedh, Citation2017), which allows variables to vary across different groups of observations in order to account for unobserved heterogeneity found in crash data due to time variation and small sample size.
Utilising different data processing techniques such as converting the traffic volume magnitude into natural logarithm values to fit the developed model (Kraidi & Evdorides, Citation2020).
4.2.2 Road characteristics
In compliance with the aim of this study and the literature review, the following roadway environment characteristics were collected for each signalised intersection and included in the modelling process as independent variables:
Number of arms
Number of lanes
Lane width
Land use
Presence of public transit facilities
Number of permitted parking sides
4.2.3 Annual Average Daily Traffic (AADT)
AADT is an exposure factor used in the modelling process as an independent variable. For all signalised intersections, the AADT values were obtained from the Traffic Control Unit (TCU) in Amman. They were collected for the year 2018. In this study, the intersection traffic volumes represented by AADT were transformed into T scores to allow for an explanation of the resultant coefficients and to obtain a more appropriate model via converting them into Z scores and then multiplying the Z-AADT score by 10 and adding the yielded product to 50 points. The resultant standardised T-AADT score has a mean of 50 and a standard deviation of 10 points.
4.2.4 Pedestrian crossing volume
The pedestrian crossing volume is another exposure factor of pedestrian crashes that was used in the modelling process as an independent variable. The number of pedestrians who crossed the major and minor roads of each intersection was gathered by conducting observations for the selected intersections and utilising video records obtained from the TCU. All observations were conducted from December 2020 to February 2021 during weekdays between 12 p.m. and 5 p.m. (peak period).
below show the description and descriptive statistics of the collected data used in the modelling process.
Table 1. Descriptive statistics and description of quantitative variables.
Table 2. Descriptive statistics and description of categorical variables.
4.3 General statistics of pedestrian crash frequency and severity
Based on the provided information on pedestrian crashes reported in the police reports, frequencies and percentages were calculated to present a general description as well as statistics of pedestrian crashes that had occurred at signalised intersections in Amman during the previous 13 years. It was found that around 98.8% of the crashes occurred in clear weather, which means that weather conditions may not have a significant impact on pedestrian safety. Regarding seasonal variation, 15% and 25% of crashes happened in the autumn and winter seasons respectively, while 32% occurred in spring and 28% in summer. With regard to time and the lighting conditions of roads, 66% of crashes happened during the daytime, and 29% and 5% occurred at nighttime with sufficient and insufficient lighting conditions respectively. According to the injury level, it was found that 5% of pedestrian crashes were fatal, 11% were severe, 6% were moderate, and 78% resulted in slight injuries. It was obvious that the most widely involved gender in the crashes was male, as males were present in 75% of cases. In relation to pedestrians’ age, about 48% of those involved in the accidents were children aged 15 years or below, which means that there is importance in investigating the causes of this high crash frequency for this age group with regard to improving their safety on roads. It is worth indicating that the influence of such factors on pedestrian safety is beyond the scope of this study, but is recommended for investigation in future research.
5. Results and discussion
This section presents the modelling process followed in building the Generalise Linear Mixed Gamma (GLMG) regression model, discusses the modelling results in detail, and evaluates the resultant model.
5.1 Model building
To investigate the influence of roadway environment characteristics and traffic volumes on the crash frequency, a GLMM was developed using IBM SPSS Statistics (version 28). Firstly, in order to reduce the impact of multicollinearity among the independent variables, which may lead to a less precise estimation and/or bias, bivariate correlation analysis using Spearman’s Rho test was conducted between these variables. The analysis did not result in a high correlation between any two variables; therefore, all independent variables were kept in the initial model. Secondly, based on the recommendations of past studies and the observed characteristics of the crash data, which are non-normally distributed but positive right-skewed values (as shown in ), it was found that the crash data follow gamma distribution. Therefore, gamma distribution was adopted in the modelling process of the GLMM.
Furthermore, to obtain the best-fit model, only statistically significant independent variables were included in the final model, while an independent variable was considered to be significant if its p-value was lower than 0.05. This means that at the beginning, all independent variables were included in the analysis; thereafter, any insignificant one was removed before rerunning the model. As a result, a multilevel GLMG regression model was developed, the general form of the pedestrian crash frequency model, which is mainly descriptive (explanation) model rather than predictive, is as following:
GLMM(∼I (1/x), family = Gamma (link = ‘log’))
Where:
—Number of pedestrian-vehicle crashes for intersection r within u year;
EXP —Exponential function;
T-AADT and PCV —Exposure factors (T-score of the AADT and Pedestrian Crossing Volume) to traffic of pedestrians in intersection r within u year;
—Independent variable i observed on intersection r within u year;
β˳and —Model parameters (coefficients) to be estimated; and
I —Number of significant explanatory variables X ( = 5)
shows the parameter significance and the estimation results of the developed GLMG model, where all independent variables included in this study were found to be statistically significant at a level of 5%, except for number of arms variables (which were excluded from the final model). This may be due to the small variation of numbers of arms in the studied intersections, since most of them have three or four arms (38% and 55% respectively), while only 7% of them have five or six arms.
Table 3. Statistical properties of the resultant model.
In addition, presents the coefficient for each independent variable that represents the average change in the dependent variable (crash frequency) when the independent variable changes by one unit. This is called the effect size of the variable, and the sign of the coefficient (positive or negative) provides the direction of that effect. Briefly, shows that the number of lanes, the average lane width, the number of parking sides, the pedestrian crossing volume, and AADT are positively correlated with the pedestrian crash frequency, whereas commercial land use and the presence of public transit facilities are negatively correlated with the pedestrian crash frequency. The results of the developed GLMG regression model will be discussed in detail in the next subsection.
5.2 Significance of coefficients
Based on , it was found that the pedestrian crossing volume is positively associated with the pedestrian crash frequency. An increase in the number of pedestrian crossings by one crossing is associated with an average increase in the number of crashes by 0.2%. The reason behind that could be the increase in pedestrian exposure to risk with increasing pedestrian crossing volumes, which is the same relationship found by Leden (Citation2002) and Guo et al. (Citation2017).
Obviously, AADT was found to be positively associated with the pedestrian crash frequency. An increase in the T score (AADT) by one unit resulted in an average increase in the number of crashes by 4.7%. The reason for this may be that a higher vehicle volume leads to higher conflicts between vehicles and pedestrians, which increases the probability of collisions occurring. Previously, Haleem et al., (Citation2015) and Stipancic et al. (Citation2020) found the same result.
Regarding the lane width, a significant positive correlation between that factor and the number of pedestrian crashes was concluded. For each 1 m increase in the lane width, the mean number of pedestrian accidents tended to increase by a factor equal to 0.655 on average. This may be due to an increase in the crossing distance and the required time to cross, which leads to an increase in pedestrian exposure to vehicular traffic while crossing the road, and this was found to be in agreement with Quistberg et al., (Citation2015).
Moreover, the number of lanes of the intersection correlated significantly and positively with the accident count, which increases by 1.9% with any additional lane. Since an increase in the number of lanes gives a comforting feeling to drivers for speeding and manoeuvring, it increases the likelihood of colliding with a pedestrian. Some previous studies found the same significant positive correlation, including Ukkusuri et al., (Citation2012), Nabavi Niaki et al., (Citation2016) and Congiu et al., (Citation2019).
Besides, the multivariate analysis model indicated that the number of parking sides converged significantly and positively on the number of pedestrian accidents, which is in agreement with Quistberg et al., (Citation2015), Xie et al., (Citation2018) and Congiu et al., (Citation2019), suggesting that for each increase of one parking side on those intersections, the predicted mean accident count tends to increase by a factor equal to 0.014 on average. This is likely because of the resultant obstructed vision for pedestrians and drivers due to parked vehicles along a road, as well as the presence of curb parking associated with parking and unparking manoeuvres, which leads to a chaotic situation on roads as well as increased conflicts with both passing vehicles and crossing pedestrians.
In terms of land use, the findings showed that industrial land use may not necessarily differ significantly with respect to the pedestrian crash frequency in comparison to residential land use areas. Again, it seems that the small number of studied intersections located in industrial zones resulted in some difficulties in modelling any significant correlation with pedestrian crashes (6.38% of all studied intersections, as mentioned previously in ).
On the other hand, it was found that commercial land use has a significant negative effect on the pedestrian accident frequency, implying that the accident count is lower on intersections located in commercial land use areas in comparison to residential ones (a similar finding was also concluded by Guo et al., (Citation2017). This is possibly explained by the fact that commercial areas in Amman, which accommodate shops, markets, malls, mega stores, and office buildings, which are dense shared locations, usually attract high volumes of both vehicles and pedestrians at the same time, which can result in intense movement Barón et al., (Citation2021). Therefore, all road users behave with more attention, especially drivers, who reduce their driving speeds therein. In addition, the presence of many police officers in such locations, who are often found there to help in managing traffic jams and to issue traffic tickets for parking violations, can also have an effect.
Interestingly, the presence of public transit facilities, which mainly include buses, minibuses, and public shared taxis and are operating on fixed routes in Jordan (Al-Masaeid & Shtayat, Citation2016), is correlated significantly but negatively with the count of pedestrian accidents. This means lower crashes found on signalised intersections with public transit services in comparison to intersections with no such services. Most public transit modes stop upon users’ requests without committing to the defined stations, stops, and loading and unloading areas along roads. This is a common phenomenon in Jordan and many other developing countries (Lizarazo & Valencia, Citation2018). This element leads to frequent sudden stops and loading/unloading and parking/unparking manoeuvres, which make drivers more aware and drive at lower speeds to avoid hitting other vehicles and/or crossing pedestrians, which is safer. Furthermore, many roads in front of and/or behind public transit stations in Amman are equipped with pedestrian bridges, bringing about lower risks.
5.3 Model evaluation
Apparently, the resultant GLMG model was found to be the best-fit model, since it gives the lowest values of AIC = 595.51 and BIC = 604.3, as models with the lowest AIC and BIC are preferred (Akaike, Citation1974; Washington et al., Citation2010). At the same time, this model with all significant independent variables (predictors) improved the prediction of crash frequencies (shows a better fit) in comparison to their null model with no predictors included in the analytical model. This was evidenced by a significant decrease in the model fit parameters (AIC and BIC) for the resultant model in comparison to the empty one developed in the background of SPSS software.
To examine the validity of the resultant GLMG model, which means how well this model fits the observed data, the observed crash frequencies of the studied intersections were compared with the predicted crash frequencies that resulted as an output from the GLMG model. A high correlation (good agreement) was found between them, at R2 = 0.91, as shown in .
These results indicate that the full model, which includes all significant independent variables, represents a better fit than do other models with fewer independent variables. This suggests that the resultant GLMG model is more accurate and gives better crash frequency estimates.
6. Study limitations
According to the two crash data limitations found in this study, many consideration points should be highlighted to enhance pedestrian safety research in Jordan:
It is possible that the 166 pedestrian crashes recorded over a 13-year period and modelled in this study do not constitute the full record of all accidents occurring on the studied intersections, since in Jordan it is commonly known that some people involved in pedestrian – vehicle crashes, especially crashes with no/slight injury, may not report to the police. This issue of underreporting sometimes results in a relatively small sample size in pedestrian safety studies or unreliable cases of excess zero counts that could reduce the model’s validity as well as the certainty of the results (Lord & Bonneson, Citation2007; Abdulhafedh, Citation2017). Therefore, there is a need for traffic authorities to adopt supporting tools and documents to overcome this issue.
It is worth noting that there are no systematic actual traffic count records at the time of collision, specifically for pedestrian volumes, available for all locations over the analysed 13-year period in the database in Jordan. Therefore, we used peak period counts of pedestrian crossing volumes. This limitation is also found in developed countries, with traffic counts usually being many years out of date (Tulu et al., Citation2015; Obinguar & Iryo-Asano, Citation2021; Kraidi & Evdorides, Citation2020; Barón et al., Citation2021), which could affect the certainty of the study findings. Therefore, there is a vital need to overcome such limitations in traffic safety research through adopting advanced, low-cost, time-saving methods and technologies in order to keep systematic records of traffic counts to develop more reliable models and obtain more accurate results.
However, this study dealt with these two limitations and their noise in the dataset by developing a valid GLMG model based on GOF measures (the model with the lowest AIC and BIC values was selected) and the agreement between observed crash frequencies and the predicted ones (the model with the highest agreement value was selected). GLMMs generally consider the random effect in the crash data, which allows variables to vary across different groups of observations (intersections over years) in order to account for unobserved heterogeneity found in the crash data due to these limitations (Abdulhafedh, Citation2017; Kraidi & Evdorides, Citation2020; Lord & Bonneson, Citation2007). Therefore, the possible uncertainties and estimation bias were minimal in this study.
7. Conclusions
This study sought to find the most significant roadway environment characteristics that determine the frequency of pedestrian – vehicle accidents at signalised intersections and to investigate their correlation with the crash data by using a dataset collected for 47 signalised intersections in Amman over a 13-year period, considering the availability of a broad range of roadway environment characteristics in these intersections and integrating them with vehicular and pedestrian traffic volumes. By considering various common limitations of crash data and traffic volume counts, especially those that appear in developing countries, as well as issues that have arisen with previous similar studies in general, a multilevel GLMG regression model was developed and found to be statistically significant in explaining the complex effects of many roadway environment characteristics on pedestrian crash frequencies. The results of modelling indicated that most roadway environment factors included in this study, in addition to traffic volumes, were found to be correlated significantly and positively with pedestrian crash frequencies, which comprise the number of lanes, the average lane width, the number of parking sides, the pedestrian crossing volume, and AADT, while commercial land use and the presence of public transit facilities have significant negative correlations with pedestrian crashes.
Overall, the results of the valid model could be used by traffic authorities and planners to evaluate other existing signalised intersections in Jordan with regard to pedestrian safety through identifying hotspots that need safety interferences as a proactive approach. Furthermore, this study presents a new vision for developing countries in determining and explaining the causes of pedestrian crashes in the challenging context that exists.
8. Recommendations
Based on the results of this study, special consideration is required for pedestrian safety in Jordan in terms of built environment planning, roadway geometric design, and enforcement, as follows:
There is an increasing need to restrict on-street parking that brings about a high risk for pedestrians. Alternatively, authorities are encouraged to provide multi-floor garage buildings or smart parking services on roads with a higher demand for parking lots.
More pedestrian bridges or underpasses on wide roads should be provided to separate pedestrians from vehicular traffic movement. Alternatively, traffic authorities should review all posted speed limits on wide roads, reduce them, and adopt efficient methods with which to capture speeding vehicles to impose penalties on them.
Despite the violations committed by operators in public transit facilities resulting in fewer pedestrian crashes in Amman, it is better to enhance this sector to encourage people to use public transit modes during their trips, instead of owning private cars. This will result in fewer vehicle traffic volumes on roads and, thus, pedestrian safety will be improved, in addition to other environmental benefits.
Based on the in-depth investigation, it was concluded that commercial land use and the presence of public transit services improve pedestrian safety by increasing the attention level of road users. In Jordan, when we discuss the importance of road users’ awareness of reducing pedestrian crashes, we are biased towards pedestrians over drivers due to the absence of a law that penalises pedestrians for committing any violations. In such cases, the driver in Jordan is always guilty; therefore, it is important to address this issue and sort it out with the legislative authority in order to put things right and improve road users’ safety in Jordan (Ministry of Justice of the Hashemite Kingdom of Jordan Citation2018; Public Security Directory in Jordan, Citation2008).
Additionally, the pedestrian safety situation in some countries, like GB, was found to be promising and deserves to be explored so as to learn from experience and benefit from the methodologies and procedures followed in improving pedestrian safety there. The influence of other contributing factors to pedestrian safety should be investigated in future work, such as demographic and socioeconomic characteristics, traffic control signal factors, and road user behaviour, for a comprehensive study with a broad explanation of multiple effects.
Disclosure statement
No potential conflict of interest was reported by the authors.
Additional information
Funding
References
- Abdulhafedh, A. (2017). Road traffic crash data: An overview on sources, problems, and collection methods. Journal of Transportation Technologies, 7(2), 206–21. https://doi.org/10.4236/jtts.2017.72015
- Akaike, H. (1974). A new look at the statistical model identification. IEEE Transactions on Au-Tomatic Control, Automatic Control, IEEE Transactions On, IEEE Trans.Automat.contr, 19(6), 716–723. https://doi.org/10.1109/TAC.1974.1100705
- Al-Masaeid, H., & Shtayat, A. (2016). Performance of urban transit in Jordan. International Journal of Engineering Research and Applications, 6(8), 7–12. https://scholar.google.com/scholar_lookup?title=Performance%20of%20urban%20transit%20in%20Jordan&author=H.%20R.%20Al-Masaeid&author=A.%20Shtayat&publication_year=2016.
- Al-Omari, B. H., & Obaidat, E. S. (2013). Analysis of pedestrian accidents in Irbid City, Jordan. The Open Transportation Journal, 7(1), 1–6. https://doi.org/10.2174/1874447801307010001
- Ashraf, M. T., Dey, K., & Pyrialakou, D. (2022). Investigation of pedestrian and bicyclist safety in public transportation systems. Journal of Transport & Health, 27, 27. https://doi.org/10.1016/j.jth.2022.101529
- Atkins, D. C., Baldwin, S. A., Zheng, C., Gallop, R. J., & Neighbors, C. (2013). A tutorial on count regression and zero-altered count models for longitudinal substance use data’: Correction to Atkins et al (2012). Psychology of Addictive Behaviors, 27(2), 379. https://doi.org/10.1037/a0033147
- Barón, L., Prudêncio Jacques, E., Otila da Costa, J., Soares, F., Faria, S., & Fraga de Freitas, M. A. (2021). Effect of built environment factors on pedestrian safety in Portuguese urban areas. Applied System Innovation, 4(2), 28. https://doi.org/10.3390/asi4020028
- Bell, A., Fairbrother, M., & Jones, K. (2019). Fixed and random effects models: Making an informed choice. Quality & Quantity: International Journal of Methodology, 53(2), 1051–1074. https://doi.org/10.1007/s11135-018-0802-x
- Bono, R., Alarcón, R., & Blanca, M. J. (2021). Report quality of generalized linear mixed models in psychology: A systematic review. Frontiers in Psychology, 12. https://doi.org/10.3389/fpsyg.2021.666182
- Chen, P., & Zhou, J. (2016). Effects of the built environment on automobile-involved pedestrian crash frequency and risk. Journal of Transport & Health, 3(4), 448–456. https://doi.org/10.1016/j.jth.2016.06.008
- Congiu, T., Sotgiu, G., Castiglia, P., Azara, A., Piana, A., Saderi, L., & Dettori, M. (2019). Built environment features and pedestrian accidents: An Italian retrospective study. Sustainability (Switzerland), 11(4), 1064. https://doi.org/10.3390/su11041064
- Coxe, S., West, S., & Aiken, L. (2009). The analysis of count data: A gentle introduction to Poisson regression and its alternatives. Journal of Personality Assessment, 91(2), 121–136. https://doi.org/10.1080/00223890802634175
- Department for TRANSPORT. (2020). Reported road casualties in Great Britain, annual report: 2019. https://www.gov.uk/government/statistics/reported-road-casualties-great-britain-annual-report-2019
- Ding, C., Chen, P., & Jiao, J. (2018). Non-linear effects of the built environment on automobile-involved pedestrian crash frequency: A machine learning approach. Accident Analysis and Prevention, 112, 116–126. https://doi.org/10.1016/j.aap.2017.12.026
- Dumbaugh, E., Li, W., & Joh, K. (2013). The built environment and the incidence of pedestrian and cyclist crashes. Urban Design International, 18(3), 217–228; 228. https://doi.org/10.1057/udi.2013.2
- Elvik, R., & Bjørnskau, T. (2017). Safety-in-numbers: A systematic review and meta-analysis of evidence. Safety Science, 92, 274–282. https://doi.org/10.1016/j.ssci.2015.07.017
- Elvik, R., Sørensen, M. W. J., & Nævestad, T. (2013). Factors influencing safety in a sample of marked pedestrian crossings selected for safety inspections in the city of oslo. Accident Analysis and Prevention, 59, 64–70. https://doi.org/10.1016/j.aap.2013.05.011
- Guerra, E., Dong, X., Lin, L., & Guo, Y. (2020). Temporal analysis of predictors of pedestrian crashes. SAGE Publications Ltd Retrieved from. https://search.ebscohost.com/login.aspx?direct=true&AuthType=ip,sso&db=edselc&AN=edselc.2-52.0-85094873448&site=eds-live&scope=site&custid=s6043660
- Guo, Q., Xu, P., Pei, X., Wong, S. C., & Yao, D. (2017). The effect of road network patterns on pedestrian safety: A zone-based bayesian spatial modeling approach. Accident Analysis and Prevention, 99, 114–124. https://doi.org/10.1016/j.aap.2016.11.002
- Haleem, K., Alluri, P., & Gan, A. (2015). Analyzing pedestrian crash injury severity at signalized and non-signalized locations. Accident Analysis and Prevention, 81, 14–23. https://doi.org/10.1016/j.aap.2015.04.025
- Jacobsen, P. L., Ragland, D. R., & Komanoff, C. (2015). Safety in numbers for walkers and bicyclists: Exploring the mechanisms. Injury Prevention, 21(4), 217–220. https://doi.org/10.1136/injuryprev-2015-041635
- Kraidi, R., & Evdorides, H. (2020). Pedestrian safety models for urban environments with high roadside activities. Safety Science, 130. https://doi.org/10.1016/j.ssci.2020.104847
- Leden, L. (2002). Pedestrian risk decrease with pedestrian flow. A case study based on data from signalized intersections in Hamilton, Ontario. Accident Analysis and Prevention, 34(4), 457–464. https://doi.org/10.1016/S0001-4575(01)00043-4
- Lizarazo, C., & Valencia, V. (2018). Macroscopic spatial analysis of pedestrian crashes in medellin. colombia SAGE Publications Ltd Retrieved. https://search.ebscohost.com/login.aspx?direct=true&AuthType=ip,sso&db=edselc&AN=edselc.2-52.0-85045264514&site=eds-live&scope=site&custid=s6043660
- Lord, D., & Bonneson, J. A. (2007). Development of accident modification factors for rural frontage road segments in texas. Transportation Research Record: Journal of the Transportation Research Board, 2023(1), 20–27. https://doi.org/10.3141/2023-03
- Lord, D., & Geedipally, S. R. (2011). The negative binomial–Lindley distribution as a tool for analyzing crash data characterized by a large amount of zeros. Accident Analysis and Prevention, 43(5), 1738–1742. https://doi.org/10.1016/j.aap.2011.04.004
- Ministry of Justice of the Hashemite Kingdom of Jordan. (2018). Jordan penal code of 1960 with amendments through 2018. https://www.scribd.com/document/265701032/Jordan-Penal-Code-1960
- Miranda-Moreno, L., Morency, P., & El-Geneidy, A. (2011). The link between built environment, pedestrian activity and pedestrian–vehicle collision occurrence at signalized intersections. Ac-Cident Analysis and Prevention, 43(5), 1624–1634. https://doi.org/10.1016/j.aap.2011.02.005
- Moradi, A., Soori, H., Kavousi, A., Eshghabadi, F., & Jamshidi, E. (2016). Spatial factors affecting the frequency of pedestrian traffic crashes: A systematic review. Archives of Trauma Research, 5(4). https://doi.org/10.5812/atr.30796
- Nabavi Niaki, M. S., Saunier, N., Fu, T., Miranda-Moreno, L., Amador, L., & Bruneau, J. (2016). Road lighting effects on bicycle and pedestrian accident frequency: Case study in Montreal, Quebe. Canada National Research Council. Retrieved from https://search.ebscohost.com/login.aspx?direct=true&AuthType=ip,sso&db=edselc&AN=edselc.2-52.0-85015404640&site=eds-live&scope=site&custid=s6043660
- Narayanamoorthy, S., Paleti, R., & Bhat, C. R. (2013). On accommodating spatial dependence in bicycle and pedestrian injury counts by severity level. Transportation Research Part B, 55, 245–264. https://doi.org/10.1016/j.trb.2013.07.004
- National Academies of Sciences, Engineering, and Medicine. (2008). Highway safety manual data needs Guide. The National Academies Press. https://doi.org/10.17226/23089
- NATIONAL HIGHWAY TRAFFIC SAFETY ADMINISTRATION. (2020). 2018 PEDESTRIANS Traffic Safety Fact Sheet DOT HS. 812 850. https://crashstats.nhtsa.dot.gov/Api/Public/ViewPublication/812850
- Obinguar, D. D., & Iryo-Asano, M. (2021). Macroscopic analysis on the frequency and severity of pedestrian crashes on National Roads in Metro Manila, Philippines. IATSS Research, 45(4), 521–529. https://doi.org/10.1016/j.iatssr.2021.06.003
- Osama, A., & Sayed, T. (2017). Evaluating the impact of connectivity, continuity, and topography of sidewalk network on pedestrian safety. Accident Analysis and Prevention, 107, 117–125. https://doi.org/10.1016/j.aap.2017.08.001
- Public Security Directory in Jordan. (2008). Jordan Traffic Law. https://www.psd.gov.jo/media/devj2owl/%D9%82%D8%A7%D9%86%D9%88%D9%86-%D8%A7%D9%84%D8%B3%D9%8A%D8%B1.pdf
- Public Security Directory in Jordan. (2020). Annual report of traffic accidents in Jordan for the year 2019. التقرير-السنوي-للحوادث-المرورية-في-الأردن-لعام–2019.pdf (psd.gov.jo).
- Pulugurtha, S. S., & Srirangam, L. P. (2021). Pedestrian safety at intersections near light rail transit stations. Public Transport: Planning and Operations, 14(3), 1–26. https://doi.org/10.1007/s12469-021-00276-y
- Quistberg, D. A., Koepsell, T. D., Boyle, L. N., Johnston, B. D., Ebel, B. E., & Miranda, J. J. (2015). The walking environment in lima, Peru and pedestrian–motor vehicle collisions: An exploratory analysis. Traffic Injury Prevention, 16(3), ; 314; 314–; 321; 321. https://doi.org/10.1080/15389588.2014.930830
- Rahman, M., Kockelman, K. M., & Perrine, K. A. (2022). Investigating risk factors associated with pedestrian crash occurrence and injury severity in texas. Traffic Injury Prevention, 23(5), 283; 283–289; 289. https://doi.org/10.1080/15389588.2022.2059474
- Rankavat, S., & Tiwari, G. (2015). Association between built environment and pedestrian fatal crash risk in Delhi. India National Research Council. Retrieved from https://search.ebscohost.com/login.aspx?direct=true&AuthType=ip,sso&db=edselc&AN=edselc.2-52.0-84976603733&site=eds-live&scope=site&custid=s6043660
- Stipancic, J., Miranda-Moreno, L., Strauss, J., & Labbe, A. (2020). Pedestrian safety at signalized intersections: Modelling spatial effects of exposure, geometry and signalization on a large urban network. Accident Analysis and Prevention, 134, 105265. https://doi.org/10.1016/j.aap.2019.105265
- Strasser, S., Steward, J., Taquechel, E. P., & Dai, D. (2010). The impact of built environment on pedestrian crashes and the identification of crash clusters on an urban university campus. Western Journal of Emergency Medicine, 11(3), 294–301.
- Strauss, J., Miranda-Moreno, L., & Morency, P. (2014). Multimodal injury risk analysis of road users at signalized and non-signalized intersections. Accident Analysis and Prevention, 71, 201–209. https://doi.org/10.1016/j.aap.2014.05.015
- Sun, S., Fang, D., & Cao, J. (2020). Exploring the asymmetric influences of stop attributes on rider satisfaction with bus stops. Travel Behaviour and Society, 19, 162–169. https://doi.org/10.1016/j.tbs.2020.01.004
- Su, J., & Sze, N. N. (2022). Safety of walking trips accessing to public transportation: A bayesian spatial model in hong kong. Travel Behaviour and Society, 29, 125–135. https://doi.org/10.1016/j.tbs.2022.06.003
- Tulu, G. S., Washington, S., Haque, M. M., & King, M. J. (2015). Investigation of pedestrian crashes on two-way two-lane rural roads in Ethiopia. Accident Analysis and Prevention, 78, 118; 118–126; 126. https://doi.org/10.1016/j.aap.2015.02.011
- Ukkusuri, S., Hasan, S., & Aziz, H. M. A. (2011). Random parameter model used to explain effects of built-environment characteristics on pedestrian crash frequency. Transportation Research Record: Journal of the Transportation Research Board, 2237(1), 98–106. https://doi.org/10.3141/2237-11
- Ukkusuri, S., Miranda-Moreno, L., Ramadurai, G., & Isa-Tavarez, J. (2012). The role of built environment on pedestrian crash frequency. Safety Science, 50(4), 1141–1151. https://doi.org/10.1016/j.ssci.2011.09.012
- Ulak, M. B., Kocatepe, A., Yazici, A., Ozguven, E. E., & Kumar, A. (2021). A stop safety index to address pedestrian safety around bus stops. Safety Science, 133. https://doi.org/10.1016/j.ssci.2020.105017
- Wang, X., Yang, J., Lee, C., Ji, Z., & You, S. (2016). Macro-level safety analysis of pedestrian crashes in shanghai, china. Accident Analysis and Prevention, 96, 12–21. https://doi.org/10.1016/j.aap.2016.07.028
- Washington, S. (2020). Statistical and econometric methods for transportation data analysis (Third edition. ed.) CRC Press. Retrieved from https://search.ebscohost.com/login.aspx?direct=true&AuthType=ip,sso&db=cat09707a&AN=dmu.EBC6031992&site=eds-live&scope=site&custid=s6043660
- World Health Organization. (2018). Global status report on road safety (online). Retrieved from https://www.who.int/2018.
- Xie, S. Q., Dong, N., Wong, S. C., Huang, H., & Xu, P. (2018). Bayesian approach to model pedestrian crashes at signalized intersections with measurement errors in exposure. Accident Analysis and Prevention, 121, 285–294. https://doi.org/10.1016/j.aap.2018.09.030
- Zhu, M., Sze, N. N., & Newnam, S. (2022). Effect of urban street trees on pedestrian safety: A micro-level pedestrian casualty model using multivariate bayesian spatial approach. Accident Analysis and Prevention, 176, 176. https://doi.org/10.1016/j.aap.2022.106818
Appendix A
List of the studied signalised intersections