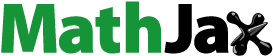
ABSTRACT
The study focuses on optimizing urban distribution in the dairy industry, specifically at Bhopal Sanchi dairy, to enhance efficiency and minimize costs. It evaluates existing routes, identifies inefficiencies, and proposes new distribution strategies. By implementing these changes, significant improvements are achieved, reducing total distribution costs and dead kilometers while improving vehicle capacity utilization. The study emphasizes the importance of addressing urban distribution challenges to meet increasing milk demand efficiently. It contributes to developing sustainable dairy distribution systems by optimizing costs and minimizing losses. ArcGIS software is utilized for route and fleet optimization analysis. Overall, the research addresses the critical need for efficient urban dairy distribution, particularly in India’s context as a major milk producer.
1. Introduction
The dairy industry holds a pivotal position in India, globally recognized as the leading producer of milk and dairy products. This sector, with its substantial domestic market, presents considerable opportunities for commercial success. Efficient supply chain management in the perishable food industry is crucial, given the short shelf life of dairy products. Urban freight distribution, integral to trading activities, brings both benefits and challenges, including infrastructure damage, congestion, resource wastage, and traffic inefficiency. In 2017, global milk production reached 811 million tonnes, with significant growth in Asia, America, and Europe, and declines in Oceania and Africa. India, contributing around 20% of the world’s milk, stands alongside the European Union (EU), followed by the United States, China, and Pakistan (FAO, Citation2017). In India, the production of milk has shown remarkable growth, increasing from 83.7 million tonnes in 2002 to 176.5 million tonnes in 2018. Concurrently, per capita milk consumption has risen from 221 grams per day to 374.7 grams per day during the same period (DAHD, Citation2018). Milk processing, a significant economic activity involving around 70 million rural households, has thrived, leading India to surpass the United States as the world’s top milk producer in 1998 (Nanda Kumar et al., Citation2022). India boasts the world’s largest livestock population, accounting for 56.5% of the global livestock population (DAHD, Citation2018). The surge in demand for processed foods due to urbanization has propelled the growth of milk production, facilitated by the expansion of milk collection and processing facilities, primarily overseen by dairy cooperatives (GoI, Citation2014). According to estimates, 48% of milk is preserved for personal and local use, while the remaining 52% is sold to urban consumers through organized (31%) and unorganized (21%) sectors (MoSPI, Citation2021). Milk cooperatives like Gujarat Cooperative Milk Marketing Federation (GCMMF) or Amul play a crucial role, with average milk purchases of 229 lakh liters per day (LPCD) in 2018–19, constituting 45% of total cooperative purchases (FAO, Citation2020). Private and cooperative enterprises share the market in the organized dairy sector. Despite the dominance of cooperatives, official figures on milk purchase volume and the private sector’s processing capacity often lack completeness (Lok Sabha, Citation2019).
In understanding the challenges of urban distribution in the dairy industry, it is crucial to understand the complex interplay of factors contributing to inefficiencies and costs. Urbanization trends, population growth, and evolving consumer preferences all influence the demand for dairy products, necessitating agile and efficient distribution systems to meet these changing needs. However, traditional approaches to distribution optimization often fall short in capturing the specific nuances and complexities of urban environments, where factors such as traffic congestion, narrow roadways, and varying delivery demands pose significant challenges. Moreover, while many studies provide insights into broader supply chain management principles and vehicle routing optimization strategies, there remains a notable gap in addressing the intricacies of urban dairy distribution, particularly within the Indian context. Limited studies do not adequately explore the unique challenges and prospects inherent in urban settings.
This research focuses on the challenges of urban distribution in the dairy industry, specifically in the Bhopal Sanchi dairy. The research question can be defined as: How to optimize cost and efficiency of urban dairy distribution in the Bhopal Sanchi dairy? To address this question, the study evaluates key performance indicators, assesses existing routes for efficiency, and proposes a model to minimize the overall cost of urban dairy distribution. The research aims to contribute to the development of more efficient and sustainable dairy distribution systems. The research also assesses the current distribution infrastructure in Bhopal Sanchi and explores strategies for enhancing urban distribution efficiency in the dairy industry. The methodology employed in this study addresses the unique challenges and intricacies associated with urban distribution in the dairy supply chain, particularly within the Old Bhopal zone. Traditional approaches to distribution optimization often fall short in capturing the specific nuances of our context, necessitating the development of a novel methodology. By optimizing costs and minimizing losses, the research aims to enhance supply chain efficiency and meet the growing demand for milk. The use of ArcGIS software contributes value to the research by providing route optimization analysis, recommendations, and fleet optimization strategies for the Bhopal Sanchi dairy.
2. Literature review
The milk-run dairy distribution supply system and the optimization of the vehicle routing problem were the subjects of a detailed review of the literature. The literature review study has shown the need for research in the area of dairy supply chain management for efficient optimization, since transportation costs have a substantial impact on the entire cost of the good or service.
2.1. Just-in-time material supply system
The key focus of lean manufacturing logistics is the just-in-time material supply system (Michel Baudin, Citation2004). It represents the lean mindset for logistical tasks within the factory, emphasizing efficient coordination between manufacturers and suppliers (Vaidyanathan et al., Citation1999) explored the JITVRP (Just-in-time vehicle routing problem), presenting a nonlinear mathematical model for vehicle routing. It proposed a two-stage heuristic method as an effective solution, aiming to minimize transportation and inventory costs. Describing the JIT periodic material delivery system for assembly lines (Satoglu & Sahin, Citation2013), used mathematical routing techniques and heuristic algorithms, focusing on cost minimization (Domingo et al., Citation2007) discussed a milk-run material supply system for lean assembly lines, presenting an effective technique for pickups and deliveries. by (Álvarez et al., Citation2009) conducted a case study on assembly line redesign with lean techniques, emphasizing the need for a suitable material-handling vehicle in conjunction with a Kanban production management system (Boysen & Bock, Citation2011) addressed JIT part supply scheduling for a mixed-model assembly line on different floors, employing dynamic programming and simulated annealing (Hao & Shen, Citation2008) developed a prototype software system to assess the effectiveness of a Kanban-based milk-run system supplying an assembly line. Lastly (Nemoto et al., Citation2010), described JIT external milk-run applications at the Toyota automotive assembly factory in Thailand, demonstrating the system’s ability to regulate procurement processes and optimize the supply network even in congested traffic conditions.
2.2. Vehicle routing in milk-run operations
Vehicle routing in milk-run operations involves optimizing routes for a fleet of vehicles to efficiently deliver supplies to various locations. Several transportation systems are taken into consideration in large-scale companies in order to deliver the stuff. However, it is quite difficult, particularly when dealing with large-scale companies where a huge quantity of supplies must be supplied to several places (Patel et al., Citation2014). A study (Patel et al., Citation2017) addressed this problem using optimization approaches to minimize material handling costs in a milk-run system. It proposed a mathematical model employing a standard branch and bound algorithm and a mathematical programming algorithm. Despite the challenges, the column generation-based branch and bound method demonstrated rapid issue resolution. Additionally, a local search method was presented for vehicle routing, aiming to reduce the need for vehicles in the supply chain by shortening travel distances. The study highlighted the need for more efficient heuristic approaches in large-scale problems.
2.3. Classification and modelling for milk-run distributions system
Milk-run material supply systems can be classified in various ways according to the application in industry (Kilic et al., Citation2012) have used assignment methodology for the classification and modelling of the problem. The general assignment problem, specific assignment problem, and set time periods assignment problem are the three primary categories into which the milk-run distribution system may be categorized. Each main category can be subdivided based on the number of routes and service period of the vehicle. As the routes and time period are uncertain, the first category general assignment problem can be further separated into single routed vehicle and multiple routed vehicles. These can be further divided as different time period and equally time period according to the time period. A dedicated assignment problem can also be divided as differently time period and equally time period since the routes are known but time period needs to be determined. The last category is sub divided into the one routed vehicle and multiple-routed vehicles since time periods are known but routes are unknown.
2.4. Vehicle routing and scheduling
The supply chain management system heavily depends on customer service planning and vehicle routing, significantly impacting the quality of supply chain industries. Routing and scheduling issues aim to reduce overall transportation costs within specified time frames, covering purchase prices, ongoing expenses, and fixed vehicle costs. The size of the supply network, the vehicle capabilities, and the routing and scheduling goals of the delivery system all affect how routing and scheduling issues are classified. It includes the Chinese postman problem, traveling salesperson problem, and vehicle routing difficulty (Haksever et al., Citation2000) discuss vehicle routing, scheduling, and combined routing and scheduling problems, employing various mathematical programming methods. Vehicle routing and scheduling are crucial for supply chain service providers, requiring effective planning to meet consumer needs in terms of time and cost effectiveness. Describing service aspects, delivery-time restrictions, and vehicle capacity is crucial in solving routing and scheduling challenges (Goli & Tirkolaee, Citation2023) addresses product portfolio design in dairy supply chain management, focusing on maximizing net cash flow and shareholder payouts. The study employs the ABD algorithm to optimize the model, contributing to the dairy industry by integrating financial and physical flows (Goli, Citation2023) proposes a framework for integrating blockchain-enabled closed-loop supply chain with robust product portfolio design, aiming to maximize changes in equity and satisfaction from the blockchain network (Goli, Ala, & Hajiaghaei-Keshteli, Citation2023) investigates the optimization of non-permutation flow-shop scheduling problems and lot-sizing with a focus on energy efficiency, introducing novel meta-heuristic algorithms (Goli et al., Citation2022) addresses the challenges of designing an efficient two-echelon distribution system using electric vehicles and introduces an Improved Moth-Flame Optimization algorithm (Goli, Ala, & Mirjalili, Citation2023) presents a robust framework for optimizing organ transplant supply chains, addressing uncertainty in shipment times through possibilistic programming and simulation.
2.5. Milk-run distribution systems for express industry
Milk-run material supply systems have been utilized in various logistics industries, but their application in express industries is limited. The integration of the milk-run system with the express industry aims to minimize transportation costs and enhance customer satisfaction. In a feasibility study (You & Jaio, Citation2014), introduced the milk-run supply system into the express distribution business based on a realistic market scenario. The approach combines the benefits of the milk-run system and express distribution, reducing transportation costs through multi-objective path optimization. The scheduling of trucks is achieved using conventional models and a savings method, and is distinct from traditional distribution modules.
2.6. Effect of milk-run supply system in global warming
Implementing the milk run logistics helps reduce the environmental impact of global warming caused by direct shipment. The milk-run material supply system optimizes overall transportation costs in a logistics network, contributing to a decrease in carbon emissions. A study (Brar & Saini, Citation2011), indicates that, even in highly dense traffic conditions, milk run logistics can be more effective, optimizing the number of trucks and reducing vehicles on the road. This reduction in vehicle utilization leads to a decrease in carbon emissions. The milk-run supply chain management system enhances vehicle utilization, decreases the number of vehicles, and effectively schedules routes to reduce travel distances, making it environmentally significant. The milk-run system also increases transportation reliability.
2.7. Research gap
The existing literature provides insights into various aspects of urban distribution and vehicle routing optimization in the dairy industry. Existing studies have explored related topics like vehicle routing in milk-run operations and just-in-time material supply systems but lack comprehensive research tailored to the dynamics of urban dairy distribution. However, a noticeable gap remains in addressing the specific challenges and optimization opportunities within urban contexts. Furthermore, while some studies have discussed the environmental benefits of milk-run logistics, further investigation is needed to understand how such optimization strategies can mitigate negative impacts in rapidly growing urban centers. Therefore, this research aims to fill this gap by analyzing the unique challenges and proposing cost optimization strategies specifically tailored to urban dairy distribution.
3. Case study
Bhopal is the capital city of Madhya Pradesh, India, and the second largest city of Madhya Pradesh with a population of 18,86,100 as per census 2011.
3.1. Case area selection: Sanchi Bhopal
‘Bhopal Sahakari Dugdh Sangh Maryadit’, affiliated to Madhya Pradesh State Cooperative Dairy Federation, Bhopal, covers 11 districts and deals with 22 milk chilling centers in its area of operation. Total procurement is 3,70,000 liters from all 11 districts, Bhopal’s contribution in the procurement of milk is 22,500 liters only, and sale in Bhopal urban area is 2,68,000 liters and the remaining district is 52,000 liters. The remaining procured milk is used for other dairy products. More than 85% of raw milk is used as processed milk and the remaining is used for other dairy products (Bhopal, Citation2021).
3.2. Zone characteristics
The ‘Bhopal Dugdh Sangh, Sanchi’, handles dairy procurement, processing, and distribution across 11 districts. The study considered the urban limits of Bhopal municipal corporation. Sanchi Bhopal is divided into three zones to facilitate the smooth movement of milk and other dairy products, with separate distribution vehicles for each zone. The zone-wise details of retail outlets and milk demand are described in .
Table 1. Zone-wise details of outlets.
4. Data collection and sampling methodology
The data collection includes various surveys like operator, stakeholder interviews, retail outlets interviews, as described in . For collecting data on freight distribution and agri-supply chain operations in the city where reconnaissance and face-to-face interviews were conducted in the identified freight generating and freight handling areas by adopting clustered sampling to easily identify the different homogenous strata based on a total number of employments, total shops, transport operators, etc. EquationEquation (1)(1)
(1) is used to obtain the sample size (Özturk Calik, Citation2022).
Table 2. Zone-wise sample survey.
where,
SS = Sample size,
e = percentage of error (in decimal) = 0.08,
z = z- score
N = population size.
In this study, cluster sampling approach, was adopted, a method commonly used in marketing research. Cluster sampling is usually more practical than representative sampling or simple sampling. It is used where multiple clusters of individuals are created from a population where they are indicative of homogenous characteristics and have an equal chance of being a sample of the market, transport operator, and distribution center in the study. The sample numbers need to be increased to realize accurate results, but the value savings involved made this process of accelerating clusters attainable. Data collection involved three main stakeholders: the processing plant, transport operators, and retail outlets. For the processing plant, the basic functioning of Bhopal Dugdh Sangh was mapped out, and stakeholder interviews were conducted to gain insights into losses, supply chain, costs, and distribution models. A total of 61 transport operators, each assigned to a specific route, were surveyed using a questionnaire to understand travel time, penalties, fuel consumption, vehicle idle time, loading, and unloading processes. Furthermore, data was gathered from retail outlets to gauge market demand, cross-check supply and demand information, and gather data on morning and evening shift demands as well as any additional costs involved. The use of cluster sampling, stakeholder interviews, and surveys of transport operators and retail outlets ensures a comprehensive and representative dataset for our study on urban distribution challenges in the dairy industry.
5. Results
5.1. Zone assessment
All zones are included in the city evaluation, which is based on a few standard metrics. These indicators are used to compare all zones. The city evaluation encompasses all zones, utilizing a set of standard metrics for comparison. The first indicator, distribution time, compares transit time and handling time, revealing the old Bhopal zone with the highest ratio of handling time to transit time. This is attributed to congested and narrow roads, with the average transit time being less than 38% of the average handling time in old Bhopal, as described in . The next indicator is the distance traveled, which examines dead kilometers (kilometers without actual distribution) and effective kilometers. The old Bhopal zone has an excessively high ratio of dead kilometers to effective kilometers, posing a challenge for the zone. Bhel zone follows with the minimum dead kilometers due to its plant location, as described in . Another key indicator is vehicle capacity utilization, which plays a crucial role in freight study, comparing the total payload capacity (in liters) with vehicle capacity utilization. The old Bhopal zone experiences the lowest vehicle capacity utilization in the evening due to low demand and consistent fleet use. as described in . Additionally, the indicator of average effective kilometers versus unloading time highlights the old Bhopal zone as having the lowest average effective kilometer and yet the highest unloading time, as described in . The zone faces significant challenges due to congested and narrow roads, leading to extended unloading times.
Based on extensive analysis, it is concluded that the Old Bhopal zone presents the most troublesome aspects among the three zones, warranting further detailed research in this area.
5.2. Old Bhopal zone assessment
Old Bhopal zone assessment is carried on various key indicators and for morning and evening routes separately. The distribution of milk takes place twice a day i.e. during morning and evening. The details of the morning and evening routes are mentioned in . The distribution cost is calculated by the following formula:
Table 3. Details of morning and evening route distribution.
Total Distribution CostVehicle Operating Cost + Idle time cost
Where;
This formulation accounts for both the operational expenses associated with vehicle usage and the idle time cost, which considers factors such as the Wholesale Price Index (WPI) of milk, the Price Competitiveness Index (PCI) of Bhopal relative to that of India, and the economic cost associated with operating Light Commercial Vehicles (LCVs).
Decision Variables:
Xij: Binary decision variable representing the utilization of route i in scenario j, where i denotes the route number and j denotes the scenario number.
Dij: Binary decision variable indicating the combination of routes i and j in the scenario-building process.
Parameters:
Cij: Vehicle operating cost for route i in scenario j.
WPI: Wholesale Price Index of milk.
PCI(Bhopal): Price Competitiveness Index of Bhopal.
PCI(India): Price Competitiveness Index of India.
Economic cost of running LCV: Economic cost associated with running a Light Commercial Vehicle (LCV).
3.2.1. Distribution time and distance travelled
The handling time in morning distribution routes 6, 7, 16, and 28 was found to be the highest, making them some of the most challenging routes due to their high handling time to transit time ratio. These routes also experience longer distribution times as they have the highest demand and also pass through city areas. Similarly, in the evening distribution, routes 11, 26, and 61 have the maximum handling time due to their high handling time to transit time ratio caused by the high demand in the evening. These routes have the longest handling times in the evening. On the other hand, route 7, with the lowest demand, has the shortest handling time, as shown in .
Additionally, routes 16, 26, 28, and 63 were identified as problematic routes for morning distribution in the old Bhopal zone due to their maximum differences in the ratio of dead kilometers to effective kilometers. Routes 28 and 63 have the highest dead kilometers due to their distant initial distribution points from the plant. On the other hand, routes 26, 58, and 59 were identified as problematic routes for evening distribution due to their maximum differences in the ratio of dead kilometers to effective kilometers, with route 26 having a dead kilometer ratio five times that of an effective kilometer, and routes 58 and 59 having four times that ratio, as depicted in , respectively.
5.2.2. Vehicle capacity utilization and distribution cost
Vehicle capacity utilization analysis revealed that routes 26, 27, 59, and 61 in the morning distribution had a utilization capacity of less than 50%, while route 9 exhibited the highest capacity utilization due to its strategic location. On the other hand, routes 6, 7, 28, 45, and 58 had a vehicle utilization capacity of less than 25% in the evening distribution, with route 61 having the highest utilization capacity of 60%. The variation in vehicle usage capacity in the evening was attributed to using the same fleet for both morning and evening distributions, despite the significantly lower demand in the evening, resulting in relatively low vehicle utilization capacity. Routes 6 and 7 had the lowest capacity utilization in the evening, as they employed the same fleet with less evening demand, as described in .
Meanwhile, the distribution cost analysis for morning distribution in the old Bhopal zone considered vehicle operating costs and idle time costs. Among the 24 routes, route 28 had the highest total distribution cost of 518 rupees, and route number 37 had the lowest cost of 256 rupees. The average total distribution cost was found to be 343 rupees, with a total of 8232.8 rupees for all routes. The distribution cost per kilometer ranged from a maximum of 39 paisa for route number 63 to a minimum of 7 paisa for route number 37, with an average of 17 paisa per kilometer. For evening routes, route number 28 had the highest total distribution cost at 526.2 rupees, while route number 11 had the lowest at 262.2 rupees. The total distribution cost for all evening routes summed up to 7348.4 rupees. The distribution cost per kilometer varied from a maximum of 1.5 rupees for route number 7 to a minimum of 2 paisa for routes 10, 11, 15, and others, with an average of 8 paisa per kilometer, as described in .
5.2.3. Load factor
The load factor is calculated as the ratio of carrying demand to that of maximum capacity both in liters. Out of 24 distribution routes in the morning, routes 10, 26, 27, 59, and 61 had the lowest load factors while routes 9 had the highest load factors. Due to low demand, routes 10, 26, 27, 59, and 61 have a minimum load factor, whereas route 9 has a high load factor. Routes 6, 7, 43, and 45 of the 21 evening distribution routes have the lowest load factors because of the low demand. This happens because for the provided routes, the fleet utilization in the evening distribution is the same as it is in the morning distribution, despite the fact that the demand is lower in the evening than it is in the morning, resulting in a very low load factor. Routes 6, 7, 43, and 45 have the lowest load factors due to the lack of demand.
5.3. Scenario building
The old Bhopal zone was evaluated, and the problematic routes were identified. Using ArcGIS software to overlay all the routes, it was determined that some of them had extremely high dead kilometers, which was the key factor contributing to high distribution costs. Considering the carrying capacity of the fleet and the demand for milk along that particular route, certain routes with overlapping dead kilometers were combined in an effort to reduce distribution costs. The routes were categorized based on a few assumptions, including the first that supply and demand are equal. Secondly, the entire distance should not exceed 30 kilometers. Thirdly, the combined route should have less dead kilometers than the original routes, and fourth, the same fleet should be employed. The new routes were organized into 4 groups: Morning-N, Evening-1, Evening-2, Morning & Evening. In the ‘Morning-N’ group, 16 new routes were formed after merging the existing routes to reduce dead kilometers. These routes are exclusively utilized in the morning. The same ‘Morning-N’ routes (16) were utilized in the ‘Evening-1’ group to meet the demand solely in the evening. After combining the previous routes in the ‘Evening-2’ group and taking into account evening distribution individually, 12 additional routes were discovered. The distribution of milk to retail outlets will only take place in the morning in the last ‘Morning + Evening’ group because there is very little demand for milk in the evening. As a result, the routes for morning and evening distribution were combined, and there will be no fleet utilization in the evening. Under this category, all 24 routes will be considered. The detailed distribution of each group is described in . Three scenarios were developed based on the aforementioned four groups of routes in order to lower distribution costs and reduce dead kilometers. Scenario 1 combines the routes from the ‘Morning-N’ and ‘Evening-1’ groupings. Scenario 2 is the combination of the ‘Morning-N’ and ‘Evening-2’ groups of routes. And lastly, scenario 3 includes ‘Morning and Evening’ groups of routes.
Table 4. Distribution scenario building.
5.3.1. Scenario comparison
The three scenarios were built, and comparisons were made between them as well as with the existing scenario of dairy evaluation. Following is a breakdown of the key differences between the scenarios:
Scenario 1: Combines the routes from the ‘Morning-N’ and ‘Evening-1’ groupings.
Scenario 2: Involves the combination of the ‘Morning-N’ and ‘Evening-2’ groups of routes.
Scenario 3: Includes routes from both morning and evening distribution groups.
Total kilometers, total distribution cost (in rupees), total route demand (in liters), total route vehicle capacity (in liters), and vehicle capacity utilization (in percentage) were the key parameters used for the comparison of scenarios. The comparison will provide a clearer picture of how the scenarios differ in terms of distribution costs, total kilometers, and fleet utilization. After comparison, it was concluded that the overall distance is decreased by 17% in scenario 1, 20% in scenario 2, and 45% in scenario 3. Distribution costs are lowered by 23% in scenario 1, 30% in scenario 2, and 30% in scenario 3. Vehicle capacity (liter) is lowered by 26% in scenario 1, 36% in scenario 2, and 47% in scenario 3. Vehicle capacity utilization increases by 16% in scenario 1, 26% in scenario 2, and 42% in scenario 3. Moreover, it was also found that scenarios 1,2 and 3 were better performing than the existing scenario. The detailed scenario comparison is described in .
Table 5. Scenario comparison.
6. Discussion
Old Bhopal routes are proposed in such a way that routes are eliminated as well as combined focusing on minimizing the dead kilometer, the total kilometer, total distribution cost in rupees, total vehicle capacity in liters, and improving the vehicle utilization capacity which is calculated in percentage. The total kilometer of the proposed routes is decreased by removing overlapping routes and minimizing dead kilometers. The supply of milk remains the same along the distribution routes, due to the constant demand in both the proposed and existing networks. As the number of routes decreases overall, so does the carrying capacity of vehicles on newly proposed routes. The proposed routes focus on the distribution of milk in the old city Bhopal region during morning and evening distribution. The details of proposed morning and evening routes are mentioned in .
Table 6. Proposed evening distribution routes.
Table 7. Proposed morning distribution routes.
6.1. Proposed old bhopal morning distribution routes
The proposed routes include 16 total routes of distribution in the morning. Eight routes were eliminated from the proposed morning distribution route when it is compared to the existing routes. In comparison to existing routes, the proposed routes cost around 2100 rupees less than that of existing routes. The total kilometers in the proposed routes are likewise 75 kilometers less. The proposed routes had a higher vehicle capacity utilization of 81%, compared to the previous scenario’s morning distribution routes in Bhopal’s old city, where the vehicle capacity utilization was about 60%. In the proposed routes, route number 7 which is the combination of old route 7 and route 63 is the longest with a total distance of approximately 29 kilometers which is still less than the constraints, which was considered as 30 kilometers. Route 15 is the lowest kilometer route with a total kilometer of 8.5 kilometers this route is the same as the old route. The maximum distribution cost in all of the morning distribution routes is of route number 7 which is the combination of old route 7 and route 63, the cost is 558.65 rupees. The minimum cost of distribution in proposed routes is route number 15, which is 279.8 rupees. The maximum demand in proposed routes is also of route number 7, which is 5865 liters. The demand of route 7 and route 63 in the existing scenario is combined and a fleet with a carrying capacity of 6000 liters is used to distribute the combined demand. The minimum demand in proposed routes of morning distribution is route 25, which is 2000 liters. The proposed route is also the same as that of the existing route. The demand is fulfilled by using a vehicle carrying a capacity of 3500 liters.
6.2. Proposed old bhopal evening distribution routes
When the proposed route is compared with the existing routes, 9 routes are reduced in the proposed routes of evening distribution. The cost of distribution in the proposed routes is approximately 2563 rupees less than that of existing routes. The vehicle capacity utilization in proposed routes is increased to 55 % whereas in the existing scenario the vehicle capacity utilization was around 29% in evening distribution routes of old city Bhopal. In the proposed evening routes, route number 6 which is the combination of old route 6 and route 36 are the longest with a total distance of approximately 29 kilometers which is still less than the constraints. Route 57 is the shortest route, with a total length of 14 kilometers. It is the same as the old route. The maximum distribution cost in all of the evening distribution routes is of route number 6 which is the combination of old route 6 and route 36, the cost is 576.6 rupees. The minimum distribution cost in proposed routes is of route number 43, which is 330 rupees. The new proposed route 43 is the combination of old route 43 and route number 59. The maximum demand in proposed routes is also of route number 6, which is the combination of old, route 6, and route 36, and also for route number 35 new proposed which is the combination of old route 35 and route 61, the demand is 3100 liters each. The demand of route 6 and route 36 in the existing scenario is combined, and the demand of route 35 and route 61 is combined and a fleet with a carrying capacity of 3500 liters is used to distribute the combined demand for each route. The minimum demand in proposed routes is of route 60, which is 900 liters. This proposed route is also the same as that of the existing route. The demand is fulfilled by using a vehicle carrying a capacity of 3500 liters.
Conclusion and directions for future studies
The study of the urban distribution of dairy supply in the Old Bhopal zone, Madhya Pradesh, India, has yielded valuable insights into optimizing the dairy supply chain. By analyzing key performance indicators (KPIs) this study aimed to identify areas for improvement and cost optimization in the distribution process. The analysis of various KPIs, including distribution time, distance traveled, vehicle capacity utilization, average effective kilometer, vehicle operating cost, and total demand, revealed substantial opportunities for improvement. The application of ArcGIS software and the scenario-building approach has been pivotal in addressing the complexities of urban distribution challenges within the dairy industry, specifically in the Old Bhopal zone. The selection of ArcGIS facilitated a comprehensive assessment of distribution routes, enabling the identification of inefficiencies and opportunities for optimization. Additionally, the scenario-building methodology provided a structured framework for evaluating alternative distribution strategies, leading to cost reduction and enhanced efficiency. The proposed routes, aimed at eliminating redundancies and reducing dead kilometers, led to a notable decrease in the total kilometers of the proposed routes. This optimization resulted in cost savings and improved vehicle utilization capacity, highlighting the potential for increased efficiency in the distribution of milk. The identification of problematic routes and the subsequent scenario-building process address critical gaps in the current understanding of urban distribution challenges within the dairy industry. By providing insights into route optimization and cost reduction, our methodology contributes significantly to the existing body of knowledge. The findings underscore the significance of optimizing the distribution of milk in the Old Bhopal region to enhance sustainability and profitability in the dairy supply chain. The proposed routes, if implemented, have the potential to streamline the distribution process and reduce operational costs. This study contributes to the broader understanding of logistics optimization in the dairy industry, emphasizing the importance of efficient distribution practices for cost-effectiveness and reduced environmental impact. Acknowledging the scope of this study is confined to the Old Bhopal zone, it is imperative to recognize its limitations. Future research should extend the investigation to other zones within Bhopal and explore urban distribution challenges in different cities with similar as well as diverse characteristics. Additionally, while the focus of the study has been on the distribution of milk, expanding the research to analyze other dairy products and perishable commodities could provide a more comprehensive understanding of supply chain efficiency and cost optimization strategies for a broader range of products.
Disclosure statement
No potential conflict of interest was reported by the author(s).
References
- Álvarez, R., Calvo, R., Peña, M. M., & Domingo, R. (2009). Redesigning an assembly line through lean manufacturing tools. The International Journal of Advanced Manufacturing Technology, 43(9–10), 949–18. https://doi.org/10.1007/s00170-008-1772-2
- Boysen, N., & Bock, S. (2011). Scheduling just-in-time part supply for mixed-model assembly lines. European Journal of Operational Research, 211(1), 15–25. https://doi.org/10.1016/j.ejor.2010.10.029
- Brar, G. S., & Saini, G. (2011). Milk run logistics: Literature review and directions. Proceedings of the World Congress on Engineering, London, UK, July 6–8, 2011.
- DAHD. (2018). National action plan for dairy development—vision 2022. Department of Animal Husbandry and Dairying, Government of India.
- Domingo, R., Alvarez, R., Peña, M. M., Calvo, R., & Karjalainen, I. (2007). Materials flow improvement in a lean assembly line: A case study. Assembly Automation, 27(2), 141–147. https://doi.org/10.1108/01445150710733379
- FAO. (2017, November 11). Food Outlook Biannual Report on Global Food Markets. Food Outlook – Biannual Report on Global Food Markets. https://doi.org/10.4060/cb7491en
- FAO. (2020). World Milk Production Trends in 2018. http://www.fao.org/3/ca8341en/CA8341EN.pdf
- GoI. (2014). Key indicators of situation of agricultural households in India. NSS 70th Round. National Sample Survey Office, Ministry of Statistics and Programme Implementation, Government of India, New Delhi.
- Goli, A. (2023). Integration of blockchain-enabled closed-loop supply chain and robust product portfolio design. Computers and Industrial Engineering, 179, 109211. https://doi.org/10.1016/j.cie.2023.109211
- Goli, A., Ala, A., & Hajiaghaei-Keshteli, M. (2023). Efficient multi-objective meta-heuristic algorithms for energy-aware non-permutation flow-shop scheduling problem. Expert Systems with Applications, 213, 213. https://doi.org/10.1016/j.eswa.2022.119077
- Goli, A., Ala, A., & Mirjalili, S. (2023). A robust possibilistic programming framework for designing an organ transplant supply chain under uncertainty. Annals of Operations Research, 328(1), 493–530. https://doi.org/10.1007/s10479-022-04829-7
- Goli, A., Golmohammadi, A. M., & Verdegay, J. L. (2022). Retracted article: Two-echelon electric vehicle routing problem with a developed moth-flame meta-heuristic algorithm. Operations Management Research, 15(3–4), 891–912. https://doi.org/10.1007/s12063-022-00298-0
- Goli, A., & Tirkolaee, E. B. (2023). Designing a portfolio-based closed-loop supply chain network for dairy products with a financial approach: Accelerated benders decomposition algorithm. Computers and Operations Research, 155, 155. https://doi.org/10.1016/j.cor.2023.106244
- Haksever, C., Render, B., Russell, R., & Murdick, R. (2000). Vehicle routing and scheduling, service management and operations (2nd ed.). Prentice Hall. Upper Saddle River.
- Hao, Q., & Shen, W. (2008). Implementing a hybrid simulation model for a Kanban-based material handling system. Robotics and Computer-Integrated Manufacturing, 24(5), 635–646. https://doi.org/10.1016/j.rcim.2007.09.012
- Kilic, H. S., Durmusoglu, M. B., & Baskak, M. (2012). Classification and modeling for in-plant milk-run distribution systems. The International Journal of Advanced Manufacturing Technology, 62(9–12), 1135–1146. https://doi.org/10.1007/s00170-011-3875-4
- Lok Sabha. (2019). Statement by Ministry of Fisheries, Animal Husbandry and Dairying.
- Michel Baudin. (2004). Lean logistics: The nuts and bolts of delivering materials and goods. Productivity Press.
- MoSPI. (2021). National accounts statistics 2020. Central Statistical Organization. Ministry of Statistics and Program Implementation. Ministry of Statistics and Programme Implementation, Government of India,
- Nanda Kumar, T., Das, S., & Gulati, A. (2022). Dairy value chain. https://doi.org/10.1007/978-981-33-4268-2_6
- Nemoto, T., Hayashi, K., & Hashimoto, M. (2010). Milk-run logistics by Japanese automobile manufacturers in Thailand. Procedia - Social & Behavioral Sciences, 2(3), 5980–5989. https://doi.org/10.1016/j.sbspro.2010.04.012
- Özturk Calik, E. (2022). Research design: Qualitative, quantitative and mixed methods approaches 4th, eds Creswell, J. W. CA: Sage. (2014). ELT Research Journal, (2), Vol. 11.
- Patel, D., Patel, M. B., & Vadher, J. (2017). Design and development of milk-run material supply system with time windows and simultaneous pickups and deliveries. IJIRST-International Journal for Innovative Research in Science & Technology|, 4(2). https://www.ijirst.org
- Patel, D., Patel, M. B., & Vadher, J. A. (2014). Implementation of milk run material supply system in vehicle routing problem with simultaneous pickup and delivery. International Journal of Application or Innovation in Engineering & Management (IJAIEM), 3(11), 122–124. https://www.semanticscholar.org/paper/Implementation-of-milk-run-material-supply-system-Patel-Patel/370d90021921e6303daa8aeb6bed291d8baeff8a
- Sanchi, B. (2021). Milk union progress report. Bhopal Sahakari Dugdh Sangh Maryadit. https://www.sanchibhopal.com/report.aspx
- Satoglu, S. I., & Sahin, I. E. (2013). Design of a just-in-time periodic material supply system for the assembly lines and an application in electronics industry. The International Journal of Advanced Manufacturing Technology, 65(1–4), 319–332. https://doi.org/10.1007/s00170-012-4171-7
- Vaidyanathan, B. S., Matson, J. O., Miller, D. M., & Matson, I. E. (1999). A capacitated vehicle routing problem for just-in-time delivery. IIE Transactions (Institute of Industrial Engineers), 31(11), 1083–1092. https://doi.org/10.1080/07408179908969909
- You, Z., & Jaio, Y. (2014). Development and application of milk-run distribution systems in the express industry based on saving algorithm. Mathematical Problems in Engineering, 2014, 1–6. https://doi.org/10.1155/2014/536459