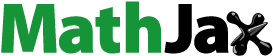
Abstract
Objective
Artificial intelligence (AI) offers new opportunities for objective quantitative measurements of imaging biomarkers from positron-emission tomography/computed tomography (PET/CT). Clinical image reporting relies predominantly on observer-dependent visual assessment and easily accessible measures like SUVmax, representing lesion uptake in a relatively small amount of tissue. Our hypothesis is that measurements of total volume and lesion uptake of the entire tumour would better reflect the disease`s activity with prognostic significance, compared with conventional measurements.
Methods
An AI-based algorithm was trained to automatically measure the prostate and its tumour content in PET/CT of 145 patients. The algorithm was then tested retrospectively on 285 high-risk patients, who were examined using 18F-choline PET/CT for primary staging between April 2008 and July 2015. Prostate tumour volume, tumour fraction of the prostate gland, lesion uptake of the entire tumour, and SUVmax were obtained automatically. Associations between these measurements, age, PSA, Gleason score and prostate cancer-specific survival were studied, using a Cox proportional-hazards regression model.
Results
Twenty-three patients died of prostate cancer during follow-up (median survival 3.8 years). Total tumour volume of the prostate (p = 0.008), tumour fraction of the gland (p = 0.005), total lesion uptake of the prostate (p = 0.02), and age (p = 0.01) were significantly associated with disease-specific survival, whereas SUVmax (p = 0.2), PSA (p = 0.2), and Gleason score (p = 0.8) were not.
Conclusion
AI-based assessments of total tumour volume and lesion uptake were significantly associated with disease-specific survival in this patient cohort, whereas SUVmax and Gleason scores were not. The AI-based approach appears well-suited for clinically relevant patient stratification and monitoring of individual therapy.
Introduction
Positron emission tomography/computed tomography (PET/CT) is increasingly being used in several types of malignancies, including prostate cancer [Citation1–4]. PET/CT has been proposed as the sole imaging modality for primary lymph node and bone staging of prostate cancer based on higher sensitivity and specificity compared to conventional imaging techniques [Citation5]. Detection of intraprostatic tumour localisation with PET/CT to guide targeted biopsy has also been suggested [Citation6].
However, the analysis and clinical reports for PET/CT still rely predominantly on visual assessment and semi-automated measurements; probably due to the fact that proper quantification of disease processes is time-consuming and mainly based on manual procedures. Visual assessment is associated with inter-observer variability both in clinical reporting and research. Further, the most easily obtained measurement available is the maximum standardised uptake value (SUVmax). The prognostic significance of the sum of SUVmax in patients with prostate cancer was presented several years ago [Citation7], though the clinical value of this measurement has been shown to be relatively limited, especially when it comes to evaluation of tumour response [Citation8,Citation9]. One reason for this could be that SUVmax may be biased by disproportionate uptake in a relatively small amount of tissue, and may not correlate to total disease burden.
Meanwhile, artificial intelligence (AI) offers new opportunities to analyse scans and provide objective, observer-independent measurements of tumour detection, segmentation and classification in a range of malignancies [Citation10–12]. The bone scan index (BSI) is an AI-based two-dimensional approach used to quantify skeletal metastasis in bone scan scintigraphy, which has recently been validated and approved for clinical use [Citation13,Citation14]. It provides important prognostic information, not contained in the visual reports. AI-based three-dimensional methods applied to whole-body PET/CT could most likely have an even greater clinical impact [Citation15].
A recent study developed an AI-based method for automated analysis of the prostate in PET/CT. The resulting measurements of lesion uptake in the tumour were associated with overall survival in a group of patients with high-risk prostate cancer and known bone metastases [Citation14,Citation15]. In the current study, we take this approach one step further by applying an improved AI-based method to a group of high-risk prostate cancer patients considered for curative treatment at the time of staging and using disease-specific rather than overall survival as the endpoint.
Thus, the aim of this retrospective study was to investigate the association between AI-derived measurements of tumour volume and lesion uptake and disease-specific survival, compared to the prognostic value of other clinical data including, age, Gleason score, prostate specific antigen (PSA), and treatment.
Materials and methods
Patients
The AI-algorithm was trained and validated using 143 PET/CT scans from a recent study [Citation16]. The characteristics of the patient groups have been described in detail elsewhere [Citation16,Citation17].
The algorithm was then applied to a separate test set of 304 prostate cancer patients, who were examined using 18F-choline PET/CT for primary staging (). The PET/CT examinations in this set were performed at another institution and with different cameras than the training/validation set in [Citation16]. The patients had been part of previous studies with the following inclusion criteria: newly diagnosed high-risk prostate cancer patients (defined as PSA above 20 ng/ml, and/or clinical tumour stage T3, and/or Gleason score 8–10) with normal or inconclusive bone scintigraphy [Citation18,Citation19]. The exclusion criteria were hormonal therapy before PET/CT, or PSA ≥150 ng/ml. The PET/CT examinations were performed between April 2008 and July 2015, the results of which were not part of the exclusion criteria. Clinical information was collected from the local medical records up to April 2019. The treatment for each patient was selected by the treating urologist in consultation with the patient. In general, patients with no evidence of metastatic disease received curative treatment (radical prostatectomy or radiation therapy), while patients that had metastatic disease received palliative treatment (androgen deprivation therapy) based on the PET/CT findings. The clinical characteristics of the 285 patients are shown in .
Table 1. Clinical characteristics of patients in the test set (n = 285).
These retrospective studies were approved by the Research Ethical Review Board at the University of Lund (EPN LU 552/2007 and 2016/61) and the Regional Ethics Review Boards of Sweden (295-08 and 2016/103) and Denmark (3-3013-1692/1).
Imaging protocols
Training/validation set
Training and validation data were obtained using two different PET/CT scanners and protocols: an integrated PET/CT camera (Siemens Biograph 64 Truepoint), with a low dose CT scan (64-slice helical, 120 kV, 30 mAs, and CT slice thickness 5 mm) and a PET/CT scan (Discovery VCT, GE Healthcare), with a contrast-enhanced CT scan (64-slice helical, 120 kV, ‘smart mA’ maximum 400 mA, and CT slice thickness 3.75 mm), approximately 60 min after administration of 4 MBq/kg of 18F-choline. Both PET/CT scans were obtained from the base of the skull to mid-thigh [Citation16].
Test set
PET/CT scans were acquired by means of an integrated PET/CT system (Philips Gemini TF, Philips Medical Systems, Cleveland, OH, USA) at the Centre for Medical Imaging and Physiology, Skåne University Hospital in Lund or Malmö [Citation18,Citation19].
Patients fasted for 4 h before 18F-choline injection. Whole-body PET was acquired 1–1.5 h after intravenous injection of 4 MBq/kg (max. dose 400 MBq) of 18F-fluorocholine with 2 min per bed position. A diagnostic quality CT scan was performed immediately prior to the PET scan with 1000 ml oral contrast given 60 min before the scan, and intravenous contrast (Omnipaque 350 mg I/mL) given by an automatic injection pump with an injection speed of 2.5 mL/s. A multidetector spiral CT scanner was used, with 5 mm reconstructed slice thickness, rotation speed 0.75 s, 120 kV and with high-beam tube current modulation (120–300 mA) based on the patient’s total body mass.
Image processing and interpretation
AI-model
The model from a recent study [Citation20] was used to automatically segment the prostate gland. This model uses both the PET image and the CT image to get an accurate segmentation and position of the prostate gland even when there is misalignment between the PET and the CT image.
Imaging biomarkers
The standardised uptake value (SUV) of PET images was automatically calculated for the whole prostate volume. Each voxel in the region classified as prostate with an SUV above 2.65 was considered abnormal [Citation21]. The AI included voxels of all the prostate lesions that could represent multifocal disease, even if these lesions were located separately from each other. In order to remove uptake leaking from surrounding tissue, Meyer’s flooding algorithm was used. This assigns each high abnormal voxel to a local maximum in the PET image. If this local maximum lies outside the region segmented as the prostate, we assume that the abnormal voxel is actually due to leakage, and we change the classification to normal.
Automated measures were obtained for the total volume of the gland in ml, maximum SUV within the prostate (SUVmax), mean SUV of voxels considered abnormal (SUVmean) and volume of abnormal voxels in ml (lesion VOLUME). To reflect total lesion uptake (TLU), the product SUVmean × VOLUME was calculated. Finally, the quotient of VOLUME related to the total volume of the prostate gland was defined as FRACTION.
Statistical analysis
Associations between automated PET/CT measurements, age, PSA, Gleason score and disease-specific survival were investigated using a univariate Cox proportional hazards regression model. Disease-specific survival was calculated from the date of PET/CT scan to the date of prostate cancer death or last follow-up. Hazard ratios (adjusted for type of treatment: curative or palliative) and 95% confidence intervals (CI) were estimated (bivariable analysis). Univariate analysis for patients with metastatic disease (defined as N1and/or M1) was also performed. Hazard ratio accounts for one unit change of each of the automated PET variables, based on a median follow-up of five years. The level of significance was set at 0.05. The patients for the Kaplan-Meier analysis were grouped according to the median value of the variable of interest. The patients were divided into two different groups based on their Gleason score (Gleason ≤ 7 and Gleason > 7). SPSS version 25 (IBM, Armonk, NY, USA) was used for statistical analysis.
Results
Patient group and death rate
The algorithm failed to perform automated measurements in 14 (5%) patients due to artefacts, caused by either hip prosthesis, peroral contrast in the bowel or tracer radioactivity in the urinary bladder. Five patients were excluded due to missing follow-up (). Of the remaining 285 prostate cancer patients, 219 (77%) received curative treatment.
Figure 1. Study selection process. The Standards for Reporting Studies of Diagnostic Accuracy (STARD) flow chart.

Five patients were considered censored (death of other causes) before the first prostate cancer death, making no contribution to the estimation and are thus not used.
Out of a total of 285 patients, 23 died of prostate cancer (8%) with a median survival time of 3.8 years (IQR 2.8–4.8) during a median follow-up of five years (IQR 4.4–5.7). Of these 23 patients, 18 (78%) received palliative treatment.
Disease-specific survival in association with imaging biomarkers
The univariate Cox analysis showed that three of the volumetric measurements (lesion VOLUME, TLU and FRACTION) made automatically by the AI-based algorithm, as well as age, were significantly associated with disease-specific survival in contrast to the other PET/CT measurements (SUVmax and SUVmean), PSA (logarithmic) and Gleason score for patients receiving palliative treatment as well as for all patients after adjusting for treatment. Further, the univariate analysis showed that patients with metastatic disease had worse survival than those with local cancer, even if they receive curative treatment (). The Kaplan-Meier survival was similar for patients with a Gleason score above and below 7. Patients with a log-PSA value above the median (3.04) tended towards worse survival during the whole follow-up time, which did not reach statistical significance (p = 0.07). The survival curves of patients with SUVmax above and below the median crossed each other and after 6 years of follow-up, there was a tendency towards worse survival for patients with SUVmax above the median value (6.5). Finally, the Kaplan-Meier survival was significantly worse for patients with lesion VOLUME above the median value (18.1 mL, p < 0.001, ).
Figure 2. Kaplan-Meier survival plots of the patients in the test group. The figure compares the survival curves between Gleason score, prostate SUVmax, PSA and lesion VOLUME (n = 285). The upper curves of each graph represent the proportion of patients with values equal or below the median level of the variable of interest (or Gleason ≤ 7 respectively), whereas the lower curves represent the proportion of patients with values above the median level of the variable of interest (or Gleason > 7 respectively). p-value was calculated using the log-rank test.
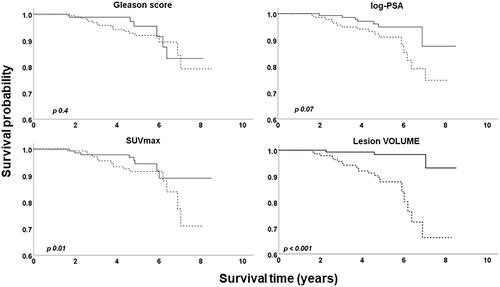
Table 2. Associations between disease-specific survival and AI-based PET/CT measurements of biomarkers, as well as clinical data using univariate proportional regression analysis.
Discussion
The AI-based image analysis was able to accurately identify the prostate in 95% of the PET/CT scans and the automated quantification of total prostate tumour burden in these patients was significantly associated with disease-specific survival for those who received non-curative treatment. Although not specifically studied, it is possible that this could be used as an objective marker for selecting which patients with metastatic disease should receive more intensive treatment.
The lesion volume, the tumour fraction of the prostate volume, and the total lesion uptake, based on the AI algorithm resulting in a 3 D-based image analysis, seem to reflect the total tumour infiltration of the gland. The volume-based measurements were better prognosticators than SUVmax, probably because they provide more information about the total tumour than only a small number of cancer cells. Similar results in relation to progression-free survival have been demonstrated in the manual analysis of 11C-choline PET/MRI [Citation22].
The application of an AI algorithm to the prostate and its association with overall survival with a high level of reliability compared to experienced radiologists (Dice-Sørensen coefficient 0.78–0.79) has previously been shown [Citation16]. This AI method has been further developed as described in a recent study, which showed a significant correlation between the automated volumetric measurements of the prostate and the manual segmentations as well as the gland’s weight from the histopathological specimens [Citation20]. In the present study, the improved version of the algorithm was used and the number of patients was increased compared to the results from Polymeri et al. [Citation16]. At the same time, the test set consisted of a large number of patients from another institution that used a different PET/CT camera, increasing the accuracy and generalisability of the algorithm. Further, disease-specific rather than overall survival was used as the endpoint, while bivariable analysis was performed after adjusting for treatment. A prospective study with prostate-specific radiotracers comparing the automated measurements with the histopathological results or other known prognostic factors could be considered in the future.
Other volume-based clinical measures, such as staging by transrectal ultrasound (TRUS), have been shown to be important tools for cancer staging and prognosis [Citation23]. However, the heterogeneity of cancer itself [Citation24] makes the volumetric lesion analysis in TRUS suboptimal and examiner-dependent. The Gleason Score for the tumour is another known prognostic factor, however, the grading is highly subjective and its reproducibility is not always perfect [Citation25,Citation26], with the biopsies only sampling a small part of the tumour. In contrast, the automated analysis of a PET/CT scan provides information about the whole tumour and may offer a more consistent result. Moreover, other well-known pre-treatment risk stratification tools for prostate cancer have been analysed and compared in a recent study, showing that they can contribute to treatment decision making in different ways [Citation27]. Analysis of imaging PET/CT measurements reflecting the tumour infiltration of the prostate could be a compatible tool in establishing new prognostic variables. Other prospective studies are needed in the future in order to analyse PET/CT variables in combination with other known prognostic factors.
The main strength of the present study is the large proportion of scans that were successfully analysed by the AI algorithm, including patients with unilateral hip prostheses. Further training of the algorithm on a group of patients with anatomical variations in the pelvic region could, thus, potentially improve its performance. Moreover, the potential future use of metal artefacts’ reduction algorithms could further improve the segmentation and quantification process by AI-based algorithms.
Limitations
The most important limitations of the study are the relatively short follow-up time, with few terminal events, and the use of historical PET/CT scans, which were part of the staging workup and thus influenced the treatment selection. The local lesion uptake in the prostate was not, however, considered at the time of treatment, as the original aim of the PET/CT scans was to detect metastasis. In addition, the patients in the test group were examined by 18F-choline-PET, due to the availability of the scans at the time of this retrospective study. Other prostate cancer-specific tracers have been increasingly used in the last few years with a significant improvement in sensitivity and specificity, if compared with Choline-based tracers, such as prostate-specific membrane antigen radiopharmaceuticals [Citation3,Citation28,Citation29], which are being preferred. However, even with the use of radiolabeled choline in the present study, the AI algorithm showed promising results. Further, the long-time use of this tracer in several medical centres gives the advantage of conducting studies with longer follow-up. To the best of our knowledge, the prognostic value of AI-derived volumetric prostate tumour measurements in PET/CT is still undefined. The use of more prostate cancer-specific tracers in future studies may thus further improve the prognostic significance and clinical value of the algorithm.
Moreover, the patient cohort for the present study consisted of a rather heterogeneous group of individuals with different T-stages and different treatments. By definition, these factors alone could be important for prognosis, although that was not found here which is likely due to all the patients included having high-risk cancer [Citation27]. Further, the selection of patients with high PSA values and tumour staging limits the generalisability of our study to patients with advanced prostate cancer disease. Moreover, the small number of prostate cancer-specific events during the follow-up period prevented us from performing multivariate analyses [Citation30]. A larger patient cohort including more events could be considered in future studies.
In conclusion, fully automated AI-based measurements of imaging biomarkers on PET/CT reflecting prostate tumour burden showed prognostically significant results. These measurements may be a clinically valuable tool in the future for patient stratification and monitoring of individual therapy.
The AI tool developed in this project is available upon reasonable request for research purposes at www.recomia.org.
Ethical considerations
The following ethical approvals were obtained: Research Ethical Review Board at the University of Lund (EPN LU 552/2007 and 2016/61) and the Regional Ethics Review Boards of Sweden (295-08 and 2016/103) and Denmark (3-3013-1692/1).
Abbreviations | ||
BSI | = | bone scan index |
CNN | = | convolutional neural network |
PET | = | positron emission tomography |
CT | = | computed tomography |
PSA | = | prostate specific antigen |
SDI | = | Sørensen-Dice index |
SUV | = | standardized uptake value. |
Acknowledgements
We would like to thank Anna Grimby-Ekman for her valuable input on the appropriate statistical method used in our manuscript.
Disclosure statement
LE was employed as Scientific Director by EXINI Diagnostics AB (Lund, Sweden).
Data availability statement
The data that support the findings of this study are available from the corresponding author, [EP], upon reasonable request.
Additional information
Funding
References
- Perera M, Papa N, Roberts M, et al. Gallium-68 prostate-specific membrane antigen positron emission tomography in advanced prostate cancer-updated diagnostic utility, sensitivity, specificity, and distribution of prostate-specific membrane antigen-avid lesions: a systematic review and meta-analysis. Eur Urol. 2020;77(4):403–417.
- Giovacchini G, Guglielmo P, Mapelli P, et al. 11C-choline PET/CT predicts survival in prostate cancer patients with PSA1 NG/ml. Eur J Nucl Med Mol Imaging. 2019;46(4):921–929.
- Evangelista L, Briganti A, Fanti S, et al. New clinical indications for (18)F/(11)C-choline, new tracers for positron emission tomography and a promising hybrid device for prostate cancer staging: a systematic review of the literature. Eur Urol. 2016;70(1):161–175.
- Kitajima K, Nakajo M, Kaida H, et al. Present and future roles of FDG-PET/CT imaging in the management of gastrointestinal cancer: an update. Nagoya J Med Sci. 2017;79(4):527–543.
- Esen T, Kilic M, Seymen H, et al. Can Ga-68 PSMA PET/CT replace conventional imaging modalities for primary lymph node and bone staging of prostate cancer? Eur Urol Focus. 2020;6(2):218–220.
- Bodar YJL, Jansen B, Van Der Voorn P, et al. Detection of intraprostatic tumour localisation with 18F-PSMA PET/CT compared to radical prostatectomy specimens: is PSMA-targeted biopsy feasible? –the DeTeCT trial–. Eur Urol Suppl. 2019;18(10):e3377–e3378.
- Jadvar H, Desai B, Ji L, et al. Baseline 18F-FDG PET/CT parameters as imaging biomarkers of overall survival in castrate-resistant metastatic prostate cancer. J Nucl Med. 2013;54(8):1195–1201.
- Wahl RL, Jacene H, Kasamon Y, et al. From RECIST to PERCIST: evolving considerations for PET response criteria in solid tumors. J Nucl Med. 2009;(Suppl 1):122S–150S.
- Young H, Baum R, Cremerius U, et al. Measurement of clinical and subclinical tumour response using [18F]-fluorodeoxyglucose and positron emission tomography: review and 1999 EORTC recommendations. Eur J Cancer. 1999;35(13):1773–1782.
- Schwyzer M, Ferraro DA, Muehlematter UJ, et al. Automated detection of lung cancer at ultralow dose PET/CT by deep neural networks - initial results. Lung Cancer. 2018;126:170–173.
- Zhong Z, Kim Y, Plichta K, et al. Simultaneous cosegmentation of tumors in PET-CT images using deep fully convolutional networks. Med Phys. 2019;46(2):619–633.
- Huang B, Chen Z, Wu PM, et al. Fully automated delineation of gross tumor volume for head and neck cancer on PET-CT using deep learning: a dual-center study. Contrast Media Mol Imaging. 2018;2018:1–12.
- Anand A, Morris MJ, Larson SM, et al. Automated bone scan index as a quantitative imaging biomarker in metastatic castration-resistant prostate cancer patients being treated with enzalutamide. EJNMMI Res. 2016;6(1):23
- Armstrong AJ, Anand A, Edenbrandt L, et al. Phase 3 assessment of the automated bone scan index as a prognostic imaging biomarker of overall survival in men with metastatic Castration-Resistant prostate cancer: a secondary analysis of a randomized clinical Trial. JAMA Oncol. 2018;4(7):944–951.
- Hoilund-Carlsen PF, Edenbrandt L, Alavi A. Global disease score (GDS) is the name of the game!. Eur J Nucl Med Mol Imaging. 2019;46(9):1768–1772.
- Polymeri E, Sadik M, Kaboteh R, et al. Deep learning-based quantification of PET/CT prostate gland uptake: association with overall survival. Clin Physiol Funct Imaging. 2020;40(2):106–113.
- Poulsen MH, Petersen H, Hoilund-Carlsen PF, et al. Spine metastases in prostate cancer: comparison of technetium-99m-MDP whole-body bone scintigraphy, [(18) F]choline positron emission tomography(PET)/computed tomography (CT) and [(18) F]NaF PET/CT. BJU Int. 2014;114(6):818–823.
- Kjolhede H, Ahlgren G, Almquist H, et al. ¹(8)F-fluorocholine PET/CT compared with extended pelvic lymph node dissection in high-risk prostate cancer. World J Urol. 2014;32(4):965–970.
- Kjolhede H, Almquist H, Lyttkens K, et al. Pre-treatment (18)F-choline PET/CT is prognostic for biochemical recurrence, development of bone metastasis, and cancer specific mortality following radical local therapy of high-risk prostate cancer. Eur J Hybrid Imaging. 2018;2(1):16.
- Mortensen MA, Borrelli P, Poulsen MH, et al. Artificial intelligence-based versus manual assessment of prostate cancer in the prostate gland: a method comparison study. Clin Physiol Funct Imaging. 2019;39(6):399–406.
- Reske SN, Blumstein NM, Neumaier B, et al. Imaging prostate cancer with 11C-choline PET/CT. J Nucl Med. 2006;47(8):1249–1254.
- Tseng JR, Yang LY, Lin YC, et al. Metabolic volumetric parameters in 11C-Choline PET/MR are superior PET imaging biomarkers for primary high-risk prostate cancer. Contrast Media Mol Imaging. 2018;2018:8945130.
- Buhmeida A, Pyrhonen S, Laato M, et al. Prognostic factors in prostate cancer. Diagnostic Pathol. 2006;1:4.
- Seisen T, Roupret M, Gomez F, et al. A comprehensive review of genomic landscape, biomarkers and treatment sequencing in castration-resistant prostate cancer. Cancer Treat Rev. 2016;48:25–33.
- Ozkan TA, Eruyar AT, Cebeci OO, et al. Interobserver variability in Gleason histological grading of prostate cancer. Scand J Urol. 2016;50(6):420–424.
- Goodman M, Ward KC, Osunkoya AO, et al. Frequency and determinants of disagreement and error in Gleason scores: a population-based study of prostate cancer. Prostate. 2012;72(13):1389–1398.
- Zelic R, Garmo H, Zugna D, et al. Predicting prostate cancer death with different pretreatment risk stratification tools: a head-to-head comparison in a nationwide cohort study. Eur Urol. 2020;77(2):180–188.
- Eapen RS, Nzenza TC, Murphy DG, et al. PSMA PET applications in the prostate cancer journey: from diagnosis to theranostics. World J Urol. 2019;37(7):1255–1261.
- Morigi JJ, Stricker PD, van Leeuwen PJ, et al. Prospective comparison of 18F-Fluoromethylcholine versus 68Ga-PSMA PET/CT in prostate cancer patients who have rising PSA after curative treatment and are being considered for targeted therapy. J Nucl Med. 2015;56(8):1185–1190.
- Peduzzi P, Concato J, Feinstein AR, et al. Importance of events per independent variable in proportional hazards regression analysis. II. Accuracy and precision of regression estimates. J Clin Epidemiol. 1995;48(12):1503–1510.