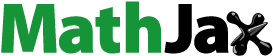
ABSTRACT
One of the goals of the Industry 4.0 paradigm is the sustainable success within a mass customization environment, which in turn, depends on the manufacturing efficiency of the transformation processes. In this paper we propose to represent manufacturing efficiency within the context demand fulfillment. The main original contribution of this paper are two demand fulfillment analytical expressions. Their novelty is on the way they are expressed, that is, in terms of the structural elements that define a mass customization environment. Their usefulness was put to the test via a Discrete Event Simulation (DES) model of two different demand fulfillment strategies (namely inventory-oriented level and capacity-oriented chase strategies). The obtained results suggest that the analytical expressions act as a fairly good trend indicator of the missed demand values increase/decrease. A discussion of the managerial implications of these findings are presented at the end of this document.
1. Introduction
The central idea behind the Industry 4.0 paradigm is the implementation of a fully automated and digitalized production environment (Duarte & Cruz-Machado, Citation2018), by combining technologies such as the Internet of Things (IoT), Big Data, and Cyber-Physical Systems (CPS), which in turn have clear sustainability implications (Junior et al., Citation2018). Appendix A discusses some of the different Industry 4.0 reference architectures that have been proposed by both the Academia and the Industrial practitioners, for those readers interested into this topic. Now, this way of operating has a direct impact in the customer relationship business process, as the customer now has the opportunity of tailoring of products and/or services (Cornelis de Man & Strandhagen, Citation2017). This last relates directly to the mass customization paradigm, which consists on giving the customers the opportunity to design and define their own individual products and/or services (Duarte & Cruz-Machado, Citation2018). At this point, and before proceeding further, it is important to observe the difference between mass customization and mass individualization, a paradigm that shares the same three basic actions of mass customization – namely, design the product, make the product, and sell the product – but in a different sequence and in the customer’s role and involvement in buying the product. According to Koren et al. (Citation2016), (Citation2015):
In mass customization, all modules are designed by the product manufacturer and offered to customers as optional product choices, the customer selects the modules, pays for the product, and then the final product is made and delivered.
In mass individualization, the manufacturer designs the product platform with a large variety of possible interfaces for new modules, and defines the interfaces, the customer selects a platform and searches on the Internet for desired certified modules that fit the selected platform, pays for the platform as well as for the selected modules, and then the modules are sent to the manufacturer and the final product is made and delivered.
Moreover, it has been stated that the central notion of Industry 4.0 is a quick response to the demand of highly customized products, in and profitable way, and considering the environmental and social impacts that guarantees a durable competitiveness (Dziurzanski et al., Citation2018). In this way, the goal of Industry 4.0 – among several others – becomes the sustainable success in a mass customization market (Blecker & Friedrich, Citation2007), (Latorre-Biel et al., Citation2018), where the customers’ requirements increase in diversity (Efthymiou et al., Citation2012), (Man & De Strandhagen, Citation2017), as products are designed to their individual specifications (Duarte & Cruz-Machado, Citation2018), (Citation2017, Citation2017) via the combination of functions and components (Pfisterer et al., Citation2016), and without having to pay a high price premium (Fan-Tien et al., Citation2000), (Zawadzki & Zywicki, Citation2016), (Sievänen et al., Citation2010). Finally, manufacturing enterprises have to find new ways to produce ‘more with less’, as the result from the pressure of customers demanding for eco-efficient manufacturing processes (Heilala et al., Citation2013), (Mishima, Citation2013), (Fysikopoulos et al., Citation2014). This eco-efficiency refers to producing the same amount of products in the right time, with the right quality consuming less energy (Stich et al., Citation2013), (Li, Citation2015), an important aspect of sustainable development (Kang, Citation2016). As the sustainability payoff – the proper balance between the economic and environmental perspectives (Gabriel & Pessl, Citation2016), (Thiede, Citation2018) – is inherent to an Industry 4.0 environment, next section reviews the relationship between the Industry 4.0, Manufacturing Efficiency, and Sustainability topics. Derived from this literature review, we proceed to enunciate our research features: (1) research gaps and opportunities; (2) research proposal (3) proposed research methodology; and (4) research originality, usefulness, validity, and contributions.
2. Literature review
2.1. Manufacturing efficiency
According to Ivanov (Citation2018), the proper or poor alignment among the strategic and operational levels of a manufacturing organization, affects the overall performance. For this reason, it becomes necessary to take that issue into account, as the achievement of high levels of sustainability derives from avoiding a poor efficiency in both the managerial and transformation processes. The Customer–Product–Process–Resource (CPPR) framework (), proposed by Martínez-Olvera et al. (Citation2006), is a comprehensive framework that represents a manufacturing enterprise from a SC standpoint, via a set of structural elements and configuration variables. Within the CPPR framework, there is a set of within-and-among alignment conditions (Martínez-Olvera, Citation2008) necessary for the proper alignment between the strategic and operational levels of manufacturing organization. This proper alignment is a necessary condition for the achievement of the manufacturing efficiency of the transforming processes (Martínez-Olvera & Davizon-Castillo, Citation2015), (Cornelis de Citation2017). The use of the CPPR framework has been extended in order 1) to establish the set of documents that promote proper manufacturing execution and, with this, an efficient performance (Martínez-Olvera, Citation2007); 2) to put establish the set of structural elements that define a mass customization environment (Martínez-Olvera et al., Citation2019).
Figure 1. The CPPR framework (Martínez-Olvera et al., Citation2006).

2.2. Sustainability & industry 4.0
Sun et al. (Citation2012), Brettel et al. (Citation2014), and Citation2016 mention the opportunities of Industry 4.0 for achieving sustainability. Jabbour, Jabbour, Foropon et al. (Citation2018, Citation2018) consider that Industry 4.0 has clear implications for sustainability in organizations. Yadav et al. (Citation2020) introduced the notion of a framework to achieve sustainability in manufacturing organisations of developing economies. A. Jamwal et al. (Citation2021a) proposes a sustainability-based Industry 4.0 framework for MSMEs in for emerging economies. Davis et al. (Citation2012), Kang et al. (Citation2016), and Citation2018 state that a promise of Industry 4.0 – through the use of the so-called smart manufacturing systems – is that it will make it possible to achieve higher agility, productivity, and sustainability levels. Yoon et al. (Citation2012) mentions sustainability as one of the three main requirements of a smart factory. Wang (2016) contends that a smart factory would help implement the sustainable production mode to cope with global challenges. Kusiak (Citation2017a) presents sustainability as one of six pillars considered to be important in setting up smart factories.
On the other hand, Zipkin (Citation2001) and Andersen et al. (Citation2017) state that a critical enabler for an efficient mass customization is the process flexibility of the production system – that is the capability to offer product mix and changeover (Blecker & Friedrich, Citation2007) – as it allows a fast and easy reconfiguration of production facilities (Dhungana et al., Citation2017). However, the higher process flexibility is, the more difficult it is to achieve a high manufacturing efficiency, an issue that can be properly address by the use of automation (Gullander et al.,). From here the idea of addressing the mass customization challenge through the use of an Industry 4.0 environment (Citation2018), (Yao et al., Citation2019), (Mourtzis et al., Citation2018), more specifically, the use of a CPS-based smart manufacturing system, which in turn, according to Thiede (Citation2018), there is an ‘environmental backpack’ due to the introduction of CPS-related components.
For a more extensive discussion of the relationship and link between Industry 4.0 and Sustainability, the reader is referred to R. Jamwal et al. (Citation2021b).
2.3. Sustainable mass customization 4.0
The concept of Sustainable Mass Customization 4.0 (SMC4.0), introduced by Martínez-Olvera and Davizon-Castillo (Citation2015) summarizes the ideas stated in the previous sections: a mass customization environment that focuses in the production of short lead time, tailored products – based on a re-configurable smart CPS, where the sustainability element is derived from the manufacturing efficiency of the transformation processes (Yao et al., Citation2019), (Bordeleau et al., Citation2018), (Citation2018), (Mourtzis et al., Citation2018), (Ghobakhloo, Citation2020) that in turn, allows an optimal resource and energy productivity/efficiency (Otto et al., Citation2014), (Lachenmaier et al., Citation2017). A transformation process can be understood as the value creation chain of steps necessary to transform the input material’s form, shape, and/or properties into the output finished products, in the face of environmental impacts derived from the consumption of energy and other auxiliary resources (Li, Citation2015).
2.4. Research features
Based on the literature review (), the previous section can be summarized as follows: one of the goals of a SMC4.0 system – defined in this paper as a mass customization production system operating within a re-configurable CPS context – is to achieve high levels of sustainability, which can be achieved by the manufacturing efficiency of the transformation processes (understood these last as a set of value creation process chains). Derived from this finding, we identify the following research opportunity: to establish the SMC4.0 paradigm in the context of an energy-efficient, manufacturing process chain. This last can be stated as the following research question: is there a way to quantify the ability of a manufacturing organization to produce ‘more with less’ (for the case of a mass customization environment), so manufacturing efficiency can be expressed within a sustainability context? Following this idea, we state our research proposal to be the development of an analytical expression that quantifies manufacturing efficiency for the case of a mass customization environment. In order to accomplish our research proposal, we propose to proceed in the following way: use the CPPR framework to express the demand fulfillment feasibility equations – presented by Martínez-Olvera et al. (Citation2006) into the context of a mass customization environment. These equations act as an indicator of the demand fulfillment feasibility (ability a manufacturing organization has to achieve a demanded volume), one of many ways on which manufacturing efficiency can be assessed. The original contributions of the research work proposed in this paper are two: 1) A Casual Loop Diagram (CLD) that establishes the relationships derived from the set of structural elements that define a mass customization environment; 2) the proposed demand fulfillment analytical expression, for the case of a mass customization environment. The rest of the paper is organized as follows: Section 3 presents the presents the theory foundation behind the demand fulfillment analytical expression, for the case of a mass customization environment. The usefulness and validity of the analytical expression is demonstrated in Section 4, where the development of a discrete-event simulation (DES) model of a hypothetical mass customization production system allows testing different operational conditions, in order to compare the obtained results with those ones obtained by the analytical expression. A discussion of the managerial implications of the obtained results is presented in Section 5, together with the final conclusions and the identified future research venues.
Table 1. Literature review summarizing table
3. Mass customization manufacturing efficiency
3.1. Mass customization casual loop diagram (CLD)
In his work of 2019, Martínez-Olvera proposed the use of the CPPR framework to establish a set of structural elements that define a mass customization environment (). In this section, we expand this work to derive a Casual Loop Diagram (CLD) that establishes the relationships derived from those structural elements (). (in Appendix B), explain the rationale behind these relationships, and express them in terms of a System Dynamics (SD) model equations (the development of such model is an area of opportunity that will be explored in future research).
Table 2. Mass customization structural elements (Martínez-Olvera et al., Citation2019)
3.2. Demand fulfillment and manufacturing efficiency
According to Chen (Citation2008), the performance of a manufacturing organization can be expressed in terms such as customer satisfaction, product quality, speed in completing manufacturing orders, productivity, diversity of product line, flexibility in manufacturing new products, etc. Following this idea, of reflecting the performance of a manufacturing organization, Cesar Martínez-Olvera (Citation2010) proposed the use of two demand fulfillment formulations (EquationEquations 1(1)
(1) and Equation2
(2)
(2) ):
in this way, when demand uncertainty is low (U = 0), a business model make-to-stock is recommended (BM = 0), and an inventory-oriented level strategy should be used. This strategy requires the use of a rigid continuous production line, where the process environment flexibility is low (F = 0), as the special-purpose equipment used allows to profitably manufacture high-volumes of products with high standardization (S = 1).
in this way, when demand uncertainty is high (U = 1), a business model make-to-order is recommended (BM = 1), and a capacity-oriented chase strategy should be used. This strategy requires the use of a flexible job shop, where the process environment flexibility is high (F = 1), as the general-purpose equipment used allows to profitably manufacture low-volumes of products with low standardization (S = 0).
EquationEquations 1(1)
(1) and Equation2
(2)
(2) represent the contributions made by the inventory-oriented and capacity-oriented strategies to the fulfillment of a certain demand level D, where the closer the result is to this last, the more feasible it will be for the manufacturing organization to achieve the demanded volume (and it must not be taken as an estimator of the final values of the fulfilled demand). One of the findings of this study was that the same total backlog values (i.e. the amount of unfulfilled demand) can be obtained through different combinations of U, BM, F, and S. It is our belief that these equations can be used to represent manufacturing efficiency, as they can be used to determine the optimal U, BM, F, and S levels that would allow achieving a high frecuency of lower total backlog values (that is, produce ‘more with less’, the core concept behind manufacturing efficiency).
3.3. Mass customization demand fulfillment
Based on the relationships established in , we propose to re-write EquationEquations 1(1)
(1) and Equation2
(2)
(2) to reflect the performance of a manufacturing organization working in a mass customization environment, in terms of demand fulfillment (see Equations 3 and 4). For this matter:
Standardization S becomes Customization LC, understood as the total number of manufacturing operations required to obtain a certain finished item (#O).
Flexibility F becomes System´s reconfiguration LSR, understood as the total number of optional manufacturing routes that can be used in the process of building a finished item (#R).
The elements Technification LTECH (understood as the total number of manufacturing operations a certain workcenter can perform, #OM) and Labor Skills LS (understood as the total number of workcenter manufacturing operations a single operator can handle, #S) are added. The inclusion of the LTECH and LS elements is supported by relationships 1, 4, 6, 7, 8, and 10 (see dotted lines in Equations 3 and 4).
(3)
(4)
According to the structure of Equations 3 and 4, the following should be expected:
In a manufacturing scenario with demand uncertainty U= 0, a high level of demand fulfillment can be achieved if an inventory-oriented level strategy is followed (), as long as LC = LTECH = LS = LSR = 0. That is, a manufacturing scenario where the total number of required manufacturing operations, workcenter manufacturing operations, workcenter manufacturing operations to be handled, and optional manufacturing routes is small.
In a manufacturing scenario with demand uncertainty U= 1, a high level of demand fulfillment can be achieved if a capacity-oriented chase strategy is followed (), as long as LC = LTECH = LS = LSR = 1. That is, a manufacturing scenario where the total number of required manufacturing operations, workcenter manufacturing operations, workcenter manufacturing operations to be handled, and optional manufacturing routes is large.
Now, the inventory-oriented level strategy () can be explained as follows:
Customer orders with a varying degree of demand quantity, arrive periodically.
If the arriving customer order quantity ≤ current inventory level, then the Customer order is fulfilled immediately, and the inventory level is adjusted accordingly, i.e. new inventory level = old inventory level – customer order quantity.
If the new inventory level ≤ reorder point level, then the production process is activated:
The current inventory level is replenished with the output of the production process, in the amount equal to batch size.
The production process stops when the current inventory level ≥ target level.
(4) If the customer order quantity ≥ current inventory level, then the customer order is partially fulfilled, and the total amount lost (of unfulfilled customer order) is increased accordingly, i.e. new amount lost = old amount lost + unfulfilled customer order.
and the capacity-oriented chase strategy () can be explained as follows..
Customer orders with a varying degree of demand quantity, arrive periodically.
The production process is activated and the customer order is fulfilled in a batch-size to batch-size basis, until the next customer order arrives.
If by the time the next customer order arrives, the produced amount of units (provided by the production process) ≥ current customer order quantity, then:
The production process stops.
The current level of production capacity is decreased, i.e. the current number of manufacturing resources is decreased by one.
If by the time the next customer order arrives, the produced amount of units (provided by the production process) ≤ current customer order quantity, then:
The customer order is partially fulfilled, and the total amount lost (of unfulfilled Customer order) is increased accordingly, i.e. new amount Lost = old amount lost + unfulfilled customer order.
The current level of production capacity is increased, i.e. the current number of manufacturing resources is increased by one.
The production process starts fulfilling the new customer order.
For both strategies, each incoming customer order arrives periodically and has a varying demand size quantity; each unit composing the demand size quantity is assigned the same number of manufacturing operations; each manufacturing operation is assigned the same processing time (lead time). For both strategies, the production process consists of the following features:
A unit composing the demand size quantity can follow a number of optional manufacturing routes.
Each optional manufacturing route has a workcenter that can perform several different manufacturing operations:
In the case the total number of manufacturing operations to be performed (in a single unit composing the demand size quantity) ≥ the total number of manufacturing operations the workcenter can perform, then that unit must leave the workcenter and wait in line to re-enter it, to complete the remain of the manufacturing operations
Each workcenter has an operator associated to it, that can handle several different workcenter manufacturing operations:
In the case the total number of workcenter manufacturing operations that can be handled by the associated operator ≤ the total number of manufacturing operations the workcenter can perform, then a new operator must be appointed to the workcenter.
3.4. Simulation model of the demand fulfillment strategies
The usefulness of Equations 3 and 4, to reflect the performance of a manufacturing organization working in a mass customization environment (in terms of demand fulfillment), is tested via a discrete-event simulation (DES) model of both the inventory-oriented level and capacity-oriented chase strategies. A justification for following a DES approach is presented in Appendix C, as well as an excerpt of the DES model developed for the inventory-oriented level strategy (Figure C1). The DES model was developed in ARENA (Kellton et al., Citation2004) and used to generate statistical output regarding the performance of each strategy. The following operational conditions were used:
Each incoming customer order arrives periodically (every 15 minutes).
Each incoming customer order is assigned with a demand size quantity, according to a normal distribution (with a mean and a standard deviation parameters).
Each unit composing the demand size quantity, is assigned the same number of manufacturing operations, according to a uniform distribution (with a min and max parameters).
Each manufacturing operation is assigned the same processing lead time.
The production process is assumed to be operating continuously, i.e. breakdowns, changeover, setup, and load/unload times are assumed to be negligible.
All the workcenters can process only one unit (of the demand size quantity) at a time and have a constant processing lead time.
A simulation run time of 1,440 minutes (one day) was used.
The simulation run output was examined for reasonableness, according to the verification and validation approach suggested by Hwarng et al. (Citation2005). Thirty replications were used for each scenario, in order to avoid significant variation in the observed results. Confidence intervals of 90% were used in order to provide the proper statistical basis for making inferences and conclusions. Sixtyfour different combinations were tested under these operative conditions (). These combinations are the result of varying the elements at two different variation levels (note: in the case of normally distributed values, they have parameters μ and σ, and are expressed as N(μ,σ). In the case of uniformly distributed, they have parameters a and b, and are expressed as U(a,b):
Demand uncertainty (1.- U). High variation values, U = N(50, 25); low variation values, U = N(50, 2.5).
Number of operations LC (3.- #O). High variation values, #O = U(4,6); low variation values, #O = U(1,3).
Number of routes LSR (4.- #R). High variation values, #R = 2; low variation values, #R = 1.
Number of workcenter operations LTECH (5.- #OM). High variation values, #OM = U(4,6); low variation values, #OM = U(1,3).
Number of workcenter operations able to be handled by an operator LS (6.- #S). High variation values, #S = U(4,6); low variation values, #S = U(1,3).
Processing lead time (7.- LT). High variation values, LT = 1.0; low variation values, LT = 0.1.
Table 3. Tested combinations (a cell highlighted in gray refers to the use of a high variation value)
Each combination is identified with a nomenclature that identifies which of those elements were varied at the same time, i.e. combination 37 refers to a combination where elements 3 and 7 were varied. Moreover, each combination is assigned with a numeric value so it can be ranked later on, and associated to the results obtained from the DES.
4. Scenario results and analysis
4.1. The inventory-oriented level and capacity-oriented chase strategies
shows the simulation results of the sixty four tested combinations under the inventory-oriented level strategy, while do the proper for the capacity-oriented chase strategy. In both cases, the missed demand values are sorted from largest to smallest value, where a high value of missed demand – defined as the total amount lost demand due to unfulfilled customer orders – is the result of a low level of demand fulfillment. Moreover, for the case of , ‘time producing’ refers to the amount of time the production process is activated, while for , ‘workload’ refers to the amount of work needed to be performed, that is, #O * LT. On the other hand, presents the normalized values of , respectively. In both cases, all the values above the dotted line correspond to combinations with a high U, #O, #R, #OM, and #S (or UOROMS) values.
Table 4. DES results: inventory-oriented level strategy
Table 5. DES results: capacity-oriented chase strategy
Table 6. Combinations not complying with the premises of Equations 3 and 4
Now, the premise behind Equation 3 is that an inventory-oriented level strategy allows the achievement of a high level of demand fulfillment, for a manufacturing scenario with low UOROMS values. In the case of Equation 4, the premise is that a capacity-oriented chase strategy allows the achievement of a high level of demand fulfillment, for a manufacturing scenario with high UOROMS values. However, for both premises, there is a set of combinations that do not comply with them ().
An analysis of reveals the following..
Regarding Equations 3 and 4; high missed demand values are always obtained when there are high LT values, and low missed demand values are always obtained when there are low LT values. This means that the role of the LT must be incorporated into the structure of Equations 3 and 4, as in their current form, they fail to reflect these results (Equations 3ʹand 4’, expressed in terms of UOROMS).
Inventory contribution = D*(1–U)*(1–BM)*(1 – #O)*(1 – #OM) *(1 – #S)*(1 – #R)*(1 – LT) (3’)
Capacity contribution = D*U*BM*#O*#OM*#S*#R*(1 – LT) (4’)
Regarding Equation 3; within the combinations not complying with the premise of Equation 3:
high #O (↑#O) values lead to increase the level of missed demand, in the presence of low U, high LT values.
high #O (↑#O) values lead to decrease the level of missed demand, in the presence of high U, low LT values.
high #R (↑#R) values lead to decrease the level of missed demand, always.
These findings can be expressed by the following expression (see, ):
Table 7. Findings from results
[(1 – U)*(1 – #O)*(LT) + (U)*(#O)*(1 – LT)]
Regarding Equation 4; within the combinations not complying with the premise of Equation 4..
high #O (↑#O) values lead to increase the level of missed demand always, independently of the LT level of variation.
high #R (↑#R) values lead to decrease the level of missed demand, most of the time.
This finding can be expressed by the following expression (see, ):
[(U)*(1 – #O)*(LT) + (1 – U)*(#O)*(1 – LT)]
4.2. Managerial implications
According to the premises behind Equations 3 and 4, the theoretically lowest missed demand values should be obtained at combination 0 (for the Inventory-oriented level strategy), and at combination 13,456 (for the capacity-oriented chase strategy). However, this is not the case. When those combinations not complying with the premises of Equations 3 and 4 (), are taken out from , and we focus only on the ‘low LT values’ side of these Tables – that is, where the low missed demand values are obtained – we obtain . For both strategies, there are eleven combinations that offer lower missed demand values than the theoretically lowest missed demand values. A closer look to these results reveals that..
Those conditions that contribute to lower missed demand values, for the case of the capacity-oriented chase strategy, are the same that contribute to higher missed demand values (red lines), for the case of the inventory-oriented level strategy. The opposite situation presents the same results (black lines).
For both strategies; the #R (number of routes), #OM (number of workcenter operations), and #S (number of workcenter operations able to be handled by an operator), appears almost equally distributed within these eleven combinations.
Table 8. Conditions for lower than theoretically lowest missed demand values
According to Andersen et al. (Citation2017), a critical enabler for an efficient mass customization production system is its process flexibility, so, the findings presented in can be understood from this perspective. In the words of Peng et al. (Citation2008), process flexibility is a bundle of routines and resources that contribute to performance improvement. Moreover, they allow the production system to adapt to diverse customer requirements, a necessary condition mentioned by Blecker and Friedrich (Citation2007) and Dhungana et al. (Citation2017). From this point of view, the number of routes #R, workcenter operations #OM, and workcenter operations able to be handled by an operator #S, contribute to process flexibility, as they allow to produce ‘more with less’ (through several different combinations), precisely the core concept behind manufacturing efficiency. However, even though, increasing the level of flexibility of the mass customization production system brings an improvement of manufacturing efficiency (in the form of low missed demand values), it also comes with some drawbacks..
It is a fact that the higher flexibility a system has, the more difficult it is to achieve high efficiency, due to the increasing level of system’s complexity (Gullander et al.,). This translates into longer waiting times and queue lengths (at each workcenter), something that in turn affects the ability to perform a robust resources scheduling, and with this, the desired manufacturing efficiency (Martínez-Olvera et al., Citation2016).
Within a highly flexible production environment, typical of an Industry 4.0 context, the sequence of manufacturing steps necessary to build a customized product, cannot longer be pre-defined, as the product’s value creation chain has to be created ad hoc. This makes hard to performing the energy consumption what-if analyses required by a serious Sustainability analysis (Citation2020).
Fortunately, the smart components of Industry 4.0 – namely, smart products and machines – can help reduce the complexity inherent to managing the mass customization production system (Kagermann et al., Citation2013), via the use of information technologies (Boer et al., Citation2017), as long as there is no lack of information quality and availability for the use of these associated technologies (Graefenstein et al.,). Also, a set of entropy-based formulations has been developed as an alternative way of performing the initial steps of the energy consumption what-if analyses (Citation2020). Summarizing: in order to truly benefit from the flexibility provided by the #R, #OM, and #S elements, it becomes necessary to provide the mass customization production system with the ‘smart’ element, which is precisely the idea behind of the SMC4.0 mentioned in section 2.3.
5. Conclusions and future research
The central idea behind the Industry 4.0 paradigm is the implementation of a fully automated and digitalized production environment, which is directly related to the mass customization concept, and that has clear sustainability implications. This has forced manufacturing enterprises to find new ways to produce ‘more with less’, through the use of energy-efficient manufacturing processes. In this research effort, we considered that the first step to be taken towards this goal is to quantify the ability of a manufacturing organization to produce ‘more with less’ (for the case of a mass customization environment), so manufacturing efficiency can be expressed within a sustainability context. The original contributions of this paper are a CLD that define a mass customization environment, and the demand fulfillment analytical expressions, for the case of a mass customization environment. The usefulness of the analytical expressions were put to the test via a DES model of two different demand fulfillment strategies (namely inventory-oriented level and capacity-oriented chase strategies). From the obtained results, several conclusions can be derived:
1.- The analytical expressions act as a fairly good trend indicator of the missed demand values increase/decrease. The analytical expressions fail to act as estimator of the missed demand final values.
2.- The accuracy of the expression – regarding how close it follows the missed demand behavior (that is, the values increase/decrease) – is related to the role played by the LT. The missed demand behavior (that is, the values increase/decrease) is affected by the flexibility of the manufacturing process.
Based on these findings, some of the recommendations for future research include:
1.- To introduce a methodology to perform ‘what-if’ scenario analysis, that could guide the process of finding the best alternative, regarding the achievement of high levels of demand fulfillment. The entropy-based (entropic) formulation presented in Citation2020, have probed to be a fairly good trend indicator of the increase/decrease performance parameters (i.e. queue length and waiting times) of a mass customization production system operating within an Industry 4.0 context. The structure of the entropic formulation can be modified to include the number of routes, workcenter operations, and workcenter operations able to be handled by an operator (as in its current form, it only reflects the number of operations) and to act as a good trend indicator of the increase/decrease demand fulfillment ability.
2.- To explore the impact of the smart components of Industry 4.0 in the achievement of high levels of demand fulfillment, and the inclusion of them into the structure of the analytical expressions. These smart components refer to smart products and smart machines, which ‘orchestrate the required resources and negotiate the next step’ to complete the production processes). The challenge is to include this ‘social’ way of working into the demand fulfillment analysis. Regarding this point, some of the initiatives mentioned in Appendix C could be used as the starting point of this new research effort.
Data Availability
The DES model used to support the findings of this study is available from the corresponding author upon request.
Disclosure statement
No potential conflict of interest was reported by the author(s).
References
- Andersen, A. L., Larsen, J. K., Nielsen, K., Brunoe, T. D., & Ketelsen, C., “Exploring barriers toward the development of changeable and reconfigurable manufacturing systems for mass-customized products: An industrial survey”, Customization 4.0. proceedings of the 9th world mass customization & personalization conference (MCPC 2017), S. Hankammer, K. Nielsen, F. T. Piller, G. Schuh, & N. Wang, Editors, Aachen, Germany, November 20th-21st, 2017. Springer International Publishing AG, Gewerbestrasse 11, 6330 Cham, Switzerland.
- Bednar, S., Modrak, J., & Soltysova, Z. (2017). “Assessment of assembly process complexity and modularity in mass customized manufacturing”, Proceedings of 5th international conference on advanced manufacturing engineering and technologies, lecture notes in mechanical engineering, V. Majstorovic & Z. Jakovljevic (eds.), Springer International Publishing, held in Belgrade, Serbia. https://doi.org/10.1007/978-3-319-56430-2_17
- Blecker, T., & Friedrich, G. (2007). “Guest editorial: Mass customization manufacturing systems. IEEE Transactions on Engineering Management, 54(1), 4–11. https://doi.org/10.1109/TEM.2006.889063
- Boer, H. E. E., Nielsen, K., & Brunoe, T. D., “Can the SME successfully adopt mass customization?, customization 4.0,”Proceedings of the 9th world mass customization & personalization conference (MCPC 2017), November 2017, Aachen, Germany, Springer Proceedings in Business and Economics.
- Bonvoisin, J., & Boujut, J., “Open design platforms for open source product development: Current state and requirements”, Proceedings of the 20th international conference on engineering design, Jul. 27-30 2015, Politecnico Di Milano, Italy.
- Bordeleau, F. E., Mosconi, E., & Santa-Eulalia, L. A., “Business intelligence value creation: a multiple case study in manufacturing SMEs undergoing an industry 4.0 transformation”, Proceedings of the 51st Hawaii international conference on system sciences, January 3-6 2018AIS Electronic Library (AISeL), 2018. HICSS 2018, Hilton Waikoloa Village, Hawaii, USA,Waikoloa, HI, Volume,pp. 3944–3953
- Brettel, M., Friederichsen, N., Keller, M., & Rosenberg, M. (2014). How virtualization, decentralization and network building change the manufacturing landscape: An industry 4.0 perspective. International Journal of Mechanical, Aerospace, Industrial and Mechatronics Engineering, 8(1), 37–44. https://doi.org/10.5281/zenodo.1336426
- Chen, -C.-C. (2008). An objective-oriented and product-line-based manufacturing performance measurement. International Journal of Production Economics, 112(1), 380–390. https://doi.org/10.1016/j.ijpe.2007.03.016
- Cornelis de Man, J., & Strandhagen, J. O. (2017). An Industry 4.0 research agenda for sustainable business models. Procedia CIRP, 63(2017), 721–726. https://doi.org/10.1016/j.procir.2017.03.315
- Davis, J., Edgar, T., Porter, J., Bernaden, J., & Sarli, M. (2012). Smart manufacturing, manufacturing intelligence and demand-dynamic performance. Computers & Chemical Engineering, 47(20 December), 145–156. https://doi.org/10.1016/j.compchemeng.2012.06.037
- Dhungana, D., Haselböck, A., & Taupe, R., “A marketplace for smart production ecosystems”, Customization 4.0. Proceedings of the 9th world mass customization & personalization conference (MCPC 2017), S. Hankammer, K. Nielsen, F. T. Piller, G. Schuh, & N. Wang, Editors, Aachen, Germany, November 20th-21st, 2017. Springer International Publishing AG, 11, 6330 Gewerbestrasse, Cham, Switzerland.
- Duarte, S., & Cruz-Machado, V. 2018. “Exploring linkages between lean and green supply chain and the industry 4.0”, Proceedings of the eleventh international conference on management science and engineering management, lecture notes on multidisciplinary industrial engineering springer international publishing AG 2018. Xu Jiuping, G. Mitsuo, H. Asaf, Fang L. Cooke, (Eds.), 103. Proceedings of the Eleventh International Conference on Management Science and Engineering Management, Springer International Publishing. https://doi.org/10.1007/978-3-319-59280-0
- Dziurzanski, P., Swan, J., & Indrusiak, L. S.(July 15–19, 2018).Value-Based manufacturing optimization in serverless clouds for industry4.0.GECCO’18, Kyoto, Japan. ACM978-1-4503-5618-3/18/07, GECCO '18: Proceedings of the Genetic and Evolutionary Computation Conference July 2018, 1222–1229. https://doi.org/10.1145/3205455.3205501
- Efthymiou, K., Pagoropoulos, A., Papakostas, N., Mourtzis, D., & Chryssolouris, G. (2012). Manufacturing systems complexity review: Challenges and outlook. Procedia CIRP, 3(2012), 644–649. https://doi.org/10.1016/j.procir.2012.07.110
- Fan-Tien, C., Yang, H. C., Kuo, T. L., Feng, C., & Jeng, M. D. (2000). Modeling and analysis of equipment managers in manufacturing execution systems for semiconductor packaging. IEEE Transactions on Systems, Man, and Cybernetics — Part B: Cybernetics, 30(5), 772–782. https://doi.org/10.1109/3477.875451
- Fysikopoulos, G., Pastras, T. A., Chryssolouris, G., & Chryssolouris, G. (2014). On a generalized approach to manufacturing energy efficiency. The International Journal of Advanced Manufacturing Technology, 73(9–12), 1437–1452. https://doi.org/10.1007/s00170-014-5818-3
- Gabriel, M., & Pessl, E. (2016). Industry 4.0 and sustainability impacts: Critical discussion of sustainability aspects with a special focus on future of work and ecological consequences. ANNALS of Faculty Engineering HunedoarHunedoara Tomo. 14(2 May 2016), 131–136. https://www.proquest.com/openview/1150ebdb5a42a699c7f77b433a236a43/1?pq-origsite=gscholar&cbl=616472
- Ghobakhloo, M. (2020). Industry 4.0, digitization, and opportunities for sustainability. Journal of Cleaner Production, 252(10 April 2020), 119869. https://doi.org/10.1016/j.jclepro.2019.119869
- Graefenstein, J., Scholz, D., Seifert, O., Winkels, J., Henke, M., & Rehof, J., “Automated Processing of Planning Modules in Factory Planning by Means of Constraint Solving Using the Example of Production Segmentation,“ Springer Proceedings in Business and Economics, In S. Hankammer, K. Nielsen, F. T. Piller, G. Schuh, & N. Wang (Eds.), 157–172. Springer.
- Gullander, P., Davidsson, A., Dencker, K., Fasth, Å., Fässberg, T., Harlin, U., & Stahre, J., “Towards a production complexity model that supports operation, re-balancing and man-hour planning”, Proceedings of the 4th international swedish production symposium: SPS11 3-5 May,Lund Publisher Swedish Production Academy, 2011.
- Heilala, J., Paju, M., Montonen, J., Ruusu, R., Sorli, M., Armij, A., Bermell-Garcia, P., Astwood, S., & Quintana, S. (2013). “Advances in Production Management Systems“, Competitive Manufacturing for Innovative Products and Services. In C. Emmanouilidis, M. Taisch, & D. Kiritsis (Eds.), APMS 2012, 142–150. Springer.
- Helmann, A., Deschamps, F., & Rocha, E. D. F. (2020). Advances in Transdisciplinary Engineering. “Reference architectures for industry 4.0: literature review”, transdisciplinary engineering for complex socio-technical systems – Real-life applications, 12, 171–180. https://doi.org/10.3233/ATDE200074
- Hwarng, H. B, Chong, C. S. P., Xie, N. & Burgess, T. F. (2005). Modeling a complex supply chain: Understanding the effect of simplified assumptions. International Journal of Production Research, 43(13), 2829–2872. https://doi.org/10.1080/00207540500066812
- Ivanov, D. (2018). Structural dynamics and resilience in supply chain risk management. In International series in operations research & management science (1st ed., Vol. 265). Springer International Publishing AG. https://doi.org/10.1007/978-3-319-69305-7_10
- Jabbour, A. B. L. S., Jabbour, C. J. C., Foropon, C., & Godinho, F. (2018). When titans meet –Can industry 4.0 revolutionise the environmentally sustainable manufac-turing wave? The role of critical success factors. Technological Forecasting and Social Change, 132(July 2018), 18–25. https://doi.org/10.1016/j.techfore.2018.01.017
- Jabbour, A. B. L. S., Jabbour, C. J., Godinho, F., & Roubaud, D. (2018). Industry 4.0 and the circular economy: A proposed research agenda and original roadmap for sustainable operations. Annals of Operations Research, 270(1–2), 273–286. https://doi.org/10.1007/s10479-018-2772-8
- Jamwal, R., Agrawal, M. S., Giallanza, A., & Giallanza, A. (2021b). Industry 4.0 technologies for manufacturing sustainability: A systematic review and future research directions. Applied Sciences, 11(12), 5725. https://doi.org/10.3390/app11125725
- Jamwal, A., Agrawal, R., Sharma, M., Kumar, V., & Kumar, S. (2021a). Developing A sustainability framework for industry 4.0. Procedia CIRP, 98, 430–435. https://doi.org/10.1016/j.procir.2021.01.129
- Jiang, P., Leng, J., Ding, K., Gu, P., & Koren, Y. (2016). Journal of Engineering Manufacture. Proceedings of the Institution of Mechanical Engineers, 230(10), https://doi.org/10.1177/0954405416666903
- Johar, I., Lipparini, F., & Addarii, F. (2015). “Document prepared for the European Commission“, In Peter, Ramsden (Ed.), Policy paper April 2015. https://ec.europa.eu/docsroom/documents/20541
- Junior, J. A. G., Busso, C. M., Gobbo, S. C. O., & Carreão, H. (2018). Making the links among environmental protection, process safety, and industry 4.0. Process Safety and Environmental Protection, 117, 372–382. https://doi.org/10.1016/j.psep.2018.05.017
- Kagermann, H., Helbig, J., Hellinger, A., & Wahlster, W., “Recommendations for implementing the strategic initiative industrie 4.0: Securing the future of German manufacturing industry,” Final Report of the Industrie 4.0 Working Group, Forschungsunion 2013.
- Kang, H. S., Lee, J. Y., Choi, S. S., Kim, H., Park, J. H., Son, J. Y., Kim, B. H., & Noh, S. D. (2016). Smart manufacturing: Past research, present findings, and future directions. Int J Pr Eng Man-GT, 3(1), 111–128. https://doi.org/10.1007/S40684-016-0015-5
- Kellton, D., Sadowski, R. P., & Sturrock, D. T. (2004). Simulation with ARENA. In McGraw-hill higher education, (1st ed.,).
- Koren, Y., Hu, S., Gu, P., & Shpitalni, M. (2016). “Social manufacturing as a sustainable paradigm for mass individualization” Proceedings of the Institution of Mechanical Engineers, Journal of Engineering Manufacture,230 (10), https://doi.org/10.1177/0954405416666903
- Koren, Y., Shpitalni, M., Gu, P., & Hu, S. J. (2015). Product design for mass-individualization. Procedia CIRP, 36, 64–71. https://doi.org/10.1016/j.procir.2015.03.050
- Kostakis, V., & Papachristou, M. (2014). Commons-based peer production and digital fabrication: The case of a RepRap-based, Lego-built 3D printing-milling machine. Telematics and Informatics, 31(3), 434–443. https://doi.org/10.1016/j.tele.2013.09.006
- Kusiak. (2017a). Smart manufacturing. International Journal of Production Research, 508–517. Accepted 29June, 2017. https://doi.org/10.1080/00207543.2017.1351644
- Lachenmaier, J. F., Lasi, H., & Kemper, H. G. (2017). Simulation of production processes involving cyber-physical systems. Procedia CIRP, 62, 577–582. https://doi.org/10.1016/j.procir.2016.06.074
- Latorre-Biel, J. I., Faulín, J., Juan, A. A., & Jiménez-Macías, E. (2018). Petri net model of a smart factory in the frame of industry 4.0. IFAC PapersOnLine, 51(2), 266–271. https://doi.org/10.1016/j.ifacol.2018.03.046
- Leng, J., & Jiang, P. (2018). Evaluation across and within collaborative manufacturing networks: A comparison of manufacturers’ interactions and attributes. International Journal of Production Research, 56(15), 5131–5146. https://doi.org/10.1080/00207543.2018.1430903
- Leng, J., & Jiang, P. (2019a). Dynamic scheduling in RFID-driven discrete manufacturing system by using multi-layer network metrics as heuristic information. Journal of Intelligent Manufacturing, 30(3), 979–994. https://doi.org/10.1007/s10845-017-1301-y
- Leng, J., Jiang, P., Kailin, X., Qiang Liu, J. L. Z., Bian, Y., Shi, R., & Shi, R. (2019b). Makerchain: A blockchain with chemical signature for self-organizing process in social manufacturing. Journal of Cleaner Production, 234, 767–778. https://doi.org/10.1016/j.jclepro.2019.06.265
- Leng, J., Yan, D., Liu, Q., Xu, K., Zhao, J. L., Shi, R., Lijun, W., Zhang, D., & Chen, X. (2020). ManuChain: Combining permissioned blockchain with a holistic optimization model as Bi-level intelligence for smart manufacturing. IEEE Transactions on Systems, Man, and Cybernetics: Systems, 50(1), 182–192. https://doi.org/10.1109/TSMC.2019.2930418
- Li, W. (2015). Efficiency of manufacturing processes energy and ecological perspective, sustainable production. In C. Herrmann (Ed.), Life cycle engineering and management series (2015th ed.,). Springer. 21940541 2194055X (electronic), 9783. 21940541 2194055X (electronic), 9783319173641, 9783319173641, 9783319173658 (eBook) 319173658 (eBook) https://doi.org/10.1007/978-3-319-17365-8
- Man, J. C., & De Strandhagen, J. O. (2017). An industry 4.0 research agenda for sustainable business models. Procedia CIRP, 63, 1–21. http://dx.doi.org/10.1016/j.procir.2017.03.315,2017
- Martínez-Olvera, C. (2007). Reference model of the manufacturing execution activity in make-to-order environments. International Journal of Production Research, 47(6), 1635–1659. https://doi.org/10.1080/00207540701636330
- Martínez-Olvera, C. (2008). Methodology for realignment of supply-chain structural elements. International Journal of Production Economics, 114(2), 714–722. https://doi.org/10.1016/j.ijpe.2008.03.008
- Martínez-Olvera, C. (2010). Impact of the alignment between the strategic and operational levels of a manufacturing enterprise. International Journal of Production Research, 48(4), 1195–1215. https://doi.org/10.1080/00207540802534723
- Martínez-Olvera, C. (2020). an entropy-based formulation for the support of sustainable mass customization 4.0. Mathematical Problems in Engineering, 2020, 1–21. https://doi.org/10.1155/2020/3840426
- Martínez-Olvera, C., & Davizon-Castillo, Y. A. (2015). Modeling the supply chain management creation of value: A literature review of relevant concept. In Applications of contemporary management approaches in supply chains; InTech. 978-953-51–2045-2. IntechOpen Limited, The Shard 25 th floor, 32
- Martínez-Olvera, C., Davizón-Castillo, Y., & Mora-Vargas, J. (2016). Entropy-based quantification of a product’s BOM blocking effect. Production & Manufacturing Research, 4(1), 175–189. https://doi.org/10.1080/21693277.2016.1234950
- Martínez-Olvera, Mora-Vargas, J., & Martínez-Olvera, C. (2019). A comprehensive framework for the analysis of industry 4.0 value domains. Sustainability, 11(10), 2960. https://doi.org/10.3390/su11102960
- Martínez-Olvera, Shunk, D., & Martínez-Olvera, C. (2006). Comprehensive framework for the development of a supply chain strategy. International Journal of Production Research, 44(21), 4511–4528. https://doi.org/10.1080/00207540600621698
- Mishima, N. (2013). “Sustainable production: Eco-efficiency of manufacturing process”. In J. Kauffman & K.-M. Lee Eds., Handbook of Sustainable Engineering, 519–540. Springer. https://doi.org/10.1007/978-1-4020-8939-8
- Mourtzis, D., Fotia, S., Boli, N., & Pittaro, P. (2018). Product-service system (PSS) complexity metrics within mass customization and industry 4.0 environment. The International Journal of Advanced Manufacturing Technology, 97(1–4), 91–103. https://doi.org/10.1007/s00170-018-1903-3
- Otto, J., Henning, S., & Niggemann, O. (2014). Why cyber-physical production systems need a descriptive engineering approach –a case study in plug & produce. Procedia Technology, 15, 295–302. https://doi.org/10.1016/j.protcy.2014.09.083
- Peng, D. X., Schroeder, R. G., & Shah, R. (2008). Linking routines to operations capabilities: A new perspective. Journal of Operations Management, 26(6), 730–748.https://doi.org/10.1016/j.jom.2007.11.001
- Pfisterer, D., Radonjic-Simic, M., & Reichwald, J. (2016). Business model design and architecture for the internet of everything. Journal of Sensor and Actuator Networks, 5(2), 7. https://doi.org/10.3390/jsan5020007
- Raza, A., Haouari, L., Pero, M., & Absi, N., “Impacts of industry 4.0 on the specific case of mass customization through modeling and simulation approach,” in Proceedings of the 9th world mass customization & personalization in business and economics, Springer, Cham, Switzerland, June 2018.
- Sievänen, M., Heiskala, M., Tiihonen, J., Stiina Paloheimo, K., & Siirilä, T., “Analyzing service mass customization business models.” MCP-CE 2004 Proceedings, Maciej, Piotrowski. Mass Customization And Personalization - Community of Europe Comittee, September 22-24, 2010, pp. 72–78.
- Stich, V., Hering, N., Starick, C. P., & Brandenburg, U. (2013). Energy-efficiency concept for the manufacturing industry. In V. Prabhu, M. Taisch, & D. Kiritsis (Eds.), 20th Advances in Production Management Systems (APMS), IFIP Advances in Information and Communication Technology Series, 86–93. State College, PA, United States: Springer.
- Stock, T., & Seliger, G 2016. “Opportunities of sustainable manufacturing in industry 4.0”. Procedia CIRP, 40, 536–541. http://dx.doi.org/10.1016/j.procir.2016.01.12
- Sun, Y., Yan, H., Lu, C., Bie, R., & Thomas, P. (2012). A holistic approach to visualizing business models for the internet of things. Communications in Mobile Computing, 1(1), 1–7. https://doi.org/10.1186/2192-1121-1–4
- Thiede, S. (2018). Environmental sustainability of cyber physical production systems. Procedia CIRP, 69, 644–649. https://doi.org/10.1016/j.procir.2017.11.124
- Woo, J., Shin, S. J., Seo, W., & Meilanitasari, P.(2018). Developing a big data analytics platform for manufacturing systems: Architecture, method, and implementation. The International Journal of Advanced Manufacturing Technology, 99, 2193–2217. https://doi.org/10.1007/s00170-018-2416-9
- Wulfsberg, J. P., Redlich, T., & Bruhns, F. L. (2011). Open production: Scientific foundation for co-creative product realization. Production Engineering, 5(2), 127–139. https://doi.org/10.1007/s11740-010-0286-6
- Yadav, G., Kumar, A., Luthra, S., Garza-Reyes, J. A., Kumar, V., & Batista, L. (2020). A framework to achieve sustainability in manufacturing organizations of developing economies using industry 4.0 technologies’ enablers. Computers in Industry, 122, 103280. https://doi.org/10.1016/j.compind.2020.103280
- Yao, X., Zhou, J., Lin, Y., Li, Y., Yu, H., & Liu, Y. (2019). Smart manufacturing based on cyber-physical systems and beyond. Journal of Intelligent Manufacturing, 30(8), 2805–2817. https://doi.org/10.1007/s10845-017-1384-5
- Yoon, J. S., Shin, S. J., & Suh, S. H. (2012). A conceptual framework for the ubiquitous factory. International Journal of Production Research, 50(8), 2174–2189. https://doi.org/10.1080/00207543.2011.562563
- Zawadzki, P., & Zywicki, K. (2016). Smart product design and production control for effective mass customization in the industry 4.0 concept. Management and Production Engineering Review, 7(3), 105–112. https://doi.org/10.1515/mper-2016-0030
- Zipkin, P. (2001). The limits of mass customization. MIT Sloan Manag. Rev, 42(3), 81–87. https://sloanreview.mit.edu/article/the-limits-of-mass-customization/
Appendix A
After reading 75 selected articles and applying the ProKnow-C method, Helmann et al. (Citation2020) identified six architectures that could be used to develop an intelligent manufacturing solution and promote systematic standardization within Industry 4.0/smart manufacturing:
Reference Architecture Model Industrie 4.0 (RAMI 4.0); within this proposal, there are six-layers of integration responsible for making this relationship between all components of the architecture: Business, Functional, Information, Communications, Integration, and Asset layers.
The Industrial Internet Reference Architecture (IIRA); within this proposal, there are five-layers to describe the functions in an industrial system, their interrelation, structure, and interactions: Business, Application, Information, Operations, and Control layers.
IBM Industry 4.0 Architecture (IBM); within this proposal, there are three levels: Edge, Plant/factory/shop floor, and Enterprise or back-end levels.
Smart Manufacturing Ecosystem (SME); within this proposal, there are four dimensions: Product, Production, Business, and Manufacturing dimensions.
Intelligent Manufacturing System Architecture (IMSA); within this proposal, three-dimensions are observed: Intelligent functions, Lifecycle, and System levels.
Industrial Value Chain Reference Architecture (IVRA); within this proposal, three views are observed: Asset, Activity, and Management views.
Finally, the Object Management Group proposes the Industrial Internet Reference Architecture, which proposes four views: Business, Usage, Functional, and Implementation viewpoints. As the reader can observe, all of these proposals have elements in common, so right now, it is too early to call one of them as the dominant over the others. The reader is invited to review the details of each proposal and determine which one fits better to their particular interests.
Appendix B
Table B1. Mass customization CLD relationships, explanation (Source: Author Constructed)
Table B2. Mass customization CLD relationships, system dynamics (SD) model equations (Source: author constructed)
Appendix C
As explained in Section 1, the main difference between the mass customization and the mass individualization paradigm, is the role played by the customer into the design of the final product. Due to the of open source/architecture nature of the mass personalized products, the level of manufacturing interconnection required results in a set of crowded, clustered, and decentralized characteristics (Leng & Jiang, Citation2018). In order to address this ‘social’ way of working, some initiatives have been proposed: Open Production, (Wulfsberg et al., Citation2011); Peer Production (Kostakis & Papachristou, Citation2014); Crowd-Manufacturing (Bonvoisin & Boujut, Citation2015); Maker Manufacturing (Johar et al.,); crowdsourcing-driven/community-based social manufacturing (Jiang et al., Citation2016). Now, in order to reflect the performance of this ‘social’ way of working, special approaches are needed. Without claiming this to be an extensive cover of them (as this falls out of the main topic of this paper), we can identify three main approaches:
1) Holistic; like the one proposed by Leng and Jiang (Citation2019a), where a model of the of discrete manufacturing system dealing with the mass customization paradigm is built, and coupled with the radio frequency identification (RFID) technology, that in this case, is used for performance tracking – at different levels of abstraction – of the elements composing the manufacturing system.
2) Decentralized self-organizing; like the one proposed by Leng et al. (Citation2019b), where a decentralized blockchain-driven model is presented to track the authenticity and quality of the co-created, personalized, open architecture products.
3) Hybrid; like the one proposed by Leng et al. (Citation2020), where a a permissioned blockchain-driven IIoT (Industrial Internet of Things) model can enable partially decentralized self-organization.
As we are addressing the mass customization paradigm, which does not require such a high degree of customer involvement as the mas personalization paradigm, in this paper we followed an approach similar to the one presented in Raza et al. (Citation2018), where a discrete-event simulation (DES) model of a mass customization production system was built to study the impact of introducing the Industry 4.0 paradigm.