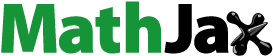
ABSTRACT
In this paper, we propose a new method of measuring social benefits of bioethanol supply chains, which considers job creation, biomass purchase and different impact of economic activities in different locations. A multi-objective MILP model is developed to address the optimal design of a bioethanol supply chain which maximizes both economic and social benefits. The ε-constraint method is employed, and a set of Pareto-optimal solutions is obtained. The developed model is applied to case studies in Heilongjiang Province in Northeast China. Actual data are collected as practical as possible for the feasibility and effectiveness of the result. The result shows the bioethanol supply chain can bring both economic and social benefits in the given area and offer governments a possible better and more efficient way to bring more social benefits. The effect of the government subsidy on enterprises’ decisions about economic and social benefits is discussed.
1. Introduction
With the high-speeded economic growth, China is facing the situation of energy shortage. In 2017, China imported a total of 400 million tons of oil from all over the world, and the ratio of import dependence of oil has grown from 49.0% in 2008 to 65.9% in 2017 (Ministry of Natural Resources of the People’s Republic of China, Citation2018). The development of the bioenergy industry can mitigate this situation to a certain extent. Compared to energy shortage, there is another rigid goal prompting China to develop the bioenergy industry. As the largest carbon emission country in the world, China promised to reduce the carbon emissions per unit GDP by 40–50% by 2020 (China announces carbon target for Copenhagen, Citation2018). The bioenergy consumption is actually nearly carbon neutral because carbon is just released back into the air (Steele et al., Citation2012).
The bioenergy industry is also helpful to control air pollution. Farmers are used to burning agricultural residues (e.g. stover and straw) in farmland after harvesting crops, which is much easier than to remove them away. Research has shown that straw burning is one of the major causes of air pollution in China, and the substantial reduction of open field straw burning would dramatically improve air quality (Zhang et al., Citation2016). The development of bioenergy industries turns farmers into the biomass suppliers. In this way, straw and stover are not burned but collected and transported to biorefineries. At the same time, selling biomass makes farmers richer. It is of much concerned by the Chinese government to raise the income of the poor.
With all the above concerns, the Chinese government has proposed a grand and ambitious plan for the development of bioenergy. In the plan, the output of the bioenergy industry should be not less than the equivalent of 58 million tons of coal by 2020, including 6.8 million of liquid fuel such as bioethanol and biodiesel (National Development and Reform Commission, Citation2018).
This paper addresses the optimal design of the supply chain of bioethanol which is one of the main forms of bioenergy. Bioethanol conversion technologies have been developed for decades. The early conversion technologies convert edible crops, such as sugarcane, corn and wheat. Although this type of technology works in high conversion efficiency, it is not acceptable and unsustainable because of its consumption of edible food. The current conversion technologies are developed based on agricultural residuals or other lignocellulosic materials. This type of technology will not cause food shortages. So, from the current technical view, developing bioethanol supply networks has no negative economic, social or environmental impact.
As for social benefits, it is planned that the development of the bioenergy industry by 2020 will provide 4 million jobs and increase farmers’ income by 3 billion dollars every year (National Development and Reform Commission, Citation2018). The social benefits of bioenergy supply chains have been researched in many literatures. However, the social benefits mean much more in China than other developed countries. Despite decades of rapid economic growth, China is still a developing country. Even in eastern provinces with relatively developed economies, many people were liberated from poverty just for a short time.. The development of the bioenergy industry provides a way to reduce the poverty population and increase the household income.
With different configurations of the bioenergy supply chain, the same increase in farmers’ income may bring different social benefits. The same purchase amount of biomass has different effects in developed and impoverished regions, and so does the job creation. It may offer more social benefits if the created jobs are allocated in impoverished regions or biomass are purchased from farmers in these regions. So, the design of the bioenergy supply chain has a great impact not only on economic benefits but also on social benefits.
Thus, a comprehensive and practical analysis of economic and social benefits is needed by both enterprises and governments. In this paper, we formulate the optimal design of bioethanol supply chains as a multi-objective MILP model, which assists decision makers to ensure the efficiency and effectiveness of biorefinery location, selection of biomass suppliers and transportation network of biomass and products. The objectives are to maximize the economic and social benefits.
A new method of quantifying social benefits is proposed in this paper. In the prior research, the main indicator of social benefits is the job creation. Based on job creation, more comprehensive measuring models are used for quantifying social benefits in some research. You et al. (Citation2012) extended the scope of social benefits beyond job creation and take into account other direct, indirect and induced economic activities of biofuel supply chains. Some research considered the different impacts of new jobs based on their location (Claudia & Taraneh, Citation2016), in which the social benefits were calculated as the weighted sum of the newly created job and the weights were location dependent.
In this paper, both the extended scope and the impact factors based on locations are considered. Besides job creation, biomass purchase activities are also accounted for social benefits. The social benefits are calculated as the weighted sum of job creation and biomass purchase, where the weights are assigned to regions according to their per capita incomes. The core idea of the method is to consider the fact that the same job creation and purchase activity may bring greater social benefits to more poorer regions.
A factor that cannot be ignored is uncertainty. The bioenergy industry needs biomass as raw materials. The output of biomass is highly dependent on weather conditions. The biomass availability obviously has a significant impact on biorefinery location, purchasing costs and transportation costs. Besides the biomass availability, the fluctuations in price and demand also affect the benefits of bioethanol supply chains (Rozakis & Sourie, Citation2005). We develop the model based on the Monte Carlo technology to incorporate uncertainties, in which scenes are used to reflect uncertainties. The software package CPLEX is employed to solve the model.
The ε-constraint method is employed to solve the multi-objective model, and a set of Pareto-optimal solutions is obtained which shows the tradeoff between the two objectives. With the Pareto-optimal curve, the relationship between the gain of social benefits and the loss of economic benefits can be observed. The role of the government subsidy in the decisions about the two objectives is discussed.
The model is applied to case studies in Heilongjiang, a province in Northeast China. Heilongjiang has a high level of development in both heavy industry and agriculture and suitable conditions for developing bioenergy industry.
The rest of this paper is organized as follows. The related literature is reviewed in Section 2 briefly. The problem is described in detail and formulated as a multi-objective MILP model in Section 3. The case studies and the discussion are given in Section 4. Conclusions are presented in the last section.
2. Literature review
In the late 1980s, with the gradual increase of energy prices and the development of bioenergy conversion technologies, the bioenergy industry began to develop rapidly, which led to the development of the research of bioenergy supply chain. Early research focused on the comparison and selection of conversion technologies of biorefineries. Solantausta et al. (Citation1992) first conducted the comparison of the economics of conversion technologies for biorefineries based on different biomass, and the production and transportation costs were calculated with the given biomass prices and capital recovery factor. Considering storage, scheduling and transportation costs, Cundiff et al. (Citation1997) formulated an optimal design problem of biomass delivery systems as a linear programming model, in which uncertainty was addressed. A decision support system was developed which assisted to assess the techno-economic feasibility of biomass to ethanol schemes by Mitchell et al. (Citation1995). Various biomass, conversion technologies and generating cycles were considered in the research. Forsberg, (Citation2000) used life cycle inventory as a method to investigate the environmental load of the bioenergy transportation chains. In the study, biomass for energy was transported from Sweden to Holland. The result showed that emissions of CO2 from long-range transportation performed with ships were of minor importance compared to emissions from local bioenergy systems and the long-range transportation did not lose environmental benefits.
Numerous research studies have been conducted to support the development of the bioenergy supply chain. According to the attributes of the given parameters, models can be categorized into deterministic and stochastic categories.
In deterministic models, all parameters are given and deterministic. The optimal design and operation of bioenergy supply chains are formulated deterministically as linear programming (Lin et al., Citation2016; Radics et al., Citation2016), mixed integer linear programming (Akgul & Seckiner, Citation2021; Ren et al., Citation2015; Sharifzadeh et al., Citation2015) and mixed integer non-linear programming (Nixon, Citation2016).
Deterministic models have been widely used for decades, but it has limitations because it is too idealistic that all parameters or constraints have to be known and deterministic. So, stochastic models are employed to incorporate uncertainties into studies. Awudu and Zhang (Awudu & Zhang, Citation2012) reviewed stochastic models and uncertainties in research of bioenergy supply chains. Uncertainties are categorized into five groups: biomass supply uncertainties, transportation and logistics uncertainties, production and operation uncertainties, demand and price uncertainties and other uncertainties. Razm, Dolgui et al. (Citation2021) proposed a two-phase sequential approach to design bioenergy supply chains under uncertainties with the consideration of social concerns. In the first phase, various factors were considered to find some appropriate locations to simplify the problem, and in the second phase, a robust model was built to cope with the uncertainties based on the result of the first phase. The Monte Carlo simulation technique was considered an effective means to solve uncertainties (Awudu and Zhang, Citation2013).
Some research formulates the bioenergy supply chain optimization as a single-objective model in which only the economic benefits, maximization of profits or minimization of total costs, are considered. Dunnett et al. (Citation2008) developed a combined production and logistics model to investigate cost-optimal system configurations for biomass supply and bioethanol distribution in which the spatially explicit technique was employed. Kim et al. (Citation2011) developed a MILP to maximize the overall profit of the bioenergy supply chain which enabled the selection of fuel conversion technologies, capacities, biomass locations and the logistics of transportation.
In addition to economic benefits, the potentials in environmental and social benefits of bioenergy supply chains have been noticed by researchers. McBride et al. (Citation2011) defined 19 environmental indicators to support the assessment of the environmental sustainability of bioenergy systems. Numerous research integrated the environmental and economic objectives together to analyze the impacts of bioenergy supply chain, in which the environmental objective was formulated as the maximization of GHG emissions savings (Cambero et al., Citation2015) or the minimization of GHG emissions (Giarola et al., Citation2011; Liu et al., Citation2014).
Dale et al., Citation2013) identified 16 indicators for assessing the socioeconomic sustainability of bioenergy systems, including job creation and an increase in household income. You et al. (Citation2012) addressed the optimal design and operations of cellulosic ethanol supply chains under economic, environmental and social criteria which were measured by the number of accrued local jobs. Razm, Brahimi et al. (Citation2021) developed an optimization model of bioenergy supply chains considering social concerns which were mainly estimated as job creation. The job creation was also used to measure the social benefits in (Ayoub et al., Citation2009; Santibanez-Aguilar et al., Citation2014; Yue et al., Citation2014). Only a few studies considered different levels of impact for jobs created in different locations. Mota et al. (Citation2015) proposed a multi-objective model in which the created jobs were more favored in less developed regions. Claudia & Taraneh (Citation2016) incorporated social benefits in a multi-objective optimization of bioenergy and biofuel supply chains. The social benefit indicator was calculated based on the weighted sum of all the new jobs created across the supply chain, and the assigned weights are based on the preferability of creating each type of job in each location.
Not only job creation, but buying biomass from farmers also contribute to social benefits, and the same purchase account from different regions or the same wages paid to regions may offer different levels of social benefits.
To the extent of the author’s knowledge, no bioenergy supply chain optimization study has considered both the impact of economic activities other than job creation and the different impact of economic activities on different locations.
3. Description and model of the problem
3.1. Problem description
The problem in this study can be described as the following. The area to be analyzed is divided into regions according to the administrative divisions, and each region is deemed as a node which may have three roles in the problem. First, it is a potential biomass supplier with a supply capacity related to the yield of corn and rice. The supply capacity is uncertain since it is significantly affected by weather conditions. The biomass, such as stover and straw, need to be collected, dried and stored before being transported to biorefineries. So, if a node is selected to be a biomass supplier, a collection station has to be built. The fixed cost for the facility construction and the variable cost for collecting unit biomass are known. Collection stations bring regional social benefits by purchasing biomass and job creation.
A node is also a customer which has bioethanol demand. The demand and price are affected by populations, development levels, of course, and macroeconomic situations. So, the demand and the price of nodes are both considered uncertain.
Thirdly, a node is a candidate location of biorefineries. The biorefinery location is the most important decision in the optimal design, which influences all the other decisions. And the biorefinery location affects the social benefits caused by the created jobs since the weight is decided by biorefinery locations.
Besides the facility location, the conversion technology and scale of each biorefinery are to be determined as well. The number of created jobs, the conversion rate and the unit production cost are all influenced by the selection of conversion technologies and scales.
The bioethanol supply chain addressed in this paper is shown in , which has three levels: collection stations, biorefineries and customers. The model has two objectives to maximize, the economic and social benefits. The former is measured by calculating the difference between the revenue and the total cost. The revenue comes from the price times the fulfilled demand, and the total cost consists of six components, including the annualized cost for facility constructions; the purchase cost of biomass; the loading and transportation costs of biomass and bioethanol; the cost of collecting biomass in collection stations and the production cost in biorefineries. The social benefits are measured by the weighted sum of the purchase expenditure and the salary expenditure in collection stations and biorefineries.
Prior studies usually use the total number of created jobs, the weighted sum of job hours in (Claudia & Taraneh, Citation2016) or the number of equivalent jobs of all economic activities in (You et al., Citation2012) as the indicator of social benefits. All these indicators are not used in this study, and instead, the total wages are used because the total social benefits also involve the purchase expenditure of biomass. It is necessary to set the weights for two indicators if using the number of created jobs or job hours. Compared to set the weights, it is much simpler to use the weighted sum of expenditures for salary and purchase as the indicator of social benefits.
With the objectives, the decisions to be made include (1) biorefinery location, (2) selection of conversion technologies, (3) selection of scales of biorefinery, (4) selection of biomass suppliers, (5) production of bioethanol and (6) transportations among nodes.
Model of the Problem
Parameters will be introduced when used. Indices, sets and decision variables are given as follows.
Indices/sets
set of nodes
index of candidate locations for biomass suppliers,
index of candidate locations for biorefineries,
index of demand zones,
set of biomass type, indexed by
L set of scales of biorefineries, indexed by
T set of conversion technologies, index by
set of scenarios, indexed by
Decision variables
the amount of biomass r purchased from supplier i in scene s
the amount of biomass r transported from i to j in scene s
the amount of bioethanol transported from j to m in scene s
the amount of biomass r converted in biorefinery j with scale l and technology t in scene s
the amount of bioethanol output in biorefinery j in scene s
binary: 1, if a collection station is to be built at i; 0, otherwise
binary: 1, if a biorefinery with scale l and technology t is to be built at j; 0, otherwise
With the above notions and variables, a multi-objective model is given below. Economic objective – maximizing annualized profit
Social objective – maximizing the social benefits measured by the weighted sum of wages of jobs created and expenditure of purchasing biomass-
In EquationEq. (1(1)
(1) ),
The economic objective is to maximize the total net profit in EquationEq. (1)(1)
(1) . The average revenue comes from selling bioethanol to customers in EquationEq. (3
(3)
(3) ), which is calculated as the sum of revenues in all scenes divided by the number of scenes. The average total cost is the sum of annualized fixed cost for the constructions of biorefineries and collection stations, the average cost of purchasing biomass for all scenes, the average cost of transporting biomass from collection stations to biorefineries and bioethanol from biorefineries to customers, the average operation cost of collection stations and the average cost of converting biomass into bioethanol.
The annualized fixed cost for constructions is given in EquationEq. (5(5)
(5) ),
where IR is the discount rate, P is the project lifetime in terms of years and FC is the total cost for facility constructions and calculated as EquationEq. (6)(6)
(6) .
where ici is the construction cost of building a collection station in i, and icjlt is the construction cost of building a biorefinery in j with scale l and conversion technology t.
where is the cost of purchasing unit biomass r in supplier i.
where is the cost of loading unit biomass r in supplier i and
is the cost of transporting unit biomass r from supplier i to biorefinery j.
where is the operation cost of collecting unit biomass r in supplier i.
where is the production cost of converting biomass r with conversion technology t and scale l in biorefinery j.
where is the cost of transporting unit bioethanol from biorefinery j to customer m.
In Eq. (2),
is the salary expenditure of collecting unit biomass r in station i, and
is the salary expenditure of converting unit biomass in a biorefinery with scale l and technology t in j.
and
are the weights of node i or j for the impact of economic activities on social benefits. The value of
and
are related to regional per capita income. The regions with lower per capita income have larger weights.
=
, when i = j.
Constraints
is the available amount of biomass r in supplier i in scene s. Eq. (14) assures the purchase amount of biomass is no more than its available amount. M is a sufficiently big number, and Eq. (15) indicates that biomass cannot be purchased where no collection station is built. Eq. (16) assures the total amount of biomass r transported to all biorefineries does not exceed the purchase amount. Eq. (17) assures that the amount of transportation from collection stations does not exceed the capacity limitation of stations.
EquationEq. (18)(18)
(18) indicates a biorefinery can only produce with the designated scale and technology. Of course, no production is allowed if no biorefinery is built. EquationEq. (19)
(19)
(19) is the mass balance constraint in biorefineries. The righthand is the sum of the amount of biomass r transported to biorefinery j from all suppliers in scene s. The left hand is the amount of biomass r to be used in biorefinery j with scale l and technology t in scene s. EquationEq. (20)
(20)
(20) is the mass balance constraint in production, where
is the conversion factor from biomass r to bioethanol with the selected scale and technology. EquationEq. (21)
(21)
(21) and (Equation22
(22)
(22) ) define the maximum and minimum annual production capacity, where
is the production capacity of biorefineries with scale l and θl is the minimum production amount as a percentage of capacity.
Eq. (23) assures the amount of bioethanol transported to customers from biorefinery j in scene s does not exceed the output of the biorefinery. Eq. (24) assures the amount of the bioethanol transported to customers does not exceed customers’ demand.
Eq. (25) indicates at most one configuration of scale and technology can be selected for a biorefinery.
4. Case study and discussion
The developed multi-objective model is applied to case studies in Heilongjiang Province, located in Northeastern China. The province has a high energy demand and its agriculture is quite developed. The agricultural products in this area are dominated by corn and rice. The good accessibility of biomass provides the foundation for developing the bioenergy industry. As a developed province in China, Heilongjiang Province has a good transportation infrastructure.
The model is coded in CPLEX Studio 12.6.1 and solved by the same optimization software package. All the computational studies were performed on a Lenovo L440 laptop with Intel i7-4712MQ CPU and 8 GB RAM.
4.1. Input data
Parameters are presented in this subsection. Sources of parameters can be categorized into three types. The first type is parameters that can be found precisely in references, such as availabilities of local biomass and distances among nodes. The second type of parameters is obtained by conducting investigations by the authors, such as transportation costs of biomass and bioethanol from local transportation companies. The rest of the parameters are approximated from various literature sources.
As described above, the case studies are conducted for Heilongjiang Province which comprises 14 cities. Each city is considered a node. The map of Heilongjiang is presented in .
The distance between each pair of adjacent cities is obtained from Heilongjiang Province Atlas (Heilongjiang Jingwei Surveying & Mapping Programming Construction Co., Ltd, Citation2014), and the distance between each pair of any two cities is calculated using the shortest path method. The unit transportation costs of biomass and bioethanol are obtained from the weekly report of road freight index issued by China Federation of Logistics and Purchasing, (Citation2018) and the investigation to local transportation companies. The parameters of transportation costs are presented in .
Table 1. Parameters of transportation costs
With the statistical yearbooks (Heilongjiang Statistics Bureau, Citation2020), annual gasoline consumption of each city in the past 10 years can be obtained. In 2017, an implementation plan of promoting the mandatory use of bioethanol gasoline for vehicles was issued by the National Energy Administration of China (National Energy Administration of China, Citation2018a), in which the gasoline with 10% bioethanol content will be used nationwide by 2020. With this ratio, the bioethanol consumption of the past 10 years can be calculated. Based on the historical data, bioethanol demands of each city are generated for scenes, assuming the demands follow the normal distribution. The bioethanol demands of each city are presented in ).
Figure 3. Data of regions: (a) the demand for bioethanol (thousand tons); (b) the available amount of biomass (thousand tons); (c) per capita income ($).

The price of bioethanol in China is not determined by the energy market, and it is settled to 0.911 times the price of gasoline 92 which is adjusted by the Chinese government regularly (National Energy Administration of China, Citation2018b). The prices of the bioethanol in scenes are generated based on the historical prices of the past years.
Both the prices and the available amount of biomass belong to the first type of parameters. The yield of corn and rice can be obtained from Heilongjiang Province Yearbook of Statistical (Heilongjiang Statistics Bureau, Citation2020). The available amount of stover and straw can be calculated by the ratio between corn/rice and stover/straw. The price of biomass can be found in the authors’ previous work (Gao et al., Citation2014). The total available amounts of stover and straw of regions are presented in ).
There is only one size for collection stations. Its fixed construction cost and variable cost for collecting unit biomass are obtained from an investigation of a bioethanol producer. The construction cost of collection stations is $120,000, and the collecting cost for stover and straw is $12.9 per ton.
The conversion technology used in the existing bioenergy industry in China is mainly the first-generation technology that converts corn into bioethanol. Because of the fact that the first-generation conversion technology consumes a large amount of food, it has been limited by the Chinese government which decided to promote the development of cellulose bioenergy. The third-generation technology is able to convert cellulose and woody biomass into bioethanol. Given the fact that Heilongjiang is a developed area in agriculture and does not have many forests, technologies suitable for woody biomass are excluded from consideration, and the simultaneous saccharification and fermentation conversion technology is the only technology considered in the case studies. The techno-economic parameters of biorefinery of two scales, 45 million gallons per year (MGY) and 80 MGY, are obtained from You et al. (Citation2012) and the Aspen Plus process model.
The wages of producing unit bioethanol are obtained by conducting questionnaires to the bioethanol producer and investigating annual reports of listed companies. The wage of collecting unit biomass is obtained from questionnaires to enterprises as well. The wage of collecting unit biomass is different from the costs of collecting and loading biomass which include labor costs, equipment costs and other material costs. The gathered data are averaged and adjusted considering income differences among provinces. The wages of producing unit bioethanol are $17.42 per ton in the large-scale biorefinery and $18.32 in the small one. The wage of collecting unit biomass is $7.3 per ton.
The social benefit weights are related to regional per capita income, PCi, which can be obtained from the yearbooks (Heilongjiang Statistics Bureau, Citation2020). Let denote the value of the lowest per capita income. The weight of the region i is calculated as
. The values of regional per capita income are presented in ).
4.2. Case studies
In this subsection, two case studies considering only economic benefits are conducted. Both of the two case studies pursue the profit maximization. The difference is, the supply chain does not have to meet all demand in the first one, and all demands must be met in the second.
4.2.1. Case Study 1. Bioethanol supply does not have to meet all the demands
In such condition, the model with the economic objective in EquationEq. (1)(1)
(1) and constraints (14–25) is solved. The optimal net profit is found to be $36,355,293. The total revenue and cost are, respectively, $347,355,880 and $311,000,586, where the profit margin is 10.47%. The percentages of components in the total cost are shown in . The production cost accounts for the largest proportion of total cost 42%. The rest 58% consists of the annualized construction cost 11%, the total transportation cost 14%, the total cost of purchasing biomass 21% and the cost of collection stations 12%.
The biorefinery locations and the supply of biomass and bioethanol are shown in . A large-scale biorefinery and a small one are located in Harbin and Qiqihar, respectively. The average demand of all scenes is 368.2 thousand tons, and the minimum and maximum total demand in all scenes are 352.0 and 389.1 thousand tons, respectively. The sum of production capacity of a large- and a small-scale biorefinery is 373.3 thousand tons. In most scenes, all demands are met, but in a few scenes, not all demands are met because of the fluctuations in demands. Daxinganling and Heihe are far away from other cities, their demand is fulfilled in 79 scenes, and not fulfilled in 21 scenes. Since it is not required to meet all demand in all scenes, the decision of biorefineries is acceptable.
Figure 5. Bioethanol supply chain in Case Study 1. (a) Selected biomass supplier; (b) customer regions supplied by biorefineries.

Harbin is the economic and geographical centers of Heilongjiang and has the most bioethanol demand and is the largest grain producer. It is quite reasonable to locate a large-scale biorefinery in Harbin. Qiqihar has the fourth most demand for bioethanol and is the second-largest grain producer in Heilongjiang. Two biorefineries and their biomass suppliers can be seen in ), and the customers supplied by the biorefineries can be seen in ) where the region in the third color is supplied bioethanol by both two biorefineries simultaneously.
4.2.2. Case Study 2. All demands need to be met
China has launched a series of bioenergy development plans, one of which is to promote the mandatory use of bioethanol gasoline in future. With this prospect, a case study is conducted with a new constraint, EquationEq. (26)(26)
(26) taking place of Eq. (24), which means in all scenes the demands of all nodes need to be fully met.
Solving the updated model with the economic objective function and constraints (14–23, 25–26), in the optimal solution, three small-scale biorefineries are to be constructed, respectively, in Qiqihar, Harbin and Jiamusi, as shown in ). The supply regions of the three biorefineries are presented in ). The regions in the fourth color are supplied with bioethanol by two biorefineries simultaneously.
Figure 6. Bioethanol supply chain in Case Study 2. (a) Selected biomass supplier; (b) customer regions supplied by biorefineries.

Since it is required to meet all demand in all scenes and a large- and a small–-cale biorefinery are not able to supply enough bioethanol, two large-scale or three small-scale biorefineries are needed. Both two options can meet the bioethanol demand. The first option has the lower unit production cost but needs the more total investment than the second one. And adding a biorefinery can make biorefineries closer to biomass suppliers and customers, thereby reducing the total transportation costs. Given the parameters, the second option has the lower total cost.
The optimal net profit is found to be $35,979,126, and the total revenue and the total cost are, respectively, $348,307,766 and $312,328,640, where the profit margin is 10.33%. The percentages of components in the total cost and the comparison with Case Study 1 are shown in .
4.3. Comparision of the two case studies
In Case Study 2, all demand for bioethanol has to be met in all scenes. In Case Study 1, demands in 21 scenes are not met because of the fluctuation in demand. So, more production capacity is required.
Compared with the two large-scale refineries in Case Study 1, three small-scale ones are planned in Case Study 2 to meet all demands in all scenes. The change in the struct of the supply networks leads to the changes in costs in three aspects: production cost, annualized construction cost and transportation cost.
Large-scale biorefineries convert biomass into bioethanol at a relatively lower unit cost than small-scale ones, which means the supply chain given in Case Study 2 has a higher total production cost.
Although a small-scale biorefinery is cheaper than a large one, the total annualized construction cost of three small-scale biorefineries is higher than that of the two large-scale ones.
Unlike the increase in the production cost and annualized construction cost, the transportation cost decreases. In the supply chain of Case Study 2, more biorefineries are planned, which means that the biorefineries are closer to the biomass suppliers and bioethanol customers.
The supply chain of Case Study 2 has more revenue and more total cost than the supply chain of Case Study 1, because of the obligation to meet all needs. The increase in cost exceeds the increase in revenue, resulting in a decrease in the total profit.4.3 Discussion of relationship between economic and social benefits
The economic and social benefits and their relationship are considered in the discussion. The ε-constraint method is employed to obtain a set of Pareto-optimal solutions to show the tradeoff between the two objectives, which has been used in prior studies (Claudia & Taraneh, Citation2016; Cucek et al., Citation2012; You et al., Citation2012). The nature of the ε-constraint method is to convert one of the objectives into a constraint in which the requirement of the converted objective can be tuned to obtain Pareto solutions. The procedure of the method is presented as followings,
Step 1. Solve the model with objective EquationEq. (27)(27)
(27) and constraints (14–25), find the value of the social benefits and let the value be the minimum value of the social benefits.
where λ is a very small number (on the order of 10−6).
Step 2. Solve the model with the social objective EquationEq. (11(11)
(11) ), constraints (14–25) and (28),
which requires the revenue to be greater than the total cost. Let the value of the social benefits be the maximum value of the social benefits.
Step 3. Let and p = 1.
Step 4. Let , and solve the model with the objective function (27), constraints (14–25) and constraint (29),
Step 5. If p < 19, p = p + 1, go to Step 4; else the method ends.
With the method, a set of Pareto-optimal solutions is obtained. The economic and social benefits of the solutions are shown in , and the supply chain configurations of the solutions are shown in .
Table 2. Configurations of Pareto solutions
In , it can be seen that the two objectives, the economic and social benefits, are conflicting. Through constraint (29), the requirement on the social benefits can be changed. When more social benefits are required, the economic benefits decrease. The confliction is explained by elaborating on the supply chain configurations of the Pareto-optimal solutions in .
There are nine different configurations of biorefineries and collection stations corresponding to the different Pareto-optimal solutions, which are presented in in increasing order of the social benefits. A supply chain configuration may result in more than one Pareto solution by increasing or decreasing the purchase amount of biomass or the job creation in different cities, which is why the number of the Pareto solutions is more than the supply chain configurations.
In , all city names are followed by the numbers representing the ranking of the per capita GDP of the corresponding city in the province. It can be observed that with the social benefits increasing, facilities tend to be located in less-developed regions which have greater weights.
The number following the city name is the ranking of the city’s per capita income in Heilongjiang Province.
As biorefineries and biomass suppliers are located in less-developed regions, the total cost becomes higher. In Configuration 1, the configuration under the only economic consideration, two biorefineries are located in Harbin (1) and Qiqihar (10) which are the economic, bioethanol demand and agricultural centers of the province. Compared to Configuration 1, in Configuration 9, three biorefineries are located, respectively, in Qiqihar (10), Qitaihe (11) and Suihua (12). This configuration comes from a high demand of social benefits. In , the following facts can be observed: (1) Suihua and Qitaihe have no strong bioethanol demand; (2) Suihua and Qitaihe are not the economic centers of the province and (3) Qitaihe does not even have a high output of biomass. The reason why these Qitaiher and Suihua are selected as the sites of biorefineries is that they are among the economically least developed cities in this province and have greater weight on the social benefits.
Although the number of the biorefineries increases, the total transportation cost increases as well, because the selected cities are not close to the demand or the biomass suppliers. Besides the transportation cost, more facilities bring the higher annualized construction cost.
Configuration 9 is the one that offers the most social benefits on the premise of ensuring that the economic profit is greater than 0. With the constraint of higher social benefits, the supply chain will not be able to earn any profits.
4.4. Further discussion of the Pareto optimal solutions
To discuss the Pareto optimal solutions further, two indicators are introduced, sq and asq. The meaning and the calculation method of the indicators are as follow.
All Pareto optimal solutions are arranged in increasing order of the social benefits and indexed by q. The slope sq (2 ≤ q≤ 20) between adjacent solutions is calculated as
where Socialq and Economicq denote the social and economic benefits of solution q in the Pareto set. sq means the ratio between the decrement of economic benefits and the increment of social benefits of adjacent solutions.
The accumulative slope asq between solution q and solution 1 is calculated as,
asq (2 ≤ q≤ 20) represents the ratio between the decrement of economic benefits and the increment of social benefits of solution q compared to solution 1. Both sq and asq are shown in .
Nowadays, more and more enterprises consider their social responsibility and the social benefits they offer when making business planning. Being socially responsible allows enterprises to improve their reputation which may bring various kinds of non-economic interests.
Under the context of this paper, when the decision maker is willing to be socially responsible to some extent, shows how much economic benefits are needed to provide the wanted social benefits and the ratio between them. If the decision maker simply pursues the maximization of economic profits, Configuration 1, the leftmost of the curve Economic in the figure, would be the choice. The curve AccSlope in shows the ratio between the loss of economic profits and the gain of social benefits of the different configurations compared to . It can be seen, Curve AccSlope is below 0.5 in a large scope, which means the ratio of the increment of the social benefits to the loss of economic benefits can be greater than 2.
The curves are also helpful for governments to determine subsidy policies. In China, the government spends lots of funds on guaranteeing the basic living of poor people and developing poor regions every year. The development of the bioethanol industry offers a possibly better and more efficient way to use the funds. Subsidizing the bioethanol supply chains may bring social benefits which is much more than the spent subsidy fund for these social benefits.
If the decision maker of the bioethanol supply chain does not want to lose any profits to offer more social benefits, it may be an option for governments to subsidize the supply chain to compensate for its economic loss so that the supply chain may choose the configurations offering more social benefits.
For example, the decision maker simply pursuing the profits chooses the configuration with the economic benefits of and the social benefits of
. There is another configuration with the economic of
and the social of $
, respectively. The government could compensate the supply chain’s economic loss of $
in return for obtaining
on the social benefits.
The curve of asq helps governments to decide how much the subsidy is to get the appropriate input–output ratio. In our case, the value of as2 is 0.09, which means the social benefits of more than 10 times the subsidy can be offered through the bioethanol supply chain.
5. Conclusion
Energy shortage, emission of GHG and air pollution have been prompting the government to develop bioethanol industry in China. It is necessary to research the optimal design of bioethanol supply chain to assist enterprises and government to make decisions. China has liberated a large number of people from poverty. The bioethanol supply chain can offer social benefits in many ways, which is a good opportunity for China to increase incomes of poor people. It was the main motivation for this study to analyze the potentials of economic and social benefits of the bioethanol supply chain in Northeast China and the relationship between the two objectives.
A multi-objective MILP model was built to formulate the problem. A new method of measuring the social benefits was proposed. Two case studies were applied to Heilongjiang Province to analyze the economic potentials of the bioethanol supply chain. In both cases, the bioethanol supply chain was proved profitable, and the profit margins were 10.47% and 10.33%, respectively. The ε-constraint method was used to obtain the Pareto-optimal curve between the economic and social benefits. The curve showed that the bioethanol supply chain could bring much more social benefits with a relatively small loss of profit. In our case, the ratio between the loss in economic benefits and the gain in social benefits could be as small as 0.09. It was implied that governments may play an important role through subsiding enterprises so that enterprises can offer social benefits without any losses on economic benefits.
Disclosure statement
No potential conflict of interest was reported by the author(s).
References
- Akgul, A., & Seckiner, S. U. (2021). Optimization of biomass to bioenergy supply chain with tri-generation and district heating and cooling network systems. Computers & Industrial Engineering, 137, 106017. https://doi.org/10.1016/j.cie.2019.106017
- Awudu, I., & Zhang, J. (2012). Uncertainties and sustainability concepts in biofuel supply chain management: A review. Renewable and Sustainable Energy Reviews, 16(2), 1359–1368. https://doi.org/10.1016/j.rser.2011.10.016
- Awudu, I., & Zhang, J. (2013). Stochastic production planning for a biofuel supply chain under demand and price uncertainties. Applied Energy, 103, 189–196. https://doi.org/10.1016/j.apenergy.2012.09.025
- Ayoub, N., Elmoshi, E., Seki, H., & Naka, Y. (2009). Evolutionary algorithms approach for integrated bioenergy supply chains optimization. Energy Conversion and Management, 50(12), 2944–2955. https://doi.org/10.1016/j.enconman.2009.07.010
- Cambero, C., Sowlati, T., & Pavel, M. (2015). Economic and life cycle environmental optimization of forest-based biorefinery supply chains for bioenergy and biofuel production. Chemical Engineering Research & Design, 107, 218–235. https://doi.org/10.1016/j.cherd.2015.10.040
- China announces carbon target for Copenhagen. (Retrieved 17 September 2018), from: http://energy.cngold.org/c/2018-01-12/c5604479.html
- China Federation of Logistics and Purchasing, China road logistics tariff weekly index report. (Retrieved 20 September 2018), from http://www.chinawuliu.com.cn/lhhkx/201809/14/335036.shtml
- Claudia, C., & Taraneh, S. (2016). Incorporating social benefits in multi-objective optimization of forest-based bioenergy and biofuel supply chains. Applied Energy, 178, 721–735. https://doi.org/10.1016/j.apenergy.2016.06.079
- Cucek, L., Varbanov, P. S., Klemes, J. J., & Kravanja, Z. (2012). Total footprints-based multi-criteria optimization of regional biomass energy supply chains. Energy, 44(1), 135–145. https://doi.org/10.1016/j.energy.2012.01.040
- Cundiff, J., Dias, N., & Sherali, H. (1997). A linear programming approach for designing a herbaceous biomass delivery system. Bioresource Technology, 59(1), 47–55. https://doi.org/10.1016/S0960-8524(96)00129-0
- Dale, V. H., Efroymson, R. A., Kline, K. L., Langholtz, M. H., Leiby, P. N., Oladosu, G. A., Davis, M. R., Downing, M. E., & Hilliard, M. R. (2013). Indicators for assessing socioeconomic sustainability of bioenergy systems: A short list of practical measures. Ecological Indicators, 26(26), 87–102. https://doi.org/10.1016/j.ecolind.2012.10.014
- Dunnett, A., Adjiman, C., & Shah, N. (2008). A spatially explicit whole-system model of the lignocellulosic bioethanol supply chain: An assessment of decentralized processing potential. Biotechnology for Biofuels, 1(1), 1–17. https://doi.org/10.1186/1754-6834-1-13
- Forsberg, G. (2000). Biomass energy transport. Analysis of bioenergy transport chains life cycle inventory method. Biomass & Bioenergy, 19(1), 17–30. https://doi.org/10.1016/S0961-9534(00)00020-9
- Gao, C., Yang, J., Guan, Z. M., Zheng, J. T., & Shi, X. C. (2014). Optimal design of non-food bioenergy supply chain – Taking Liaoning area as the subject. Industrial Engineering Journal, 17(1), 99–104. https://doi.org/10.3969/j.issn.1007-7375.2014.01.017
- Giarola, S., Zamboni, A., & Bezzo, F. (2011). Spatially explicit multi-objective optimization for design and planning of hybrid first and second generation biorefineries. Computers Chemical Engineering, 35(9), 1782–1797. https://doi.org/10.1016/j.compchemeng.2011.01.020
- Heilongjiang Jingwei Surveying & Mapping Programming Construction Co., Ltd. (2014). Heilongjiang Province Atlas. Sino Maps Press.
- Heilongjiang Statistics Bureau. (2020). Heilongjiang statistical yearbook 2019. China Statistical Press.
- Kim, J., Realff, M. J., Lee, J. H., Whittaker, C., & Furtner, L. (2011). Design of biomass processing network for biofuel production using an MILP model. Biomass & Bioenergy, 35(2), 853–871. https://doi.org/10.1016/j.biombioe.2010.11.008
- Lin, Y. Q., Pan, F., & Srivastava, A. (2016). A linear programming optimization model of woody biomass logistics integrating infield drying as a cost-saving preprocess in michigan. Forest Products Journal, 66(7–8), 391–400. https://doi.org/10.13073/FPJ-D-15-00077
- Liu, Z., Qiu, T., & Chen, B. (2014). A study of the LCA based biofuel supply chain multi-objective optimization model with multi-conversion paths in China. Applied Energy, 126, 221–34. https://doi.org/10.1016/j.apenergy.2014.04.001
- McBride, A. C., Dale, V. H., Baskaran, L. M., Downing, M. E., Eaton, L. M., Efroymson, R. A., Garten, C. T., Kline, K. L., Jager, H. I., Mulholland, P. J., Parish, E. S., Schweizer, P. E., & Storey, J. M. (2011). Indicators to support environmental sustainability of bioenergy systems. Ecological Indicators, 11(5), 1277–1289. https://doi.org/10.1016/j.ecolind.2011.01.010
- Ministry of Natural Resources of the People’s Republic of China, Imports of China’s bulk minerals continued to rise in 2017. (Retrieved 17 September 2018), from http://www.geoglobal.mlr.gov.cn/zx/kysc/kcpmy/201801/t20180115_6669660.htm
- Mitchell, C. P., Bridgwater, A. V., Stevens, D. J., Toft, A. J., & Watters, M. P. (1995). Technoeconomic assessment of biomass to energy. Biomass & Bioenergy, 9(1–5), 205–226. https://doi.org/10.1016/0961-9534(95)00092-5
- Mota, B., Gomes, M. I., Carvalho, A., & Barbosa-Povoa, A. P. (2015). Towards supply chain sustainability: Economic, environmental and social design and planning. Journal of Clean Production, 105, 14–27. https://doi.org/10.1016/j.jclepro.2014.07.052
- National Development and Reform Commission, Biomass energy development “13th five-year plan”. docx (Retrieved 17 September 2018), from http://www.ndrc.gov.cn/fzgggz/fzgh/ghwb/gjjgh/201708/W020170809598545774468
- National Energy Administration of China, Ethanol gasoline will not affect food safety. (Retrieved 20 September 2018b), from: http://hzj.nea.gov.cn/adminContent/initViewContent.do?pk=4028481a5e73e011015e83b17cba001d
- National Energy Administration of China, Implementation plan for expanding biofuel ethanol production and promoting the use of ethanol gasoline for vehicles. (Retrieved 20 September 2018a), from http://www.nea.gov.cn/2017-09/13/c_136606035.htm
- Nixon, J. D. (2016). Designing and optimising anaerobic digestion systems: A multi objective non-linear goal programming approach. Energy, 111, 814–822. https://doi.org/10.1016/j.energy.2016.08.053
- Radics, R. I., Dasmohapatra, S., & Kelley, S. S. (2016). Use of linear programming to -optimize the social, -environmental, and economic impacts of using woody feedstocks for pellet and -torrefied pellet production. Biofuels Bioproducts & Biorefining-BioFPR, 10(4), 446–461. https://doi.org/10.1002/bbb.1658
- Razm, S., Brahimi, N., Dolgui, A., & Hammami, R. (2021). Designing bioenergy supply chains under social constraints. Advances in Production Management Systems, 630, 387–396. https://doi.org/10.1007/978-3-030-85874-2_41
- Razm, S., Dolgui, A., Hammami, R., Brahimi, N., Nickel, S., & Sahebi, H. (2021). A two-phase sequential approach to design bioenergy supply chains under uncertainty and social concerns. Computers & Chemical Engineering, 145, 107131. https://doi.org/10.1016/j.compchemeng.2020.107131
- Ren, J. Z., Dong, L., Sun, L., Goodsite, M. E., Tan, S., & Dong, L. (2015). Life cycle cost optimization of biofuel supply chains under uncertainties based on interval linear programming. Bioresource Technology, 187, 6–13. https://doi.org/10.1016/j.biortech.2015.03.083
- Rozakis, S., & Sourie, J. C. (2005). Micro-economic modeling of biofuel system in France to determine tax exemption policy under uncertainties. Energy Policy, 33(2), 171–182. https://doi.org/10.1016/S0301-4215(03)00208-8
- Santibanez-Aguilar, J. E., Gonzalez-Campos, J. B., Ponce-Ortega, J. M., Serna-Gonzalez, M., & El-Halwagi, M. M. (2014). Optimal planning and site selection for distributed multiproduct biorefineries involving economic, environmental and social objectives. Journal of Clean Production, 65, 270–294. https://doi.org/10.1016/j.jclepro.2013.08.004
- Sharifzadeh, M., Garcia, M. C., & Shah, N. (2015). Supply chain network design and operation: Systematic decision-making for centralized, distributed, and mobile biofuel production using mixed integer linear programming (MILP) under uncertainty. Biomass & Bioenergy, 81, 401–414. https://doi.org/10.1016/j.biombioe.2015.07.026
- Solantausta, Y., Beckman, D., Bridgwater, A. V., Diebold, J. P., & Elliott, D. C. (1992). Assessment of liquefaction and pyrolysis systems. Biomass & Bioenergy, 2(1), 279–297. https://doi.org/10.1016/0961-9534(92)90104-X
- Steele, P., Puettmann, M. E., Penmetsa, V. K., & Cooper, J. E. (2012). Life-cycle assessment of pyrolysis bio-oil production. Forest Products Journal, 62(4), 326–334. https://doi.org/10.13073/FPJ-D-12-00016.1
- You, F., Tao, L., Graziano, D. J., & Snyder, S. W. (2012). Optimal design of sustainable cellulosic biofuel supply chains: Multi-objective optimization coupled with life cycle assessment and input–output analysis. AiChe Journal, 58(4), 1157–1180. https://doi.org/10.1002/aic.12637
- Yue, D., Slivinsky, M., Sumpter, J., & You, F. (2014). Sustainable design and operation of cellulosic bioelectricity supply chain networks with life cycle economic, environmental, and social optimization. Industrial & Engineering Chemistry Research, 53(10), 4008–4029. https://doi.org/10.1021/ie403882v
- Zhang, L. B., Liu, Y. Q., & Hao, L. (2016). Contributions of open crop straw burning emissions to PM 2.5 concentrations in China. Environmental Research Letters, 11(1), 014014. https://doi.org/10.1088/1748-9326/11/1/014014