ABSTRACT
Although previous studies have reported the dysregulation of respiratory tract microbiota in infectious diseases, insufficient data exist regarding respiratory microbiota imbalances in the lower respiratory tracts (LRTs) of children with Mycoplasma pneumoniae pneumonia (MPP). Here, we analysed the microbial community using 16S rRNA gene sequencing. Finally, bronchoalveolar lavage fluid (BALF) samples from 158 children with MPP and 29 with bacterial or viral pneumonia (control group) were collected. The diversity of the microbial community was significantly different between the two groups. A significantly increased abundance of Tenericutes and Mycoplasma was detected in the MPP group, exceeding 67% and 65% of the total bacterial population, respectively. Using Mycoplasma abundance as the diagnostic method, the sensitivity and specificity of the model was 97.5% and 96.6%, respectively. Compared to the mild MPP group, lower alpha diversity and significantly increased Mycoplasma abundance were found in the severe MPP group (P < 0.01). The abundance of Mycoplasma was positively correlated with complications and clinical indices in children with severe MPP compared with children with mild MPP. Our study describes the features of the LRT microbiota of children with MPP and uncovered its association with disease severity. This finding may offer insights into the pathogenesis of MPP in children.
Introduction
Mycoplasma pneumoniae (MP) is a major pathogen associated with community-acquired pneumonia (CAP). The latest nationwide prospective surveillance study of all-age patients with acute respiratory infections in China showed that MP is the second leading bacterial pathogen associated with CAP, accounting for 18.6% of CAP cases [Citation1]. MP pneumonia (MPP) accounts for 20-40% of CAP cases in children [Citation2]. Increasing numbers of severe MPP cases have been reported recently, often with heterogeneous clinical presentations and accompanied by extrapulmonary complications that are sometimes life-threatening [Citation1,Citation3].
Host immune reactions play an essential role in the disease severity induced by MP infection [Citation4]. Recent studies have illustrated that microbe-microbe interactions can also significantly impact the aetiology, pathogenesis, and frequency of respiratory diseases [Citation5]. Increasing evidence has indicated that the respiratory microbiota may prime the immune system and provide resistance to pathogen colonization [Citation6]. The respiratory tract microbiota is an important factor regulating and shaping pulmonary immune responses; slight variations in the composition of the microbiota might have great impacts on host immunity due to differences in the structural ligands and metabolites produced by each species [Citation7]. Although several studies demonstrated an imbalance of respiratory tract microbiota in children with MPP [Citation8,Citation9], the potential mechanism of commensal flora in the regulation of MP pathogenesis has not been explored. Knowledge in this field is useful for helping to develop modalities to limit MP overgrowth and infection in the lung. Therefore, this knowledge is essential to improving the prevention and treatment of respiratory disorders.
Serological tests and nucleic acid amplification techniques (NAATs) based assays are the most commonly used methods for diagnosing MPPs in clinical settings. Serological methods are the main diagnostic tools for MP detection. However, several days are usually required for antibody production, especially in extremely young children, and these antibodies persist long after the infection has cleared [Citation10]. The availability of extremely sensitive and timely NAATs allows better interpretation of the serological test results [Citation11]. False negative results may also occur because of the low bacterial loads or during amplification when using poor-quality specimens, such as those collected without adequate skill or not processed in a timely manner [Citation12]. Therefore, for patients without MP antibodies or NAATs-positive results, empirical treatment is often performed based on a clinical diagnosis of the presenting signs and symptoms; thus, misdiagnoses or overtreatment may occur. However, the bacterial microbiota in MPP children with various levels of antibodies and different pathogenic bacterial loads is not clear.
In this study, we enrolled children with CAP, who were indicated for flexible bronchoscopy, to analyse the respiratory microbiota in the lower respiratory tract (LRT) using 16S ribosomal RNA (rRNA) analysis of samples acquired using bronchoalveolar lavage. Gene-based 16S rRNA taxonomic profiling of the bacterial microbiota represented in respiratory specimens may facilitate the study of the overall respiratory microbiota and may identify many bacteria, including potential pathogens [Citation13]. Therefore, this may also provide clues for microbe-microbe interactions in patients infected with MP. We aimed to explore imbalances in the respiratory microbiota in children with signs and symptoms of suspected pneumonia. We also aimed to analyse the association between altered LRT microbiota and disease severity.
Material and methods
Study participants
This study was performed between February 2016 and July 2019 at Beijing (China) Children's Hospital. Children with CAP were enrolled in the study if they were indicated for flexible bronchoscopy and were simultaneously tested for MP antibodies and nucleic acid. Children were diagnosed with MPP if they presented with: (1) fever, cough, tachypnoea, and abnormal breath sounds; (2) typical chest imaging changes (interstitial infiltrates, segmental and lobar consolidations, hilar lymph node enlargement); (3) positive nucleic acid amplification testing (NAAT) results for MP RNA using pharyngeal swab specimens; (4) positive serum MP antibody results which were defined as (i) MP antibody titre ≥ 1:160 on admission or (ii) MP antibody titre seroconversion from negative (<1:80) to positive (≥1:160) within two weeks. The control group included children diagnosed with CAP but not diagnosed with MPP. The exclusion criteria were as follows: an inability to undergo NAAT and antibody testing on admission; an insufficient volume of bronchoalveolar lavage fluid (BALF) for extracting respiratory microbiota DNA or a failure to construct the sequencing library; immune deficiency or immunosuppression; and incomplete clinical information.
Cases of MPP were classified into three subgroups based on the laboratory diagnostic evidence. Children meeting criteria (1), (2), and (3) were classified into the MPP with positive RNA results subgroup (MPR). Children meeting criteria (1), (2), and (4) were classified into the MPP with positive MP antibody results (MPA) subgroup. Children meeting criteria (1) and (2) with a positive response to empirical treatment with anti-mycoplasma drugs were classified into the clinically diagnosed MPP (MPC) subgroup (Fig. S1).
Severe MPP was defined based on the CAP criteria: (1) cyanosis and dyspnoea; (2) pleural effusion; (3) increased breathing rate; (4) lung necrosis/lung abscess; (5) pulmonary atelectasis; and (6) extrapulmonary complications [Citation14-16].
All clinical data, including age, sex, concomitant disease(s), and combined systemic lesions were retrieved from each patient’s medical records. The study was approved by the Ethical Committee of Beijing Children’s Hospital (No. 2022-E-004-Y). All procedures performed in this study were in accordance with the ethical standards of the committee.
Specimen collection and testing
BALF specimens were obtained using a flexible bronchoscope by instilling sterile physiological saline (twice, 10–20 mL each time) into the bronchus, retrieving (5–10 mL each time) the fluid, and transferring it to a 50 mL sterile plastic tube, which was immediately stored at −80 °C for 16S rRNA gene sequencing. Pharyngeal swab specimens for MP RNA detection were collected and tested using MP simultaneous amplification and testing kits (Rendu Biotechnology, Shanghai, China), including nucleic acid extraction and isothermal amplification detection. Two millilitres of peripheral venous blood were centrifuged at 1700 × g and 4 °C for 15 min for serum separation to detect MP-specific antibodies. Subsequently, the serum was separated and assayed using SERODIA MYCO-II (Fuji Rebio Ltd., Tokyo, Japanese), which was performed using artificial gelatine particles sensitized with cell membrane components of MP. The result was considered positive if the titre was 1:160 or higher (≥1:160). Antibodies against MP were detected on admission. When the samples were negative, a paired antibody test was requested seven days later. The process operations and results interpretation were based on the manufacturer’s instructions.
Respiratory microbiota
The total respiratory bacteria DNA was extracted from BALF samples by the hexadecyltrimethylammonium bromide dithiotreitol based method and purified using the Qiagen Gel Extraction Kit (Qiagen, Hilden, Germany). The 16S rRNA genes of the V3-V4 hypervariable region were amplified using with forward primer 341F (5’-CCTAYGGGRBGCASCAG-3’) and reverse primer 806R (5’-GGACTACNNGGGTATCTAAT-3’). The amplification conditions were: 1 min at 98°C for initial denaturation, followed by 30 cycles of denaturation at 98°C for 10 s, annealing at 50°C for 30 s, and elongation at 72°C for 30 s, finally, 72°C for 5 min. Then, the products were purified and quantified, following which the normalized equimolar concentrations of amplicons were pooled and sequenced using an Illumina NovaSeq platform at the Novogene Bioinformatics Technology Co., Ltd. The paired-end reads were generated and assigned to each sample based on their unique barcode and then were merged with FLASH (Fast Length Adjustment of SHort reads) (V1.2.7) software [Citation17]. High-quality filtering of the raw tags was performed to obtain clean tags using QIIME (Quantitative Insights Into Microbial Ecology) (V1.9.1) [Citation18]. After that, chimeric sequences were compared with Silva Database [Citation19] and using the UCHIME algorithm [Citation20] to detect and remove chimera sequences. A final effective sequence analysis was performed using Uparse software (Uparse V7.0.1001) [Citation21].
Data analysis
Sequences with a similarity index greater than 97% were assigned to the same operational taxonomic units (OTUs). Alpha diversity metrics (Shannon and Simpson Indices) were calculated for the OTU table using alpha-rarefaction. The beta diversity metric (Weighted Unifrac distance) was calculated on the OTU table and visualized using principal coordinate analysis (PCoA). Permutational multivariate analysis of variance (PERMANOVA) was performed to compare the global microbiota compositions at the OTU level. The Galaxy Huttenhower Platform (http://huttenhower.sph.harvard.edu/galaxy) was used to calculate the Linear Discriminant Effect Size Analysis (LEfSe) algorithm and to obtain a linear discriminant analysis (LDA) score histogram. In this histogram, the microbial taxa that demonstrated significant differences (using the Kruskal-Wallis Rank-Sum test) among the groups of samples were visualized.
Statistical analysis
All data were analysed using R (Version 4.0.5; R Foundation for Statistical Computing, Vienna, Austria). Parametric continuous variables are expressed as means ± standard deviations and were tested for between-group differences using the Kruskal-Wallis test or Student’s t-test. Categorical variables are presented as numbers and proportions; testing for between-group differences was performed using the Chi-square or Fisher’s exact test. Analysis of receiver operation characteristics (ROCs) and areas under the curve (AUCs) were used to assess the accuracy of the diagnostic model. A logistic regression model was used to analyse the risk of other comorbidities in children with MPP, and the results were further presented in a forest plot. The concordance of the abundance of Mycoplasma spp. with the complication number was presented using Spearman’s rank correlation coefficients. All statistical tests were two-sided, and P < 0.05 was considered statistically significant.
Results
Study population
Among the 187 enrolled paediatric patients with CAP, 158 were diagnosed with MPP and 29 were diagnosed with bacterial or viral pneumonia. The 158 children with MPP included 99 who were positive for MP RNA (MPR), 51 who were positive for MP-specific antibodies (MPA) and 8 with clinically diagnosed MPP (MPC). The characteristics of the subjects are presented in . The microbial pathogens associated with the enrolled patients are shown in Table S1. Children with MPP were older than those in the control group (7.8 ± 2.9 years vs. 5.2 ± 4.5 years, P = 0.005). Clinical presentations were compared between children with MPP and those in the control group using logistic regression, adjusting for age as a covariate. The forest plot shows that children with MPP had a higher risk of fever (OR = 2.86, P = 0.015), cough (OR = 3.94, P = 0.028), pleural effusion (OR = 3.44, P = 0.033), and blood coagulation dysfunction (OR = 6.48, P < 0.001) than the controls ().
Figure 1. Forest plot for the risk factors associated with MPP when compared with the control group. The odds ratio (OR) was based on the logistic regression model and adjusted for a potential confounding factor (age). MPP, Mycoplasma pneumoniae pneumonia patient; Control, other bacterial or viral pneumonia; CI, confidence interval.

Table 1. Characteristics of the study population.
Analysis of respiratory microbiota in children with MPP
Characteristics of LRT microbiota in children with MPP
The diversity of the microbial community estimated by the Shannon ((a), P < 0.01) and Simpson ((b), P < 0.001) indices in the MPP group was lower than in the control group. The PCoA based on Weighted Unifrac distance matrix and confirmed with the PERMANOVA test indicated a significant difference in beta diversity between the two groups (P = 0.001, (c)). We also compared the respiratory microbiota composition of the two groups at the bacterial phylum level. The Firmicutes and Proteobacteria were the most predominant phyla in the control group, accounting for 47.9% and 32.5% of the total sequences, respectively, whereas a significantly increased abundance of Tenericutes was detected in the MPP group, exceeding 67% of the total microbiota ((d)). LEfSe was performed on the statistically significant bacterial taxa obtained using multivariate analysis and confirmed using the Kruskal-Wallis sum-rank test. The results, ranked by LDA score histogram, showed that Mycoplasma spp. were significantly enriched in the MPP group ((e)), accounting for 65% of the respiratory microbiota. Streptococcus and Haemophilus were the next most abundant genera, representing 6% and 3% of the lung microbiota in the MPP group, respectively. In the control group, the abundance of Streptococcus and Haemophilus increased to 41% and 18%, respectively, representing the dominant isolates in the lung microbiota ((d)).
Figure 2. Comparisons of respiratory microbiota in MPP and Control groups. (A) Alpha diversity was evaluated by the Shannon index. (B) Alpha diversity was evaluated by the Simpson index. (C) Principal coordinate analysis based on the Weighted Unifrac matrix between MPP and Control groups. (D) Relative abundance of respiratory microbiota at the phylum and genus level. (E) The species with significant differences in abundance between the two groups by using LEfSe analysis. MPP, Mycoplasma pneumoniae pneumonia patient; Control, other bacterial or viral pneumonia. **P < 0.01, ***P < 0.001.
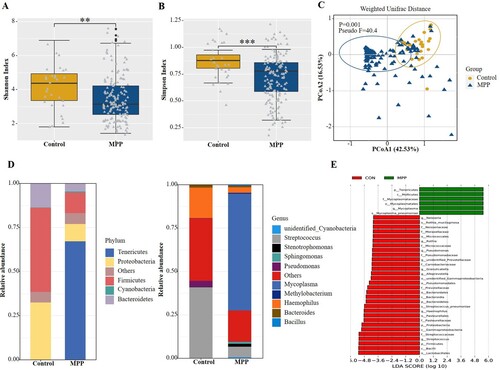
Association of LRT microbiota with severity of MPP
Of the 158 patients with MPP, 57 (36.1%) had severe MPP and 101 (63.9%) had mild cases. The results showed that the alpha diversity of the respiratory microbiota was significantly lower in the severe MPP group than in the mild group (P < 0.01, (a,b)). No significant difference in beta diversity was found between the severe and mild groups (P = 0.997, (c)). The abundance of Tenericutes and Mycoplasma increased significantly in children with severe MPP ((d,e)). Additionally, a higher percentage of Haemophilus spp. was also detected in children with severe MPP when compared with those with mild disease.
Figure 3. Comparisons of respiratory microbiota in Control, severe MPP, and mild MPP groups. (A) Alpha diversity was evaluated by the Shannon index. (B) Alpha diversity was evaluated by the Simpson index. (C) Principal coordinate analysis based on the Weighted Unifrac matrix. (D) Relative abundance of respiratory microbiota at the phylum and genus level. (E) The species with significant differences in abundance between the mild and severe MPP groups by using LEfSe analysis. MPP, Mycoplasma pneumoniae pneumonia patient; Control, other bacterial or viral pneumonia. ***P < 0.001.
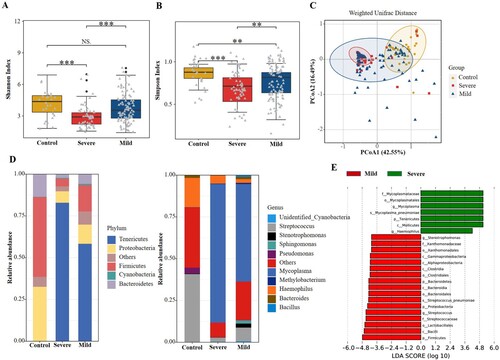
Respiratory microbiota associated with MPP based on different diagnostic standards
The respiratory microbiota was analysed in children with MPP diagnosed based on positive MP RNA (n = 99), positive MP-specific antibody results (n = 51) and clinical evidence (n = 8). No significant differences were found in the alpha ((a)) or beta ((b)) diversities among the three groups. Similarly, we analysed the composition of microbiota among the three groups and found no compositional differences. In all three groups, Tenericutes and Mycoplasma accounted for the greatest abundance of organisms at the phylum ((c)) and genus ((d)) levels, respectively.
Figure 4. Comparisons of respiratory microbiota in MPP children enrolled based on different diagnostic standard. (A) Alpha diversity was evaluated by the Shannon index. (B) Principal coordinate analysis based on the Weighted Unifrac matrix. (C) Relative abundance of respiratory microbiota at the phylum level. (D) Relative abundance of respiratory microbiota at the genus level. NS, no significance.

Respiratory microbiota analysis assists discriminating MPP from other types of CAP
We analysed the microbiota composition at the phylum, family, and genus levels and found that, when compared with the control group, the average abundance of Tenericutes (0.08% vs. 67.20%, P < 0.001), Mycoplasmataceae (0.06% vs. 67.17%, P < 0.001), and Mycoplasma (0.06% vs. 67.17%, P < 0.001) were significantly increased in the MPP group, suggesting their potential value in identification children with MPP ((a–c)). Based on a cutoff value of 0.126% for Mycoplasma at the genus level, the 16S rRNA analysis of respiratory microbiota presented high sensitivity (97.5%, 154/158) and specificity (96.6%, 28/29) for distinguishing MPP from other types of CAP; the area under the ROC curve was 0.994 in this model ((c)). Analysis based on the global microbiota composition at the OTU level illustrated that Mycoplasma is the dominant genus of the phylum Tenericutes (Fig. S2). Table S2 displays the details of the relative abundances of Tenericutes, Mycoplasmataceae, and Mycoplasma for predicting MPP in children.
Figure 5. Relative abundance of Tenericutes, Mycoplasmataceae and Mycoplasma in MPP and Control groups. (A) Tenericutes. (B) Mycoplasmataceae. (C) Mycoplasma. MPP, Mycoplasma pneumoniae pneumonia patient; Control, other bacterial or viral pneumonia. ***P < 0.001.

Four children with positive MP antibody titres were clinically diagnosed as having MPP. However, using our diagnostic model based on the microbiota composition and using the Mycoplasma abundance set at 0.126%, they were re-classified as not having MP infections but as having either Streptococcus spp. infections (two children) or Haemophilus spp. infections (two children) (), consistent with the bacterial culture results (Table S3). Among these children, three had a disease course longer than 20 days and all had received azithromycin treatment at the time of their BALF sample collection.
Correlation analysis between microbiota and disease severity
We found that children with severe MPP were more likely to have more complications than those in the mild MPP group (P < 0.001, Table S4). The concordance of the MP abundance with complications numbers was analysed in the 158 children with MPP ((a)) and showed that the abundance of Mycoplasma was associated with the number of complications.
Figure 7. Correlation networks between microbiota and disease severity. (A) Concordance of the MP abundance and complication numbers. (B) Correlation between the different abundance of respiratory microbiota at the genus level and the clinical indices in children with severe MPP when compared with mild MPP.
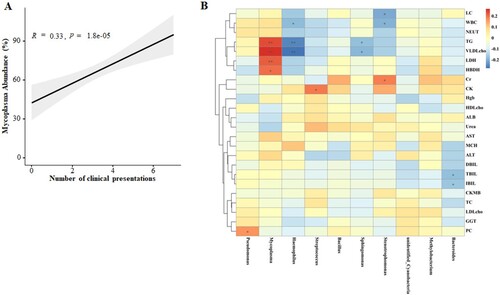
We also analysed the correlation between the abundances of respiratory microbiota constituents at the genus level and the clinical indices in children with various disease severities ((b)). The abundance of Mycoplasma was positively correlated with the levels of HBDH and LDH, suggesting a higher likelihood of cardiovascular injury induced by MP infection in children with severe MPP. Additionally, positive correlations between Mycoplasma abundance and TG and VLDL cholesterol levels were also observed, supporting the premise that lipid metabolism dysregulation occurs in patients with severe MPP. Together, the results suggest that the microbiota composition may impact MPP severity and progression.
Discussion
In the present study, BALF samples were collected to explore the lower respiratory tract respiratory microbiota of children with MPP. Few studies have attempted to explore the characteristics of paediatric lower airways, the primary disease site, because of the practical difficulties involved in collecting LRT samples. Data from our study suggested that MP burden and microbiota in the lung of MPP children were differently from those in the upper respiratory tract [Citation22]. This cross-sectional study described the imbalance of the LRT microbiota of children with MPP and uncovered its association with disease severity.
Appreciable numbers of bacteria are now known to inhabit the lungs of healthy individuals and these populations are known to be altered during chronic and acute lung diseases [Citation23]. In healthy lungs, Firmicutes and Bacteroidetes are the most common phyla of bacteria present [Citation24]. In the lungs of children with MPP, the present study demonstrated that the abundance of Tenericutes increased significantly, exceeding 65% of the entire population The respiratory commensals decreased significantly, with Mycoplasma spp. being dominant. Analysis based on the global microbiota composition at the OTU level demonstrated that Mycoplasma accounted for 99.99% of the total OTUs linked to Tenericutes. The results indicated that MP can deplete other respiratory bacteria through direct competition or by activating bacterial clearance [Citation25]. In healthy individuals, the presence of environmental or upper respiratory tract bacteria in the LRT are quickly cleared through mucociliary and immune mechanisms to maintain a low-level microbial community [Citation26]. MP and its community-acquired respiratory distress syndrome toxin are capable of inducing mucin hypersecretion [Citation27,Citation28], which in turn causes the increased local replication of MP [Citation23]. The positive feedback mechanism may explain the high abundance of Mycoplasma in MPP patients observed in our study.
Recent studies have revealed that the respiratory microbiome is predictive of outcomes in diverse lung diseases [Citation29]. We found decreased alpha diversity, a marker of the number of taxa present and the evenness of their distribution, in the LRT microbiomes of children with severe MPP compared with the findings in children with mild MPP. In this study, we also found that the MP abundance was concordant with the severity of the disease, including the complications and specific blood indicators of organ damage. MP is considered to be a cause of blood-related diseases that may lead to cardiovascular system and gastrointestinal tract damage, including MP-related myocarditis, pericarditis, nephritis and also meningitis [Citation30]. The recognition of MP by innate immune cells and their consequent activation is considered to be the main cause of chronic inflammation and some of the important MP infection symptoms and signs [Citation31]. The counts of MP that are exposed to immune cells are the most important reasons associated with immune response outcomes [Citation32]. The pathogenic mechanism of MP also includes direct damage to the respiratory epithelia and immune cells, including adhesion damage, invasive damage, toxic damage, inflammatory damage, and immune damage [Citation33]. Therefore, early targeted treatment of MP infection is important for promoting pathogen clearance and reducing disease-related damage.
Using 16S rRNA sequencing, we also identified co-infecting bacterial pathogens other than Mycoplasma [Citation22]. The relative abundance of Streptococcus and Haemophilus in children with MPP indicated a high risk of mixed infection by these pathogens and Mycoplasma spp., which was consistent with a previous study reported by our hospital [Citation34]. The significant increase in the abundance of Haemophilus was detected in patients with severe MPP compared with those with mild MPP, possibly promoting an exaggerated inflammatory response and leading to more severe respiratory disease because of the co-infection [Citation35]. Therefore, the other advantage of 16S rRNA sequencing is its ability to analyse the entire respiratory bacterial microbiome, facilitating a complete clinical diagnosis and the targeted treatment of co-infecting pathogens.
The standard microbiological method for identifying the pathogens involved in LRT infections includes culturing bacteria from respiratory samples. But the method is of limited clinical value for non- or poorly culturable pathogens, such as MP [Citation36]. This suggests that the detection of an increased lung MP burden using 16S rRNA sequencing may have diagnostic significance in children with MPP. In recent years, respiratory tract microbial communities in disease have been the subject of study [Citation37]. However, few studies have attempted to correlate changes in the lung microbiome with disease diagnosis. In this study, we analysed alterations in the LRT microbiota and constructed a MPP diagnostic model that was effective in distinguishing MPP from other types of CAP. Using a 16S rRNA-based diagnostic model, we successfully detected 97.5% (154/158) of children with MPP, including those who had negative NAAT or serological results. The production of anti-MP antibodies by the immune system is generally accepted to be slow, resulting in a high proportion of paediatric patients with MPP having negative serological results upon admission. In addition, an antibody titre can persist long after the MP infection has cleared, possibly obscuring the nature of a current infection due to a prior history of MP infection. In this study, four children were originally diagnosed with a co-infection involving MP (based on positive antibody results) and another bacterium (based on culture results). However, using our diagnostic model, all four were confirmed to have current infections only caused by the cultured organisms. We should note that BALF sampling is an invasive procedure, and 16S RNA sequencing is relatively time-consuming. The results using BALF suggested the potential diagnostic role of 16S rRNA gene sequencing in the analysis of infectious diseases using respiratory specimens, which might be extended to non-invasive specimens including sputum and nasopharyngeal swabs. With the development of genetic analysis technologies, updated technologies, such as MinION™ nanopore sequencing [Citation38], might overcome the time-consuming disadvantages of NGS. In general, the diagnostic model built in this study has been restricted to children with indications permitting flexible bronchoscopy.
This study had some limitations. First, BALF samples were collected for the present study. Sputum and BALF are more common approaches for sampling the LRT microbiome. As a noninvasive sample type, spontaneous and induced sputum samples can be collected serially but represent a mix of upper and LRT organisms. Although BALF is less influenced by contamination than sputum, this methodology requires an invasive procedure, which has prevented its use in routine respiratory microbiota analyses. Thus, the presented BALF data was restricted to those subjects for whom bronchoscopy was performed as part of their clinical care. Therefore, data were not available for healthy controls. Secondly, the sample size was relatively small. Future, large cohort studies are warranted to confirm the findings of this study. For example, virus infection was detected in six children with MPP and five children in the control group. Because of the limited sample size, the effects of viral infection on the respiratory microbiota were not analysed in the present study. Thirdly, 16S rRNA gene profiling is unable to differentiate between live and dead bacteria. After the bacteria have been killed, their DNA sequences may persist for several days or even longer [Citation39]. Therefore, 16S rRNA gene profiling does not reflect the activity of the microbiota. Both live and dead bacteria could modulate immune function, via metabolite production by live organisms, and pattern recognition receptors by both [Citation23]. Thus, further investigations into the roles of live and dead bacteria in the induction of inflammation during infectious disease are needed. Fourthly, 16S rRNA gene sequencing was still expensive compared to the now-existing methods such as real time PCR, and difficult to being applied in general situations. This method probably exhibited its specific convenience for its ability to identify other pathogenic bacteria simultaneously for some particular situations, for example in severe MPP cases with atypical clinical manifestations.
Conclusions
Our study characterized the features of the LRT microbiota of children with MPP and uncovered its association with disease severity. Our results suggested that the abundance of MP in the LRT has potential to assist in the diagnosis of MPP. Future, large-scale prospective studies are needed to determine the validity of the diagnostic model we constructed and to fully explore the mechanisms responsible for the associations observed.
Transparency declaration
The lead author and guarantor affirm that the manuscript is an honest, accurate and transparent account of the study being reported; that no important aspects of the study have been omitted; and that any discrepancies from the study as planned and registered have been explained.
Supplemental Material
Download MS Word (29.5 KB)Supplemental Material
Download JPEG Image (260.4 KB)Supplemental Material
Download JPEG Image (133 KB)Disclosure statement
No potential conflict of interest was reported by the author(s).
Correction Statement
This article has been corrected with minor changes. These changes do not impact the academic content of the article.
Additional information
Funding
References
- Li ZJ, Zhang HY, Ren LL, et al. Etiological and epidemiological features of acute respiratory infections in China. Nat Commun. 2021 Aug 18;12(1):5026.
- Gao LW, Yin J, Hu YH, et al. The epidemiology of paediatric Mycoplasma pneumoniae pneumonia in North China: 2006 to 2016. Epidemiol Infect. 2019 Jan;147:e192.
- Kutty PK, Jain S, Taylor TH, et al. Mycoplasma pneumoniae Among Children Hospitalized With Community-acquired Pneumonia. Clin Infect Dis. 2019 Jan 1;68(1):5–12.
- Waites KB, Talkington DF. Mycoplasma pneumoniae and its role as a human pathogen. Clin Microbiol Rev. 2004 Oct;17(4):697–728. table of contents.
- Wu BG, Segal LN. The lung microbiome and its role in pneumonia. Clin Chest Med. 2018 Dec;39(4):677–689.
- Esposito S, Principi N. Impact of nasopharyngeal microbiota on the development of respiratory tract diseases. Eur J Clin Microbiol Infect Dis. 2018 Jan;37(1):1–7.
- Budden KF, Shukla SD, Rehman SF, et al. Functional effects of the microbiota in chronic respiratory disease. Lancet Respir Med. 2019 Oct;7(10):907–920.
- Dai W, Wang H, Zhou Q, et al. The concordance between upper and lower respiratory microbiota in children with Mycoplasma pneumoniae pneumonia. Emerg Microbes Infect. 2018 May 23;7(1):92.
- Zhou Q, Xie G, Liu Y, et al. Different nasopharynx and oropharynx microbiota imbalance in children with Mycoplasma pneumoniae or influenza virus infection. Microb Pathog. 2020 Jul;144:104189.
- Loens K, Ieven M. Mycoplasma pneumoniae: current knowledge on nucleic acid amplification techniques and serological diagnostics. Front Microbiol. 2016;7:448.
- Li J, Sun L, Wu X, et al. Early diagnosis of Mycoplasma pneumoniae in children: simultaneous amplification and testing (SAT) is the key. Front Pediatr. 2019;7:441.
- Räty R, Rönkkö E, Kleemola M. Sample type is crucial to the diagnosis of Mycoplasma pneumoniae pneumonia by PCR. J Med Microbiol. 2005 Mar;54(Pt 3):287-291.
- Kelly BJ, Imai I, Bittinger K, et al. Composition and dynamics of the respiratory tract microbiome in intubated patients. Microbiome. 2016 Feb 11;4:7.
- Harris M, Clark J, Coote N, et al. British Thoracic Society guidelines for the management of community acquired pneumonia in children: update 2011. Thorax. 2011 Oct;66(Suppl 2):ii1–23.
- Subspecialty Group of Respiratory Diseases. The society of pediatrics, C.M.A.E.B., Chinese journal of pediatrics., guidelines for management of community acquired pneumonia in children (the revised edition of 2013) (I) [in Chinese]. Zhonghua Er Ke Za Zhi. 2013;51:745–752.
- Li M, Chen Y, Li H, et al. Serum CXCL10/IP-10 may be a potential biomarker for severe Mycoplasma pneumoniae pneumonia in children. BMC Infect Dis. 2021 Sep 4;21(1):909.
- Magoč T, Salzberg SL. FLASH: fast length adjustment of short reads to improve genome assemblies. Bioinformatics. 2011 Nov 1;27(21):2957–2963.
- Bokulich NA, Subramanian S, Faith JJ, et al. Quality-filtering vastly improves diversity estimates from Illumina amplicon sequencing. Nat Methods. 2013 Jan;10(1):57–59.
- Quast C, Pruesse E, Yilmaz P, et al. The SILVA ribosomal RNA gene database project: improved data processing and web-based tools. Nucleic Acids Res. 2013 Jan;41(Database issue):D590–596.
- Edgar RC, Haas BJ, Clemente JC, et al. UCHIME improves sensitivity and speed of chimera detection. Bioinformatics. 2011 Aug 15;27(16):2194–2200.
- Edgar RC. UPARSE: highly accurate OTU sequences from microbial amplicon reads. Nat Methods. 2013 Oct;10(10):996–998.
- Lu Z, Dai W, Liu Y, et al. The alteration of nasopharyngeal and oropharyngeal microbiota in children with MPP and Non-MPP. Genes (Basel). 2017 Dec 11; 8(12):380
- Whiteside SA, McGinniss JE, Collman RG. The lung microbiome: progress and promise. J Clin Invest. 2021 Aug 2;131(15):e150473.
- Charlson ES, Bittinger K, Haas AR, et al. Topographical continuity of bacterial populations in the healthy human respiratory tract. Am J Respir Crit Care Med. 2011 Oct 15;184(8):957–963.
- Wang H, Zhou Q, Dai W, et al. Lung microbiota and pulmonary inflammatory cytokines expression vary in children with tracheomalacia and adenoviral or Mycoplasma pneumoniae pneumonia. Front Pediatr. 2019;7:265.
- Dickson RP, Erb-Downward JR, Freeman CM, et al. Spatial variation in the healthy human lung microbiome and the adapted island model of lung biogeography. Ann Am Thorac Soc. 2015 Jun;12(6):821–830.
- Hao Y, Kuang Z, Jing J, et al. Mycoplasma pneumoniae modulates STAT3-STAT6/EGFR-FOXA2 signaling to induce overexpression of airway mucins. Infect Immun. 2014 Dec;82(12):5246–5255.
- Medina JL, Coalson JJ, Brooks EG, et al. Mycoplasma pneumoniae CARDS toxin induces pulmonary eosinophilic and lymphocytic inflammation. Am J Respir Cell Mol Biol. 2012 Jun;46(6):815–822.
- Bosch A, de Steenhuijsen Piters WAA, van Houten MA, et al. Maturation of the Infant respiratory microbiota, environmental drivers, and health consequences. a prospective cohort study. Am J Respir Crit Care Med. 2017 Dec 15;196(12):1582–1590.
- Waites KB, Balish MF, Atkinson TP. New insights into the pathogenesis and detection of Mycoplasma pneumoniae infections. Future Microbiol. 2008 Dec;3(6):635–648.
- Shimizu T. Inflammation-inducing Factors of Mycoplasma pneumoniae. Front Microbiol. 2016;7:414.
- Naghib M, Hatam-Jahromi M, Niktab M, et al. Mycoplasma pneumoniae and toll-like receptors: A mutual avenue. Allergol Immunopathol (Madr). 2018 Sep-Oct;46(5):508–513.
- He J, Liu M, Ye Z, et al. Insights into the pathogenesis of Mycoplasma pneumoniae (Review). Mol Med Rep. 2016 Nov;14(5):4030–4036.
- Song Q, Xu BP, Shen KL. Bacterial co-infection in hospitalized children with Mycoplasma pneumoniae pneumonia. Indian Pediatr. 2016 Oct 8;53(10):879–882.
- Welp AL, Bomberger JM. Bacterial community interactions during chronic respiratory disease. Front Cell Infect Microbiol. 2020;10:213.
- Edin A, Granholm S, Koskiniemi S, et al. Development and laboratory evaluation of a real-time PCR assay for detecting viruses and bacteria of relevance for community-acquired pneumonia. J Mol Diagn. 2015 May;17(3):315–324.
- Dicker AJ, Lonergan M, Keir HR, et al. The sputum microbiome and clinical outcomes in patients with bronchiectasis: a prospective observational study. Lancet Respir Med. 2021 Aug;9(8):885–896.
- Rozas M, Brillet F, Callewaert C, et al. MinION™ nanopore sequencing of skin microbiome 16S and 16S-23S rRNA gene amplicons. Front Cell Infect Microbiol. 2021;11:806476.
- Sulaiman I, Wu BG, Li Y, et al. Functional lower airways genomic profiling of the microbiome to capture active microbial metabolism. Eur Respir J. 2021 Jul 29;58(1):2003434.