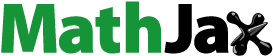
ABSTRACT
Understanding the mammalian pathogenesis and interspecies transmission of HPAI H5N8 virus hinges on mapping its adaptive markers. We used deep sequencing to track these markers over five passages in murine lung tissue. Subsequently, we evaluated the growth, selection, and RNA load of eight recombinant viruses with mammalian adaptive markers. By leveraging an integrated non-linear regression model, we quantitatively determined the influence of these markers on growth, adaptation, and RNA expression in mammalian hosts. Furthermore, our findings revealed that the interplay of these markers can lead to synergistic, additive, or antagonistic effects when combined. The elucidation distance method then transformed these results into distinct values, facilitating the derivation of a risk score for each marker. In vivo tests affirmed the accuracy of scores. As more mutations were incorporated, the overall risk score of virus heightened, and the optimal interplay between markers became essential for risk augmentation. Our study provides a robust model to assess risk from adaptive markers of HPAI H5N8, guiding strategies against future influenza threats.
Introduction
Influenza A virus (IAV) is a ubiquitous pathogen infecting a wide range of avian species and exhibiting species specificity. Barriers typically constrain the spread of virus between species, yet occasional breaches can lead to severe outbreaks, particularly when avian hosts transmit the virus to humans [Citation1, Citation2]. Several viral genetic factors, such as the host receptor binding protein (Hemagglutinin, HA) [Citation3, Citation4], and the polymerase complex [Citation5, Citation6], are instrumental in the inter-species transmission and adaptation of IAVs. The polymerase complex comprises three proteins: polymerase basic protein 2 (PB2), polymerase basic protein 1 (PB1), and polymerase acidic protein (PA) [Citation7]. Alterations in these proteins significantly influence the cross-species transmission and adaptation of IAV through various host-adaptive viral changes [Citation5, Citation8–10]. Notably, an amino acid substitution at position 627 of the PB2 gene (E627K) increases polymerase activity, enhancing the replication efficiency of various AIVs in mammalian hosts [Citation11–15]. Additional adaptive mutations in PB2, such as D701N, Q591R/K, T271A, and E158G, along with mutations like P708S in PB1 and T97I, K142E, and I353R in PA, support replication fitness and pathogenicity in mammalian cells and hosts [Citation12, Citation14, Citation16–22].
Historically, mathematical and machine learning models have shown immense utility in predicting viral load kinetics, characterizing infection dynamics, modelling host immune responses, and analyzing co-infection kinetics of IAVs [Citation23–25]. These models also estimate vaccine and antiviral therapy effectiveness [Citation26], and predict avian-to-human transmission and other interspecies transmissions by scoring viral protein mutations, including those in the polymerase complex [Citation25, Citation27]. Incorporating quantitative experimental data, viral kinetic models provide comprehensive insights into influenza virus replication kinetics across various experimental systems [Citation28–30]. These models contribute significantly to our understanding of the dynamic differences across various influenza strains and inform strategies for future pandemic control [Citation31–37].
In this study, we investigated the adaptation of the highly pathogenic avian influenza (HPAI) H5N8 virus in mammalian hosts. Using next-generation sequencing (NGS), we examined viruses from mouse lung samples over five passages. We then generated viruses with specific adaptive markers using reverse genetics (RG) and analyzed their growth, adaptation, and RNA load dynamics. Using regression analysis and the distance method, we derived risk scores for each marker, and validated these scores by assessing recombinant virus pathogenicity In-vivo. We also cross-validated our model using another H5N8 virus, RG468, to confirm its accuracy. Our findings provide a clear understanding of adaptive markers in viral pathogenicity. This model is essential for assessing risks from new IAV strains, enhancing surveillance, and guiding preventive strategies.
Materials and methods
Cell cultures and viruses. We cultivated A549 and 293T human cell lines in Dulbecco Modified Eagle Medium, augmented with 10% fetal calf serum and 1% antibiotics. In contrast, MDCK cells were maintained in Eagle Minimum Essential Medium with a 5% FCS addition and 1% antibiotics. Two HPAI H5N8 viruses, specifically A/Mallard duck/Korea/W452/2014 (W452, Accession No. KJ746111) and A/Environment/Korea/W468/2015 (W468, Accession No. KX297870), were utilized in this study.
Deep sequencing. To investigate the molecular dynamics of adaptive markers, we utilized lung homogenate samples from each mouse adaptation passage of the mouse-adapted 452 (ma452) and ma468 strains in BALB/c mice (P1-P5). These samples were derived from a prior study [Citation20]. Viral RNA isolation was performed using the RNeasy Minikit (Qiagen) while the conversion of RNA to cDNA was achieved through the MMLV reverse transcriptase kit (Enzynomics), each following the guidelines of manufacturer with minor modifications. PCR amplification and subsequent PCR product purification were conducted using Cosmogenetech DNA purification kit. Next, sequencing libraries were created utilizing the Nextera XT DNA library preparation kit (Illumina). These libraries were then sequenced on the MiSeq platform (Illumina). The raw sequence reads obtained were then processed through several steps: demultiplexing, fast quality check, grooming, and trimming, and filtering. This was performed using the CLC Genomics Workbench 7 software suite (Qiagen, CLC Bio). Finally, all reads were mapped against the W452 and W468 reference sequences. We utilized a quality-based variant detection pipeline to identify any possible mutations.
Construction of recombinant viruses with adaptive markers. The recombinant H5N8 viruses, including the wild type (WT) (RG452-WT and RG468-WT) and variants with adaptive markers, were constructed using RG (Supplementary Table 1). This process entailed the amplification of the eight gene segments from the progenitor virus as per the previous studies [Citation38]. Site-direct mutagenesis created a recombinant virus with a mutated polymerase gene. Each polymerase plasmid (PB2, PB1, PA) subjected to PCR (0.1µg per plasmid) with a Phusion enzyme and specific primer for mutagenesis (Supplementary Table 2). Mutated gene segments were cloned after DpnI treatment, verified by sequencing. For transfection, 1µg of each mutated or wild-type polymerase plasmid and 1µg of remaining gene plasmids were introduced using Lipofectamine in Opti-MEM medium. Co-culture with a 3:1 ratio of 293T and MDCK cells occurred at 37°C with 5% CO2. After 18 h, supernatants were replaced, and 48 h post-transfection, TPCK trypsin was added. Supernatant was propagated in SPF embryonated chicken eggs, stored at −80°C, and titres were determined by TCID50 assays in MDCK cells, expressed in log10.
Development of a model. We examined the growth kinetics, competitive selection intensity, and RNA load of both individual adaptive markers and their combinations. The development and validation of our model involved the following experimental and mathematical systems.
Growth kinetics and regression analysis
In the analysis of growth kinetics, both recombinant RG452-WT and variant viruses were used to infect monolayers of A549 cells with a multiplicity of infection (MOI) of 0.01. After a one-hour incubation at 37°C, the supernatants were replaced with an infection medium that did not contain TPCK-trypsin. Cell culture supernatants were harvested at 12, 24, 36, 48, and 60 days’ post infection (dpi). Viral titres were determined on MDCK cells and expressed as Log10TCID50/mL. Growth speed (GS) of the virus were evaluated using regression analysis.
The growth pattern of the influenza virus, unlike that of other viruses, follows an S-shaped trajectory, prompting the use of a logistic model using the “growth rate” package in R. Viral titre was measured at 12-hour intervals from 0 to 60 h. The following model was assigned for the expression of growth kinetics:
In this model, “K” denotes the maximum titre value, “y0” signifies the minimum value, “time” is the end time, and “m” is a parameter derived through regression analysis. The stay period for growth is 1.8 times. This value was considered as the “stay” time to better reflect the growth pattern of virus, transforming the equation for replication kinetics to:
Here, “stay” represents the stay time, with all other variables remaining as previously defined.
This equation only depicts the GS during the influenza virus growth phase, excluding the stay phase. Therefore, it was necessary to determine a new growth rate for the influenza virus. Thus, the average GS of the influenza virus was obtained using the following model, which employed estimated values derived from regression analysis of the influenza virus:
In this equation, “n” represents the maximum time and “s” is the average GS of the influenza virus.
Competitive selection kinetics and its regression analysis
A549 cells were transfected with recombinant RG452-WT and variant viruses at three different ratios (1:100, 1: 1,000, and 1: 10,000). Following transfection, supernatants were replaced with an infection medium. After three days of incubation, the supernatants were collected and used to infect A549 cells for five consecutive passages. The viral stocks were stored at −80°C for subsequent analyses.
To estimate the cellular competitive selection rate of the viruses containing adaptive markers, we passaged the viruses and employed index sequencing with NGS for the 0th, 1st, 3rd, and 5th passages. We set a threshold value of 2, performing random sampling 100 times, and thus developed a model with a minimum value of 2 and a maximum value of 100 for the logarithmic growth curve. The equation used for this model is:
In this model, the “slope” represents the rate at which influenza virus particles carrying the mutation adapt to the cells. The passage number at which the virus adapts and acquires mutation stability is denoted as “stay.” Considering this “stay” phase, we created a curve using a model with a threshold value of 2, where the mutation proportion is close to 100%. The model representing the “stay” phase for graphical representation is:
To assess the competitive selection intensity of RG452 variant viruses against RG452-WT, values were consolidated into a singular metric by determining the area under the curve (AUC) values. These AUC values subsequently illustrated the competitive selection rate of the recombinant variants equipped with adaptive markers in comparison to RG452-WT.
RNA load kinetics and regression analysis
A549 cells were infected with RG452-WT and variant viruses, each at MOI of 0.1. Four hours’ post-infection, infected cells were collected and their total RNA was extracted using a Qiagen RNeasy Minikit. cDNA was synthesized from the NP viral RNA segment with gene-specific primers (Supplementary Table 3). Quantitative real-time PCR was then conducted on a LightCycler® 96 system (Roche), in accordance with previously described methods and conditions [Citation39].
A549 cells were infected with RG452-WT and variant viruses, each at a MOI of 0.1. Four hours’ post-infection, infected cells were collected and their total RNA was extracted using a Qiagen RNeasy Minikit. For cDNA synthesis of vRNA, cRNA, and mRNA, mix 10 ul of RNA, 1 ul (10 pmol) of Revese Transcription primer, and 4 ul of distilled water heat at 65°C for 10 min, immediately cool on ice for 5 min, and then add 2 μl dNTP mixture (10 mM each), 1 μl Superscript III enzyme, 2 ul DDT (0.1M) were mixed and heated at 60°C for 1 h. To inhibit the enzyme, it was incubated at 70°C for 10 min. Quantitative real-time PCR was then conducted on a LightCycler® 96 system (Roche) with the assistance of Superscript III Reverse Transcriptase (Life Technologies) and FastStart SYBR Green Master (Sigma-Aldrich), in accordance with previously described methods and conditions [Citation39]. The 3 ul of 10-fold diluted cDNA was added to the qPCR reaction mixture containing 10 μl SYBR GreenER qPCR SuperMix, 2 μl forward primer (5 μM), 2 μl reverse primer (5 μM), 3 μl double distilled water. Cycling conditions for qPCR were 95°C for 10 min, followed by 40 cycles of 95°C for 15 s and 60°C for 1 min. A standard curve was generated using 10-fold serial dilutions (109, 108, 107, 106, 105, 104, and 103 copies/μl) of synthetic viral RNA standards.
After relative quantitation of the RNA, we then conducted a regression analysis, incorporating metrics from GS and competitive selection rate (CSR) as dependent variables and the RNA fold change values as independent variables to achieve the weight of each viral RNA type.
The weights assigned to each RNA type were determined by the arithmetic mean of the values gleaned from the regression analysis. The model is as follows:
In this case, weight1 and weight2 are parameters obtained from the regression analyses of the replication kinetics and competition kinetics against RNA fold change, respectively. Following the attainment of the weights for cRNA, vRNA, and mRNA via regression analysis, the sum of these ratios was utilized to create a comprehensive RNA load value.
Assessment of quantitative risk scores
The parametric values gleaned from the regression analyses – GS, CSR, and RNA load value – were all scaled to one. These scaled values were then subjected to a calculation of Euclidean distance, generating quantitative risk scores for all adaptive markers, as per the following model:
In this equation, “d” represents the Euclidean distance. The ordered pairs (x1, y1, z1) and (x2, y2, z2) denote the initial and final coordinate points, respectively. This model essentially offers a straightforward way to quantify the “distance” or difference between the values of adaptive markers, enabling a more systematic and mathematically rigorous assessment of their respective risk levels.
Cross-validation of the model. We cross-validated our model using another H5N8 virus, RG468, to confirm its ability to accurately predict the quantitative risk scores (QRS) of all adaptive markers for the influenza A virus. Similar to RG452, we extracted all metrics such as GS, CSR, RNA, risk score for RG468. Overall, cross-validation with six recombinant RG468 viruses with the adaptive markers and the statistical parameters affirm the reliability and sensitivity of the model in predicting the quantitative scores of all adaptive markers for influenza A virus.
In vivo model validation: sensitivity and specificity analysis
Animal subjects
The study used 6–7-week-old female BALB/c mice procured from the Jackson Laboratory (Bar Harbor, Maine, USA) to validate the model in vivo. We split the mice into eight experimental groups, with five mice per group (N = 5), to analyze weight change and survival. Additionally, for systemic infection analysis of the recombinant RG452 viruses, we formed eight more groups of three mice each (N = 3).
Survival, weight change, systemic infection analysis of the RG452 viruses
Mice were intranasal infected with five varying viral titres of eight distinct recombinant RG452 virus, ranging from 1 to 6 log10TCID50/mL, and monitored over for 14 days for weight change, mortality, and other signs of morbidity. In a separate experiment, mice were infected with a 4 log10TCID50/mL virus dose, and the systemic spread of the virus was examined in various organs, including the lungs, brain, heart, spleen, and kidneys, which were collected at 5 dpi for viral titre estimation.
Performance evaluation of the risk scores of each recombinant RG452 virus containing the single and multiple adaptive markers
We compared In vitro QRS with In vivo data on survival rates, weight changes, and systemic viral spread to validate the model. Receiver operating characteristic (ROC) curves were generated, plotting sensitivity against the relative false-positive rate (1-specificity) [Citation40]. The AUC measures the predictive accuracy of each adaptive marker. A ROC curve passing through the upper left corner, corresponding to an AUC of 1, indicates an ideal quantitative trait with 100% sensitivity and 100% specificity.
Statistical analysis
We utilized the rstatix package in R to obtain the ANOVA summary statistical information across various study objects. The statistical significance between the wild type and other adaptation markers was executed using a t-test of the ggsignif package in R. The survminer package in R was utilized for Kaplan–Meier analysis to assess overall survival across the study groups. Significance in the difference of overall survival was determined using the log-rank test. For all the study groups, statistical significance when compared to RG452-WT is denoted by “ns” for non-significant, *p < 0.05, **p < 0.01, and ***p < 0.001.
Results
Dynamics of mammalian adaptive markers during sequential passage of H5N8 viruses in mice
offers a comprehensive schematic representation of the study. We previously conducted mouse adaptation studies using two HPAI H5N8 viruses, W452 and W468 [Citation20]. These viruses were serially passage through four mouse groups for five consecutive passages (P1–P5). In this current study, lung sample homogenates from two out of the four mouse groups for each adaptation passage were subjected to NGS to confirm the mutations leading to amino acid substitutions. In the W452-WT infected mouse groups, the PB2D701N substitution, a recognized adaptive mutation [Citation41], was observed with a frequency of 50-90% after just one passage ((A,B)). Alongside PB2D701N, the PB2Q591K mutation, another recognized mammalian adaptive mutation [Citation17], was detected, although its frequency increased more gradually, only becoming predominant (over 50% of the population) after the fourth passage in one of the mice ((A,B)). PB1P708S mutation was detected in both virus passage groups and also gradually increased, resulting in 60-95% of the population after five passages ((A,D)). The PB2E627K substitution, a well-documented mammalian adaptive marker [Citation2], was exclusively detected in the W468-WT infected mouse groups. It became predominant (over 85% of the population) within just two passages, ultimately representing nearly 100% of the viral population ((C,D)). Though the PAT97I substitution was detected in both virus passage groups, it only dominated (over 80%) when combined with PB2E627K ((A,C)). Lastly, the PAQ556R mutation was recently found in one of the W452-WT infected mice but never represented more than 50% of the viral population ((B)). These results demonstrate that each adaptive marker correlates with differential viral dynamics, influencing the intensity, frequency, and viral state within the host.
Figure 1. Study overview. This research delves into mice adaptation to identify adaptive markers using NGS. Utilizing reverse genetics, we generated viruses with various adaptive markers to conduct three experiments: replication dynamics, adaptation analysis, and RNA quantification. A nonlinear regression model was then applied to derive metrics, which were used to compute individual QRS for each marker. In vivo experiments subsequently validated these scores for sensitivity and specificity. GS-growth speed, QRS-quantitative risk scores.

Figure 2. Mapping intensity of adaptive markers in ma452 and ma468 viruses through five sequential passages. The figures display an escalating mapping intensity for specific adaptive markers in viruses: (A) ma452-G1, (B) ma452-G3, (C) ma468-G1, and (D) ma468-G2. The deep-sequencing was performed using sequentially passaged mice lung samples in previous study. Each of these viruses embodies unique adaptive markers situated within their viral polymerase genes. ma denotes mouse-adapted.

Growth speed values facilitate quantitative analysis of distinct replication kinetics in recombinant viruses with various mammalian adaptive markers
Eight recombinant viruses, each embodying distinct adaptive markers, were produced using the RG technique with the background of A/EM/W452/Korea/14 (H5N8, W452) virus (Supplementary Table 1). To analyze the replication capacity of these RG452 viruses, including WT, that harbour either individual or multiple adaptive markers, they were introduced to A549 cells at a MOI of 0.01. The RG452 viruses endowed with individual mutations, such as PB2Q591K, PB1P708S, and PAQ556R, displayed replication patterns either equivalent to or marginally superior to RG452-WT. The RG452-PB2D701N virus, however, exhibited the most pronounced replication advantage in comparison to both RG452-WT and other single mutants, at all-time points evaluated ((A,B)). The highest replicative competence was displayed by the triple mutant RG452-PB2Q591K/D701N + PB1P708S and the double mutant RG452-PB2D701N + PAQ556R, followed by the double mutant RG452-PB2Q591K/D701N ((A)). Although the combination of multiple markers in RG452 viruses generally enhanced replication compared to single mutants, the RG452 virus with the double mutations RG452-PB2Q591K/D701N interestingly exhibited a similar to slightly lower proliferation efficiency relative to the single mutant RG452-PB2D701N ((A,B)). Our observations highlight the crucial role of the PB2D701N mutation in boosting viral growth potential, typically synergizing effectively with other mutations. Nonetheless, we noted potential antagonistic replication when the PB2D701N mutation was combined with the PB2Q591K mutation. This antagonism was mitigated upon inclusion of the PB1P708S mutation, as observed in the triple mutant RG452-PB2Q591K/D701N + PB1P708S ((A,B)).
Figure 3. In vitro growth dynamics and speed of recombinant viruses with single and combined adaptive markers. (A) This panel displays the traditional In vitro growth kinetics of eight recombinant viruses, each with distinct single or combined markers, produced via reverse genetics. This kinetics are plotted over various time intervals: 12HPI, 24 HPI, 36 HPI, 48 HPI, and 60 HPI. Statistical relevance when compared to RG452-WT is signified with asterisks (*p < 0.05, **p < 0.01, ***p < 0.001, ****p < 0.0001); “ns” represents non-significance. HPI is an abbreviation for Hours Post Infection. (B) This segment showcases the logistic nonlinear regression analysis applied to recombinant RG452 viruses with adaptive markers, using time vs. titre data to derive GS values. This analysis evaluates the adaptive capacity of various RG452 markers, listing them alongside their respective titres. GS denotes growth speed.

The replication kinetics and growth potential of all these viruses can be succinctly interpreted through straightforward graphical representations. Acknowledging the advantages of quantitative comparison, we assessed replication capacity using non-linear regression analysis. Our analyses yielded R2 values ranging from 0.85 to 0.98 (Supplementary Table 4), suggesting the curves were well-fitted and displayed superior replication kinetics for all the viruses. We then computed the average growth rate spanning the duration from when an uptick in viral amount was first observed to the point where a peak viral amount was achieved. This allowed us to estimate the average hourly virus production capacity. Among the single mutants, the RG452-PB2D701N virus displayed the most accelerated growth speed (GS = 2.34). This was followed by the RG452-PB1P708S (GS = 1.53), PB2Q591K (GS = 1.49), and PAQ556R (GS = 1.33) viruses ((C)). Considering the multiple mutants, both RG452-PB2D701N + PAQ556R (GS = 2.56) and PB2Q591K/D701N + PB1P708S (GS = 2.55) viruses exhibited greater growth speed, followed by the double mutant RG452-PB2Q591K/D701N virus (GS = 2.18) ((C)). Interestingly, the GS of the double mutant RG452-PB2Q591K/D701N virus was lower than its single mutant counterpart, RG452-PB2D701N, aligning with the viral growth kinetics observed earlier ((C)). We noted that each single adaptive marker conferred unique quantitative characteristics to GS, and the introduction of additional markers influenced the speed of replication. The PAQ556R single mutant exhibited a lower GS than other single mutants. However, its GS was significantly boosted when combined with the PB2D701N mutation (RG452-PB2D701N + PAQ556R), reaching one of the highest GS values among the RG452 viruses ((C)).
Quantitative comparison utilizing competitive selection rate accurately mirrors the relative selectivity of mammalian adaptive markers
To explore further into the influence of individual and combined mammalian adaptive markers present in the HPAI H5N8 virus on the host specificity in humans, we conducted competitive selection kinetics (CSK) studies. For this, we exposed A549 human lung adenocarcinoma cells to mixtures of RG452-WT and mutant viruses in dilutions of 1:100, 1: 1,000, and 1: 10,000 and performed five sequential passages. The NGS was employed to evaluate the proportions of mutant viruses in the supernatants of the 1st, 3rd, and 5th passages. The results demonstrated that among single mutants, RG452-PB2Q591K had the least competitive edge against RG452-WT, as evident from the competitive selection observable only at the least dilution concentration of 1:100. This was followed by RG452-PB2Q556R, and RG452-PB1P708S, which showed competitive selection until a 1: 1,000 dilutions ((A)). Remarkably, the single mutant RG452-PB2D701N revealed clear competitive selection up to the highest dilution concentration of 1: 10,000 ((A)). Examining multiple mutants, it was observed that they all exhibited clear competitive selection until a 1: 10,000 dilutions. However, the triple mutant RG452-PB2Q591K/D701N + PB1P708S outperformed others by displaying the most pronounced competitive selection across all dilution concentrations ((A)). This evidence suggests that the mutation PB2D701N enhances host selectivity at lower viral concentrations more than other single mutations, and its effect is further amplified when combined with additional mutations.
Figure 4. Competitive selection and kinetics of adaptive markers in RG452 viruses. (A) Competitive Selection Dynamics: The panel displays In vitro competitive selection intensities of individual and combined adaptive markers for recombinant RG452 viruses. Selection intensities of these markers are depicted when diluted at ratios of 1:100, 1:1000, and 1:10000 against the wild-type RG virus, revealing the competitive prowess of each adaptive marker. (B) CSK: Here, the nonlinear regression analysis evaluates the selection kinetics of various RG452 adaptive markers, including RG452-WT, RG452-PB2Q591K, RG452-PB2D701N, RG452-PB1P708S, and others. Analysis was carried out at three concentration ratios: 1:100, 1:1000, and 1:10000, utilizing the index sequence value as the criterion. (C) AUC Analysis: This segment showcases the AUC plots derived from adaptation values across different MOIs. The resultant AUC values corresponding to each adaptive marker are enumerated. AUC refers to the area under the curve.

To quantify the effect of CSK on the mammalian adaptive markers, we employed a two-step quantitative conversion. We initially conducted a nonlinear regression analysis ((B)) to calculate the CSK value of all mutants, considering the viral concentration at passages for each mutant. Then, we computed the competitive selection rate (CSR) of the mutants from the AUC, using the CSK values at dilutions of 1:100, 1: 1,000, and 1: 10,000. The R² values (0.8∼0.9) obtained from the regression analysis were within acceptable limits, confirming the suitability of the model. When examining the single mutants, all displayed CSK values ranging between 60.4 and 128.6 (R² = 0.88–0.96) at the lowest dilution point (1:100) (Supplementary Table 5). However, most of them, with the exception of the single RG452-PB2D701N mutant, experienced a rapid decline in CSK values at higher dilutions (1: 1,000 and 1: 10,000) ((B) and Supplementary Table 5). On the other hand, the multiple mutants presented higher CSK values ranging from 125.4 to 340.4 (R² = 0.85–0.99) at the lowest dilution and consistently maintained higher CSK values at increased dilutions compared to the single mutants ((B) and Supplementary Table 5). Nonetheless, the double RG452-PB2Q591K/D701N mutant recorded values akin to the single RG452-PB2D701N.
The CSR value, obtained by calculating the AUC from three CSK values, effectively illustrates the relative selectivity of the mutants and further validates the effectiveness of the model ((C)). Among the single mutants, RG452-PB2D701N demonstrated the highest CSR value (CSR = 178.71), followed by RG452-PB1P708S (CSR = 110.03), RG452-PB2Q556R (CSR = 105.13), and RG452-PB2Q591K (CSR = 30.20) mutants ((C)). Observations of combinatory mutations show various effects. An antagonistic effect was observed in the double RG452-PB2Q591K/D701N mutant (CSR = 179.32). Additive effects were identified in the RG452-PB2D701N + PAQ556R mutant (CSR = 375.61), and synergistic effects were present in the triple RG452-PB2Q591K/D701N + PB1P708S mutant (CSR = 514.71).
The RNA weight model enhances the RNA scoring reliability of m, c, and vRNA through the influence of mammalian adaptive markers
Given the significance of viral RNA expression levels in influencing viral kinetic properties, we analyzed the expression of mRNA, cRNA, and vRNA across all recombinant RG452 viruses, including the WT. RG452 viruses with mammalian adaptive mutations displayed a higher RNA expression profile than the RG452-WT, whereas the RG452-PB2Q591K showed a comparable RNA profile relative to the RG452-WT. In parallel to GS and CSR, RG452-PB2D701N demonstrated the highest expression levels of cRNA and mRNA among single mutants, even though its vRNA profile was lower than that of RG452-PAQ556R. The single PAQ556R mutation was found to have a more pronounced effect on the RNA expression pattern, compared to its impact on GS and CSR. The negative synergy between the PB2Q591K and PB2D701N mutations was especially evident in the viral RNA profile, a drawback that was significantly offset by the incorporation of the PB1P708S mutation (RG452-PB2D701N/Q591K + PB1P708S) ().
Figure 5. RNA expression levels of adaptive markers in RG452 virus. (A) vRNA Expression Dynamics: This panel depicts the fold change intensity of vRNA for each single and combined adaptive marker in the RG452 virus. (B) cRNA Expression Dynamics: Similarly, this segment displays the cRNA fold change intensity associated with each adaptive marker. (C) mRNA Expression Dynamics: This section presents the mRNA fold change intensity measurements for each adaptive marker. For all panels, statistical significance when compared to RG452-WT is denoted by: “ns” for non-significant, *p < 0.05, **p < 0.01, and ***p < 0.001.

We first validated the weight of each viral RNA to accurately quantify the intra-cellular expression patterns of m, c, and vRNA, highlighting the unique influence of each RNA type on viral attributes. Using RNA fold change values from each virus and GS in our regression analysis, we obtained an R² value under 1 and achieved statistical significance (p < 0.002) (). This indicates a dependable expression profile across the three RNA types. The robustness of our model was further underscored by regression analyses of RNA fold change values associated with each virus and CSR, which consistently yielded significant R² values and p-values (p < 0.001) (). Notably, the regression values for RNA-GS and RNA-CSR in each RNA type were nearly identical, emphasizing the accuracy of RNA weight estimation through our regression approach. We then defined the average quantitative RNA-GS and RNA-CSR regression values as RNA weight. Here, cRNA registered the highest weight, trailed by mRNA and vRNA, with respective weights of 0.38, 0.34, and 0.28 ().
Table 1. RNA weight model based on three RNA types and RNA load values for single and combination adaptive markers of RG452.
An RNA load value was ascertained by evaluating the mean values of mRNA, cRNA, and vRNA for wild-type and mutant viruses. Among the single mutants, RG452-PB2D701N and RG452-PAQ556R achieved the highest RNA load values (). Although the combined PB2D701N and PAQ556R mutations led to an improved RNA load value, the enhancement was only modest (). Conversely, the RNA load value of RG452-PB2D701N/Q591K notably diminished when the PB2Q591K mutation was incorporated. This decline was significantly counteracted with the combination of triple mutations (RG452-PB2D701N/Q591K + PB1P708S), resulting in substantial improvement among both single and multiple mutants ().
The quantitative risk scores can effectively represent the magnitude of mammalian adaptive marker’s effect on the viral properties
Finally, quantitative risk scores (QRS) were derived using regression parameters associated with GS, CSR, and RNA load values. Each of these three parameters was scaled to a value of 1, allowing for a maximum cumulative value of 3. Among the single mutants, RG452-PB2D701N scored the highest, while RG452-PB2D701N + PAQ556R led among the double mutants, and the triple mutant RG452-PB2Q591K/D701N + PB1P708S scored the highest value across all other mutants ((A) and Supplementary Table 6). The model was then evaluated using a three-dimensional Euclidean distance calculation, generating QRS for all adaptive markers ((B)). PB2D701N, a dominant mutation commonly found in avian influenza viruses isolated from mammalian hosts, displayed the highest QRS (0.9623) compared to other single mutations. The triple mutant RG452-PB2Q591K/D701N + PB1P708S ranked highest (QRS = 1.7261) among all single and multiple mutants ((B)). While PB2D701N mutation alone conferred the highest QRS, it exhibited an antagonistic effect when combined with PB2Q591K (QRS = 0.8571). Conversely, the same PB2D701N mutation was linked to an additive effect when co-occurring with PAQ556R (QRS = 1.2789). The single mutations PB2D701N, PB2Q591K (QRS = 0.3652), and PB1P708S (QRS = 0.4327) demonstrated distinct QRS, and in combination, the triple mutants displayed an additive effect, achieving the highest overall QRS ((B)). The evaluation and interpretation of this model revealed that each adaptive marker can be characterized by a specific risk score, and the single digital values accurately represent the experimentally characterized viral properties. Generally, the emergence of additional mutations correspondingly increases the QRS of virus, thereby enhancing the ability to predict its adaptive potential.
Figure 6. Scaled scores and tri dimensional representation of RG452 and RG468. (A) The scaled scores of replication, competition, and RNA kinetics values of the single and combination adaptive markers of the RG452. (B) The tri dimensional representation of the single and combinational adaptive markers associated with replication, competition, and RNA kinetics of the RG452 yielded the QRS of each adaptive markers of the RG452. (C) The normalized scaled scores of replication, competition, and RNA kinetics of the single and combinational adaptive markers of the RG458 (D) The three-dimensional depiction of the single and combinational adaptive markers linked to replication, competition, and RNA kinetics within of RG468 generated the QRS of each adaptive marker of the RG468. QRS refers to the quantitative risk scores.

Cross-validation of quantitative risk scores with RG468 virus and its adaptive markers confirms perfect accuracy
The QRS model, developed and evaluated using RG452-WT and its mutants, were further cross-validated with wild-type virus RG468 and associated adaptive markers PB2E627K, PB1P708S, PAT97I, PB2E627K + PB1P708S, and PB2E627K + PAT97I. The consistency observed in the metrics generated by this model substantially contributes to its accuracy, underscoring its importance in risk assessment. In alignment with predictions from the developed model, all mutant viruses demonstrated a higher QRS compared to the RG468-WT virus ((B,C) and Supplementary Table 7). Particularly, PB2E627K, known as a major mammalian adaptive marker, conferred a higher QRS (1.2943) among single mutants. The double mutant RG468-PB2E627K + PAT97I exhibited higher QRS (1.6131) compared to RG468-PB2E627K + PB1P708S (QRS = 1.4268). In congruence with the adaptive markers observed with RG452, additive QRSs were also noted with the combination of mammalian adaptive markers, reflecting patterns observed in in vitro viral characteristics.
In vitro viral properties correlate with in vivo phenotypes providing adequate sensitivity and specificity yields for the quantitative risk scores of the various mammalian adaptive markers
The In vitro viral properties associated with mammalian adaptive markers were precisely evaluated to validate their correlation with the In vivo phenotypes of the viruses. The 50% mouse lethal dose (MLD50) was determined using viral concentrations ranging from 1 to 6 log10TCID50/mL. Survival and weight loss of infected mice were monitored over a 14-day period, with humane euthanization if their body weight fell below 80% of the initial value. Infection with the high doses (6 and 5 log10TCID50/mL) of RG452-WT virus led to peak weight loss from 8-10 dpi, with a survival rate of 40% ((A) and Supplementary Figure S1(A)). All single mutants exhibited enhanced lethality compared to RG452-WT ((A–E)). Among them, RG452-PB2Q591K was the least lethal ((B)), while RG452-PB1P708S displayed greater lethality ((D)). RG452-PB2D701N and RG452-PAQ556R were the most lethal among single mutants ((C,E)). Consistent with In vitro findings, the RG452-PB2Q591K/D701N double mutant presented similar weight loss and lethality to the single RG452-PB2D701N mutant ((C,F)). Conversely, another double mutant, RG452-PB2D701N + PAQ556R, manifested increased lethality and weight loss ((G) and Supplementary Figure S1(G)). The triple mutant RG452-PB2Q591K/D701N + PB1P708S demonstrated the most pronounced weight loss and heightened lethality at doses of 3, 4, 5, and 6 log10TCID50/mL ((H) and Supplementary S1(H)). Systematic analysis of the viral doses and their effects on weight and survival led to the calculation of MLD50 values as follows: 4.83, 4.5, 3.5, 3.83, 3.5, 3.5, 3.37, and 2.5 for RG452-WT, -PB2Q591K, -PB2D701N, -PB1P708S, -PAQ556R, -PB2Q591K/D701N, -PB2D701N + PAQ556R, and -PB2Q591K/D701N + PB1P708S, respectively.
Figure 7. Survival analysis and model evaluation for adaptive markers in BALB/c mice. (A–H) Survival Metrics of RG452 Adaptations: Each panel showcases the survival rates of BALB/c mice after inoculation with varying dilutions (from 106 to 101) of the RG452 recombinant viruses, each carrying distinct adaptive markers: (A) RG452-WT, (B) RG452-PB2Q591K, (C) RG452-PB2D701N, (D) RG452-PB1P708S, (E) RG452-PAQ556R, (F) RG452-PB2D701N/Q591K, (G) RG452-PB2D701N + PAQ556R, (H) RG452-PB2-D701N/Q591K + PB1P708S. Survival patterns were monitored for 14 dpi. The Kaplan–Meier survival plots provide insights into the survival rates for each adaptive marker group. (I) ROC Curve Analysis: The graph contrasts the false positive rate (x-axis) with the true positive rate (y-axis) to evaluate the performance of the model built on weight, multi-organ titre, and survival metrics of RG452. The AUC values for each RG virus variant, listed sequentially in the figure, shed light on the sensitivity and specificity of the associated risk scores.

To further assess systemic viral spread, mice were infected with 4 log10TCID50/mL and their organs (lungs, heart, brain, spleen, and kidney) were harvested and titrated at 5 dpi. While no systemic infection was observed in RG452-WT and -PB2Q591K-infected mice, those infected with RG452-PAQ556R and -PB1P708S showed moderate spread and low organ titres (Supplementary Figure S2). Conversely, RG452-PB2D701N had the highest viral titre in multiple organs among the single mutants. Multiple mutants, namely RG452-PB2Q591K/D701N + PB1P708S, -PB2D701N + PAQ556R, and -PB2Q591K/D701N, demonstrated escalating systemic infections in that order (Supplementary Figure S2).
In vivo assessments, which included lethality, weight loss, and systemic infection in vital organs, were used to evaluate the performance of the obtained QRS for RG452-WT and its mutant strains. Utilizing a ROC analysis, which plots sensitivity against 1-specificity, most viral strains exhibited ROC curves near top-left corner of the graph ((I)). This location signifies accurate risk discernment for each virus type. Quantitatively, the AUC values for single and multiple mutations ranged from 0.682 to 0.868 ((I)), underscoring the efficacy of our risk model in relation to In vivo observations. Moreover, sensitivity and specificity of the model crucial for prediction model evaluation, were consistently reliable across the mutant strains, enhancing the credibility of our model in assessing risks associated with mammalian adaptive markers.
Discussion
IAV, particularly the HPAI H5N8 subtype, have attracted significant attention due to their potential for cross-species transmission and adaptation. Central to these phenomena are adaptive mutations within their polymerase complex, which consists of essential proteins such as PB2, PB1, and PA. Prior research underscores the pivotal roles of these proteins in cross-species dynamics of IAV [Citation5, Citation6, Citation7, Citation20, Citation42, Citation43]. Building on this foundational knowledge, our study focuses on mutations, including the well-documented PB2 mutations like E627K and D701N [Citation15], known to have a significant influence on mammalian adaptation. Through our studies, we’ve observed the broad spectrum of adaptive mutations within the HPAI H5N8 virus. In particular, the mutations PB2D701N and PB2Q591K in infected mouse groups showcase the differential influence of these mutations on host viral dynamics. We’ve also identified an upward trend in PB2E627K and PB2D701N mutations, indicating their distinct evolutionary trajectories. Central to our efforts is understanding the subtle roles of these adaptive markers. Our goal is to provide insights into their effects on host adaptation and virulence in AIVs and to translate these findings into a reliable quantitative risk assessment.
In assessing viral growth, we observed a strong correlation between growth speed (GS) values and traditional viral growth kinetics. The R2 values, ranging from 0.85 to 0.98, affirm the reliability of our non-linear regression model for such studies. This utility of the model extends to measuring and comparing replication capacities across different mutants. For example, while the PAQ556R single mutant indicated a subdued GS, its combination with PB2D701N displayed one of the stronger GS values, suggesting intricate interactions between mutations. Mathematical modelling allowed us to quantify GS values for each viral variant, establishing a framework for assessing viral growth dynamics. Intriguingly, while some mutants like double or triple combinations showed enhanced replication abilities as previously reported [Citation44–47], the RG452 virus bearing the PB2Q591K/D701N double mutations presented an anomaly with diminished replication efficiency when contrasted with its PB2D701N single mutant counterpart. This unique observation, potentially highlighting an interplay between the two mutations influenced by PB1P708S, calls for deeper investigation.
The competitive selection kinetics studies provide an unprecedented quantitative comparison of individual and combined mammalian adaptive markers’ impact on host specificity. Furthermore, our calculated competitive selection rate (CSR) quantifies these effects, elucidating antagonistic, additive, and synergistic relationships between mutations. The finding that the RG452-PB2Q591K/D701N double mutant registered CSR values similar to the single RG452-PB2D701N mutant uncovers a potential cancellation effect. These complex interactions underscore the necessity for nuanced risk assessment and emphasize the ability of virus to adapt and evolve within the host. While our recombinant viruses were designed to limit mutation acquisition, additional mutations in the polymerase genes were observed during in vitro passages (Supplementary Table 8). Such mutations could potentially affect the growth and competitive properties of both the wild-type and mutant recombinant viruses, potentially impacting the precise competitive properties of the adaptive markers.
Understanding the roles and dynamics of three distinct types of influenza A viral RNAs (vRNA, cRNA, and mRNA) in the life cycle of the virus within mammalian cells is key to unravelling the infectious properties of virus. Building upon previous studies that analyzed the kinetic behaviour of these RNAs [Citation48–52], our work delves deeper into their cellular functions, especially in the context of potential viral adaptations. Our statistical model effectively bridges experimental viral RNA expression data with quantitative values, facilitating straightforward comparisons of overall RNA replicative properties across viruses with adaptive markers. The model consistently captures the influence of various adaptive markers on RNA levels across all RNA types, underscoring its accuracy. Furthermore, the RNA weight model introduced in this study clarifies the impact of each RNA type on viral attributes. This provides insight into the interplay between RNA expression and viral dynamics, enhancing the precision of the RNA load value. The RG452-PAQ556R mutation demonstrated a moderate impact on the GS and CSR but showed the highest RNA load value, particularly for vRNA, among the single mutants. This suggests an enhancing role in viral genome replication, potentially through interaction with the PB1 protein [Citation22]. The interaction between PB2Q591K and PB2D701N mutations was notably antagonistic in RNA load value while the triple mutant (RG452-PB2Q591K/D701N + PB1P708S) displayed significantly elevated RNA levels by adding the PB1P708S mutation. This suggests the intricate interactions between these mutations are pivotal for viral adaptation, aligning with the observations of this triple mutant post-adaptation in a mammalian model [Citation6, Citation20]. Such results underscore the distinctive impact specific mutations exert on RNA replication within the virus. The alignment between predictions of our model and the actual data validates its robustness, enriching our comprehension of the factors governing replication and competition in the influenza A virus.
We also developed a set of quantitative risk scores that serve as a valuable tool for evaluating how likely different viral strains are to adapt and cause outbreaks. The identification of a particular mutation, PB2D701N, as having the highest associated risk emphasizes its importance and suggests it could be a focal point for future surveillance and containment efforts. Validating these findings with another wild-type virus, RG468, further bolsters credibility of our model and demonstrates its broader applicability. Despite these advancements, our work leaves some questions unanswered. The utility of the model for other viral strains or host species remains to be explored, and further research could help adapt our insights to human contexts, potentially aiding in the development of more effective vaccines and antiviral therapies. Our research also acknowledges the inherent limitations posed by the nature of influenza viruses. While our study employed clonal recombinant viruses, we cannot rule out the acquisition of secondary mutations during replication in the host, which could potentially alter phenotypic outcomes. Recognizing this, we emphasize the need for future studies to delve into this area of viral evolution. Moreover, while the maH5N8 viruses used in our research belong to clade 2.3.4.4a [Citation53] and lack evidence of human infection, their rapid pathogenic evolution in mammalian models necessitates further examination. This is particularly pressing in light of recent human infections caused by H5 viruses from clade 2.3.4.4b [Citation54]. Our study’s insights provide a foundation for such investigations and highlight the importance of continuous research in tracking and understanding the dynamic nature of avian influenza viruses.
In conclusion, our study on the HPAI H5N8 virus provides critical insights into the dynamics of mammalian adaptive markers and their implications in viral pathogenesis and interspecies transmission. The comprehensive model we developed, integrating experimental data with mathematical analysis, not only enhances our understanding of H5N8 but also establishes a foundation for studying other rapidly evolving infectious agents. Our established quantitative risk scoring system offers a sophisticated approach to assessing the potential impact of specific genetic mutations on viral behaviour. This tool is particularly relevant in the current period, where rapid mutation and adaptation of viruses pose constant threats to global health. Our findings emphasize the need for ongoing monitoring and analysis of viral evolution, especially in the context of emerging zoonotic diseases. The methodologies and analytical frameworks we utilized can be adapted and applied to other infectious agents, providing a valuable repertoire for early detection, risk assessment, and strategic intervention planning. Such adaptability is vital for staying ahead of evolving pathogens and for developing timely, effective responses to emerging infectious disease threats. In a time characterized by frequent outbreaks of novel pathogens, our research adds to the collection of tools necessary for understanding and addressing these challenges. By offering a model that bridges traditional virological studies with advanced quantitative analysis, we aim to boost the predictive capabilities and preparedness of the global health community, thereby contributing to more effective strategies for managing and mitigating the impacts of infectious diseases.
Ethical statement
All animal studies were carried out under the approval of the Institutional Animal Care and Use Committee of Chungbuk National University, Republic of Korea (CBNUA-1659-22-01).
Supplementary_Revised-clean
Download MS Word (271.3 KB)Acknowledgements
M.-S. S, Y.H.B and Y.K.C conceptualized and designed the study. S.CH, S.O and W.-S. C performed experiments, analyzed data and wrote the manuscript. C.I.L and J.H.J provided assistance during the experiments. B.K.K and S.C.M assistance with data analysis. J.-H. Y and E.-G. K revised and the edited the manuscript. M.-S. S supervised the study.
Disclosure statement
No potential conflict of interest was reported by the author(s).
Additional information
Funding
References
- Kuiken T, Rimmelzwaan G, van Riel D, et al. Avian H5N1 influenza in cats. Science. 2004;306(5694):241.
- Horimoto T, Kawaoka Y. Influenza: lessons from past pandemics, warnings from current incidents. Nat Rev Microbiol. 2005;3(8):591–600.
- Pappas C, Aguilar PV, Basler CF, et al. Single gene reassortants identify a critical role for PB1, HA, and NA in the high virulence of the 1918 pandemic influenza virus. Proc Natl Acad Sci USA. 2008;105(8):3064–3069.
- Yen HL, Aldridge JR, Boon AC, et al. Changes in H5N1 influenza virus hemagglutinin receptor binding domain affect systemic spread. Proc Natl Acad Sci USA. 2009;106(1):286–291.
- Mehle A, Doudna JA. Adaptive strategies of the influenza virus polymerase for replication in humans. Proc Natl Acad Sci USA. 2009;106(50):21312–6.
- Gabriel G, Dauber B, Wolff T, et al. The viral polymerase mediates adaptation of an avian influenza virus to a mammalian host. Proc Natl Acad Sci USA. 2005;102(51):18590–5.
- Velthuis AJ T, Fodor E. Influenza virus RNA polymerase: insights into the mechanisms of viral RNA synthesis. Nat Rev Microbiol. 2016;14(8):479–493.
- Mänz B, Schwemmle M, Brunotte L. Adaptation of avian influenza A virus polymerase in mammals to overcome the host species barrier. J Virol. 2013;87(13):7200–7209.
- Mänz B, de Graaf M, Mögling R, et al. Multiple natural substitutions in avian influenza A virus PB2 facilitate efficient replication in human cells. J Virol. 2016;90(13):5928–5938.
- Arai Y, Kawashita N, Daidoji T, et al. Novel polymerase gene mutations for human adaptation in clinical isolates of avian H5N1 influenza viruses. PLoS Pathog. 2016;12(4):e1005583.
- Liu WJ, Li J, Zou R, et al. Dynamic PB2-E627K substitution of influenza H7N9 virus indicates the in vivo genetic tuning and rapid host adaptation. Proc Natl Acad Sci USA. 2020;117(38):23807–23814.
- Zhang H, Li X, Guo J, et al. The PB2 E627K mutation contributes to the high polymerase activity and enhanced replication of H7N9 influenza virus. J Gen Virol. 2014;95(4):779–786.
- Cheng K, Yu Z, Chai H, et al. PB2-E627K and PA-T97I substitutions enhance polymerase activity and confer a virulent phenotype to an H6N1 avian influenza virus in mice. Virology. 2014;468:207–213.
- Sediri H, Schwalm F, Gabriel G, et al. Adaptive mutation PB2 D701N promotes nuclear import of influenza vRNPs in mammalian cells. Eur J Cell Biol. 2015;94:368–374.
- Zhou B, Pearce MB, Li Y, et al. Asparagine substitution at PB2 residue 701 enhances the replication, pathogenicity, and transmission of the 2009 pandemic H1N1 influenza A virus. PLoS One. 2013;8(6):e67616.
- Wang C, Lee HH, Yang ZF, et al. PB2-Q591K mutation determines the pathogenicity of avian H9N2 influenza viruses for mammalian species. PLoS One. 2016;11(9):e0162163.
- Li Q, Wang X, Sun Z, et al. Adaptive mutations in PB2 gene contribute to the high virulence of a natural reassortant H5N2 avian influenza virus in mice. Virus Res. 2015;210:255–263.
- Wu H, Peng X, Peng X, et al. Multiple amino acid substitutions involved in the adaptation of avian-origin influenza A (H10N7) virus in mice. Arch Virol. 2016;161:977–980.
- Zhou B, Li Y, Halpin R, et al. PB2 residue 158 is a pathogenic determinant of pandemic H1N1 and H5 influenza a viruses in mice. J Virol. 2011;85(1):357–365.
- Choi WS, Baek YH, Kwon JJ, et al. Rapid acquisition of polymorphic virulence markers during adaptation of highly pathogenic avian influenza H5N8 virus in the mouse. Sci Rep. 2017;7(1):40667.
- Zhao Y, Yu Z, Liu L, et al. Adaptive amino acid substitutions enhance the virulence of a novel human H7N9 influenza virus in mice. Vet Microbiol. 2016;187:8–14.
- Ping J, Keleta L, Forbes NE, et al. Genomic and protein structural maps of adaptive evolution of human influenza A virus to increased virulence in the mouse. PLoS One. 2011;6(6):e21740.
- Eng CL, Tong JC, Tan TW. Distinct host tropism protein signatures to identify possible zoonotic influenza A viruses. PLoS One. 2016;11(2):e0150173.
- Eng CL, Tong JC, Tan TW. Predicting zoonotic risk of influenza A viruses from host tropism protein signature using random forest. Int J Mol Sci. 2017;18(6):1135.
- Qiang X, Kou Z, Fang G, et al. Scoring amino acid mutations to predict avian-to-human transmission of avian influenza viruses. Molecules. 2018;23(7):1584.
- Smith AM. Host-pathogen kinetics during influenza infection and coinfection: insights from predictive modeling. Immunol Rev. 2018;285(1):97–112.
- Wang J, Kou Z, Duan M, et al. Using amino acid factor scores to predict avian-to-human transmission of avian influenza viruses: a machine learning study. Protein Pept Lett. 2013;20(10):1115–1121.
- Smith AM, Adler FR, McAuley JL, et al. Effect of 1918 PB1-F2 expression on influenza A virus infection kinetics. PLoS Comput Biol. 2011;7(2):e1001081.
- Heldt FS, Frensing T, Pflugmacher A, et al. Multiscale modeling of influenza A virus infection supports the development of direct-acting antivirals. PLoS Comput Biol. 2013;9(11):e1003372.
- Mitchell H, Levin D, Forrest S, et al. Higher level of replication efficiency of 2009 (H1N1) pandemic influenza virus than those of seasonal and avian strains: kinetics from epithelial cell culture and computational modeling. J Virol. 2011;85(2):1125–1135.
- Beauchemin C, Samuel J, Tuszynski J. A simple cellular automaton model for influenza A viral infections. J Theor Biol. 2005;232(2):223–234.
- Baccam P, Beauchemin C, Macken CA, et al. Kinetics of influenza A virus infection in humans. J Virol. 2006;80(15):7590–7599.
- Beauchemin CA, McSharry JJ, Drusano GL, et al. Modeling amantadine treatment of influenza A virus in vitro. J Theor Biol. 2008;254(2):439–451.
- Handel A, Longini Jr IM, Antia R. Towards a quantitative understanding of the within-host dynamics of influenza A infections. J R Soc Interface. 2010;7(42):35–47.
- Holder BP, Beauchemin CA. Exploring the effect of biological delays in kinetic models of influenza within a host or cell culture. BMC Public Health. 2011;11:1–5.
- Möhler L, Flockerzi D, Sann H, et al. Mathematical model of influenza A virus production in large-scale microcarrier culture. Biotechnol Bioeng. 2005;90(1):46–58.
- Saenz RA, Quinlivan M, Elton D, et al. Dynamics of influenza virus infection and pathology. J Virol. 2010;84(8):3974–3983.
- Hoffmann E, Stech J, Guan Y, et al. Universal primer set for the full-length amplification of all influenza A viruses. Arch Virol. 2001 Dec;146:2275–2289.
- Kawakami E, Watanabe T, Fujii K, et al. Strand-specific real-time RT-PCR for distinguishing influenza vRNA, cRNA, and mRNA. J Virol Methods. 2011;173(1):1–6.
- Hanley JA, McNeil BJ. The meaning and use of the area under a receiver operating characteristic (ROC) curve. Radiology. 1982;143(1):29–36.
- Taft AS, Ozawa M, Fitch A, et al. Identification of mammalian-adapting mutations in the polymerase complex of an avian H5N1 influenza virus. Nat Commun. 2015;6(1):7491.
- Wu H, Peng X, Lu R, et al. Virulence of an H5N8 highly pathogenic avian influenza is enhanced by the amino acid substitutions PB2 E627K and HA A149V. Infect Genet Evol. 2017;54:347–354.
- Song MS, Pascua PN, Lee JH, et al. The polymerase acidic protein gene of influenza a virus contributes to pathogenicity in a mouse model. J Virol. 2009;83(23):12325–12335.
- Czudai-Matwich V, Otte A, Matrosovich M, et al. PB2 mutations D701N and S714R promote adaptation of an influenza H5N1 virus to a mammalian host. J Virol. 2014;88(16):8735–8742.
- Yang F, Zhang X, Liu F, et al. Rapid emergence of a PB2 D701N substitution during adaptation of an H9N2 avian influenza virus in mice. Arch Virol. 2022;167(11):2299–2303.
- Arai Y, Kawashita N, Ibrahim MS, et al. PB2 mutations arising during H9N2 influenza evolution in the Middle East confer enhanced replication and growth in mammals. PLoS Pathog. 2019;15(7):e1007919.
- Song W, Wang P, Mok BW, et al. The K526R substitution in viral protein PB2 enhances the effects of E627K on influenza virus replication. Nat Commun. 2014;5(1):5509.
- Vester D, Lagoda A, Hoffmann D, et al. Real-time RT-qPCR assay for the analysis of human influenza A virus transcription and replication dynamics. J Virol Methods. 2010;168(1–2):63–71.
- Luo GU, Bergmann M, Garcia-Sastre A, et al. Mechanism of attenuation of a chimeric influenza A/B transfectant virus. J Virol. 1992;66(8):4679–4685.
- Robb NC, Smith M, Vreede FT, et al. NS2/NEP protein regulates transcription and replication of the influenza virus RNA genome. J Gen Virol. 2009;90(6):1398–1407.
- Karlas A, Machuy N, Shin Y, et al. Genome-wide RNAi screen identifies human host factors crucial for influenza virus replication. Nature. 2010;463(7282):818–822.
- Kawakami E, Watanabe T, Fujii K, et al. Strand-specific real-time RT-PCR for distinguishing influenza vRNA, cRNA, and mRNA. J Virol Methods. 2011;173(1):1–6.
- Chang N, Zhang C, Mei X, et al. Novel reassortment 2.3. 4.4 b H5N8 highly pathogenic avian influenza viruses circulating in Xinjiang, China. Prev Vet Med. 2022;199:105564.
- Gomaa M, Moatasim Y, El Taweel A, et al. We are underestimating, again, the true burden of H5N1 in humans. BMJ Global Health. 2023;8:8.