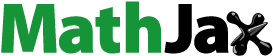
Abstract
In sports and rehabilitation settings, although haptic guidance is a general method for facilitating motor learning, the effects of verbal instruction on motor learning remain unclear when using haptic guidance. This study thus investigated the influence of verbal instruction on a novel visuo-motor task presented before practice, while also implementing haptic guidance. Thirty participants, who performed a mirror drawing task, were randomly allocated to three groups: control—without augmented verbal instruction, HG-with relying (HG-with)—instructed to rely on guidance devices, and HG-without relying (HG-without)—instructed not to rely on guidance devices. We assessed the tracing time and error area of the mirror drawing task as performance measurements. The self-made guidance devices used in the practice session were constructed of 2-mm-thick cardboard and had a 5-mm-wide groove that matched the shape of the target figure. The tracing time in HG-with condition during the practice session (median = 66.5 s, IQR = 55.3–80.5 s) was significantly shorter compared with that in control condition (median = 132.5 s, IQR = 125.0–144.8 s) (p = .019). The error area (median = 585.0 mm2, IQR = 559–687.0 mm2) was also significantly larger in the HG-with condition than in control condition (median = 413.5 mm2, IQR = 350.5–494.0 mm2) (p = .029). Additionally, HG-with condition (mean = 46 %, SD = 12.3 %) showed greater improvement rate for tracing time from pre-test to post-test than HG-without condition (mean = 30 %, SD = 8.3 %) (p = .032). Our results suggest that, even if the same haptic guidance was implemented, visuo-motor task improvement was influenced by verbal instructions.
1. Introduction
In rehabilitation and sports, various forms of manipulations have been applied in attempts to improve motor skills; these include augmentations to feedback modalities (Sigrist et al., Citation2013a), feedback timing (Sigrist et al., Citation2013b; Vander Linden et al., Citation1993), feedback frequencies (Winstein & Schmidt, Citation1990; Wulf, Shea, et al., Citation1998), practice schedule (Hebert et al., Citation1996), and attentional focus (Wulf et al., Citation2001; Wulf, Hoss, et al., Citation1998). Recently, haptic guidance (also known as forced response or response restriction) (Holding & Macrae, Citation1964), which involves physically guiding a participant’s movement using a robotic interface/device, has been practiced in sports and physical education and has attracted attention in rehabilitation settings, including in robotic rehabilitation (Liu et al., Citation2018; Marchal-Crespo et al., Citation2019; Williams & Carnahan, Citation2014). The effects of haptic guidance on motor learning were inconsistent in previous studies. For example, Marchal-Crespo and Reinkensmeyer reported that haptic guidance improved steering skill in a driving task (Marchal Crespo & Reinkensmeyer, Citation2008). By contrast, Feygin et al. (Citation2002) reported that the haptic guidance training group was less effective than the visual training group with respect to the accuracy of the desired trajectory in a tracing-a-complex-3-D trajectory task. There were reports that the effects of haptic guidance on motor learning are influenced by the method of guidance (Marchal-Crespo et al., Citation2010; Milot et al., Citation2010), amount of guidance (Marchal-Crespo & Reinkensmeyer, Citation2009), learner’s skill level (Marchal-Crespo et al., Citation2010), and the interaction between the method of guidance and task characteristic (Heuer & Luttgen, Citation2015; Marchal-Crespo et al., Citation2015).
A previous study reported that verbal instruction and visual feedback could change muscle activities (electromyogram) between conditions with the same haptic guidance presented (Israel et al., Citation2006). Additionally, some studies indicated that haptic guidance, including haptic feedback, may change the strategy of motor task to achieve a goal (Danion et al., Citation2012; Gibo & Abbink, Citation2016). Specifically, the participants were asked to grasp a haptic device and manipulate it along one degree of freedom (forward and backward movement on a horizontal plane). The movement of the haptic device was displayed on a monitor as a “hand.” In addition, a mass-spring system was attached to the hand as an “object.” In other words, the hand and object were virtually connected on the monitor. The participants were required to bring both the hand and object displayed on the monitor from the start position to target position by manipulating a haptic device as quickly as possible (within 3 seconds). As the object oscillates owing to the mass-spring system attached to the hand, this task differs from a simple reaching task. Haptic guidance was used to provide successful movements. During the task, Gibo and Abbink (Citation2016) observed that the participants’ experience of haptic guidance changed the way in which the task was performed—the haptic-guidance group adopted a movement strategy that involved faster movement compared with the no-haptic-guidance group. Therefore, the usage of haptic guidance varies within individuals, and this difference is assumed to affect motor learning.
Verbal instruction is a “commonly used means of communicating to people how to perform motor skill for learning” (Magill & Anderson, Citation2021, pp. 326–351). Indeed, there are some studies that reported that verbal instruction affects motor learning (Chow et al., Citation2014; Wulf & Weigelt, Citation1997). Therefore, verbal instruction might be able to control how learners utilize haptic guidance and be a factor that affects motor learning in the condition in which haptic guidance is implemented. However, there are no studies that investigated the effect of instruction on motor learning in the condition wherein haptic guidance is implemented. We assumed that motor learning effects differ between the conditions in which the same haptic guidance was presented but different verbal instructions were presented. This study aimed to investigate the differences of motor learning effects of verbal instruction in the condition in which haptic guidance is implemented.
2. Materials and methods
2.1. Participants
We recruited 30 healthy, right-handed adults (13 males and 17 females) with an average age of 24.3 ± 3.1 years using flyers and electronic bulletin boards; none had any neurological or orthopedic disorders that impaired their writing. Additionally, none of the participants had previously performed the study task applied in this research. As no previous studies had used a similar motor task or experimental design as those used in this study, we could not perform an a priori power analysis. Therefore, we referred to some existing studies (Manuel, Nicastro, et al., Citation2018; Sada, Citation1998) that adopted the mirror-drawing task as a motor task and had a relatively similar number of trials to the present study. As the sample size for these previous studies ranged from 9 to 12 participants in each group, we set our sample size at ten participants per group as well. A post-hoc power analysis on the obtained data for analyzing the change rate (main outcome) showed that the power was 0.61 (tracing time) and 0.66 (error area). Although our sample size is somewhat small, the effect sizes of the change rate (tracing time; η2 = .211) and performance (tracing time; η2 = .290, error area; η2 = .234) were relatively high. Therefore, despite the limitation of the small sample size, we believe that our study will demonstrate the effects of presenting different verbal instructions. Before commencing the experiment, all participants were informed of the purpose and method of the research, and they provided oral and written consent. This study was approved by the Ethical Review Board of the Ibaraki Prefectural University of Health Sciences (approval no. 925).
3. Task and apparatus
The motor task applied in this study was a mirror-drawing task (Manuel, Guggisberg, et al., Citation2018; Manuel, Nicastro, et al., Citation2018; Sada, Citation1998). Each participant was seated in a chair in front of a desk (Figure ). A sheet of paper with a random line figure (210 × 297 mm, length of the trajectory: 717.0 mm) of 0.7-mm width was placed below the participant’s right hand (Figure ), and a mirror was placed behind the sheet, perpendicular to the desk. A shield was situated on the desk so that the participant could not directly see their own hand or the figure. The participant held a pen (0.7 mm nib) with their right hand and was asked to “trace the figure as quickly and accurately as possible” while viewing their own hand and the figure through the mirror. The starting position for the drawing was the point closest to the participant, and the end position was the point furthest from the participant. Tracing from the start position to the end position represented one trial. During the measurement, only the participant’s right hand was videorecorded using digital video camera (Kenuo, China).
Figure 1. Experimental setting and test figures. The figure on the left shows an experimental setting. Participant sits on a chair and a mirror is set in front of the participant. In order to prevent from seeing own hand and figure, the shield was set. (a) The figure was used in practice session and participants traced from start position in the front to end position in the back. In test sessions, this figure was presented at 90 degrees leftward rotation (ie., the start position was right, and the end position was left). Although the figure was the same in practice and test sessions, tracing direction of figure was different in each session. (b) The figure used in the familiarization trial before the pre-test.

In this study, we used a self-made haptic guidance device (Figure ). This device was constructed of a 2-mm-thick cardboard and had a 5-mm-wide groove that matched the shape of the target figure. By using the device while performing the mirror-drawing task, participants could forcibly suppress errors of more than 2.5 mm from each side of the target line. The width of the groove was set after confirming the error area for which errors could be suppressed forcibly prior to the study. When using a guidance device, the error area does not exceed a certain value, and it is easy to maintain a constant performance; further, it is possible to shorten the tracing time by following the guidance device. Thus, by using the guidance device it was possible to adopt a motor strategy to shorten the tracing time while maintaining the error area at a certain value.
Figure 2. Guidance device. This figure shows a target line (solid line), guidance device (2 mm high wall on both sides to the target line) and a pen.

Participants’ performance in the task was determined by measuring the time taken to trace the target line (tracing time) and the area outside the target line (error area). Tracing time was calculated using the abovementioned video camera (with a sampling rate of 60 Hz). In the present study, the error area was defined as the area between the target line and the line traced by the participants from the start position to the end position. The traced figure was imported to an image-analysis software (ImageJ) to calculate the error area (unit: mm2). Lower tracing time and error area indicated better performance.
4. Procedure
Thirty participants were randomly divided into three groups (HG-without relying, HG-with relying, and Control). Both HG-without relying and HG-with relying groups used the haptic guidance device in the practice session. The HG-without relying group was instructed during the practice trials to “trace the figure while avoiding relying on the guidance device as much as possible,” and participants of HG-with relying were informed that “you can use the guidance device to help you trace the figure.” These verbal instructions were provided immediately before the practice session. The control group did not use a guidance device. Before performing the task, all groups were instructed to “trace as quickly and accurately as possible.”
The measurements in this study were performed over two consecutive days. On the first day, a two-minute familiarization trial was conducted using the figure shown in Figure . Next, as a pre-test, three trials were conducted featuring the figure shown in Figure , which was presented at a 90° leftward rotation. Then, under the practice conditions described previously, six practice trials (experimental trials) were conducted using the figure from Figure (not rotating Figure ). On the second day, a post-test was conducted using the same content as the pre-test. In all test trials, the measurement was performed without the guidance device, regardless of the practice conditions. In the practice trials, the inter-trial interval was set at one minute, and feedback was given by presenting the figure that the participant had traced in that trial and by verbally informing their tracing time. For the test trials, one minute was set as the inter-trial interval, and no feedback on task performance was provided during the trials. As the same figure was used in the test trials and practice trials, this created the possibility that, for the condition in which the practice was conducted without the guidance device (control group), the test trials would be practically the same as the practice trial; this would mean that the participants in this condition conducted the practice three times more often than those in the other condition. To avoid this inconsistency between the conditions, we altered the orientation of the figure in the test and practice trials.
5. Statistical analysis
When a normal distribution of the tracing time and error area was observed, a mixed-ANOVA was conducted on the tracing time and error area. In contrast, when a normal distribution was not observed (the log-transformed parameters also failed to show a normal distribution), a one-way ANOVA was performed after recalculating the change rate of the measurements (tracing time and error area) and confirming normality. As there is no nonparametric test for mixed-ANOVA, this recalculated index was used in the analysis of this study. The change rate was calculated using Equation 1 (Lingo VanGilder et al., Citation2018). This index is less sensitive to high and low performance in the pre-test. When significant main effects or interaction in mixed-ANOVA for tracing time or error area were found, post-hoc tests (Tukey or Bonferroni method) were conducted. Alternatively, when main effects in one-way ANOVA for change rate of tracing time or error area were found, post-hoc tests (Tukey method) were conducted.
Additionally, measurements from the six practice trials were averaged for each participant. When a normal distribution of tracing time and error area was observed, one-way ANOVA was performed. Otherwise, Kruskal-Wallis tests were performed to compare the three practice conditions in the practice session for tracing time and error area. When significant main effects were found, post-hoc tests (Bonferroni method) were conducted.
For statistical analysis, we used G*Power 3.1.9.7 for a post-hoc power analysis, R version 4.1.2 for calculating effect size, and IBM SPSS Statistics version 28 (IBM Corp, Armonk, NY) for other analyses, with a significance level of 5%.
6. Results
The Shapiro—Wilk test showed that the tracing time of the post-test was not a normal distribution (p < .001). Therefore, we calculated the change rate of both the tracing time and error area, and conducted further analysis.
The tracing times and error areas in the pre-test, practice session, and post-test are shown in box plots (Figure : tracing time, Figure : error area). Additionally, representative data (images) during practice session are shown in Figure .
Figure 3. Results of the tracing time from the pre-test to the post-test. This box plot shows the median and inter-quartile range. Dot: control, vertical line: HG-without relying, and thick dot: HG-with relying group.

Figure 4. Results pertaining to the error area from the pre-test to the post-test. This box plot shows the median and inter-quartile range. Dot: control, vertical line: HG-without relying, and thick dot: HG-with relying group.

Figure 5. Representative data from the early practice session to the post-test for each group with tracing time data. Left figure: control group, central figure: HG-without relying group, right figure: HG-with relying group. Upper line: early practice session, Lower line: post-test.

7. Change rate
Before analysis, we tested the Gaussian distribution of the change rate of the tracing time and error area using the Shapiro—Wilk test. Both parameters were normally distributed (all p > .05). Therefore, we analyzed the change rate using an ANOVA. A significant main effect was found between groups (F2, 27 = 3.613, p = .041, η2 = .211) in terms of tracing time. Multiple comparisons using Tukey method revealed a significant difference between the HG-without relying and HG-with relying (p = .032) conditions. This indicated that the change rate of tracing time in the HG-with relying condition demonstrated a better improvement compared to the HG-without relying condition. In the error area, however, a significant main effect was not found (F2, 27 = 1.357, p = .275, η2 = .091). The change rates of all groups are shown in Table .
Table 1. Average (standard deviation) of change rate for tracing time and error area
8. Performance in practice
A significant main effect was found for conditions in both tracing time (p = .015, η2 = .290) and error area (p = .034, η2 = .234) in the practice session. Multiple comparisons using Bonferroni method revealed that HG-with relying had a significantly shorter tracing time compared to control (p = .019) as well as a larger error area compared to control (p = .029). Both performance variables in the practice session are shown in Table .
Table 2. Median (inter-quartile range) of the tracing time and error area during the practice session
9. Discussion
The present study aimed to investigate the effect of differences in verbal instruction on motor change in conditions wherein the same haptic guidance was implemented. The results showed that the change rate of the tracing time varied between the conditions in which the same haptic guidance was implemented, however, the error areas did not significantly differ between the conditions. Additionally, in the practice sessions, the HG-with relying condition, which instructed to “use the guidance device to help you trace the figure,” had a shorter tracing time and larger error area compared to the control condition.
In this study, although the HG-without relying and HG-with relying conditions involved implementing the same guidance device, only the HG-with relying condition demonstrated shorter tracing time and larger error area in the practice session compared to the control condition. This result that verbal instruction altered the performance was consistent with a previous study (Israel et al., Citation2006). In the previous study, a robotic device was applied to participants with incomplete spinal cord injuries during walking, and different verbal instructions were presented. This study demonstrated that different verbal instruction changed muscle activities of the lower extremities during walking despite the use of the same robotic guidance. In the present study, differences of verbal instruction also caused a change of performance (tracing time and error area). The reason that the HG-with relying condition had shorter tracing time and larger error area than the control condition was assumed to be that the verbal instruction of the HG-with relying condition made participants trace along with the guidance device. This device was not able to suppress the error area completely. In contrast, in the control condition, participants were unable to trace figures along with the guidance device. Therefore, the control condition demonstrated longer tracing time and smaller error area than the HG-with relying condition. In this way, the phenomenon of a negative relationship between tracing time and error area is known as a speed-accuracy trade-off (Magill & Anderson, Citation2021). Although the HG-without relying condition also involved implementing a guidance device that was used in the HG-with relying condition, tracing time of the condition was not sufficiently shortened compared to the HG-with relying condition. We considered that this can be explained by participants in the HG-without relying condition being instructed not to rely on the guidance device.
In this study, it was demonstrated that the change rate of tracing time in the HG-with relying condition showed greater improvement compared to the HG-without relying condition, although the error area was not significantly different. These results are consistent with previous studies (Feygin et al., Citation2002; Lüttgen & Heuer, Citation2012; Marchal Crespo & Reinkensmeyer, Citation2008; Marchal-Crespo et al., Citation2013), which reported that haptic guidance was effective for the temporal aspect but not for the spatial aspect of task characteristic. Specifically, Feygin et al (Feygin et al., Citation2002). reported that presenting visual feedback facilitated motor learning of spatial aspect in a task that requires tracing a desired path accurately, whereas haptic guidance did not facilitate motor learning. Additionally, Adams et al. (Adams et al., Citation1975) reported that visual feedback contributes to improving the accuracy of reproducing prescribed paths compared to presenting haptic guidance. These two previous studies adopted a continuous task like in the present study. Like these previous studies, all conditions of the present study could utilize visual feedback in practice and test sessions regardless of whether haptic guidance was implemented. According to these prior studies and results of present study, it appears that the presented visual feedback caused change rates of error area (spatial aspect) to be comparable in all conditions.
On the other hand, with respect to the change rate of tracing time, the HG-with relying group demonstrated more improvement than the HG-without relying group. The HG-with relying group had only performed in a shorter tracing time compared to the control condition during the practice session (Table ). Marchal-Crespo et al (Marchal-Crespo et al., Citation2013). reported an effect of haptic guidance on learning to follow a velocity profile in a tracking task. Their results indicated that kinesthetic experiences of the target of a task assisted by haptic guidance may effectively work for learning. Therefore, in the present study, it seems that kinesthetic experience was assisted by the guidance device, which facilitated shortening the tracing time.
However, there are several studies which are inconsistent with the results of the present study. These prior studies reported that haptic guidance hindered motor learning (Sidaway et al., Citation2008; Tsutsui & Imanaka, Citation2003; Winstein et al., Citation1994; Yamaguchi et al., Citation2020). For instance, Yamaguchi et al (Yamaguchi et al., Citation2020). reported that excessive guidance hindered motor learning. In previous studies, it was reported that error between actual movement and desired movement (Krakauer et al., Citation1999) and voluntary movement (Lotze et al., Citation2003) are necessary for motor learning. Therefore, haptic guidance which makes participants depend on them would lead to excessive reduction of errors or suppression of voluntary movement. However, the HG-with relying condition, which gave permission to use the guidance device, showed a greater improvement in their performance compared to the HG-without relying condition, which instructed to avoid relying on the guidance device as much as possible. This result may be explained by the challenge point framework (Guadagnoli & Lee, Citation2004). This perspective assumes the functional task difficulty, which is determined by the relationship between the amount of given information and capability of information processing. This framework states that a too high or too low functional task difficulty decreases learning efficacy, and there is theoretically an optimal task difficulty for learning efficacy (optimal challenge point). If the capability of information processing is constant, learning efficiency is considered to be influenced by the amount of information given. In this study, we adopted the mirror-drawing task as motor task. Using the mirror-drawing task, Manuel, Guggisberg, et al. (Citation2018) reported that healthy participants showed greater improvement in a normal tracing task compared with others. These results suggest that for healthy individuals, further improvement can be expected with practice using the mirror-drawing task than the normal tracing task. Additionally, the image seen through the mirror is upside down in terms of the actual image. Therefore, when performing a tracing task, the learner must transform the spatial coordinates of the visual system and the spatial coordinates of the motor system, and as the mirror drawing task provides different visuospatial coordinates than usual, the learner is also required to engage in sensorimotor remapping. These facts and previous studies suggest that the mirror-drawing task is a relatively challenging motor task for healthy individuals. Therefore, based on the challenge point framework, we assumed that because the amount of information to be processed had increased for the HG-without relying group, the functional task difficulty for this group would have shifted from the optimal challenge point toward too difficult compared with the HG-with relying condition. Consequently, the HG-with relying group demonstrated a better change rate for tracing time than the HG-without relying group. If a simple visuomotor task such as a normal tracing or reaching task, was conducted in this study instead of a complex sensorimotor-remapping task, the functional task difficulty of the HG-with relying group would have shifted from the optimal challenge point to too easy because of a reduction in the amount of information to be processed. Hence, the participants in the HG-with relying group would have shown less improvement than the other groups. From the perspective of the challenge point framework, the utilization of haptic guidance is also considered important for motor learning, in addition to nominal task difficulty, participants’ skill levels, and the type or amount of haptic guidance (the environment).
This study demonstrated that verbal instruction in a condition implementing haptic guidance influences the change rate, especially the temporal aspect (tracing time). However, there are some limitations. First, although we tried to control the utilization of haptic guidance by verbal instruction, we did not confirm whether participants performed according to the verbal instruction. We showed that participants in the HG-with relying demonstrated shorter tracing time and larger error area compared to the control condition. This indicates that participants in the HG-with relying condition depended on the guidance device in the practice session. However, it was not possible to show dependency on the guidance device for each individual. Thus, while it is inferred that participants in the HG-with relying condition were dependent on the guidance device, it is unclear whether they were actually dependent on it.
The second limitation is that we did not measure tendency in individual practice sessions. The mirror-drawing task adapted in this study requires fast and accurate movement. Speed and accuracy interact with each other; in other words, increasing the speed results in decreasing accuracy, and vice versa. This phenomenon is commonly known as trade-off. In the HG-without relying condition, it was assumed that participants tended to be more accurate rather than fast, and on the other hand, that participants in the HG-with relying tended to be faster rather than accurate. However, although we found these tendencies in the present study, we did not measure actual tendencies within participants.
The third limitation is that we failed to measure functional task difficulty within all conditions. Theoretically, verbal instruction or haptic guidance would influence functional task difficulty. The relation between functional task difficulty and potential learning benefit is inverted U-shaped. From the results of the present study, it was considered that both the HG-with relying and HG-without relying conditions were far from the optimal challenge point (peak of inverted U-shaped), and that the HG-without relying condition was farther from the optimal challenge point. If we successfully measure functional task difficulty like in a previous study (Akizuki & Ohashi, Citation2015), the above hypothesis would be proven. To set up an optimal practice environment for motor learning, future research which overcomes these limitations is needed.
The fourth limitation is that we did not measure the psychological characteristics of the participants. The HG without-relying group was instructed to not rely on a guidance device. Therefore, the participants may have felt some pressure or high cognitive demand. However, the HG with-relying group may have felt more confident or enjoyment upon receiving feedback (showing a shorter tracing time than the control group). Past studies have reported that using haptic guidance increased enjoyment during a task (Crespo et al., 2017) and decreased task frustration (Yamaguchi et al., Citation2020). However, although these psychological variables may also affect motor learning, they were not measured in the present study. Future studies should measure the psychological characteristics of the participants in response to verbal instruction when haptic guidance is provided.
The final limitation of this study is its small sample size, which may have reduced the statistical power of the findings. Therefore, future studies should investigate parameters with a non-significant difference. However, the change rate of tracing time and error area during the practice session demonstrated a relatively larger effect size. Therefore, despite the small sample size, this study could demonstrate the effects of different verbal instruction.
10. Conclusion
The present study showed that verbal instruction with haptic guidance influenced the effect of motor learning. It further indicated that even if the same haptic guidance is implemented, the effect of motor learning may vary with verbal instruction.
Disclosure statement
No potential conflict of interest was reported by the author(s).
Data availability statement
The data that support the findings of this study are available from corresponding author Kazuto Yamaguchi upon reasonable request through email: [email protected].
Additional information
Notes on contributors
Kazuto Yamaguchi
Kazuto Yamaguchi is an Assistant Professor at Nihon Institute of Medical Science. His research interests are motor learning, motor control and rehabilitation.
Kazunori Akizuki
Kazunori Akizuki is a Senior Assistant Professor at Mejiro University. His research interests include motor learning, motor control and rehabilitation.
Ryohei Yamamoto
Ryohei Yamamoto is an Associate Professor at Kumamoto Health Science University. His research interests include motor learning, motor control and rehabilitation.
Jun Yabuki
Jun Yabuki is a physical therapist in Ibaraki Prefectural University of Health Sciences Hospital. His research interests include motor learning, motor control and rehabilitation.
Yukari Ohashi
Yukari Ohashi is an Emeritus Professor at Ibaraki Prefectural University of Health Sciences. Her research interests include experimental psychology, motor control, motor learning and rehabilitation.
References
- Adams, J. A., Gopher, D., & Lintern, G. (1975). The Effects of Visual and Proprioceptive Feedback on Motor Learning. Proceedings of the Human Factors Society Annual Meeting, 19(2), 162–12. https://doi.org/10.1177/154193127501900204
- Akizuki, K., & Ohashi, Y. (2015). Measurement of functional task difficulty during motor learning: What level of difficulty corresponds to the optimal challenge point? Human Movement Science, 43, 107–117. https://doi.org/10.1016/j.humov.2015.07.007
- Chow, J. Y., Koh, M., Davids, K., Button, C., & Rein, R. (2014). Effects of different instructional constraints on task performance and emergence of coordination in children. European Journal of Sport Science, 14(3), 224–232. https://doi.org/10.1080/17461391.2013.780097
- Danion, F., Diamond, J. S., & Flanagan, J. R. (2012). The role of haptic feedback when manipulating nonrigid objects. Journal of Neurophysiology, 107(1), 433–441. https://doi.org/10.1152/jn.00738.2011
- Feygin, D., Keehner, M., & Tendick, R. (2002). Haptic guidance experimental evaluation of a haptic training method for perceptual motor skill. Proceedings 10th Symposium on Haptic Interfaces for Virtual Environment and Teleoperator Systems, HAPTICS 2002, (pp. 40–47). https://doi.org/10.1109/HAPTIC.2002.998939
- Gibo, T. L., & Abbink, D. A. (2016). Movement strategy discovery during training via haptic guidance. IEEE Transactions on Haptics, 9(2), 243–254. https://doi.org/10.1109/TOH.2016.2516984
- Guadagnoli, M. A., & Lee, T. D. (2004). Challenge point: A framework for conceptualizing the effects of various practice conditions in motor learning. Journal of Motor Behavior, 36(2), 212–224. https://doi.org/10.3200/JMBR.36.2.212-224
- Hebert, E. P., Landin, D., & Solmon, M. A. (1996). Practice schedule effects on the performance and learning of low- and high-skilled students: An applied study. Research Quarterly for Exercise & Sport, 67(1), 52–58. https://doi.org/10.1080/02701367.1996.10607925
- Heuer, H., & Luttgen, J. (2015). Robot assistance of motor learning: A neuro-cognitive perspective. Neuroscience & Biobehavioral Reviews, 56, 222–240. https://doi.org/10.1016/j.neubiorev.2015.07.005
- Holding, D. H., & Macrae, A. W. (1964). Guidance, restriction and knowledge of results. Ergonomics, 7(3), 289–295. https://doi.org/10.1080/00140136408930748
- Israel, J. F., Campbell, D. D., Kahn, J. H., & Hornby, T. G. (2006). Metabolic costs and muscle activity patterns during robotic- and therapist-assisted treadmill walking in individuals with incomplete spinal cord injury. Physical Therapy, 86(11), 1466–1478. https://doi.org/10.2522/ptj.20050266
- Krakauer, J. W., Ghilardi, M. F., & Ghez, C. (1999). Independent learning of internal models for kinematic and dynamic control of reaching. Nature Neuroscience, 2(11), 1026–1031. https://doi.org/10.1038/14826
- Lingo VanGilder, J., Hengge, C. R., Duff, K., & Schaefer, S. Y. (2018). Visuospatial function predicts one-week motor skill retention in cognitively intact older adults. Neuroscience Letters, 664, 139–143. https://doi.org/10.1016/j.neulet.2017.11.032
- Liu, L. Y., Li, Y., & Lamontagne, A. (2018). The effects of error-augmentation versus error-reduction paradigms in robotic therapy to enhance upper extremity performance and recovery post-stroke: A systematic review. Journal of Neuroengineering and Rehabilitation, 15(1), 65. https://doi.org/10.1186/s12984-018-0408-5
- Lotze, M., Braun, C., Birbaumer, N., Anders, S., & Cohen, L. G. (2003). Motor learning elicited by voluntary drive. Brain A Journal of Neurology, 126(4), 866–872. https://doi.org/10.1093/brain/awg079
- Lüttgen, J., & Heuer, H. (2012). Robotic guidance benefits the learning of dynamic, but not of spatial movement characteristics. Experimental Brain Research, 222(1–2), 1–9. https://doi.org/10.1007/s00221-012-3190-9
- Magill, R., & Anderson, D. (2021). Motor learning and control. McGraw-Hill.
- Manuel, A. L., Guggisberg, A. G., Thézé, R., Turri, F., & Schnider, A. (2018). Resting-state connectivity predicts visuo-motor skill learning. NeuroImage, 176, 446–453. https://doi.org/10.1016/j.neuroimage.2018.05.003
- Manuel, A. L., Nicastro, N., Schnider, A., & Guggisberg, A. G. (2018). Resting-state connectivity after visuo-motor skill learning is inversely associated with offline consolidation in Parkinson’s disease and healthy controls. Cortex, 106, 237–247. https://doi.org/10.1016/j.cortex.2018.06.005
- Marchal-Crespo, L., McHughen, S., Cramer, S. C., & Reinkensmeyer, D. J. (2010). The effect of haptic guidance, aging, and initial skill level on motor learning of a steering task. Experimental Brain Research, 201(2), 209–220. https://doi.org/10.1007/s00221-009-2026-8
- Marchal Crespo, L., & Reinkensmeyer, D. J. (2008). Haptic guidance can enhance motor learning of a steering task. Journal of Motor Behavior, 40(6), 545–556. https://doi.org/10.3200/JMBR.40.6.545-557
- Marchal-Crespo, L., & Reinkensmeyer, D. J. (2009). Review of control strategies for robotic movement training after neurologic injury. Journal of Neuroengineering and Rehabilitation, 6(1), 20. https://doi.org/10.1186/1743-0003-6-20
- Marchal-Crespo, L., Tsangaridis, P., Obwegeser, D., Maggioni, S., & Riener, R. (2019). Haptic error modulation outperforms visual error amplification when learning a modified gait pattern. Frontiers in Neuroscience, 13, 61. https://doi.org/10.3389/fnins.2019.00061
- Marchal-Crespo, L., van Raai, M., Rauter, G., Wolf, P., & Riener, R. (2013). The effect of haptic guidance and visual feedback on learning a complex tennis task. Experimental Brain Research, 231(3), 277–291. https://doi.org/10.1007/s00221-013-3690-2
- Marchal-Crespo, L., Wolf, P., Gerig, N., Rauter, G., Jaeger, L., Vallery, H., & Riener, R. (2015). The role of skill level and motor task characteristics on the effectiveness of robotic training: First results. In 2015 IEEE International Conference on Rehabilitation Robotics (ICORR), (pp. 151–156). https://doi.org/10.1109/ICORR.2015.7281191
- Milot, M. H., Marchal-Crespo, L., Green, C. S., Cramer, S. C., & Reinkensmeyer, D. J. (2010). Comparison of error-amplification and haptic-guidance training techniques for learning of a timing-based motor task by healthy individuals. Experimental Brain Research, 201(2), 119–131. https://doi.org/10.1007/s00221-009-2014-z
- Sada, Y. (1998). Effect of active rest by oral reading on a mirror-drawing task. Perceptual and Motor Skills, 87(2), 635–642. https://doi.org/10.2466/pms.1998.87.2.635
- Sidaway, B., Ahn, S., Boldeau, P., Griffin, S., Noyes, B., & Pelletier, K. (2008). A comparison of manual guidance and knowledge of results in the learning of a weight-bearing skill. Journal of Neurologic Physical Therapy: JNPT, 32(1), 32–38. https://doi.org/10.1097/NPT.0b013e318165948d
- Sigrist, R., Rauter, G., Riener, R., & Wolf, P. (2013a). Augmented visual, auditory, haptic, and multimodal feedback in motor learning: A review. Psychonomic Bulletin & Review, 20(1), 21–53. https://doi.org/10.3758/s13423-012-0333-8
- Sigrist, R., Rauter, G., Riener, R., & Wolf, P. (2013b). Terminal feedback outperforms concurrent visual, auditory, and haptic feedback in learning a complex rowing-type task. Journal of Motor Behavior, 45(6), 455–472. https://doi.org/10.1080/00222895.2013.826169
- Tsutsui, S., & Imanaka, K. (2003). Effect of manual guidance on acquiring a new bimanual coordination pattern. Research Quarterly for Exercise & Sport, 74(1), 104–109. https://doi.org/10.1080/02701367.2003.10609069
- Vander Linden, D. W., Cauraugh, J. H., & Greene, T. A. (1993). The effect of frequency of kinetic feedback on learning an isometric force production task in nondisabled subjects. Physical Therapy, 73(2), 79–87. https://doi.org/10.1093/ptj/73.2.79
- Williams, C. K., & Carnahan, H. (2014). Motor learning perspectives on haptic training for the upper extremities. IEEE Transactions on Haptics, 7(2), 240–250. https://doi.org/10.1109/TOH.2013.2297102
- Winstein, C. J., Pohl, P. S., & Lewthwaite, R. (1994). Effects of physical guidance and knowledge of results on motor learning: Support for the guidance hypothesis. Research Quarterly for Exercise & Sport, 65(4), 316–323. https://doi.org/10.1080/02701367.1994.10607635
- Winstein, C. J., & Schmidt, R. A. (1990). Reduced frequency of knowledge of results enhances motor skill learning. Journal of Experimental Psychology: Learning, Memory, and Cognition, 16(4), 677–691. https://doi.org/10.1037/0278-7393.16.4.677
- Wulf, G., Hoss, M., & Prinz, W. (1998). Instructions for motor learning: Differential effects of internal versus external focus of attention. Journal of Motor Behavior, 30(2), 169–179. https://doi.org/10.1080/00222899809601334
- Wulf, G., McNevin, N., & Shea, C. H. (2001). The automaticity of complex motor skill learning as a function of attentional focus. The Quarterly Journal of Experimental Psychology A, Human Experimental Psychology, 54(4), 1143–1154. https://doi.org/10.1080/713756012
- Wulf, G., Shea, C. H., & Matschiner, S. (1998). Frequent feedback enhances complex motor skill learning. Journal of Motor Behavior, 30(2), 180–192. https://doi.org/10.1080/00222899809601335
- Wulf, G., & Weigelt, C. (1997). Instructions about physical principles in learning a complex motor skill: To tell or not to tell…. Research Quarterly for Exercise & Sport, 68(4), 362–367. https://doi.org/10.1080/02701367.1997.10608018
- Yamaguchi, K., Akizuki, K., Yamamoto, R., Yabuki, J., & Ohashi, Y. (2020). How does physical guidance affect motor learning and learner’s workload? Journal of Physical Therapy Science, 32(10), 621–625. https://doi.org/10.1589/jpts.32.621