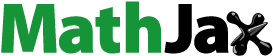
Abstract
Reducing agricultural carbon emissions (ACEs) is essential to China’s overall carbon-emission-reduction target and agricultural sustainable development. This paper analyzes the impact of agricultural technology progress (ATP) and financial support for agriculture on ACEs from a theoretical level, and empirically tests the effect of ATP on ACEs by spatial econometric model, using China’s provincial data from 2001 to 2019. The regulatory effect model is used to examine the moderating effect of financial support policies on ATP and ACEs. The sample was divided according to natural geographical environment, economic development level and agricultural production structure to study the regional heterogeneity from the spatial perspective. The main conclusions are as follows: Overall, ATP has an inhibitory effect on ACEs, and this effect has a spatial spillover effect. In terms of regulation effect, fiscal support for agriculture policy has an enhanced regulatory effect in the impact of ATP on reducing ACEs. In addition, there is obvious regional heterogeneity in the carbon emission reduction effect of ATP in the west or east of the Hu Huanyong line, western or non-western, major or non-major grain-producing regions. This paper draws the following policy implications: First, increase agricultural technology research and development and expand technology spillover channels; second, establish regional collaborative emissions reduction mechanisms and strengthen regional technical cooperation; third, improve the financial support system for agriculture; Fourth, implement regional heterogeneous agricultural policies in accordance with local conditions.
REVIEWING EDITOR:
1. Introduction
Global warming is one of the major threats to human survival, and increasing carbon emissions in the atmosphere are the main cause of global warming (Rosario, Citation2020). Agriculture is closely related to the ecological environment, and data shows that agricultural carbon emissions (ACEs, all abbreviations are shown as Appendix A) are the second important source of global greenhouse gas emission (Eggleston, Citation2006). Agricultural mode, planting structure and farmland management mode based on farmland use change the balance of vegetation cover and carbon cycle on the surface, determine the amount of organic carbon reserve and release in terrestrial ecosystem, and affect the ACEs and its proportion in the total greenhouse gas emissions. China’s ACEs account for 17% of the country’s total, significantly higher than the global average of 11% (Li et al., Citation2011). Most ACEs come from pesticides, fertilizers and other inputs’ energy consumption in the agricultural production, which are necessary for production activities, but they also cause climate warming and threaten ecological security (Campbell et al., Citation2000; Houghton, Citation2002). In the context of high-quality agricultural development, minimizing energy consumption in agricultural production activities, improving soil carbon sequestration capacity and reducing ACEs are effective ways to achieve low-carbon agriculture and mitigate the vulnerability of climate change (Ehsan et al., Citation2022; Elahi et al., Citation2021). In order to fundamentally curb ACEs, it is necessary to change the current crude agricultural development mode with high input and high emissions, and take the road of intensive development, so as to find a way to balance the reduction of ACEs and guarantee food security. Therefore, reducing ACEs is not only an urgent problem of achieving China’s ‘dual carbon’ goal, but also a crucial content to stimulate the sustainable development of agricultural ecology (Muhammad et al., Citation2023).
Many studies have shown that agricultural technological progress (ATP) is a key force in achieving agricultural production increase and carbon emission reduction (Ismael et al., Citation2018; Zhang et al., Citation2020). Such as carbon-free energy technology, fossil energy utilization technology, carbon dioxide capture and storage, agricultural breeding biotechnology, etc., can directly reduce carbon emissions in agricultural production activities. it is of great significance for reducing ACEs and increasing carbon sinks in agriculture to strengthen agricultural technology R&D efforts and promote energy-saving and environmentally friendly technologies. The endogenous growth theory holds that technological progress can improve resource utilization efficiency and save factor costs while promoting sustainable economic growth (Adewale & Sunday, Citation2023). As for ATP, it can optimize the factors allocation by penetrating into land, capital and labor, intervene in farming system, planting mode and energy input structure, thus changing structure of energy consumption and improving efficiency, resulting in soil carbon sink function and the reduction of farmland carbon emissions (Adebayo et al., Citation2023). Moreover, agricultural knowledge, technology, and production experience all have diffusion characteristics, and the scope and degree of its influence on ACEs can be further expand through spillover effects. Obviously, ATP has become the key way of ACEs reduction, and it is significant to further accelerate the R&D, promotion and application of agricultural Low-carbon technology.
Due to the vulnerability characteristics of multiple risks and low benefits in agriculture, the policy of financial support to agriculture has become an important means of national macro-control of agricultural development. In order to ensure food security and promote agricultural economic growth, China’s central and local governments have continuously increased financial support for agriculture. By the end of 2022, China’s fiscal expenditure on agriculture had reached 6.6 trillion yuan, accounting for 25 percent of China’s fiscal expenditure, providing important financial support for promoting agricultural development. The policies of financial support for agriculture that increase capital factors inputs to agriculture, such as subsidies for the purchase of agricultural machinery, good seeds, and agricultural subsidies for conservation tillage, grain cultivation and reforestation, may lead to transformation of agricultural land use patterns and agricultural technology diffusion, which in turn may affect the carbon footprint and emissions.
Therefore, In the context of China’s implementation of the rural revitalization strategy and the dual carbon goal, it is essential to further clarify the internal mechanism of technological progress, agricultural fiscal support policies and green production in the agricultural sector, explore how to make rational use of technology to achieve agricultural sector’s production increase and emission reduction, and how to strengthen national macro-control through the government’s ‘visible hand’, making agricultural policy and technical progress coupled with local resource endowment, so as to achieve high-quality development. This paper aims to study the internal influence mechanism of ATP and financial support policy on ACEs, and explore the spatial effects and heterogeneity characteristics, in order to provide a theoretical and practical basis for the government to promote the application of ATP and formulate financial incentive policies of low-carbon farmland use mode. The possible innovations as follows: This paper extends the scope of research on technological progress and carbon emissions to agriculture, and incorporates the fiscal support agriculture policy as a macro-control factor into the theoretical framework to analyze the regulation mechanism to ATP and ACEs. Furthermore, considering spatial correlation of ATP and ACEs, the spatial spillover effects are decomposed by partial differential spatial Durbin model. In addition, the heterogeneity analysis was carried out in the western or non-western regions, east or west of Hu-Huanyong line, and in the main or non-main grain-producing regions. This paper contributes more new perspectives for clarifying the influence of ATP and financial support policies on agricultural ecology.
2. Literature review
ACEs are influenced not only by natural endowments and regional characteristics, but also by socio-economic conditions. In terms of natural factors, such as animal manure, crop structure, energy type and soil attributes, will affects the agricultural greenhouse gas emissions. There is no doubt that hydroelectricity and natural gas consumption have direct impact on carbon emissions (Tomiwa et al., Citation2023). In terms of socio-economic factors, scholars mainly focus on labor, economic development level, urbanization, finance, technology, and policy to analyze their effects on total and intensity of ACEs. There is evidence that the quantity and structure of rural labor have different effects on ACEs (Zhang et al., Citation2020). According to the environmental Kuznets curve, economic growth and ACEs show an inverted U-shaped relationship, however, Zhang et al. (Zhang et al., Citation2019) found that ACEs and economic growth have a short-run and long-run bidirectional causality. Xu and Bin (Xu & Lin, Citation2017) demonstrated that urbanization are positively correlated with ACEs, and presents spatial spillover effect and heterogeneity characteristics. In general, financial development affects ACEs by adjusting the agricultural factors structure and preventing agricultural risks. With the rise of digital economy, its role in reducing carbon emissions cannot be ignored. Chang (Chang, Citation2022) utilized the threshold effect model for empirical analysis, found that digital economy has an inhibition effect on ACEs, and this effect shows nonlinear characteristics. In addition, green finance provides a convenient channel for financing green agricultural subjects by increasing support for enterprises related to the production, distribution and sale of low-consumption, low-residue and low-pollution agricultural inputs, thereby promoting ACEs reduction (Adebayo & Ullah, Citation2023a). Agricultural policies affect crop output and structure through macro-control, thus affecting ACEs. For example, policies of major grain-producing areas promote ACEs reduction by increasing grain planting the proportion (Yang et al., Citation2021).
Agricultural technological progress (ATP) is usually divided into narrow sense and broad sense. The narrow sense of ATP refers to the technology in agricultural production activities, also known as natural science and technology or hard technology progress; the broad sense includes agricultural management technology and service technology, that is, social science technology or soft technology progress (Dong et al., Citation2015; Gu, Citation1994). In recent years, Total Factor Productivity in agriculture has become increasingly mainstream (Chen et al., Citation2008; Jin et al., Citation2002). There are a number of measures, such as DEA and SFA methods, that can decompose total factor productivity growth into two components: one is technological progress, which refers to technological progress in agriculture in the narrow sense, the other is efficiency gains, which reflect advances in soft technologies such as agricultural management techniques and the rational allocation of resources (Huang et al., Citation2022). Therefore, total factor productivity is essentially a ‘broad’ category of ATP (Alexandros & Mazharul Anwar, Citation2011; Kalirajan et al., Citation1996). Concerning the influence of ATP on carbon emission, the academic circle has analyzed from different angles and obtained numerous views. One view is that advances in agricultural technology can help reduce ACEs. Most scholars believe that technological progress is the fundamental way to realize green agriculture (Fan & Wei, Citation2016). Hayami et al. and Vernon divide classified ATP as biochemical and mechanical technological progress in their study of agricultural theory (Hayami & Herdt, Citation1977; Vernon, Citation1982). Generally speaking, the advance of mechanical technology leads to energy consumption reduction and production efficiency improvement (Guan et al., Citation2023). Some scholars found that the technological progress in material use and resource circularity is significant to reducing ACEs and maintaining environmental sustainability (Alola & Adebayo, Citation2023). The bio-based technological progress, such as breeding technology and green planting technology, is conducive to the carbon sequestration and sink increase of crops, thus reducing the total carbon emissions. For example, Song et al. (Citation2022) analyzed the impacts of ATP on China’s ACEs based on multi-scenario analysis, and proved that the co-progress of production technology and energy technology is the most effective measure to reduce ACEs under the energy intensity constraint. On the contrary, another view is that advances in agricultural technology may increase carbon emissions to some extent. Yang & Li (Citation2017) demonstrated that technological progress will reduce unit production cost, and producers tend to pursue profit maximization and invest more factors to make up for the capital invested in promoting technological progress, resulting in energy rebound effect, which may increase carbon emissions. Tian & Yin (Citation2021) scientifically measured the rebound effect of ACEs in China’s 30 provinces from 2001 to 2018 by constructing rebound effect model of ACEs against the background of ATP. Zhang et al. (Citation2019) also found that ATP has increased the total amount of ACEs, which has the characteristics of strong path dependence and lag (Zhang et al., Citation2019). In addition, the third view is that the influence of technological progress on ACEs shows nonlinear characteristics. There are research results show that the digital technology help promote the agricultural carbon productivity by intermediate transmission mechanism of industrial upgrading and large-scale agricultural operation.
Previous studies have found that the ecological effect of agriculture fiscal support policy is closely related to the way of financial subsidies. Serra et al. (Citation2005) argued that decoupling agricultural subsidy policy was conducive to reducing the use of pesticides, thus reducing carbon emissions and pollution. Taheripour et al. (Citation2008) and Taheripour et al. (Citation2008) holds that the combination of agricultural direct subsidy and environmental tax could reduce nitrogen pollution to a certain extent. Scholars have mainly studied the direct and regulatory effects of agriculture fiscal support on ACEs. In terms of direct impact, Wu (Citation2019) used the fixed effects model and generalized least squares (GLS) to conduct empirical test, and found that agricultural fiscal expenditure has an incremental effect on the ACEs of farmland utilization, but this is affected by technological progress. The aggravating effect of Agricultural financial funds on ACEs is weaker in higher coupling regions; Moreover, due to the different agricultural planting structure, there exists some regional heterogeneity in the influence of agricultural finance and its interaction term with technological progress on ACEs. In terms of regulatory effects, Liu & Xiao (Citation2020) constructed a moderated mediation effect, based on using Chinese provincial dynamic panel data from 2000-2016, and found that the higher levels of agricultural fiscal expenditure strengthen the negative relationship between the proportion of grain cultivation and ACEs, and weaken the positive relationship between fertilizer input intensity and ACEs.
To sum up, the existing research on technological progress and ACEs provides the basis for this paper, but there are few literature combining ATP with financial support for agriculture to test their impact on ACEs, and the theoretical influence mechanisms are still unclear; As for the indicators of ATP, most of them are static indicators that can’t reflect the dynamic characteristics and factor allocation efficiency, such as expenditure on scientific research, patent input, level of agricultural mechanization and agricultural technicians from statistical yearbooks, and there are relatively few studies measuring the index of ATP from a dynamic perspective; In addition, some studies have ignored the influence of geospatial factors and heterogeneous characteristics, resulting in the lack of pertinence and accuracy of research results. Therefore, this paper combines ATP calculated by total factor productivity with fiscal support policies for agriculture to study their impact on ACEs and the spatial effects.
3. Theoretical analysis and research hypothesis
3.1. Impact of agricultural technological progress on carbon emissions
On the one hand, ATP has a direct impact on ACEs. as for mechanical ATP, represented by improving agricultural machinery facilities, enhancing the maintenance and management of machinery, can greatly enhance energy utilization efficiency and decrease ACEs; as for biological ATP, represented by the improvement of agricultural breeding and cultivating technology, can make crops adapt to the complex agricultural production environment, enhance the crop production efficiency, reduce the high-carbon raw materials input, improve the carbon sequestration capacity of soil to achieve the effect of reducing ACEs and increasing carbon sinks (Fei & Lin, Citation2017). On the other hand, ATP has an indirect impact on ACEs. ATP can optimize the allocation of factors by infiltrating into production factors such as land, capital and labour, and by intervening farming systems, cropping structures and farm management practices, thus changing the quantity and structure of energy consumption and promoting new energy utilization, resulting in the increase of soil carbon sink function and the reduction of farmland carbon emissions. However, The ‘return effect’ theory in energy economics points out that technological progress may cause an increase in energy consumption while improving production efficiency (He, Citation2023). The reason is that, when ATP reduces unit production cost, producers tend to investing more factors to improve output, such as input of agricultural machinery equipment, fertilizers, and pesticides, in order to pursing profit maximization and making up for the capital expenditure on technology (Tian & Yin, Citation2021). Overall, most research results confirm that the energy rebound effect of ATP is small, and the effect of ATP on reducing ACEs is greater than energy rebound effect. Therefore, the total effect is assumed here first to be a reduction in carbon emissions, for later verification.
Research hypothesis H1: ATP may have a dampening effect on ACEs.
3.2. The spatial spillover effects of agricultural technological progress on carbon emissions
There may be a spatial correlation between ATP and the behavior of ACEs. The behavior of ACEs is characterized by regional strategic competition and spatial emulation (Jeroen & Neda, Citation2012; Wu et al., Citation2019). As neighboring regions share similar natural conditions for agricultural production and face the same national environmental regulations, fierce competition among neighboring regions may be reflected in mutual imitation, whereby the carbon emission decision-making in one region are influenced by the emission behaviors of neighboring regions through the adoption of more consistent agricultural economic and environmental strategies (Wang et al., Citation2019). The reducing effect of ATP on ACEs may have spatial spillover Characteristics (Wu & Feng, Citation2019). Agricultural technology, knowledge and production experience in one region will radiate to neighboring regions through the diffusion effect, especially for neighboring regions with the advantage of convenient transportation and low cost of mobility of production factors, and technological spillover as a key way to reduce ACEs makes neighboring regions benefit significantly (Chakrabarti, Citation2016; He et al., Citation2022). Therefore, hypothesis H2 is further proposed as follows.
Hypothesis H2: There may be a spatial correlation in the behavior of ACEs, and a spatial spillover effect of ATP in reducing ACEs.
3.3. The moderation mechanism of agricultural fiscal policies
On the one hand, financial support for agriculture influences ACEs through the channel of technology R&D and application and promotion. A large part of financial subsidies to agriculture is special public funds for agricultural production technology R&D, which can attract talents, purchase technology R&D equipment, and reduce private cost in technology R&D. Among them, the three expenses of science and technology can directly stimulate ATP, and improve the total factor productivity of agriculture. Financial funds for agriculture can also directly enhance investment in energy-saving and environmental protection technologies. For example, special funds for low-carbon agricultural technology R&D effectively stimulate agricultural technology innovation, increase the output of patented technology related to agricultural production materials, and encourage the research of energy-saving and environmentally friendly agricultural machinery. Moreover, agricultural fiscal support policies include subsidies for the purchase of technically qualified, safe and environmentally friendly agricultural machinery, which directly and effectively mitigates the use of high-energy-consuming machinery.
On the other hand, financial support for agriculture promotes technological innovation and ACEs reduction by influencing agricultural production scale and structure. The government mainly provides public services such as information, production service, and technological service for grain producers by using financial funds to support agriculture. Financial subsidies for agriculture can effectively increase input quantity of factors such as labor, land and machinery, thus promoting large-scale agricultural operation and professional mechanical operation. In the process of specialized production, capital, labor and information resources can be shared, the allocation efficiency of production factor input can be optimized, and the cost of information search can be reduced, which is benefit to the promotion and diffusion of technology and knowledge (Appiah et al., Citation2018). Such as, the establishment of agricultural price support system, direct food subsidies and producer subsidies promotes agricultural production specialization and intensification that is conducive to the agricultural technical efficiency, which in turn affects ACEs. This paper proposes the research hypothesis H3.
Hypothesis H3: Fiscal support policies for agriculture may have a strengthening regulatory effect in the impact of ATP on reducing ACEs.
3.4. Regional heterogeneity
The impact of ATP and financial support policies on ACEs may show obvious regional differences depending on the natural geographical conditions, economic development and agricultural structure. Firstly, due to different natural geographical conditions such as topography and distance, there are differences in the costs of knowledge, technology and talent mobility. In regions with harsher natural geographical conditions, coupled with restricted transport infrastructure conditions and fewer inter-regional links, it is difficult for the agricultural technology spillover effect to work. Secondly, different regions have Strengths and weaknesses in their resources such as capital, labour and knowledge and information, and differences in the traction and impetus for technology and talent. Hard and soft technologies such as advanced agricultural technologies, expertise and management experience usually flow among developed regions. Even the mechanisms are established in developed regions for sharing agricultural specialized production services and information technology resources. It facilitate the spillover of systematic agricultural technologies, as well as the regional linkages for agricultural carbon reduction. In addition, there are disparities in the factors allocation and the specialization of agricultural production among areas with different agricultural cultivation structures. In regions with high intensive and specialized agricultural production, the resource endowments and technological progress are more closely aligned, the conditions for agricultural technology R&D and application are better. fiscal expenditure for agriculture can play an indispensable policy role in areas with intensive and specialized agricultural production, thus encouraging ‘grainization’ and promoting the ‘technologization’ and ‘decarbonization’ of agriculture. Therefore, due to regional differences, there are also differences in the cost, direction, and dynamics of agricultural technology and research talent mobility, which may lead to regional heterogeneity in the effects of agricultural technology on ACEs.
Hypothesis H4: There may be regional heterogeneity in the spatial carbon abatement effects of technological advances in agriculture.
4. Materials and Methods
4.1. Model construction
Previous studies have shown that carbon emissions have a strong spatial correlation, so the spatial dependence of ACEs needs to be measured first. This paper uses the global Moran’s I index to test the spatial correlation of ACEs in 30 provinces of China. The formula is as follows:
(1)
(1)
Where denotes the 30 provinces of China;
denotes the ACEs of
province;
denotes the mean value of ACEs in each province, w is adjacency weight matrix used for spatial effects analysis. moran’s I index takes values in the range [-1,1], and when the value is greater than 0, it indicates positive autocorrelation; when the value is less than 0, it indicates negative autocorrelation; when the value is 0, it indicates no autocorrelation.
According to Moran’s I index and its statistical tests, China’s ACEs are significantly spatially correlated, thus requiring the introduction of a spatial panel econometric model. Spatial model estimation is carried out using adjacent geographic weight matrix (Xie et al., Citation2019), and it is calculated as follows:
(2)
(2)
In Equationequation (2)(2)
(2) ,
and
are the space section numbers,
. In contrast, the Queen adjacency rule defines all spatial samples that share a common boundary and common vertices with a spatial sample as their neighbouring units. As a result, spatial samples based on the Queen adjacency rule are often more closely related to their surrounding spatial units.
Firstly, this paper conducts Wald test and LR test, and the results suggest that SDM cannot degenerate into SAR and SEM, so SDM should be chosen. Secondly, the Hausman test statistic rejected the random effect model at 1% confidence level, suggesting that the fixed effect model should be selected. Meanwhile, the LR effect test results all rejected the original hypothesis at 1% significance level, meaning that the time-space dual fixed effect model is optimal when choosing the SDM. Therefore, this paper constructs the benchmark model for tests the impact of ATP on ACEs as follows.
(3)
(3)
where subscript
is the province,
is the neighbouring province;
1 is spatial autoregressive coefficients, describing the spatial spillover intensity and direction of ACEs in neighboring provinces;
,
represent regression coefficients of ATP and control variables in local regions; θ2 and ρ2 indicate the influence of ATP and control variables in neighboring regions on local ACEs
and
are individual and time fixed effects respectively, and
is the random disturbance term.
According to the research hypothesis (3), based on the baseline model, a model of the moderating effect of fiscal support policies on the ATP and ACEs is constructed by adding the interaction term of fiscal support policies and ATP. It is showed in Equationequation (4)(4)
(4) .
(4)
(4)
In Equationequation (4)(4)
(4) , fesait is the moderating variable, atechit *fesait denotes the interaction effect of ATP and agricultural fiscal support policy, γ1 and γ2 denote the regression coefficients of direct effect. γ3 and γ4 represent the regression coefficients of indirect effect respectively.
4.2. Variable selection
4.2.1. Explained variable
The explanatory variable is ACEs, and take it logarithm (lnnyco2). According to the recommended methodology (Eggleston, Citation2006), the main sources of carbon in agriculture were defined as agricultural fertilizer, pesticides, diesel, plastic film, crop sown area and agricultural irrigated area. Referring to Li et al. (Citation2011) the corresponding emission factors for each carbon source are 0.8956 kg/kg, 4.9341 kg/kg, 0.5927 kg/kg, 5.18 kg/kg, 312.6 kg/km2 and 20.476 kg/hm2. the carbon emission calculation formula as follows:
(5)
(5)
In the above equation, represents total ACEs,
represents ACEs from the kth carbon source in province
at year
,
is the quantity of the
carbon source, and
is the carbon emission factor.
4.2.2. Core explanatory variable
ATP (atech) is measured by total factor productivity in agriculture, which is calculated by DEA-Malmquist method (Coelli & Rao, Citation2005). Refer to Tian Yun’s research (2021) (Tian & Yin, Citation2021), the output indicator includes the total output value of agriculture, forestry, animal husbandry and fishery, while the input indicator includes the amount of fertilizer applied, sown area, agricultural machinery power and number of people employed in agriculture.
4.2.3. Moderating variable
Fiscal expenditure for supporting agriculture (fesa) is expressed as the ratio of fiscal expenditure for supporting agriculture to total fiscal expenditure. Due to the different statistical method and caliber of data, the items of fiscal support for agriculture were subdivided into forestry expenditure, fisheries expenditure, agricultural expenditure before 2006, and these items are uniformly included from 2006 to the present. Therefore, in order to maintain consistency of the data, the post-2006 algorithm is the share of fiscal agriculture, forestry and water affairs expenditure; the pre-2006 algorithm is the sum of agricultural expenditure, forestry expenditure and fisheries expenditure divided by the total fiscal expenditure.
4.2.4. Control variables
Factors affecting ACEs may also involve urbanization, agricultural land use, industrial structure, and the living standards of residents. Therefore, the control variables are as follows: ①Urbanization (urban): measured by the ratio of the number of urban residents to the total resident population. ②farmland management scale (fms): The formula is the cultivated area divided by the agricultural population (ha/per). ③Cultivation structure (pgc): Expressed as the percentage of grain crops sown area in the total sown area (%), which can reflect the basic situation of crop structure in each region. ④Secondary industry share (secondindu): the percentage of secondary industrial output in GDP. ⑤Tertiary industry share (thirdindu): calculated with the proportion of tertiary industrial output in GDP. ⑥Urban-rural income gap (incomegap): urban households income divided by per capita disposable income of rural households. ⑥Consumption levels of rural residents (consumrur): rural residents’ consumption divided by rural population (yuan/per).
4.3. Data Source
Based on the data availability, the selected samples contain 30 provinces (municipalities) in China from 2001 to 2019. The original data for the measurement of ACEs and ATP are obtained from the China Agricultural Yearbook, the China Rural Statistical Yearbook, and the rest of the data are obtained from the China Financial Yearbook, the China Statistical Yearbook, as well as the provincial statistical yearbooks. shows descriptive results of each variables.
Table 1. Descriptive statistics results.
5. Empirical results and discussion
5.1. Analysis of benchmark regression results
The global Moran’s I index of ACEs in the sample regions was calculated by STATA. As shown in , it can be seen that Morans’ I for ACEs in each regions of China from 2000-2019 is significantly positive, meaning that the ACEs of China’s 30 provinces shows a positive spatial dependence.
Table 2. Results of the spatial correlation test.
reports the baseline results of SDM and SAR. To avoid the problem of possible estimation bias in point estimation tests of spatial spillover effects, the total effect is decomposed into direct and indirect effects in this paper. As it is verified above that the SDM is superior to the SAR, only the results of the SDM in are analyzed and interpreted here. From , the total effect coefficient is -0.572, with a significance level of 1%, indicating that agricultural technology has a suppressive effect on ACEs. This result is similar to the results of a study on energy efficiency and CO2 emissions, which proves that technological progress mitigates greenhouse gas emissions through energy structure optimization (Özkan et al., Citation2023). The direct and indirect influence coefficients of ATP on ACEs are significant positive, which means agricultural technology not only has a suppressive effect on ACEs in the local regions, but also in the surrounding areas. The absolute value of the indirect coefficient is larger than the direct coefficient, suggesting that the spillover effect of agricultural technology advancement on promoting ACEs’ reduction is very obvious, and the application of advanced agricultural technology and expertise has a strong externality. In addition, The coefficient of the spatial lag term of ACEs is significant positive, implying that there are regional strategic competition and spatial emulation characteristics of ACEs behavior, which means that ACEs from neighbring regions can form a positive spatial spillover effect on local ACEs, and every 1% increase in ACEs from neighbring regions will cause 0.5% increase in ACEs in the region. Thus, it is difficult to manage the regional ecological environment alone, and a joint strategy of regional prevention and control is needed.
Table 3. Baseline regression results.
Among the other influencing factors estimated by the spatial Durbin model, each control variable has significant effect on ACEs. The proportion of grain crops plays spatial dampening role on ACEs, because grain crops consume less carbon sources during cultivation compared to cash crops; Increasing levels of urbanization has a negative impact on ACEs; the scale of farmland operations in land use patterns intensify carbon emissions, probably because the expansion of farmland scale is accompanied by more land concentration and more frequent land plowing activities, leading to a decrease in the carbon sequestration effect of farmland; the adjustment of industrial structure also cause to an increase in local ACEs and a decrease in surrounding area’s ACEs; the income and consumption of urban and rural residents also have effect on ACEs.
5.2. Robustness tests
This paper uses three ways to test the robustness: ①data are subjected to tail shrinkage:To avoid the interference of outliers on results, this paper performs a robustness check by means of a 1% shrinkage tail on the explanatory variable. ②Reduce the sample size: The stability of the sample was tested by deleting the first and last years of the sample. ③Replace the spatial weight matrix: transform adjacency matrix into inverse distance squared matrix. shows the robustness test results. The regression coefficients are all significantly negative, which proves the previous benchmark analysis is strongly robust.
Table 4. Robustness test results.
5.3. Regional heterogeneity analysis
Considering the disparities in natural geography, economic conditions and agricultural structure of each regions, which may result in heterogeneity in ACEs’ reduction effects of ATP. The sample was divided into regions west and east of the Hu Huanyong Line (HHYL) according to natural geography, main and non-main grain-producing regions based on differences in agricultural structure, and into western and non-western regions depending on economic development level. The empirical results are presented in .
Table 5. Regional heterogeneity.
The coefficients of both the direct and indirect effects of ATP on ACEs in areas east of the HHYL are significant negative. However, in areas west of the HHYL, the direct coefficient is significant negative and indirect coefficient is not significant. It suggests that the spillover effect of ATP on reducing ACEs is not obvious in areas west of the HHYL. The possible explanation for this phenomenon is that, due to the areas west of the HHYL are constrained by complex topographical, geographical distance and poor transport infrastructure, the cost of agricultural knowledge and technology circulation is relatively high. In contrast, areas east of the HHYL are densely populated and well connected, and frequent inter-regional connections promote spatial spillovers of agricultural technologies, which in turn contribute to ACEs’ reductions in neighboring areas. This view has also been proved by related studies (Yang et al., Citation2021).
ATP has a inhibition effect on ACEs in both western and non-western regions, but the agricultural technology spillover effect in western regions is not obvious. Due to the western regions are economically underdeveloped, which is less attractive to agricultural specialized knowledge and technical talents, while the non-western region has better condition for agricultural technology R&D and distribution, thus producing significant spillover effect of high-quality technology resources and forming demonstration effect of ACEs reduction among regions. Similar conclusions have also been reached in related studies: China’s non-western provinces are regions with a high level of urbanization, and they have good conditions for technological R&D and expansion, high energy efficiency and renewable energy utilization technology, so the reducing carbon emission effect of technological progress is more obvious among regions with high urbanization levels (Adebayo & Ullah, Citation2023b).
The direct and indirect coefficients of ATP on ACEs are both significant negative in the main grain-producing regions, not significant in the grain-producing areas. It shows that agricultural technological advances can significantly contribute to the reduction of ACEs in the main grain-producing regions. Referring to the research of Zhang et al. (Citation2019) and Zhang et al. (Citation2019), following explanations can be obtained: the major grain-producing areas are highly intensive and specialized, which optimizes the allocation efficiency of production factor and promotes the technology R&D, promotion and application. However, the grain-producing areas has a higher degree of fragmentation, a lower degree of specialization and management efficiency, so the ACEs reduction effect of ATP needs to be further developed. This view has also been proved by related studies.
5.4. The regulatory mechanism test
reports the results of moderating mechanism of fiscal support policies on ATP and ACEs. As shown in the , after adding the fiscal support policy for agriculture(fesa) and its interaction term with technological progress(atechfesa) to the baseline model, the direct coefficient of the interaction term is -0.151, which is significantly negative, and the coefficient of technological progress(atech) is still significant negative, implying the agricultural fiscal support policy can strengthen the ACEs reduction effect of ATP. This result is similar to Liu and Xiao’s the findings (Liu & Xiao, Citation2020). The reason is that the special funds for scientific and technological R&D and subsidies for the purchase of environmentally friendly agricultural machinery in fiscal expenditure for support agriculture decrease high energy-consuming machinery utilization, and fiscal support policies such as subsidies for grain farmers and minimum purchase prices for grain promote agricultural specialization, which is conducive to ACEs reduction, verifying research hypothesis H3.
Table 6. The regulatory effects test results.
6. Conclusions
In order to explore the impact of ATP and agricultural fiscal policies on ACEs, this paper analyzes the impact of ATP on ACEs by spatial model, and examines the regulating mechanism of fiscal support policies on ATP and ACEs by moderating effects model, using China’s provincial data during 2001-2019. The sample was divided according to natural geographical environment, economic development level and agricultural production structure to study the heterogeneity from spatial perspective.
These are main conclusions: On the whole, ATP has an inhibitory effect on ACEs. ACEs show positive spatial correlation, and the impact of ATP on reducing ACEs also have a spatial spillover characteristic, that is, ATP not only has suppressive effect on local carbon emissions, but also on surrounding areas’ carbon emissions. From the perspective of regional heterogeneity, the impact of ATP on ACEs has a spatial spillover in the east of the HHYL, but no spillover effect in the west of the HHYL, reflecting that the demonstration and learning effects of technological advances in agriculture have not yet span the HHYL; Compared with western China, the ACEs reduction effect of ATP is more significant in non-western China; Compared with non-main grain-producing regions, the ACEs reduction effect of ATP is obvious in main grain-producing regions. In terms of regulatory effect of agricultural fiscal policy, it has an enhanced regulatory role in the impact of ATP on reducing ACEs, that is, the higher level of financial support to agriculture can promote the inhibitory effect of ATP on ACEs.
Based on the above findings, some policy implications are as follows: First, increase technology R&D and expand technology spillover channels in agricultural. On the one hand, it is necessary to reduce energy use and ACEs by ATP, create a benign environment for technology research and development, continuously improve the intellectual protection system, encourage enterprises and research subjects to conduct R&D on low-carbon-based machinery and equipment, irrigation technology and bio-based agricultural technology, and strengthen supervision and management of investment in research funds; on the other hand, the government should build a good environment for the spillover of technologies, promote energy-saving technologies, and guide the flow of technical talents to poor areas through fiscal and tax preferential policies, so as to release the central area’s diffusion effect and the surrounding regions’ the learning effect. Second, establish a synergistic emission reduction mechanism and strengthen regional technical cooperation. It is possible to establish inter-regional joint prevention and control policies and ecological compensation systems for ACEs, and strengthen regional joint environmental law enforcement and supervision by building a unified inter-regional monitoring platform for ACEs and implementing regional environmental information sharing and joint early warning. At the same time, agricultural economic ties and technical cooperation among regions should be strengthened, and the strategies of regional coordinated development and industrial integration should be made full use of to promote ‘strong alliances’ and ‘complementary strengths’. In addition, agricultural technology transfer platforms should be set up between and within regions to solve problems such as information blockage, capital shortage and technology application risks. Third, improve the fiscal support system for agriculture, strengthen the financial support in guiding technological progress, and reinforce ecological orientation. It is necessary to appropriately increase fiscal subsidies for low-carbon agricultural production technology research and development, government services and agricultural talent training and other ‘green box policies’ to support agriculture, adjust the ‘yellow box policies’ that lead to a sharp rise in carbon emissions in short term due to excessive use of factors, and enhance the efficiency of the transformation of financial subsidies for agriculture into total factor productivity in agriculture. Subsidies should be given to farmers and agricultural enterprises for the purchase of energy-saving agricultural machinery, and farmers should be encourage to use low-carbon fertilizers, films and other agricultural production materials to reduce carbon emissions. Fourth, implement regional differentiated ACEs reduction policies. Considering the heterogeneous characteristics of resource endowments, encourage ATP and carbon emission reduction strategies coupled with local resource endowments, and implement strict environmental regulation policies to mitigate ACEs. The government should appropriately tilt towards the less developed western regions when distributing agriculture-related fiscal funds, and increase subsidies for agricultural technology innovators and farmers who low-carbon technologies to realize the ‘win-win’ of income increase of farmers and carbon emission reduction in the western areas. In addition, the government should appropriately increase agricultural financial input in non-main grain-producing areas in accordance with resource endowments the characteristics, considering the input structure of different crop factors, encourage technological progress of different crops through refined policies, and restrain the excessive increase of carbon emissions from farmland use.
This paper acknowledges some limitations and future research endeavors. Firstly, due to the difficulty of obtaining data at the prefecture-level, this paper uses data at the provincial level. Future researchers could consider obtaining some micro data at the prefecture-level, selecting typical areas for field research to collect first-hand data. Secondly, this paper does not find the appropriate instrumental variable for endogeneity test. In the future research, it is essential to accurately explore the instrumental variable of agricultural technology and improve the method of endogeneity test. Thirdly, the theoretical mechanism of this paper still needs to be deepened. As a direction for future research, specific mechanism analysis can be carried out from a more micro perspective, such as farmers’ behavior and agricultural enterprises, so as to put forward targeted policy implications.
Disclosure statement
The authors declare that we have no known competing financial interests or personal relationships that could have appeared to influence the work reported in this paper.
Additional information
Funding
References
- Adebayo, T. S., & Ullah, S. (2023a). Formulating sustainable development policies for China within the framework of socioeconomic conditions and government stability. Environmental Pollution (Barking, Essex: 1987), 328, 1. https://doi.org/10.1016/j.envpol.2023.121673
- Adebayo, T. S., & Ullah, S. (2023b). Towards a sustainable future: The role of energy efficiency, renewable energy, and urbanization in limiting CO2 emissions in Sweden. Sustainable Development, 1–16. https://doi.org/10.1002/sd.2658
- Adebayo, T. S., Kartal, M. T., Ağa, M., & Al-Faryan, M A. b. d S. (2023). Role of country risks and renewable energy consumption on environmental quality: Evidence from MINT countries. Journal of Environmental Management, 327, 116884. https://doi.org/10.1016/j.jenvman.2022.116884
- Adewale, A. A., & Sunday, A. T. (2023). The potency of resource efficiency and environmental technologies in carbon neutrality target for Finland. Journal of Cleaner Production, 389, 136127. https://doi.org/10.1016/J.JCLEPRO.2023.136127
- Alexandros, M. T., & Mazharul Anwar, M. (2011). A comparison of DEA and SFA methods: a case study of farm households in Bangladesh. The Journal of Developing Areas, 45(1), 95–14. https://doi.org/10.1353/jda.2011.0004
- Alola, A. A., & Adebayo, T. S. (2023). Analysing the waste management, industrial and agriculture greenhouse gas emissions of biomass, fossil fuel, and metallic ores utilization in Iceland. The Science of the Total Environment, 887, 164115. https://doi.org/10.1016/j.scitotenv.2023.164115
- Appiah, D. O., Akondoh, A. C. K., Tabiri, R. K., & Donkor, A. A. (2018). Smallholder farmers’ insight on climate change in rural Ghana. Cogent Food & Agriculture, 4(1), 1436211. https://doi.org/10.1080/23311932.2018.1436211
- Campbell, C. A., Zentner, R. P., Liang, B.-C., Roloff, G., Gregorich, E. C., & Blomert, B. (2000). Organic C accumulation in soil over 30 years in semiarid southwestern Saskatchewan – Effect of crop rotations and fertilizers. Canadian Journal of Soil Science, 80(1), 179–192. https://doi.org/10.4141/S99-028
- Chakrabarti, A. S. (2016). Stochastic Lotka–Volterra equations: A model of lagged diffusion of technology in an interconnected world. North-Holland, 442, 214–223. https://doi.org/10.1016/J.PHYSA.2015.09.030
- Chang, J. X. (2022). The role of digital finance in reducing agricultural carbon emissions: evidence from China’s provincial panel data. Environmental Science and Pollution Research International, 29(58), 87730–87745. https://doi.org/10.1007/s11356-022-21780-z
- Chen, P. C., Ming-Miin, Y. U., Chang, C. C., & Hsu, S. H. (2008). Total factor productivity growth in china’s agricultural sector. China Economic Review, 19(4), 580–593. https://doi.org/10.1016/j.chieco.2008.07.001
- Coelli, T. J., & Rao, D. P. (2005). Total factor productivity growth in agriculture: A Malmquist index analysis of 93 countries, 1980–2000. Agricultural Economics, 32(s1), 115–134. https://doi.org/10.1111/j.0169-5150.2004.00018.x
- Dong, W., Chou, J. M., Wang, S., & Fu, Y. (2015). Quantitative analysis of agricultural land use change in China. Physics and Chemistry of the Earth, 87-88, 3–9. https://doi.org/10.1016/j.pce.2015.08.011
- Eggleston, S. (2006). IPCC guidelines for national greenhouse gas inventories. Energy (Oxf), (2).
- Ehsan, E., Zainab, K., & Zhang, Z. X. (2022). Understanding farmers’ intention and willingness to install renewable energy technology: A solution to reduce the environmental emissions of agriculture. Applied Energy. 309, 118459. https://doi.org/10.1016/J.APENERGY.2021.118459
- Elahi, E., Khalid, Z., Tauni, M. Z., Zhang, H., & Lirong, X. (2021). Extreme weather events risk to crop-production and the adaptation of innovative management strategies to mitigate the risk: A retrospective survey of rural Punjab, Pakistan. Technovation, 117(4), 102255. https://doi.org/10.1016/j.technovation
- Fan, C. Z., & Wei, T. Y. (2016). Effectiveness of integrated low-carbon technologies Evidence from a pilot agricultural experiment in Shanghai. International Journal of Climate Change Strategies and Management, 8(5), 758–776. https://doi.org/10.1108/IJCCSM-04-2015-0045
- Fei, R. L., & Lin, B. Q. (2017). Technology gap and CO2 emission reduction potential by technical efficiency measures: A meta-frontier modeling for the Chinese agricultural sector. Ecological Indicators. 73, 653–661. https://doi.org/10.1016/j.ecolind.2016.10.021
- Gu, H. Y. (1994). Discussion on the connotation and function of agricultural technology progress. Agricultural Technology Economy, (4), 24–27.
- Guan, N. N., Liu, L. Y., Dong, K., Xie, M., & Du, Y. J. (2023). Agricultural mechanization, large-scale operation and agricultural carbon emissions. Cogent Food & Agriculture, 9(1), 2238430. https://doi.org/10.1080/23311932.2023.2238430
- Hayami, Y., & Herdt, R. W. (1977). Market price effects of technological change on income distribution in semisubsistence agriculture. American Journal of Agricultural Economics, 59(2), 245–256. https://doi.org/10.2307/1240014
- He, Y. (2023). Food security and agriculture value-added: Do they asymmetrically matter for Korean environmental sustainability? Cogent Food Agric, 9, 2213525. https://doi.org/10.1080/23311932
- He, Y. Q., Cheng, X. Y., & Wang, F. (2022). Regional spillover effects of agricultural carbon emissions from the perspective of technology diffusion. Agro-Technical Economy, (04), 132–144. https://doi.org/10.13246/j.cnki.jae.20211208.003
- Houghton, R. A. (2002). Magnitude, distribution and causes of terrestrial carbon sinks and some implications for policy. Climate Policy, 2(1), 71–88. https://doi.org/10.1016/S1469-3062(02)00012-8
- Huang, X., Feng, C., Qin, J., Wang, X., & Zhang, T. (2022). Measuring China’s agricultural green total factor productivity and its drivers during 1998–2019. The Science of the Total Environment, 829, 154477. https://doi.org/10.1016/j.scitotenv.2022.154477
- Ismael, M., Srouji, F., & Boutabba, M. A. (2018). Agricultural technologies and carbon emissions: evidence from Jordanian economy. Environmental Science and Pollution Research International, 25(11), 10867–10877. https://doi.org/10.1007/s11356-018-1327-5
- Jeroen, W., & Neda, Z. (2012). Hegemony and asymmetry: multiple-chessboard games on transboundary rivers. International Environmental Agreement, 12(3), 215–229. https://doi.org/10.1007/s10784-012-9177-y
- Jin, S. Q., Huang, J. K., Hu, R. F., & Rozelle, S. (2002). The creation and spread of technology and total factor productivity in china’s agriculture. American Journal of Agricultural Economics, 84(4), 916–930. https://doi.org/10.1111/1467-8276.00043
- Kalirajan, K. P., Obwona, M. B., & Zhao, S. (1996). A decomposition of total factor productivity growth: The case of Chinese agricultural growth before and after reforms. American Journal of Agricultural Economics, 78(2), 331–338. https://doi.org/10.2307/1243706
- Li, B., Zhang, J. B., & Li, H. P. (2011). Temporal and spatial characteristics and influencing factors of agricultural carbon emissions in China. China’s Population, Resources and Environment, 21(08), 80–86. https://doi.org/10.3969/j.issn.1002-2104.2011.08.013
- Liu, Q., & Xiao, H. F. (2020). The impact of agricultural land management scale and fiscal support policy on agricultural carbon emissions. Resource Science, 42(6), 1063–1073. https://doi.org/10.18402/resci.2020.06.05
- Muhammad, I., Sami, U., Asif, R., Cai, J. Y., & Tomiwa, S. A. (2023). Unleashing the dynamic impact of tourism industry on energy consumption, economic output, and environmental quality in China: A way forward towards environmental sustainability. Journal of Cleaner Production, 387, 135778. https://doi.org/10.1016/j.jclepro.2022.135778
- Özkan, O., Alola, A. A., & Adebayo, T. S. (2023). Environmental benefits of nonrenewable energy efficiency and renewable energy intensity in the USA and EU: Examining the role of clean technologies. Sustainable Energy Technologies and Assessments, 58, 103315. https://doi.org/10.1016/J.SETA.2023.103315
- Rosario, D. (2020). Tilting at windmills: Global warming and global warnings. Milbank Q, 98(1), 22–25. https://doi.org/10.1111/1468-0009.12447
- Serra, T., Zilberman, D., Goodwin, B. K., & Hyvonen, K. (2005). Replacement of agricultural price supports by area payments in the European Union and the effects on pesticide use. American Journal of Agricultural Economics, 87(4), 870–884. https://doi.org/10.1111/j.1467-8276.2005.00775.x
- Song, S. X., Zhang, L., & Ma, Y. X. (2022). Evaluating the impacts of technological progress on agricultural energy consumption and carbon emissions based on multi-scenario analysis. Environmental Science and Pollution Research International, 30(6), 16673–16686. https://doi.org/10.1007/s11356-022-23376-z
- Taheripour, F., Khanna, M., & Nelson, C. H. (2008). Welfare impacts of alternative public policies for agricultural pollution control in an open economy: A general equilibrium framework. American Journal of Agricultural Economics, 90(3), 701–718. https://doi.org/10.1111/j.1467-8276.2008.01139.x
- Tian, Y., & Yin, M. H. (2021). Do technological advances promote carbon reduction in agricultural energy? – Based on the test of rebound effect and spatial spillover effect. reform, 2021(12), 45–58. https://xueshu.baidu.com/usercenter/paper/show?paperid=1f6800g0gg790p40wk4a0ea0ha439523&site=xueshu_se&hitarticle=1
- Tomiwa, S. A., Mustafa, T. K., & Sami, U. (2023). Role of hydroelectricity and natural gas consumption on environmental sustainability in the United States: Evidence from novel time-frequency approaches. Journal of Environmental Management. 328, 116987. https://doi.org/10.1016/j.jenvman.2022.116987
- Vernon, R. (1982). Agricultural research policy. Minneapolis: University of Minnesota Press.
- Wang, Y., Zhang, Y. Q., Tian, Y., & Wang, R. (2019). The influencing factors and spatial spillover of agricultural carbon emissions in Chinas major grain producing regions. Journal of Southern Agriculture, 50(7), 1632–1639.
- Wu, H., Huang, H., Tang, J., Chen, W., & He, Y. (2019). Net greenhouse gas emissions from agriculture in China: Estimation, spatial correlation and convergence. Sustainability, 11(18), 4817. https://doi.org/10.3390/su11184817
- Wu, W. W. (2019). A study on the carbon emission effects of farmland utilization with a focus on agricultural finance and technological progress. China Land Science, 33(03), 77–84.
- Wu, Y. G., & Feng, K. W. (2019). Spatial-temporal differentiation features and correlation effects of provincial agricultural carbon emissions in China. Environmental Science and Technology, 42(3), 180–190. https://doi.org/10.19672/j.cnki.1003-6504.2019.03.026
- Xie, W. W., Deng, H. B., & Wang, N. (2019). Spatial spillover effect of geographical proximity and technological proximity on regional innovation. East China Economic Management, 33(07), 61–67. https://doi.org/10.19629/j.cnki.34-1014/f.180907023
- Xu, B., & Lin, B. Q. (2017). Factors affecting CO2 emissions in China’s agriculture sector: Evidence from geographically weighted regression model. Energy Policy, 104, 404–414. https://doi.org/10.1016/j.enpol.2017.02.011
- Yang, C., Hu, P. Q., Diao, B. D., Cheng, J. H., & Cui, H. Y. (2021). Environmental performance of policies in major grain-producing areas: from the perspective of agricultural carbon emissions. China’s Population, Resources and Environment, 31(12), 35–44.
- Yang, L., & Li, Z. (2017). Technology advance and the carbon dioxide emission in China – Empirical research based on the rebound effect. Energy Policy, 101(FEB), 150–161. https://doi.org/10.1016/j.enpol.2016.11.020
- Zhang, H., Guo, S. D., Qian, Y. B., Liu, Y., & Lu, C. P. (2020). Dynamic analysis of agricultural carbon emissions efficiency in Chinese provinces along the Belt and Road. PloS One, 15(2), e0228223. https://doi.org/10.1371/journal.pone.0228223
- Zhang, L., Pang, J. X., Chen, X. P., & Lu, Z. M. N. (2019). Carbon emissions, energy consumption and economic growth: Evidence from the agricultural sector of China’s main grain-producing areas. The Science of the Total Environment, 665, 1017–1025. https://doi.org/10.1016/j.scitotenv.2019.02.162
- Zhang, Y. Q., Tian, Y., Wang, Y., Wang, R., & Peng, Y. X. (2019). Rural human capital, agricultural technology progress and agricultural carbon release. Science and Technology Management Research, 39(14), 266–274. https://doi.org/10.3969/j.issn.1000-7695.2019.14.035