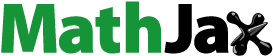
Abstract
Maize is the cheapest grain in Ethiopia, which is widely consumed by rural communities and low-income urban residents. As a result, the Ethiopian government has implemented various policy initiatives to increase its productivity. However, these policy initiatives have not contributed enough to improve maize productivity. This study utilized stochastic frontier analysis to investigate and analyze factors contributing to differences in productivity and efficiency among smallholder maize farmers in Ethiopia. The findings showed that the supply of inputs and technology significantly affected maize productivity. We found that women-managed households were more efficient than their male-managed counterparts. We also offered new insights into the non-linear effect of farmers’ education on their efficiency levels. Furthermore, we documented that households would benefit from a 14.40% productivity gain and 13.90% production cost savings if operating at full efficiency level. Land fragmentation and distance from the main market are key factors that have adversely affected the efficiency of smallholder maize farmers. Hence, policies that reduce land fragmentation and enhance rural market infrastructure development will enable Maize farmers to increase their efficiency and productivity.
PUBLIC INTEREST STATEMENT
Maize is the most productive and cheapest grain in Ethiopia. Despite its potential, there is still a significant gap between the actual and potential yield. It calls attention to the low productivity of smallholder maize farmers, who account for more than 90% of maize production in Ethiopia. In particular, this study sought to examine and identify factors contributing to productivity and efficiency differences among smallholder maize farmers. Besides the supply and access to essential inputs and technology, we documented various socioeconomic, institutional, and demographic factors affecting the productivity and efficiency of farmers. The results also show that if households operated at full economic efficiency, they would benefit from a 14.40% productivity gain and 13.90% production cost savings. It revealed that there are still opportunities to increase maize productivity in the study area. Hence, the results can be helpful for policymakers and development interventionists looking to maintain food security by improving agricultural productivity.
Reviewing editor:
1. Introduction
Cereal production and marketing are the most important subsector of the Ethiopian economy (Anteneh & Asrat, Citation2020; Rashid & Negassa, Citation2013). Teff, Maize, and Wheat are the main Ethiopian cereals comprising three-fourths of cultivated land, 29% of the agricultural GDP in 2005/06 (14% of the total GDP), 64% of the calories consumed, and 85% of all crop production (Tadesse et al., Citation2018). Therefore, it is no surprise that since the 1960s, all agricultural production policies have focused on the grain sub-sector. However, these policy initiatives do not contribute enough to improve the performance of agriculture, and the nation cannot feed its population. Ethiopia has prioritized reducing poverty by promoting agricultural productivity in selected strategic food crops such as Maize (Zea mays L., also commonly known as corn) since 2002 (Challa & Ofolsha, Citation2016; Solomon & Teshale, Citation2017). Among all cereal crops in the country, Maize is the first in productivity and the second next to Teff in cultivated area coverage. It is one of the cheapest grains for rural and low-income households living in urban areas and is versatile in terms of use (T. Abate et al., Citation2015). Maize is a major staple cereal crop supporting the livelihoods of millions of smallholders in Ethiopia. It contributes about 29% of the calorie intake from total cereal consumption, followed by wheat and Teff, contributing about 21% and 17%, respectively (Tadesse et al., Citation2018). Hence, improving the productivity and efficiency of Maize production is critical to improving food security in the country.
Despite such a significant role and potential, its productivity still needs to improve. The average yield of Maize in Ethiopia was 2.56 tonnes/hectare between 2012 and 2016, which is 44.2%, far below the global standard and mainly produced by smallholder farmersFootnote1 (Mahuku et al., Citation2015). Smallholder farmers are producers of the most staple crops, such as Maize, representing about 95% of the total agricultural output and cultivating more than 90% of the total land in Ethiopia (Zerssa et al., Citation2021). More than 9 million smallholder farmers grow Maize, and they are the main food producers in Ethiopia. Around 88% of their production is consumed for food (Van Dijk et al., Citation2020). Though smallholder farmers are responsible for a large proportion of Ethiopia’s crop production and food security, they still struggle to increase their productivity (Rapsomanikis, Citation2015). Hence, improving the productivity of smallholder farmers stands among the most exciting opportunities to address bottlenecks in the food system. Recent studies, of course, documented an increasing trend in maize production. However, growth in maize output over these years was driven by an increase in the area under cultivation (T. Abate et al., Citation2015; M. Alemu et al., Citation2022). However, given limited agricultural resources, increasing agricultural production by using more resources is an unsustainable way of farming. Efficient use of existing resources and technology is the most sustainable way to enhance agricultural production, especially for farmers in developing countries like Ethiopia, who are poor and need more capital to adopt new technologies. That is why the efficient use of available resources has received the utmost attention (Bravo-Ureta, Citation1994; Tekalign, Citation2019).
While the existing literature has extensively explored the determinants of agricultural productivity, the issue of farmers’ efficiency still needs to be addressed in the empirical literature. Abate et al. (Citation2015), Alemu et al. (Citation2008), and Sileshi et al. (Citation2022) argued that several socioeconomic and natural factors such as weeds, pests, and diseases, rainfall variations, soil erosion, low soil fertility, poor and weak infrastructure, and post-harvest crop losses are the most significant factors contributing to productivity differences among the farmers. Notably, they documented that low productivity of Maize is associated with a need for more necessary inputs such as fertilizer, improved seed, pesticides, and others. However, it is common to see different productivity levels among farmers even when all the essential inputs are met. Hence, accessing the required inputs is not a panacea for maize productivity improvement. Though farmers’ efficiency is vital in determining productivity, few studies examined the efficiency aspect of maize smallholder farmers in Ethiopia. Belete (Citation2020) and Tesema (Citation2021), for example, reported that the low productivity of Maize in Ethiopia is due to inefficiencies among farmers. Indeed, there is little systematic research on these issues. However, there has yet to be a consensus on factors contributing to efficiency and productivity differences among maize smallholder farmers, even among these few researchers. This paper aims to empirically examine productivity and efficiency differences among maize smallholder farmers in Ethiopia. Specifically, it addresses the following research questions: (1) what is the productivity and efficiency status of households in the study area? (2) what are the factors contributing to productivity and efficiency differences? (3) to what extent do these factors contribute to households’ productivity and efficiency differences?
This study makes a significant contribution to the literature on the efficiency and productivity of smallholder farmers in various ways. Unlike previous efficiency studies that primarily focused on farmers’ technical efficiency, this study looked at all efficiency aspects, such as technical, allocative, and economic efficiencies. Furthermore, conventional efficiency analysis studies typically use stochastic frontier analysis (Ahmed et al., Citation2018; Deribe et al., Citation2022; Tesema, Citation2021, Citation2022). In addition to stochastic frontier analysis techniques, this study used quantile regression techniques to investigate the non-linear effect of cofactors on farmers’ efficiency levels.
2. Materials and methods
2.1. Description of the study area
The study was conducted in Mecha district, one of the thirteen districts found in the West Gojam Administrative Zone, located 30 km southwest of Bahir Dar town, the capital of the Amhara Region, Ethiopia. The climatic conditions of the study area alternate between summer rain and the winter dry season with a mean annual rainfall of 1500–2200mm. The mean temperature is between 24 °C and 27 °C, and the altitude ranges from 1800 to 2500 m above sea level (Salew, Citation2018). The livelihood of the district is based on mixed farming. It comprises crop production and livestock rearing. The main crops grown in the area are sorghum, Maize, Teff, barley, common beans, highland pulses, and many other vegetable crops such as potatoes, onion, garlic, and leafy vegetables (Tassew & Seifu, Citation2009).
2.2. Data and sampling
The study used primary cross-sectional data collected from farmers in the Mecha district for the 2018/19 cropping season. A multistage sampling technique was used with a combination of different sampling techniques. Mecha district was selected as a priority because of its importance in maize production. In the second stage of sample selection, a total of 40 Kebles in the district were grouped into two different strata based on their irrigation access, i.e., strata 1 (kebelesFootnote2 within Koga medium-size dam irrigation scheme) and strata 2 (kebeles outside the irrigation scheme). Here, we assumed that each stratum is relatively homogenous. Two kebeles were selected from each stratum in the third sampling stage using a simple random sampling technique. Finally, 198 sample households were determined using Yamane’s (1967) formula.
(1)
(1)
i.e.
Where: n = the sample size, N = the total number of households in each Kebele, e = level of significance (precision)
The number of households interviewed in each Kebele was allocated proportionally ().
Table 1. Sample size per kebele.
2.3. Data analysis and estimation techniques
We used a Stochastic Frontier Approach (SFA) method to estimate and analyze the factors determining maize productivity in the study area. To overcome the sample size problem, we used bootstrap techniques with 9,994 replications. The two-stage approach was used to identify and analyze factors affecting farmers’ efficiency levels. In the first stage, Technical Efficiency (TE) and Economic Efficiency (EE) scores were estimated using the maximum likelihood estimation (MLE) technique. And we computed Allocative Efficiency (AE) scores using the estimated TE and EE scores. In the second stage, the parameters of the efficiency model were estimated and analyzed using the Tobit regression model.
2.4. Model specification
This study adopted the stochastic frontier analysis, initially developed by Aigner et al. (Citation1977) and Meeusen and van Den Broeck (Citation1977). The general functional form of the stochastic frontier model for this study is specified as follows:
(2)
(2)
It is convenient to write the model in the following form:
(3)
(3)
where
ln = denotes the natural logarithm, Yi = is a scalar of observed output (maize output per hectare measured in kg); Xi = is a j×l vector of input variables; Bj=is a j×l vector of the corresponding coefficient vector (they are elasticity coefficients), εi = is composed disturbance term made up of two elements (Vi and Ui) i.e., ; Vi = is a zero-mean random error that accounts for the stochastic effects beyond the farmers’ control, measurement error, and other statistical noises. Ui = is the one-sided disturbance term used to represent production inefficiency and is assumed independent of vi. It is necessary to estimate the respective TE scores to analyze factors affecting the TE of maize farmers. As a result, farm-specific technical efficiencies (i.e., the ratio of the observed output to the output level produced by a fully efficient farm) can be estimated as:
(4)
(4)
where TE takes the value between 0 and 1
Also, from the duality nature of production and cost functions, the self-dual (for EquationEquation 3(3)
(3) ) cost function can be specified as (Thabethe et al., Citation2014).
(5)
(5)
where
Ci is the total cost used in the cultivation of Maize; Wi is different input prices; β is the elasticity coefficients to be estimated, and others are described above. However, the two error terms are preceded by positive signs because inefficiencies are assumed to increase the cost. Similarly, the farm-specific EE scores, defined as the ratio of minimum total production cost (C*) to actual observed total production cost (C), are also estimated as:
(6)
(6)
where
EE takes the value between 0 and 1.
Using the Farrell (Citation1957) formula, farm-specific allocation efficiency (AE), obtained from technical and economic efficiencies estimated as follows:
(7)
(7)
We employed a Cobb-Douglas functional form in this study because it meets the requirement of being self-dual, which allows an examination of economic efficiency. Many empirical studies have used the Cobb-Douglas production function, particularly those relating to developing country agriculture (Bravo-Ureta et al., Citation2007). The Cobb-Douglas functional form for the maize farm in the study area is specified as follows for the production functions:
(8)
(8)
Furthermore, the Cobb-Douglas cost frontier function for maize farms in the study area is specified as follows.
(9)
(9)
Where C; is the total cost of maize production cost; W1 is the average price of DAP per kg; W2 is the rental value of land per hectare; W3 is the average price of Urea per kg; W4 is the average price of seed per kg; W5 is the average wage rate per day; is the estimated value of maize output using EquationEquation (8)
(8)
(8) .
After estimating TE, AE, and EE scores, the next step is identifying and analyzing the factors affecting smallholder farmers’ TE, AE, and EE. The Tobit regression model was used to identify and analyze the determinants of the efficiency of smallholder maize farmers. Then, the Tobit regression model Tobin (Citation1958) is specified as follows
(8)
(8)
Where; Εi represents scores of technical, allocative, and economic efficiencies; δi is the vector parameters to be estimated; Zi means various explanatory variables affecting the efficiencies of maize farmers, and Ui is the error term.
2.5. Variables and measures
The definition and measurements of the variables used in the Cobb-Douglas production frontier function and Tobit models are summarized below ().
Table 2. Definitions and measurements of variables.
3. Results and discussion
3.1. Descriptive statistics
Before discussing the results obtained from the econometric models, it is essential to draw a general picture of the study area by briefly presenting the respondents’ demographic, socioeconomic, and institutional characteristics. Among the 198 sample households, approximately 69% were male-headed households. The survey result shows that the average maize productivity status of the study area was 3237 kg (32.37 quintals)/hectare (ha) (). It is equivalent to the Amhara national regional state average productivity (3508 kg per hectare in 2015/16) (CSA, Citation2016). However, it is below the global average maize productivity (5,000 kg or 50 quintals) (Lavagned, Citation2021; Mahuku et al., Citation2015). The average application of DAP and UREA per hectare was 220.71 kg and 180.77 kg, respectively, which is double that of the recommended application per hectare of 100 and 50 kg of DAP and UREA, respectively. Over 78%Footnote3 of the farmland owned by sample farmers was sown by Maize. The average farm size of the farm households in the study area was 1.08 ha, which is a little bit higher than the average farm size of Ethiopian smallholder farmers (0.96 ha) documented by Devereux (Citation2000) and Headey et al. (Citation2014).
Table 3. Socio-demographic characteristics of surveyed households.
Seed quality is among the most significant factors contributing to high maize productivity (T. M. Abate et al., Citation2022). below shows that farmers who used improved maize seed are more productive than non-users. More specifically, the average maize productivity of farmers who used improved seed was 33.30 qt/ha, while the average productivity of non-users was 16.50 qt/ha. As farmers get older, they may gain experience that helps them produce more. However, as farmers get older, they become weaker, and their ability to work is declining. Then, the effect of age on farmers’ efficiency is the net effect of these two trade-off effects. In addition, a growing body of literature argued that the age of farmers affects their productivity through changes in their preference and efficiency in using agricultural inputs and technologies (Li & Sicular, Citation2013; Liu et al., Citation2019). shows that maize farmers are more efficient in the economic and technical terms than in the allocative terms. Farmers were less allocative efficient and slightly decreased with the household head’s age. However, farmers’ economic efficiency level is almost the same for all age categories.
3.2. Factors affecting maize productivity
The value of gamma ( =0.2987) was estimated using the formula
)
) where lambda (λ) is 0.426 (), indicating that 29.87% of the total variation in the productivity of the maize farmers is due to variation in their technical inefficiency. In other words, this implies that the variation in the production of maize farmers is not only due to random variation but also to differences in farmers’ practices. Therefore, in addition to identifying and analyzing factors that affect maize farmer productivity, it is vital to identify and analyze factors that affect their efficiency.
Using human labor significantly and positively affected maize productivity at a significant 1% level. The importance of labor for maize production is unsurprising since smallholder farming is expected to be labor-intensive. The result shows that if the household labor usage for maize cultivation increases by one percent, ceteris paribus, maize productivity will increase by 0.268% (). The use of labor in maize production may be for different activities such as land preparation, planting, and weeding (Geta et al., Citation2013).
Table 4. The stochastic frontier half-normal model estimation result.
The use of chemical fertilizers is a quick remedy input to get higher crop productivity given the current soil fertility depletion problem, especially in Africa (Purbajanti et al., Citation2019). In line with the result found by Adiaha (Citation2017) and Larry Essilfie (Citation2011), the result also shows a positive effect of the use of inorganic fertilizer (DAP) on maize productivity. Farmers who used improved maize seed were 16.80% more productive than local seed users. However, using UREA has been found to have adverse effects on maize productivity. Ceteris paribus, a one percent increase in the use of UREA reduces maize production by 0.079%. But we don’t think using UREA is harmful in itself. However, since UREA aggravates weeds, it can reduce productivity without proper application of it and appropriate weed control. As seen earlier (), farmers used UREA by double the recommended amount. Thus, the negative effect of UREA may be indirectly through exacerbating weeds. Moreover, farmers using irrigation technology were 15.60% more productive than non-users. Irrigation allows higher yields by improving productivity and the advantage of producing in multiple rounds on a given farm.
3.3. Factors affecting the efficiency of smallholder maize farmers
The summary result of the predicted farm-specific technical, allocative, and economic efficiency scores indicates that the average TE, EE, and AE scores were 0.856, 0.861, and 0.738, respectively (). It shows considerable efficiency differences among smallholder farmers, contradicting a large body of empirical research arguing about efficiency benefits to smallholder farmers (Barrett et al., Citation2010; Heltberg, Citation1998). The mean of TE (0.856) implies that if households in the study area operate at full efficiency level on average, they can increase their productivity by 14.40% [(1–0.856) *100], using the existing resources and level of technology. In other words, households in the study area can reduce their inputs by 14.40% to produce what they are currently producing by adopting the resources and technologies used by farmers’ best practices. Maize farmers in the study area were more efficient than a similar study result conducted by Belete (Citation2020) in Ethiopia, indicating that a given input level could increase maize production by 31.07 percent. The average AE score (0.861) suggests that, on average, the sample households in the study area could increase maize production by 26.20% [(1–0.738) *100] if the households used the right inputs and produced the right output relative to the input costs and the price of the product. If farmers could operate at full economic efficiency, they could benefit from 13.90% [(1–0.861 *100)] cost savings.
Table 5. Summary statistics.
The most allocative inefficient farmer would have an efficiency gain of 53% [(1–0.47) *100] to reach the most allocative efficient farm households. In addition, the summary statistics result verified that to reach the most economically efficient counterpart, a farmer could experience an average cost savings of 11.78% [(1–0.861/0.975) *100]. The most economically inefficient household could have an efficiency gain of 44.21% [(1–0.544/0.975) *100] to achieve the level of the most economically efficient household. All these show significant inefficiencies among smallholder maize farmers in the study area.
Identifying and analyzing factors that determine the efficiency level of individual households is the primary intention of this study behind estimating TE, AE, and EE levels. The estimated TE, AE, and EE were regressed to various demographic, socioeconomic, and institutional variables using a Tobit regression model, and the main determinant factors were identified ().
Table 6. Determinants of efficiency in maize production among sample households.
More fragmented land means several pieces of land possibly located in different locations and weather conditions that can allow farmers to reduce drought, flood, snow, and fire risks. However, land fragmentation contributes to inefficiency in various ways. For example, the allocation of land to different places leads to sub-optimal usage of factor inputs that lower overall returns to land due to losses on extra travel time, inadequate monitoring, the inability to consolidate and modernization, and so forth (G. T. Alemu et al., Citation2017; Deininger et al., Citation2014; Kakwagh et al., Citation2011). Additionally, land fragmentation increased the unused space between borders (parcels). In this study, the effect of land fragmentation was negative and significant on smallholder farmers’ technical and allocative efficiencies (). It implies that for a given farm size, as the family farm plot (parcel) increases, the technical and allocative efficiency of the maize-producing farmers would decrease.
The Tobit regression model result also shows that older farmers are less efficient than their younger counterparts. Ceteris paribus, as the age of the household head increases by one year, farmers’ technical and allocative efficiency decreases by 0.2 and 0.4 percent respectivly. It may be because as household heads get older, they may become less interested in the use of new technologies and methods of production as they stay conservative and stick to traditional methods of production (Zegeye et al., Citation2015). And also, aged farmers may be unwilling to take the risk of new experimentation and technologies (Ahmad et al., Citation2002).
Studies have widely argued various advantages of intercropping, including decreasing the risk of total crop reduction, controlling weeds, and improving agricultural resiliency and environmental sustainability (Ghiles et al., Citation2018; Hong et al., Citation2019; Legg & Cuellar, Citation2015). But little is known about its effect on farmers’ efficiency. The result of this study suggests that households that cultivated Maize with two or more crops simultaneously in a single field had a higher level of allocative efficiency than households that grew Maize alone on a specific plot. The simultaneous cultivation of two or more crops in the same area provides efficiency gain might be because it enables efficient land use. Distance from the main market and plot distance had a negative effect on TE. It implies that the farther the plot is from farmers’ residences and the main market, the lower their efficiencies. However, plot distance’s positive effect on farmers’ economic efficiency is unexpected. Here, we argue that farmers may have reduced the number of trips due to higher transport costs (longer time), which leads to substantial cost savings. Furthermore, the positive effect of the expected price of Maize is consistent with Canales et al. (Citation2021) argument that crop prices can affect farm productivity through input-output decisions. As farmers expect a higher output price, large farms intensify farming while small farms expand planted acreage. It also shows that Maize is not only a food crop but also a source of cash for farm households.
Existing evidence suggests that women are not worse than men in the efficiency sense. Instead, women are often less productive than men due to the constraints of essential agricultural inputs. The Tobit regression result shows that male-managed households were less efficient than their female counterparts. It is consistent with the finding of Dadzie and Dasmani (Citation2010) that female-managed farms are more technically efficient than male-managed farms. However, we are unsure where these efficiency differences between male-headed and female-headed households come from. Despite attempts to document male-female efficiency differences, relatively few studies account for individual characteristics of male and female farmers (Gebre et al., Citation2021; Rahman, Citation2010). Due to many socio-cultural values and norms, female-headed households have less access to various farming inputs (credit, land, oxen, etc.), creating considerable efficiency and productivity differences (Adesina & Djato, Citation1997; Gebre et al., Citation2021). As a result, we tried to check out this effect by interacting gender of the household head and access to basic farming inputs. The coefficient of the interaction effect of gender of the household head and access with inputs (Gender* Input_acc), in shows that male headed-households accessed basic farming inputs at the required time and amount have lower technical and economic efficiency (7.5% and 4.9%, respectively) than their female-managed counterparts. It is a surprising result and a good indication that men’s high productivity is due to more use of inputs rather than their production efficiency.
Education helps to improve the effectiveness of combining factors of production to produce a given output level because it opens farmers’ minds to the use of inputs and existing technology, as well as to the adoption of new technology. Education also improves farmers’ awareness and helps them make rational decisions in the production process related to resources and budget allocation (Oduro-Ofori et al., Citation2014). Among 18 studies, including analyses of 37 sets of farm data from 13 countries of Africa, Asia, Europe, and Latin America, in 31 of these data sets, education was found to have a positive and significant effect on technical efficiency in producing a cereal crop, including Maize. However, the effects of the remaining six data sets were adverse but statistically insignificant (Lockheed et al., Citation1980). Although the results were statistically insignificant, they indicate little that education can adversely affect farmers’ efficiency. Similarly, as seen above (), education had a negative but insignificant effect on the technical efficiency of maize farmers.
In addition, education negatively affects farmers’ allocation and economic efficiency, and the effect is statistically significant. However, concluding education reduces the efficiency of farmers may be misleading. And we tried to see the situation from a different perspective. As a result, we conduct a quintile regression. As it is possible to see in the quintile regression graph (), the relationship between education and the efficiency of farmers is not a simple linear relationship. Although the average effect of the linear regression model (OLS) shows the negative impact of education on farmer efficiency, the quantile regression result shows a kind of inverted U-shaped relationship. It means that education helps to improve farmers’ efficiency up to a certain number of years of schooling. However, after specific years of schooling, the efficiency effect of education decreases. It may be because although education can improve farmers’ efficiency in the short run, diverting some agricultural resources and time to education in the long run (more years of schooling) can reduce farmers’ production efficiency. It is consistent with Onphanhdala (Citation2009) argument, which states that a higher level of education is likely to improve the farmer’s ability to have market access and off-farm jobs, which can reduce farming labor and time.
4. Conclusion and policy implications
Many empirical studies suggest that smallholder farms benefit from production efficiency. However, this study documented considerable efficiency differences among smallholder farmers. Though the average maize productivity status of the study area was equivalent to the Amhara national productivity, it is below the global average. Ample studies documented that the cause of low agricultural productivity in Ethiopia is due to insufficient use of agricultural inputs. In this study, however, we found more (double) application of some inputs (DAP and UREA) than recommended. In addition, the average technical, economic, and allocative efficiency scores were 85.60%, 86.10%, and 73.80%, respectively. It also confirms that the low productivity of smallholder farmers in the study areas is not only due to a lack of agricultural inputs and technology but also due to differences in the effectiveness of combining the available inputs and technology in the production process. Although the farms in the study area were better in technical and economic efficiencies than allocative efficiency, the overall efficiency of the maize farms could be substantially improved. The results demonstrate that the age and gender of the household head, distance from the main market, and land fragmentation have adverse and significant impacts on the efficiency of farmers. Furthermore, among production inputs, access and use of improved seed and irrigation, farm labor, and UREA and DAP were among the most important determinant factors of maize productivity. This article also offers new insights into the effect of education on farmers’ efficiency levels, in which the relationship is non-linear. However, to make policy conclusions more confidently, we recommend further empirical research that can investigate the long-run effect of farmers’ education on their efficiency level.
Disclosure statement
The authors report there are no competing interests to declare.
Data availability statement
The data that support the findings of this study are available from the corresponding author, Mengistu Alemu, upon reasonable request.
Additional information
Funding
Notes on contributors

Mengistu Alemu
Mengistu Alemu is an economics lecturer and researcher at Debre Markos University, Ethiopia. He teaches courses mainly such as software applications in economics, development economics, econometrics, and development planning and project analysis. Mengistu Alemu has a specialized master’s in international and development economics from the University of Namur (offered jointly with the Catholic University of Louvain), Belgium. He also had a master’s (M.Sc.) degree in development economics from Bahir Dar University, Ethiopia. His main areas of research interest are inequality and poverty, farm and firm-level efficiency and productivity differences, and the evaluation of public interventions. He has published several articles in reputable international journals. In addition, he has also participated in various research symposiums, seminars, workshops, and conferences. Moreover, he served as a member of the research subcommittee at Debre Markos University (Bure campus).
Notes
1 There is no clear definition of smallholder farmers. The simplest and conventional meaning of a smallholder is that smallholder farmers often have a very limited area of land (less than 2 hectares of land per farm) and operate with mixed farming (growing crops and farming livestock) under a single farm, most labor comes from the family, with no or very limited amounts of hired labor, limited availability of resources, and high vulnerability to risk (Zerssa et al., Citation2021).
2 Kebele is a collection of villages and the smallest administrative unit in Ethiopia.
3 (Average area under maize/average of the total land available) *100.
4 It includes Family labor: Labor performed by members of the family/household and not considered as employees. Hired labor: Paid labor is performed either by members of the family or by non-members of the family. Exchange labor: Work performed by a person in exchange for labor, oxen, land, crops, or other kinds of work.
References
- Abate, T. M., Mekie, T. M., & Dessie, A. B. (2022). Analysis of speed of improved Maize (BH-540) variety adoption among smallholder farmers in Northwestern Ethiopia: Count outcome model. Heliyon, 8(10), 1. https://doi.org/10.1016/j.heliyon.2022.e10916
- Abate, T., Shiferaw, B., Menkir, A., Wegary, D., Kebede, Y., Tesfaye, K., Kassie, M., Bogale, G., Tadesse, B., & Keno, T. (2015). Factors that transformed maize productivity in Ethiopia. Food Security, 7(5), 965–12. https://doi.org/10.1007/s12571-015-0488-z
- Adesina, A. A., & Djato, K. K. (1997). Relative efficiency of women as farm managers: profit function analysis in Cote D’Ivoire. Agricultural Economics, 8.
- Adiaha, M. S. (2017). Economics of Maize (Zea mays L.) production in Nigeria and Maize traditional utilization. International Journal of Scientific World, 5(2), 106. https://doi.org/10.14419/ijsw.v5i2.7819
- Ahmad, M., Chaudhry, G. M., & Iqbal, M. (2002). Wheat productivity, efficiency, and sustainability: A stochastic production frontier analysis. The Pakistan Development Review, 41(4II), 643–663. https://doi.org/10.30541/v41i4IIpp.643-663
- Ahmed, M. H., Tazeze, A., Mezgebo, A., & Andualem, E. (2018). Measuring maize production efficiency in the eastern Ethiopia: Stochastic Frontier approach. African Journal of Science, Technology, Innovation and Development, 10(7), 779–786. https://doi.org/10.1080/20421338.2018.1514757
- Aigner, D., Lovell, C. A. K., & Schmidt, P. (1977). Formulation and estimation of stochastic frontier production function models. Journal of Econometrics, 6(1), 21–37. https://doi.org/10.1016/0304-4076(77)90052-5
- Alemu, M., Berihun, D. C., Lokossou, J., & Yismaw, B. (2022). (PDF) Productivity and efficiency heterogeneity among maize smallholder farmers in Ethiopia. https://www.researchgate.net/publication/365146310_Productivity_and_Efficiency_Heterogeneity_Among_Maize_Smallholder_Farmers_in_Ethiopia
- Alemu, G. T., Berhanie Ayele, Z., & Abelieneh Berhanu, A. (2017). Effects of land fragmentation on productivity in Northwestern Ethiopia. Advances in Agriculture, 2017, 1–9. https://doi.org/10.1155/2017/4509605
- Alemu, D., Mwangi, W., Nigussie, M., & Spielman, D. J. (2008). The maize seed system in Ethiopia: Challenges and opportunities in drought prone areas. African Journal of Agricultural Research 3(4), 305–314.
- Anteneh, A., & Asrat, D. (2020). Wheat production and marketing in Ethiopia: Review study. Cogent Food & Agriculture, 6(1), 1778893. https://doi.org/10.1080/23311932.2020.1778893
- Barrett, C. B., Bellemare, M. F., & Hou, J. Y. (2010). Reconsidering conventional explanations of the inverse productivity–size relationship. World Development, 38(1), 88–97. https://doi.org/10.1016/j.worlddev.2009.06.002
- Belete, A. S. (2020). Analysis of technical efficiency in maize production in Guji Zone: Stochastic frontier model. Agriculture & Food Security, 9(1), 15. https://doi.org/10.1186/s40066-020-00270-w
- Bravo-Ureta, B. E. (1994). Efficiency in agricultural production: The case of peasant farmers in Eastern Paraguay. Agricultural Economics, 11, 27–37
- Bravo-Ureta, B. E., Solís, D., Moreira López, V. H., Maripani, J. F., Thiam, A., & Rivas, T. (2007). Technical efficiency in farming: A meta-regression analysis. Journal of Productivity Analysis, 27(1), 57–72. https://doi.org/10.1007/s11123-006-0025-3
- Canales, E., Bergtold, J. S., & Featherstone, A. (2021). Farm efficiency and productivity growth: The effect of commodity prices. Journal of Applied Farm Economics, 4(1) https://doi.org/10.7771/2331-9151.1053
- Challa, T. M., & Ofolsha, M. D. (2016). Factors affecting Teff and wheat market supply in Dendi district, west Shoa zone. ETHIOPIA, 5(4), 6.
- CSA. (2016). Agricultural Sample Survey 2015/2016, Volume 1 Area and Production of Major Crops. Central Statistical Agency, Federal Democratic Republic of Ethiopia, Addis Ababa. - References—Scientific Research Publishing. https://www.scirp.org/(S(351jmbntvnsjt1aadkposzje))/reference/referencespapers.aspx?referenceid=2611372
- Dadzie, S. K. N., & Dasmani, I. (2010). Gender difference and farm level efficiency: Metafrontier production function approach.
- Deininger, K., Monchuk, D., Nagarajan, H. K., & Singh, S. K. (2014). Does land fragmentation increase the cost of cultivation? Evidence from India, 27.
- Deribe, Y., Bekele, A., Mohammed Oumer, A., & Fikadu, T. (2022). Technical efficiency of maize production in the central rift valley of Ethiopia: Panel data stochastic frontier model (SSRN Scholarly Paper 4226117). https://papers.ssrn.com/abstract=4226117
- Devereux, S. (2000). Food insecurity in ETHIOPIA
- Farrell, M. J. (1957). The measurement of productive efficiency. Journal of the Royal Statistical Society. Series A (General), 120(3), 253. https://doi.org/10.2307/2343100
- Felix Larry Essilfie. (2011). Estimation of farm level technical efficiency in small scale maize production in the Mfantseman municipality in the central region of Ghana: A stochastic frontier approach. Journal of Development and Agricultural Economics, 3(14). https://doi.org/10.5897/JDAE11.069
- Gebre, G. G., Isoda, H., Rahut, D. B., Amekawa, Y., & Nomura, H. (2021). Gender differences in agricultural productivity: Evidence from maize farm households in southern Ethiopia. GeoJournal, 86(2), 843–864. https://doi.org/10.1007/s10708-019-10098-y
- Geta, E., Bogale, A., Kassa, B., & Elias, E. (2013). Productivity and efficiency analysis of smallholder maize producers in Southern Ethiopia. Journal of Human Ecology, 41(1), 67–75. https://doi.org/10.1080/09709274.2013.11906554
- Ghiles, K., Didier, B., Samia, B., Ernest, K., Petra, C., Philippe, D., Dominique, D., Mourad, L., Marc, P., Jean-Jacques, D., & Sidi Mohamed, O. (2018). The effect of intercropping on the efficiency of faba bean – rhizobial symbiosis and durum wheat soil-nitrogen acquisition in a Mediterranean agroecosystem. Plant, Soil and Environment, 64(3), 138–146. https://doi.org/10.17221/9/2018-PSE
- Headey, D., Dereje, M., & Taffesse, A. S. (2014). Land constraints and agricultural intensification in Ethiopia: A village-level analysis of high-potential areas. Food Policy. 48, 129–141. https://doi.org/10.1016/j.foodpol.2014.01.008
- Heltberg, R. (1998). Rural market imperfections and the farm size productivity relationship: Evidence from Pakistan. World Development, 26(10), 1807–1826. https://doi.org/10.1016/S0305-750X(98)00084-9
- Hong, Y., Heerink, N., Zhao, M., & van der Werf, W. (2019). Intercropping contributes to a higher technical efficiency in smallholder farming: Evidence from a case study in Gaotai County, China. Agricultural Systems, 173, 317–324. https://doi.org/10.1016/j.agsy.2019.03.007
- Kakwagh, V. V., Aderonmu, J. A., & Ikwuba, A. (2011). Land fragmentation and agricultural development in Tivland of Benue State. 5.
- Lavagned, O. (2021). (ESS). Agricultural production statistics 2000–2021.
- Legg, J. P., & Cuellar, W. (2015). Control of plant virus diseases, 16
- Li, M., & Sicular, T. (2013). Aging of the labor force and technical efficiency in crop production: Evidence from Liaoning province, China. China Agricultural Economic Review, 5(3), 342–359. https://doi.org/10.1108/CAER-01-2012-0001
- Liu, J., Zhang, C., Hu, R., Zhu, X., & Cai, J. (2019). Aging of agricultural labor force and technical efficiency in tea production: Evidence from Meitan County, China. Sustainability, 11(22), 6246. https://doi.org/10.3390/su11226246
- Lockheed, M. E., Jamison, T., & Lau, L. J. (1980). Farmer education and farm efficiency: A survey. Economic Development and Cultural Change, 29(1), 37–76. http://www.jstor.org/stable/1153584 https://doi.org/10.1086/451231
- Mahuku, G., Lockhart, B. E., Wanjala, B., Jones, M. W., Kimunye, J. N., Stewart, L. R., Cassone, B. J., Sevgan, S., Nyasani, J. O., Kusia, E., Kumar, P. L., Niblett, C. L., Kiggundu, A., Asea, G., Pappu, H. R., Wangai, A., Prasanna, B. M., & Redinbaugh, M. G. (2015). Maize lethal necrosis (MLN), an emerging threat to maize-based food security in Sub-Saharan Africa. Phytopathology, ®105(7), 956–965. https://doi.org/10.1094/PHYTO-12-14-0367-FI
- Meeusen, W., & van Den Broeck, J. (1977). Efficiency estimation from Cobb-Douglas production functions with composed error. International Economic Review, 18(2), 435. https://doi.org/10.2307/2525757
- Oduro-Ofori, E., Aboagye, A. P., & Acquaye, N. (2014). Effects of Education on the Agricultural Productivity of Farmers in the Offinso Municipality. https://www.semanticscholar.org/paper/Effects-of-Education-on-the-Agricultural-of-Farmers-Oduro-Ofori-Aboagye/698f113528397ae62c710315fa7f4d90037ecdd0.
- Onphanhdala, P. (2009). Farmer Education and Agricultural Efficiency: Evidence from Lao PDR. 21.
- Purbajanti, E. D., Slamet, W., Fuskhah, E., Rosyida, (2019). Effects of organic and inorganic fertilizers on growth, activity of nitrate reductase and chlorophyll contents of peanuts (Arachis hypogaea L). IOP Conference Series: Earth and Environmental Science, 250, 012048. https://doi.org/10.1088/1755-1315/250/1/012048
- Rahman, S. (2010). Women’s labour contribution to productivity and efficiency in agriculture: empirical evidence from Bangladesh: Women’s labour contributionto productivity and efficiency in agriculture. Journal of Agricultural Economics, 61(2), 318–342. https://doi.org/10.1111/j.1477-9552.2010.00243.x
- Rapsomanikis, G. (2015). The economic lives of smallholder farmers
- Rashid, S., & Negassa, A. (2013). 5 Policies and performance of ethiopian cereal markets. In P. Dorosh & S. Rashid (Eds.), Food and Agriculture in Ethiopia. University of Pennsylvania Press. https://doi.org/10.9783/9780812208610.123
- Salew, D. (2018). Identification and distribution of intermediate host snails in Mecha woreda, Amhara, North West of Ethiopia. Biodiversity International Journal, 5(2), 441–445. https://doi.org/10.15406/bij.2018.02.00097
- Sileshi, A., Negeri, M., Selvaraj, T., & Abera, A. (2022). Determination of most effective insecticides against maize fall armyworm, Spodoptera frugiperda in South Western Ethiopia. Cogent Food & Agriculture, 8(1), 2079210. https://doi.org/10.1080/23311932.2022.2079210
- Solomon, A., & Teshale, J. (2017). Surveying of cereal crops market chain in west Shoa zone of Oromia regional state. Ethiopia, 6, 10.
- Tadesse, J., Leta, T., Techale, B., & Lemi, B. (2018). Genetic variability, heritability and genetic advance of Maize (Zea mays L.) inbred lines for yield and yield related traits in southwestern Ethiopia. Journal of Plant Breeding and Crop Science, 10(10), 281–289. https://doi.org/10.5897/JPBCS2018.0742
- Tassew, A., & Seifu, E. (2009). Smallholder dairy production system and emergence of dairy cooperatives in Bahir Dar Zuria and Mecha Woredas. 9.
- Tefaye, W., & Beshir, H. (2014). Determinants of technical efficiency in maize production: the case of smallholder farmers in Dhidhessa District of Illuababora Zone.
- Tekalign, F. M. (2019). Determinants of technical efficiency of maize production in Ethiopia: An empirical review. American Journal of Environmental and Resource Economics, 4(4), 144. https://doi.org/10.11648/j.ajere.20190404.15
- Tesema, T. (2021). Analysis of maize production efficiency in maize production in ethiopia evidence from low land of Gudeya Bila: Stochastic Frontier approach. Agro-Economist, 8(1) https://doi.org/10.30954/2394-8159.01.2021.1
- Tesema, T. (2022). Determinants of production efficiency of maize-dominated farmers in western parts of Ethiopia in Gudeya Bila District: Evidence under shifting cultivation area. The Scientific World Journal, 2022, 3355224. https://doi.org/10.1155/2022/3355224
- Thabethe, L., Mungatana, E., & Labuschagne, M. (2014). Estimation of Technical, Economic and Allocative Efficiencies in Sugarcane Production in South Africa: A Case of Mpumalanga Growers. 11.
- Tobin, J. (1958). Estimation of relationships for limited dependent variables. Econometrica, 26(1), 24. https://doi.org/10.2307/1907382
- Van Dijk, M., Morley, T., Van Loon, M., Reidsma, P., Tesfaye, K., & Van Ittersum, M. K. (2020). Reducing the maize yield gap in Ethiopia: Decomposition and policy simulation. Agricultural Systems, 183, 102828. https://doi.org/10.1016/j.agsy.2020.102828
- Zegeye, W., Dejene, M., & Ayalew, D. (2015). Importance of loose smut [Ustilago nuda (Jensen) Rostrup] of barley (Hordeum vulgare L.) in Western Amhara Region. Ethiopia, 9, 10.
- Zerssa, G., Feyssa, D., Kim, D.-G., & Eichler-Löbermann, B. (2021). Challenges of smallholder farming in Ethiopia and opportunities by adopting climate-smart agriculture. Agriculture, 11(3), 192. https://doi.org/10.3390/agriculture11030192