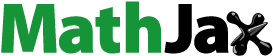
Abstract
This study is aimed at assessing agricultural and environmental performance and analyzing whether observable productivity changes stem from technologically induced or environmentally induced components. Based on individual farm household data from Hubei Province covering the period 2004 to 2010, we decompose total factor productivity (TFP) into technical efficiency change (TEC), technical change (TC), scale effect (SE), and the environmentally related allocative effect (AE) as a means of evaluating environmental performance. The empirical results indicate that the average TFP decrease rate is 2.8%, which reflects the comprehensive outcome of all relevant components. Regarding direct pollution-related inputs (fertilizer and land), improving nitrogen (N) fertilizer application efficiency and land use efficiency can contribute not only to less cropland expansion and greater productivity growth but also to N loss reduction and N pollution abatement in the short and long term. Concerning indirect pollution-related inputs (labor, intermediate input, etc.), although increases in quasi-fixed inputs (labor and intermediate input) can lead to both N and productivity growth, the magnitude of the positive effects of quasi-fixed inputs on productivity cannot offset the negative effect of fertilizer on productivity; thus, more scientific and economical fertilizer application is the key to improving agricultural productivity and benefiting the environment and the ecosystem.
Reviewing editor:
1. Introduction
Due to the key role played by nitrogen (N) in almost all crop metabolic activities and its series of transformations in soil–plant systems, N fertilizer application is economically and environmentally important worldwide. According to the Food and Agricultural Organization (FAO) (2014),Footnote1 globally, farmers use approximately 12 million metric tons of N fertilizer annually. Although fertilizers with enhanced efficiency have been developed to control N release, enabling maximum crop productivity and minimum N loss per unit of N fertilizer (Dimkpa et al., Citation2020), 50–70% of the nitrogen content in fertilizer, which is potentially lost to the environment, contributes to low nitrogen use efficiency (NUE) (Bindraban et al., Citation2020; Cassman et al., Citation2002; Coskun et al., Citation2017; Ladha et al., Citation2005). Hence, it is important to evaluate both the agricultural and the environmental effects of N fertilizer application and find the synergies and trade-offs between agricultural and environmental performance.
To simultaneously evaluate agricultural and environmental performance, two important indices, namely, environmental efficiency (EE) and environmental productivity, are introduced and widely applied in this field to analyze the environmental performance of decision-making units (DMUs). The EE is used to benchmark the environmental performance of a DMU in comparison with those of other DMUs; the environmental productivity is meant to capture the temporary dynamic changes in DMU performance from one period to another (Hoang & Coelli, Citation2011). This study focuses on the latter index. The first aim of this study is to assess agricultural and environmental performance by measuring TFP. Second, we attempt to analyze whether observable productivity changes stem from technologically induced or environmentally induced components by decomposing TFP correctly.
Conventional empirical research on the incorporation of environmental factors into productivity measurements can be divided into three types (Lauwers, Citation2009). First, the environmentally adjusted production efficiency (EAPE) is used to capture environmental performance (Faere et al., Citation1989; Iram et al., Citation2020; Piot-Lepetit & Le Moing, Citation2007; Reinhard et al., Citation1999, Citation2000). The EAPE utilizes environmental indicators as either environmentally detrimental inputs or undesirable outputs of the production function to estimate relevant environmental performance. Second, the frontier formed by multiple environmental, economic, or social factors is measured, and then the frontier eco-efficiency (FEE) is applied across a more extensive range (Callens & Tyteca, Citation1999; Kuosmanen & Kortelainen, Citation2005; Picazo-Tadeo et al., Citation2011, Citation2012). However, these methods have several limitations. Murty et al. (Citation2012) claimed that models that treat pollution as a freely disposable input or weakly disposable output can lead to an inappropriate interpretation of trade-offs among inputs and outputs. In addition, it has been demonstrated that measuring environmental efficiency as the degree to which pollution can be decreased while holding the inputs and outputs constant is infeasible in mathematics and contradicts the material balance principle (MBP) (Coelli et al., Citation2007; Hoang & Coelli, Citation2011). Third, the models follow either the first or second law of thermodynamics. Models following the first law of thermodynamics are generally known as the materials balance approach in economic models, and they have been extensively explored in recent studies. The MBP-based approach regulates the nutrients that are not used for good outputs flow into the environment as potential pollutants (Coelli et al., Citation2007; Eder, Citation2022; Førsund, Citation2021; Hoang & Coelli, Citation2011; Hoang & Nguyen, Citation2013; Reinhard et al., Citation2000; Reinhard & Thijssen, Citation2000; Rødseth, Citation2017). The models following the second law of thermodynamics are generally known as the exergy balance approach in economic studies and have been applied in practice, where EE is defined as the ratio of the total to the minimum cumulative exergy content (Hoang & Rao, Citation2010).
In this paper, we measured TFP within the framework of the stochastic nutrient frontier (SNF) and introduced a TFP decomposition method that is similar to Bauer’s (Citation1990) cost efficiency decomposition based on the Divisia index. This study is different from the existing studies in three aspects. First, it enables researchers to incorporate environmental factors into productivity decomposition and to identify whether observable productivity changes stem from technologically induced or environmentally induced components. The existing methods based on SNF that are used to decompose TFP capture only the TFP changes derived from technical components. However, environmental components should also be included since agricultural production is closely linked to the environment and the ecosystem. In this study, TFP is decomposed into technical efficiency change (TEC), technical change (TC), scale effect (SE), and the environmentally related allocative effect (AE) so that environmental performance can be evaluated. Second, existing studies emphasize the use of TFP or NUE. The first index evaluates the average productivity, and the second index directly reflects the environmental effect of N fertilizer application. We modify the traditional TFP decomposition methods to take environmental factors into account so that both agricultural productivity and environmental effects can be evaluated using the same framework, which avoids the errors and inaccuracies that can occur in a combined analysis of the two indices. Third, the decomposition of the agricultural productivity of China’s rice production industry incorporating environmental factors provides a comprehensive picture of rice production and its potential for both productivity growth and environmental sustainability. To our knowledge, even fewer studies have focused on the productivity of China’s rice production industry and considered environmental factors in productivity decomposition.
In the following section, we illustrate the theoretical framework prior to introducing the data and empirical specification in section three. The empirical results are presented and discussed in section four. Finally, the conclusions are presented in the last section.
2. Theoretical framework
Coelli et al. (Citation2007) suggested that the pollution potential of agriculture can be denoted by the nutrient balance, which equals the total amount of nutrients in inputs minus the amount of nutrients in outputs, and is denoted as follows:
(1)
(1)
where
represents the nutrient balance,
and
denote the output vector and input vector, respectively, and
and
are the vectors that represent the nutrient contents of inputs and outputs. In the context of studying N transformation in rice production, the nutrient balance equals the N that enters rice production in inputs (e.g. land and fertilizer) minus the N that leaves rice production in good outputs (e.g. rice). Considering the crop production process, the nutrient frontier is determined by the output and the nutrients contained in each input. Then, the nutrient frontier function can be denoted by EquationEquation (2)
(2)
(2) :
(2)
(2)
Here, is the minimum nutrient input into the production process given the output vector
and nutrient content vector
at time
. If we use
to denote the observed nutrients, following the Coelli et al. (Citation2007) concept, the nutrient efficiency (NE) can be defined as EquationEquation (3)
(3)
(3) :
(3)
(3)
Since the nutrient frontier function can be viewed as a counterpart of a cost frontier function, the TFP estimated within the nutrient frontier framework can be decomposed in the same way as the single-output and multiple-input case of the cost function approach introduced by Bauer (Citation1990). By taking the natural logarithm and differentiating EquationEquation (3)(3)
(3) , the following equation can be obtained:
(4)
(4)
Here, .Footnote2 After combining EquationEquation (4)
(4)
(4) and the Divisia index
, the TFP can be decomposed as EquationEquation (5)
(5)
(5) :
(5)
(5)
Furthermore, by using , EquationEquation (5)
(5)
(5) can be simplified as follows:
(6)
(6)
Here, and
represent the observed nutrient share and efficient nutrient share, respectively, i.e.
and
.
is the SE.
is the TEC,Footnote3
is the TC and
is the AE of nutrients, which reflects the presence of nutrient allocative inefficiency; i.e. it reflects nutrient allocative inefficiency exists when
, and vice versa. If there are quasi-fixed inputs
, then the nutrient function can be written as
, and there will be an extra component
in EquationEquation (6)
(6)
(6) , which shifts the formula as follows:
(7)
(7)
From EquationEquation (7)(7)
(7) , the advantages of estimating a nutrient frontier rather than other frontiers are obvious. Compared with a fertilizer frontier that considers only non-zero nitrogen input, a nutrient frontier that also involves zero nitrogen input (quasi-fixed input) may be more appropriate for productivity analysis since the quasi-fixed input can limit the use of non-zero nitrogen input and thus impact productivity. In addition, the TFP obtained based on the nutrient frontier contains information about the actual nutrient share and efficient nutrient share, which enables the evaluation of environmental performance. If nutrient allocative inefficiency exists, it indicates that farmers may be able to reallocate their input combination to become more environmentally efficient.
3. Data and empirical specification
3.1. Background
In 2010, China’s rice production reached 19.58 million tons, and the rice yield increased from 2.0 t ha-1 in the 1960s to 6.55 t ha-1 in 2010.Footnote4 As a staple food in China, the demand for rice continues to increase, requiring a 14% higher rice yield by 2030 to nourish the growing population (Cheng et al., Citation2007). There is no doubt that such an increase in rice yield leads to an increase in N fertilizer input. The amount of N fertilizer applied (209 kg ha-1) during rice production in China is 90% greater than the global average (Chen et al., Citation2014). From 2005 to 2020, N fertilizer application decreased in eastern China but increased in western China. Specifically, fertilizer use in western China increased by 0.58 Tg N (8.7%) in 2020 compared to that in 2005, while fertilizer use in eastern China decreased by 3.21 Tg N (18.3%) in 2020 compared to that in 2005 (Chen et al., Citation2022), which can be attributed to switching the type of fertilizer used under the guidance of the National Soil Testing and Nutrient Recommendation Program (Chen et al., Citation2022). Fertilizer overuse is a problem in almost all provinces, with the central region showing the highest degree of fertilizer overuse (Huang & Jiang, Citation2019). Due to the excessive use of N fertilizer, evaluating the agricultural and environmental effects of N fertilizer application and revealing the synergies and trade-offs between agricultural and environmental performance are necessary.
3.2. Data
We used individual farm household data from Hubei Province in 2004–2010, as provided by China’s Rural Fixed Observation Point Bureau. After data cleaning, 1822 observations were used in the empirical model.Footnote5 Due to the various categories of inputs and outputs in the dataset, we aggregated and split the data into five inputs, namely, land, labor, intermediate input, capital and fertilizer, and one output, namely, the total production of rice.Footnote6 The land variable was defined as the total sown area of each household as measured in mu.Footnote7 The labor variable was defined as the total number of days that each household devoted to rice plantation activities. The intermediate input variable is an aggregation of the purchases of seed, pesticide, diesel oil, plastic, etc., noted in yuan. The capital variable is given as the aggregation of the purchases of machinery, agricultural assets, etc., which reflects the input of long-term goods during production.Footnote8 Due to the nature of long-term inputs, their values were depreciated using the straight-line depreciation method, and their average was added to the calculation of capital in yuan.Footnote9 To avoid inflation effects, intermediate input and capital were also adjusted by the consumer price index (CPI).Footnote10 The fertilizer variable included all fertilizers, which were mainly urea, ammonium phosphate and ammonium bicarbonate; these fertilizers are applied during rice production in a single year, as calculated in kilograms. For outputs, the rice output was defined as the total production of rice measured in kilograms. The statistical description of the variables is presented in .
Table 1. Statistical description of the variables.
The total N input for each farm household was calculated as the land N content multiplied by the land area with the addition of the fertilizer N content multiplied by the fertilizer quantity. The land N content and fertilizer N content should be measured at the rice production unit per farm level. However, considering the limitations of the data, only the average value of the land N content variable was available. Thus, we used the ratio of the harvest area to the sown area to adjust the N supply capacity for different farm households to adapt the variable to our research framework. There were 13 observations with zero values for the land N content, 102 observations with zero values and 15 observations with missing values for the fertilizer N content. These observations may have also used other fertilizers, e.g. organic fertilizer and manure, for which calculating the N content is difficult. Although observations containing zero values were not used in the regression, we retained these observations in our dataset, as other information about these observations can be used in the calculation of instant changes at a later stage.
Among the five inputs, labor, intermediate input and capital were assumed to have zero N content. The N content for the land is reflected by the total grams of available N (alkali hydrolysable nitrogen) in the tillage layer per mu, which is generally inorganic nitrogen (nitrate nitrogen and ammonium nitrogen) and easily hydrolyzed organic nitrogen (amino acids, ammonium and easily hydrolyzed proteins). The N supply capacity of paddy soil in Hubei Province is represented by the N capacity of paddy soil at the observation location in the adjacent Hunan Province from 2004 to 2010 according to the Soil Science Database because both provinces have a long history of planting rice,Footnote11 and they are similar regarding paddy soil proportion, size and nutrient composition.Footnote12 Due to the wide range in the different soil layers from which rice roots can absorb nutrients, we chose the 20-centimeter tillage layer to calculate the soil mass. Considering soil fertility heterogeneity, we used the ratio of the harvest area to the sown area to adjust the N supply capacity of different plots. The N content in fertilizer is reflected by the ratio of the total grams of N from all types of fertilizers applied to rice to the total amount of fertilizers applied to rice. After calculating the total N contained in the different types of fertilizers and the proportion of fertilizer applied to rice relative to the total fertilizer applied, the total N contained in the fertilizer applied to rice can be obtained. Despite the possible existing bias arising from the fact that the types of fertilizer applied can vary from crop to crop, our estimation of the N in the fertilizer applied to rice is reliable and referable because rice is the main crop that farmers grow in Hubei Province.
3.3. Empirical specification
As Stijn Reinhard et al. (Citation2000) and Hoang and Nguyen (Citation2013) proposed, labor, capital and intermediate input are considered quasi-fixed inputs, while land and fertilizer are variable inputs. The SNF function (Hoang & Nguyen, Citation2013) can be written as EquationEquation (8)(8)
(8) :
(8)
(8)
which should show non-decreasing values for
and
and fulfill the concavity and linear homogeneity in
. Here,
represents all nutrients contained in the inputs at observation
and time
.
,
and
refer to the respective output
, nutrient content in input
, and quasi-fixed input
at observation
and time
.
refers to the unknown parameters to be estimated.
is assumed to be i.i.d.
.
is assumed to be i.i.d.
.
After comparing the Cobb–Douglas (CD) and translog functional forms in EquationEquation (8)(8)
(8) (Supplementary Table A1), the translog function was used to construct the empirical model. The specific translog nutrient frontier function (the subscripts for farm
and time
are omitted for simplicity) is shown in EquationEquation (9)
(9)
(9) :
(9)
(9)
Here, represents all nutrients, and
is the output vector.
refers to the nutrient content of the land and fertilizer for
.
is a quasi-fixed input vector that denotes labor, intermediate input and capital for
. To accurately estimate the variables that contain zero values, we adopted the Battese (Citation1997) method to address the capital variable, which included 748 zero values. Capital is defined as
, where
if
, and
if
. Thus,
in EquationEquation (9)
(9)
(9) .
,
,
,
and
are the parameters to be estimated. After imposing linear homogeneity on the nutrient contents, the translog nutrient frontier function in EquationEquation (9)
(9)
(9) can be rewritten as EquationEquation (10)
(10)
(10) :
(10)
(10)
Here, , and
. Due to the nature of the production function, endogeneity problems are generally encountered when estimating the production frontier. To capture the correlation between the input variables and the inefficiency term, all the input variables with firm averages were used in the inefficiency model to describe the above endogeneity problem. In the maximum likelihood estimation, all input, output and nutrient content variables were scaled by their sample mean.
4. Results
This section focuses on interpreting the estimation results and subsequently decomposing the TFP. First, the results of the parameter estimates and levels of technical efficiency are presented in . Most parameter estimates in the model were statistically significant; thus, the results of our model were acceptable. Additionally, the log likelihood values of the different models (Supplementary Table A1) indicated that the distance function and inefficiency model were appropriate. Subsequently, the components of TFP change are presented to show how different factors contribute to TFP variation.
Table 2. Parameter estimates.
4.1. Parameter estimates and technical efficiency
shows that increases in fertilizer N content, land N content, rice output, labor, and intermediate input led to N growth, and the absolute values of the nutrient elasticities reflected the relative share of N growth caused by each term. During the period 2004–2010, the N content in fertilizer and land contributed to uneven N growth, i.e. 33 and 67%, respectively. This indicates that due to limits on the type and amount of fertilizer that can be taken up by plants, a one percent increase in fertilizer N content can account for approximately one-third of the total N growth. Unlike the fertilizer N content, our sample showed that the land N content varied significantly from plot to plot, which means that fertile land can lead to an enormous increase in N growth; however, this is not a concern since the remaining N in the soil can still be used for future production. The nutrient elasticity of rice output (0.54) suggested that a 1% increase in rice output can lead to 54% N growth. For nutrient elasticities that are related to the quasi-fixed input, increases in labor and intermediate input also contributed to N growth due to the complementary relationships among inputs (Supplementary Tables A3–A5).Footnote13 Therefore, increases in labor and intermediate input also indirectly led to total N growth through increasing fertilizer use. The impact of capital on N growth was uncertain because it took a long time to appear.
Table 3. Nutrient elasticities.
The average technical efficiency was approximately 0.87 (Supplementary Table A2). Overall, the technical efficiencies exhibit a decreasing trend during the period 2004–2010, particularly in 2004–2005, which is further verified by the F-test results.Footnote14 This decreasing trend in estimated technical efficiency is in line with trends found in adjacent Asian countries (i.e. Thailand, Vietnam and the Philippines) during the same period (Alam et al., Citation2011; Orawan & Somporn, Citation2012; Villano & Fleming, Citation2006).
4.2. Components of TFP change
provides descriptive statistical information about the TFP components. The average decrease rate of TFP was 2.8%, which reflects the comprehensive outcome of all relevant components. Among all components, effect of labor, effect of intermediate input and SE exerted positive effects on TFP change. The effect of labor (0.007) and intermediate input (0.001) indicated that average productivity increased due to increases in labor and intermediate input. The positive SE (0.012) implies that TFP grows when farmers adjust their size to achieve the most productive scale size (MPSS).
Table 4. Components of TFP.
On the other hand, the negative impacts of the effect of capital (−0.023), the AE of fertilizer nutrient (−0.011), the technical regression (−0.007) and the AE of land nutrient (−0.003) were the main reasons for the decrease in TFP. The negative AE of fertilizer nutrient (−0.011) indicated that the observed nutrient share was larger than the efficient nutrient share when the instant changes in fertilizer N content decreased (Supplementary Table A6); i.e. farmers use more chemical fertilizer than needed. The overuse of N fertilizer can be directly reflected by the N surplus per unit of land, and the N surplus (58 kg N ha−1) resulting from current practices can be reduced to 16 kg N ha−1 through the use of integrated soil crop practices that utilize advanced crops and implement nutrient management (Chen et al., Citation2014). Facing increasing concern regarding the devastating environmental effects of N fertilizer overuse (Yu et al., Citation2019; Zhang et al., Citation2020), the Chinese government has endeavored to curb N fertilizer use. In 2015, the Ministry of Agriculture and Rural Affairs initiated the strategy of Zero Growth in Synthetic Fertilizer Use to improve NUE and further decrease N fertilizer use. By 2020, the N fertilizer application amount had been in decline for consecutive years, and the NUE (40.2%) had increased by 5% compared to that in 2015.Footnote15 The negative AE of land nutrient (−0.003) indicates that the current use of land is not economical, and by integrating small, fragmented areas of arable land and improving land use efficiency, both less cropland expansion and greater productivity growth can be achieved.
The negative effect of capital suggests that an increase in capital cannot lead to average productivity growth. In addition, the total N increased at an annual rate of 0.7%. There are two reasons for this: one is the improvement in industrialization, and the other is the low level of NUE. To satisfy increasing material needs and industrial growth, the rapid development of agriculture is generally associated with high energy consumption, high pollution, high waste and low efficiency. This agricultural development pattern inevitably leads to excess fertilizer use, pesticide use, etc. In addition, due to the volatile and run-off nature of N fertilizer, China’s NUE for cereal was only 25.5% in 2010, which is much lower than the global average and that value in the USA (Omara et al., Citation2019). The low NUE causes an increase in N fertilizer application, and the excess N fertilizer application inhibits the absorption of other nutrients, which results in a vicious cycle. Hence, increasing NUE and manure recycled to soil can largely reduce N loss and abate N pollution (Wang et al., Citation2023).
5. Conclusions
This empirical research yields several important findings. First, increases in fertilizer N content, land N content, rice output, labor and intermediate input all led to N growth during our research period. However, the effects of different inputs on N pollution varied. For direct pollution-related inputs (fertilizer and land), improving N fertilizer application efficiency and land use efficiency can contribute not only to less cropland expansion and greater productivity growth but also to N loss reduction and N pollution abatement in the short and long term. For indirect pollution-related inputs (labor, intermediate input, etc.), although increases in quasi-fixed inputs (labor and intermediate input) can lead to both N and productivity growth, the magnitude of the positive effects of quasi-fixed inputs on productivity cannot offset the negative effect of fertilizer on productivity; thus, scientific and economical fertilizer application is the key to improving agricultural productivity and benefiting the environment and the ecosystem. Second, considering the allocative inefficiency of land, improving the land circulation market and integrating small, fragmented areas of arable land may be beneficial for reducing N pollution and increasing productivity. Third, farmers can improve TFP by increasing TC and TEC. Considering the positive effects of technical progress and technical efficiency increase, it is necessary to strengthen the role of TEC improvement in agricultural development and fully combine TC and TEC improvement. This can be achieved, for instance, by promoting the integration of innovative agricultural talent and new technologies (Fang et al., Citation2021), strengthening technological advancements, improving production efficiency in terms of green development, expanding the agricultural opening-up level, and minimizing the energy intensity of the agricultural sector (Hamid et al., Citation2023).
Agricultural green development is the key to resolving agricultural resource problems and environmental problems in China (Hamid & Wang, Citation2022). The decomposition of TFP within the framework of SNF elucidates the relationship between agricultural performance and environmental performance, but there is still room for improvement through future research. First, due to the limitations of data acquisition, only the data from 2004 to 2010 is used in the empirical model. With the further development of databases, more sufficient empirical analysis can be carried out. Second, studies involving more nutrients and environmental factors can be conducted since land and fertilizer N taken up by plants are also affected by other nutrients and physical conditions, such as phosphorus content, potassium content, sunshine, temperature, and rainfall. Third, from the academic research perspective, the empirical model in this study is constructed through microlevel data, and an analysis from the macrolevel perspective is lacking. Due to the focus on the issue of agricultural green production in academic research, China’s agricultural development has a lot of research and discussion space in optimizing production structure and mode and enriching research perspectives.
Correction Statement
This article has been corrected with minor changes. These changes do not impact the academic content of the article.
SNF and TFP_support info.docx
Download MS Word (37 KB)Disclosure statement
No potential conflict of interest was reported by the author(s).
Additional information
Notes on contributors
Yuan Ma
Dr. Yuan Ma received the doctoral degree in Economics from University of Göttingen, Germany in 2021. Her areas of interests include Agricultural Economics and Environmental and Resource Economics.
Bernhard Brümmer
Prof. Dr. Bernhard Brümmer is working as Professor at Department of Agricultural Economics and Rural development, University of Göttingen. His areas of interest include measurement of productivity growth and transmission of policies on rural markets, reform of the Common Agricultural Policy, spatial and vertical market integration analysis.
Xiaohua Yu
Prof. Dr. Xiaohua Yu is working as Professor at Department of Agricultural Economics and Rural development, University of Göttingen. His areas of interest include Agricultural Economics, Environmental Economics, Behavioral Economics, Data Sciences and China Economy.
Notes
2 RTS refers to returns to scale.
3 Note that the TEC is not the pure technical efficiency change, which can be further decomposed into pure technical efficiency change and allocative efficiency change.
4 The data were obtained from the China Statistic Yearbook (2011).
5 The specific data processing statement can be found in the Supplementary Appendix.
6 All of the inputs and outputs such as land, labor, intermediate input, fertilizer and capital refer to the inputs and outputs in the process of rice production based on the fourth section (farm household production and operation status) of the original questionnaire, which included the specific input and output situation of each crop.
7 1 mu equals approximately 0.067 hectares.
8 In this paper, capital does not reflect the economic notion of capital appropriately. Hence, the capital variable used in this study should be interpreted with caution.
9 The long-term inputs depreciated consistently over ten years.
10 The CPI was obtained from the National Bureau of Statistics (see http://www.stats.gov.cn/). Compared with other price indices and financial indices, the CPI may not be the best choice for reflecting the true value change of intermediate input and capital, but it is a good choice for reflecting the true price change of farmers’ inputs concerning our research framework.
11 See http://vdb3.soil.csdb.cn/.
12 See http://vdb3.soil.csdb.cn/.
13 Since the prices of quasi-fixed input are measured by price indices that do not reflect the price changes faced by individuals, we estimated the relationships between the fertilizer price faced by individuals (which can be calculated from the dataset) and different quasi-fixed inputs used per land unit through the OLS model. The regression results suggest that the coefficients were statistically significant at the 10% level, and their signs can provide a preliminary view of the relationships among fertilizer price and different quasi-fixed inputs.
14 The F value and p value obtained from the F-test for equal mean technical efficiency scores over years are 2.28 and 0.03, respectively.
References
- Alam, M. J., Van Huylenbroeck, G., Buysse, J., Begum, I. A., & Rahman, S. (2011). Technical efficiency changes at the farm-level: A panel data analysis of rice farms in Bangladesh. African Journal of Business Management, 5(14), 1–10.
- Battese, G. E. (1997). A note on the estimation of Cobb-Douglas production functions when some explanatory variables have zero values. Journal of Agricultural Economics, 48(1–3), 250–252. https://doi.org/10.1111/j.1477-9552.1997.tb01149.x
- Bauer, P. W. (1990). Decomposing TFP growth in the presence of cost inefficiency, nonconstant returns to scale, and technological progress. Journal of Productivity Analysis, 1(4), 287–299. https://doi.org/10.1007/BF00160047
- Bindraban, P. S., Dimkpa, C. O., White, J. C., Franklin, F. A., Melse–Boonstra, A., Koele, N., Pandey, R., Rodenburg, J., Senthilkumar, K., Demokritou, P., & Schmidt, S. (2020). Safeguarding human and planetary health demands a fertilizer sector transformation. Plants, People, Planet, 2(4), 302–309. https://doi.org/10.1002/ppp3.10098
- Callens, I., & Tyteca, D. (1999). Towards indicators of sustainable development for firms: A productive efficiency perspective. Ecological Economics, 28(1), 41–53. https://doi.org/10.1016/S0921-8009(98)00035-4
- Cassman, K. G., Dobermann, A., & Walters, D. T. (2002). Agroecosystems, nitrogen-use efficiency, and nitrogen management. Ambio, 31(2), 132–140. https://doi.org/10.1579/0044-7447-31.2.132
- Chen, X., Cui, Z., Fan, M., Vitousek, P., Zhao, M., Ma, W., Wang, Z., Zhang, W., Yan, X., Yang, J., Deng, X., Gao, Q., Zhang, Q., Guo, S., Ren, J., Li, S., Ye, Y., Wang, Z., Huang, J., … Zhang, F. (2014). Producing more grain with lower environmental costs. Nature, 514(7523), 486–489. https://doi.org/10.1038/nature13609
- Chen, Y., Zhang, L., Zhao, Y., Zhang, L., Zhang, J., Liu, M., Zhou, M., & Luo, B. (2022). High-resolution ammonia emissions from nitrogen fertilizer application in China during 2005–2020. Atmosphere, 13(8), 1297. https://doi.org/10.3390/atmos13081297
- Cheng, S. H., Zhuang, J. Y., Fan, Y. Y., Du, J. H., & Cao, L. Y. (2007). Progress in research and development on hybrid rice: A super-domesticate in China. Annals of Botany, 100(5), 959–966. https://doi.org/10.1093/aob/mcm121
- Coelli, T., Lauwers, L., & Van Huylenbroeck, G. (2007). Environmental efficiency measurement and the materials balance condition. Journal of Productivity Analysis, 28(1–2), 3–12. https://doi.org/10.1007/s11123-007-0052-8
- Coskun, D., Britto, D. T., Shi, W., & Kronzucker, H. J. (2017). Nitrogen transformations in modern agriculture and the role of biological nitrification inhibition. Nature Plants, 3(6), 17074. https://doi.org/10.1038/nplants.2017.74
- Dimkpa, C. O., Fugice, J., Singh, U., & Lewis, T. D. (2020). Development of fertilizers for enhanced nitrogen use efficiency–trends and perspectives. The Science of the Total Environment, 731, 139113. https://doi.org/10.1016/j.scitotenv.2020.139113
- Eder, A. (2022). Environmental efficiency measurement when producers control pollutants under heterogeneous conditions: A generalization of the materials balance approach. Journal of Productivity Analysis, 57(2), 157–176. https://doi.org/10.1007/s11123-021-00623-y
- Fang, L., Hu, R., Mao, H., & Chen, S. (2021). How crop insurance influences agricultural green total factor productivity: Evidence from Chinese farmers. Journal of Cleaner Production, 321, 128977. https://doi.org/10.1016/j.jclepro.2021.128977
- Faere, R., Grosskopf, S., Lovell, C. A. K., & Pasurka, C. (1989). Multilateral productivity comparisons when some outputs are undesirable: A nonparametric approach. The Review of Economics and Statistics, 71(1), 90. https://doi.org/10.2307/1928055
- Førsund, F. R. (2021). Performance measurement and joint production of intended and unintended outputs. Journal of Productivity Analysis, 55(3), 157–175. https://doi.org/10.1007/s11123-021-00599-9
- Hamid, S., & Wang, K. (2022). Environmental total factor productivity of agriculture in South Asia: A generalized decomposition of Luenberger–Hicks–Moorsteen productivity indicator. Journal of Cleaner Production, 351, 131483. https://doi.org/10.1016/j.jclepro.2022.131483
- Hamid, S., Wang, Q., & Wang, K. (2023). The spatiotemporal dynamic evolution and influencing factors of agricultural green total factor productivity in Southeast Asia (ASEAN-6). Environment, Development and Sustainability, https://doi.org/10.1007/s10668-023-03975-7
- Hoang, V.-N., & Coelli, T. (2011). Measurement of agricultural total factor productivity growth incorporating environmental factors: A nutrients balance approach. Journal of Environmental Economics and Management, 62(3), 462–474. https://doi.org/10.1016/j.jeem.2011.05.009
- Hoang, V.-N., & Nguyen, T. T. (2013). Analysis of environmental efficiency variations: A nutrient balance approach. Ecological Economics, 86, 37–46. https://doi.org/10.1016/j.ecolecon.2012.10.014
- Hoang, V.-N., & Rao, D. P. (2010). Measuring and decomposing sustainable efficiency in agricultural production: A cumulative exergy balance approach. Ecological Economics, 69(9), 1765–1776. https://doi.org/10.1016/j.ecolecon.2010.04.014
- Huang, W., & Jiang, L. (2019). Efficiency performance of fertilizer use in arable agricultural production in China. China Agricultural Economic Review, 11(1), 52–69. https://doi.org/10.1108/CAER-12-2017-0238
- Iram, R., Zhang, J., Erdogan, S., Abbas, Q., & Mohsin, M. (2020). Economics of energy and environmental efficiency: Evidence from OECD countries. Environmental Science and Pollution Research International, 27(4), 3858–3870. https://doi.org/10.1007/s11356-019-07020-x
- Kuosmanen, T., & Kortelainen, M. (2005). Measuring eco-efficiency of production with data envelopment analysis. Journal of Industrial Ecology, 9(4), 59–72. https://doi.org/10.1162/108819805775247846
- Ladha, J. K., Pathak, H., Krupnik, T. J., Six, J., & van Kessel, C. (2005). Efficiency of fertilizer nitrogen in cereal production: Retrospects and prospects. Advances in Agronomy, 87, 85–156. https://doi.org/10.1016/S0065-2113(05)87003-8
- Lauwers, L. (2009). Justifying the incorporation of the materials balance principle into frontier-based eco-efficiency models. Ecological Economics, 68(6), 1605–1614. https://doi.org/10.1016/j.ecolecon.2008.08.022
- Murty, S., Robert Russell, R., & Levkoff, S. B. (2012). On modeling pollution-generating technologies. Journal of Environmental Economics and Management, 64(1), 117–135. https://doi.org/10.1016/j.jeem.2012.02.005
- Omara, P., Aula, L., Oyebiyi, F., & Raun, W. R. (2019). World cereal nitrogen use efficiency trends: Review and current knowledge. Agrosystems, Geosciences & Environment, 2(1), 1–8. https://doi.org/10.2134/age2018.10.0045
- Orawan, S., & Somporn, I. (2012). Efficiency change in Thailand rice production: Evidence from panel data analysis. Journal of Development and Agricultural Economics, 4(4), 101–108.
- Picazo-Tadeo, A. J., Beltrán-Esteve, M., & Gómez-Limón, J. A. (2012). Assessing eco-efficiency with directional distance functions. European Journal of Operational Research, 220(3), 798–809. https://doi.org/10.1016/j.ejor.2012.02.025
- Picazo-Tadeo, A. J., Gómez-Limón, J. A., & Reig-Martínez, E. (2011). Assessing farming eco-efficiency: A data envelopment analysis approach. Journal of Environmental Management, 92(4), 1154–1164. https://doi.org/10.1016/j.jenvman.2010.11.025
- Piot-Lepetit, I., & Le Moing, M. (2007). Productivity and environmental regulation: The effect of the nitrates directive in the French pig sector. Environmental and Resource Economics, 38(4), 433–446. https://doi.org/10.1007/s10640-007-9086-7
- Reinhard, S., Lovell, C. K., & Thijssen, G. J. (1999). Econometric estimation of technical and environmental efficiency: An application to Dutch dairy farms. American Journal of Agricultural Economics, 81(1), 44–60. https://doi.org/10.2307/1244449
- Reinhard, S., Lovell, C. K., & Thijssen, G. J. (2000). Environmental efficiency with multiple environmentally detrimental variables; estimated with SFA and DEA. European Journal of Operational Research, 121(2), 287–303. https://doi.org/10.1016/S0377-2217(99)00218-0
- Reinhard, S., & Thijssen, G. (2000). Nitrogen efficiency of Dutch dairy farms: A shadow cost system approach. European Review of Agriculture Economics, 27(2), 167–186. https://doi.org/10.1093/erae/27.2.167
- Rødseth, K. L. (2017). Axioms of a polluting technology: A materials balance approach. Environmental and Resource Economics, 67(1), 1–22. https://doi.org/10.1007/s10640-015-9974-1
- Villano, R., & Fleming, E. (2006). Technical inefficiency and production risk in rice farming: Evidence from Central Luzon Philippines*. Asian Economic Journal, 20(1), 29–46. https://doi.org/10.1111/j.1467-8381.2006.00223.x
- Wang, X., Xu, M., Lin, B., Bodirsky, B. L., Xuan, J., Dietrich, J. P., Stevanović, M., Bai, Z., Ma, L., Jin, S., Fan, S., Lotze-Campen, H., & Popp, A. (2023). Reforming China’s fertilizer policies: implications for nitrogen pollution reduction and food security. Sustainability Science, 18(1), 407–420. https://doi.org/10.1007/s11625-022-01189-w
- Yu, C., Huang, X., Chen, H., Godfray, H. C. J., Wright, J. S., Hall, J. W., Gong, P., Ni, S., Qiao, S., Huang, G., Xiao, Y., Zhang, J., Feng, Z., Ju, X., Ciais, P., Stenseth, N. C., Hessen, D. O., Sun, Z., Yu, L., … Taylor, J. (2019). Managing nitrogen to restore water quality in China. Nature, 567(7749), 516–520. https://doi.org/10.1038/s41586-019-1001-1
- Zhang, Q., Chu, Y., Xue, Y., Ying, H., Chen, X., Zhao, Y., Ma, W., Ma, L., Zhang, J., Yin, Y., & Cui, Z. (2020). Outlook of China’s agriculture transforming from smallholder operation to sustainable production. Global Food Security, 26, 100444. https://doi.org/10.1016/j.gfs.2020.100444