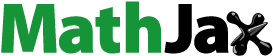
Abstract
Africa has recently experienced adverse climate changes and has recognized tremendous advancement of numerous Climate Smart Agriculture (CSA) technologies with ability to promote resilience and productivity. However, these good strategies are not only unknown, but their extensive uptake remain subtle particularly beyond the scope area, especially among poor rural small-scale farmers. There are prevailing barriers that inhibit upscaling of these practices and so far existing actions and policies to remove the challenges remain scarce. Therefore, the study’s main objective was to assess determinants and or barriers and strategies to boost scaling up pathways for adopted CSA practices in Nyando basin. Primary data was collected for baseline, end-line and financial diaries panel data from 122 households. Logistic regression model and Descriptive statistics were used to analyze the data. The main findings which were significant (p < 0.05) illustrated that shocks from the emergence of new pests/diseases, and little/high rainfall highly affected productivity, resulting in dis-adoption and scaling up. Climate variant was the main cause of floods, drought, and the occurrence of new pests on crops and livestock. There was evidence of households’ collective decisions on farming expenditures. Knowledge astute, social capital and market-based scaling pathways were potential “pull” aspects that could encourage scaling up of CSA practices beyond CSVs. The study recommends improving use of multi-faceted approaches that assimilate environmental, agronomic, molecular, and institutional scopes. Additionally, improve on market access, cooperatives and capacity building base level for small-scale farmers to relate constraints to solutions offered by CSA adoption.
Reviewing Editor:
1. Introduction
Agriculture sector is the most exposed to the effects of changing climate promoting risks of increased temperatures, unpredictable rainfall patterns, more floods and drought. These climatic uncertainties can have major impacts on water availability affecting agricultural productivity (Dhungana et al., Citation2020; Xu et al., Citation2019). Rainfall and temperature climate variables are the most essential to agricultural production (Fahad et al., Citation2018, Citation2019a). It is projected that low precipitation could affect crop planting and harvesting in the next two to three decades (Amanullah et al., Citation2020). Additionally, unexpected and higher rainfall are a reality in many parts of the world (Wester et al., Citation2019). The average and seasonal maximum temperatures are projected to continue rising (Thakuri et al., Citation2019). It is therefore paramount that farmers’ approach of agricultural systems adaptation to changing weather patterns now and in future will necessitate a multifaceted action. Adoption of harsh weather-resilient crops including other climate smart technologies is important in decreasing the effect of changing climate patterns on agriculture and safeguarding global food security (Dey, Citation2023). The approaches should comprise of new ideas, strategies and technologies that integrate environmental, agronomic, social, molecular, and institutional scopes that will be binding (Harrison et al., Citation2021; Sloat et al., Citation2020; Bell et al., Citation2015).
Globally, in the effort of Climate Change, Agriculture and Food Security (CCAFS) under the CGIAR program, has supported research on climate-smart agriculture (CSA) in different parts of the world. The main objective of the smart practices, technologies and information is to respond to the constraint of adoption to a wider horizon under unpredictable weather patterns. In spite of the vital worldwide action and outlay in CSA, there is inadequate proof of what technologies and practices work, and what the interactions and trade-offs are amongst the three pillars (productivity, adaptation and mitigation) of CSA (Martinez-Baron et al., Citation2018). Additionally, there is uncertainty if there are up-and-coming scaling strategies (comprising fiscal access) which can create a revolution of agriculture (Vernooy & Bouroncle, Citation2019). Though CSA presents noteworthy gains in standard, the time lag and the capital (finances) needed as ventures can be negative factors hampering their uptake by farmers (Gunjal, Citation2016).
In Africa CCAFS have initiated a pilot using the CSV approach in 2012–2014 in: Kenya, Tanzania, Uganda Ethiopia, Burkina Faso, Mali, Ghana, Niger, Senegal, and South Asia: Bangladesh, India, Nepal and Latin America (Vernooy & Bouroncle, Citation2019). The key aim is to depict evidence that will bring out appropriate CSA interventions focusing on poor rural farmers via team work and appraisal via research (Aggarwal et al., Citation2018). With its focus on the co-development of CSA technologies at the community level, the CSV approach is also expected to empower local communities and their supporting institutions (Bayala et al., Citation2021). However, scaling of CSV sustainably requires an active engagement of institutions. The proportion of smallholder farmers partaking CSV activities comprises a fairly minor percentage of all the farmers in the village or community. In Guatemala, Nepal and Vietnam only 10–20% of the farmers in CSVs are trained to gain skills and knowledge on technology advancement; gender dynamics; monitoring and evaluation as well as planning, (Vernooy & Bouroncle, Citation2019). In spite of CSA significance and promotion, a major concern is its low wide-scale adoption, especially amongst small-holder farmers in East Africa (Makate, Citation2019). To realize outcomes at wider and advanced scale across national, county and village level, a multifaceted approach that is inclusive and multi-objective that support scaling CSA interventions are needed but lacking (Scherr et al., Citation2012). Scaling strategies in the third wave according to Schut et al. (Citation2020) need to lean on evidence, as well as receptive to the agro-ecological and socio-economic dynamics. They should account for synergies and trade-offs between CSA’s three pillars across scales (Shirsath et al., Citation2017; Descheemaeker et al., Citation2016; Scherr et al., Citation2012).
The aim of CCAFS CSV approach on CSA is to form an assortment of options that collectively lead to the triple wins, however, this has raised some undefined issues (Aggarwal et al., Citation2018). The major dispute question is what component of house-holds need to exercise active CSA interventions to turn a community/region into a climate-smart village or region? The plan of CCAFS intended scaling to operate via financial and institutional mechanisms enabling fruitful uptake by a large number of farmers within and around CSV sites, including a more ambitious scaling goal, far beyond the CSVs (Kinyangi et al., Citation2015). CCAFS assumed that national and county governments could easily scale out the interventions through private-sector actors and NGOs in regions with similar agro-ecological conditions, through their projects and programs (Vernooy & Bouroncle, Citation2019). In a study by Kirina et al. (Citation2022), across 25 cases they sought answers on how CSA is scaled in the East African context, find gaps and entry points, and add knowledge to the scaling discourse. However, their results highlighted areas of concern that scaling drive should contemplate henceforth: lack of understanding what the end-user values, needs, and prioritizes towards scaling. CSA is critically reduced if there is ignorance of the spatial variation, and un-targeted execution may cause un-favourable feedback effects on the potential for scaling. Finally, another key challenge to scaling is creating an enabling environment through systemic change (Kirina et al., Citation2022).
In Kenya, in order to gauge the adoption rates of CSA in the study area, Oostendorp et al. (Citation2021) used this study’s primary data (Climate smart financial diaries, baseline and end line data 2018–2019). They further compared the results with secondary data from CCAFS evaluation Survey, (2017) in order to draw a conclusive synopsis of CSA adoption rates. Their findings revealed that it was evident that dis-adoption rates were relatively soaring for majority of the CSA practices. On crop management, the adoption of high yielding seed varieties >80% of the respondents in CSVs who had adopted in 2017 continued though fairly lower till 2020 unlike in the non-CSV where a decline was noted. Fertilizer application was considered a CSA mechanism because of escalating prices in 2017 and henceforth in Kenya. Its application remains unaffordable to majority of the farmers who opted not to use it till late 2022/2023 when the government subsidized the input. Pesticides and herbicides utilization increased though below 59%.
For improved breeds, reported dis-adoption rates by farmers were equally high. The respondents with improved black head Masai sheep (12%) and Galla goats (24%) in 2017 did not possess the breed in 2020 in the CSV. Though there was an increase in adoption of improved chicken, the rates remained minimal in both non-CSVs and CSVs. Additionally, for both sites land management practices indicated that more farmers were planting trees over the period 2019–2020. There was an increasing trend on mixed cropping and construction of ridges in 2019, however employing of terraces to conserve soils was declining perhaps the reason being that they were altered for tree adoption, and due to high labour intensiveness in digging and related costs. Oostendorp et al. (Citation2021) study highlights a concern on the high rates of dis-adoption of CSA practices affecting up and out scaling. This research fills the gaps highlighted above by assessing the determinants of scaling up adopted CSA affecting pathways to scaling out among smallholder farmers in Nyando, Kenya. The study used household level financial diaries and field observations from the respondents.
1.1. Literature review
According to Aggarwal et al. (Citation2018) the main objective of scaling-up the ideal CSA preferences and policies is to draw lessons from successful stories. For example using horizontal scaling out (scaling out) farmer to farmer learning is achieved at CSV demonstration sites whereby favourable agricultural technologies and practices are nurtured. Through organized field exposure visits and/or trainings, both supporting institutions and farmers learn and promote successful CSA technologies through programs, local policies, and grassroot institutions. In vertical scaling (scaling up) the success of the CSV program influences policymakers, funding agencies, and implementing organizations so that changes in policy instruments, institutions, or investments are made for the promotion of promising technologies and practices. For example in Senegal, expansion of a pilot CSA program to other regions has been feasible using farm advisories and climate forecast by collaborating partnership between institutions working across multiple levels (CCAFS, Food and Agriculture Organization [FAO], Citation2016). Subsequently, Haryana state in India, the state government has made policy changes to promote promising CSA practices under CSV programs pledging to promote it to 500 villages (Aryal et al., Citation2020). The definitive objective is to inspire achievements which will permit smallholder farmers be resilient and have ability to mitigate climate variability.
According to Andati et al. (Citation2022), farmers may attempt to adapt to prevailing shocks and their entrepreneurial acumen becomes key in farmers adapting to arising changes. However, he found out that sometimes choosing ideal CSA practices maybe delicate for farmers depending on the attributes, socio-economic characteristics and their entrepreneurial experience. Whilst there exists proof in a majority of empirical literature on the influence of farm and farmer characteristics on CSA up-take (Ngigi et al., Citation2017; Mulwa et al., Citation2017; Makate et al., Citation2016; Kurgat et al., Citation2020), there is scanty information on the influence of farmers cognitive traits. They relay learning and the logic behaviour/psychological perception of costs and risks associated with adoption of a practice (World Bank, 2015; Barzola Iza & Dentoni, Citation2020; Kangogo et al., Citation2021). Despite its potential, however, CSA adoption remain generally minimal across the SSA countries (Mulwa et al., Citation2017; Kurgat et al., Citation2020). According to Ogada et al. (Citation2020) the low uptake affecting scaling up depends on differing aspects in diverse regions and agro ecological zones in Kenya. The aspect of CSA adoption is important since it informs future up or out scaling.
A study by Njuguna (Citation2020) states that it is not possible to combine some of the CSA practices because they concurrently burden some aspects of the farming structure due to high costs, labour challenges and contest for crop residues. Taylor (Citation2018) raised concerns about CSA approaches as to whether their technical orientation nature, which falls short in consideration for labour proceeds, consumption patterns, and land ownership queries. The concepts of Climate-smart agriculture are also often misunderstood by researchers affecting up-take rates by farmers and scaling up (Saj et al., Citation2017). Additionally, according to Autio et al. (Citation2021) and FAO (Citation2013) there are several barriers advancing decline or averting the uptake of CSA, particularly by small scale farmers. These include: a long time interval of 4 or extra years before grasp of full payback, and high expenditure at initial stage. Since, the expenses are often ample it becomes a key restraint to CSA adoption by many poor rural farmers; unfortunately, this is left upon the capability and motivation of the farmers to search for guidance, training and fiscal support. These constraints are imperative and in order to come to the aid of farmers, they can only be conquered using urgent precedence, proper policies and rigorous hard work in solution enquiry by all concerned parties (Gunjal, Citation2016).
In Sub-Saharan Africa (SSA) scientific proven innovations through researches may offer significant solutions however they signify low adoption rate. There is great potential to promote sustainable agriculture with the advancement of climate-resilient crops to improve crops to be stress tolerant (Dey, Citation2023). This can use several approaches like genetic engineering like transgenic and genome editing technology applied to introduce or modify specific genes liable to stress tolerance (Biswas et al., Citation2019; Blary & Jenczewski, Citation2019). The technology creates crops with enhanced pest and disease resistance including superior heat and drought tolerance. Additionally, conventional breeding used for developing crops with desirable traits such as yield, disease resistance, and quality example is the Marker-assisted selection (MAS) (Dey, Citation2023). The approach is used to develop crops with improved stress tolerance. However, the method maybe time-consuming and unable to keep pace with the rate of environmental change. Lastly, precision agriculture approaches maybe considered using technology such as remote sensing, drones and GPS, whereby farmers can optimize crop management practices such as pest control, irrigation, and fertilizer use (Callaway, Citation2018). This approaches can help farmers to conserve resources, optimize crop yields, and reduce the impact of environmental stress on crops. Regardless of the prospective pay-off of these approaches, there are related challenges which may promote their dis-adoption by small holder farmers in developing countries. These include development of new varieties of crops maybe exorbitantly costly. Fears over the safety and environmental impact of genetic engineering remain a barrier to widespread adoption. Additionally, it is a difficult task to develop crops that are resilient to multiple environmental stresses, as dissimilar stresses may require unlike traits (Dey, Citation2023).
Further, other approaches related to crops that offer scientific solutions are vital in assessing the impact of climate change (Rahman et al., Citation2018; Ullah et al., Citation2019a). Gul et al. (Citation2020) study using CSM-CERES-Wheat model predicted phenology and yield very well for all three wheat cultivars. Results showed that increase of 1 °C caused the 10-day early anthesis reduced the time for grain filling duration, resulting in decline in yield. The increase in yield in the coldest region, thus projected increase in temperature causing a favorable condition for wheat production. Other studies Asseng et al. (Citation2014) and Ahmed et al. (Citation2019) reported that higher yields may be expected where cooler temperatures at grain filling stage of the crop delay the days to maturity. Other approaches according to Sándor et al. (Citation2020) and Liu et al. (Citation2023) modelling waterlogging stress as a function of phenology, was an important development on the majority of past knowledge. This has revealed that effects of waterlogging stress on crop sensitivity is critically reliant on the growing phase in which waterlogging occurs. These deviations propose that more benefits from yield may be realized from forward shifts in sowing time of long-season genotypes, consistent with other studies (Hunt et al., Citation2019). This result may echo the fact that areas with longer growing season are likely to have more frequent soil saturation, greater propensity for extreme rainfall events and/or higher rainfall. Future use of these approaches should be considered in Nyando basin which is prone to frequent flooding and drought spells resulting to emergence of new pests and diseases due to changing climate leading to low yields (Bernier et al., Citation2015; Nyansimi et al., Citation2017; Ruto, Citation2020).
The success of the above stated technologies and innovations are enormous, however, they have hardly been used on a variety of crops and livestock to mitigate the effects of climate change in the study site. Despite the various reasons for dis-adoption decisions by households, the wide-spread adoption rates of CSA practices appear unclear due to little initiative towards understanding the meaning, function and factors encumbering scaling-up. It is important to first understand what scaling up means, which is replication of ideas, plans and practices with an aim of achieving a similar or larger scale impact at the end point (World Bank, Citation2003). The function of up-scaling is to uplift the socio-economic of communities from a small to a wider extent area. According to Linn (Citation2012), World Bank (2015) and Neufeldt et al. (Citation2015) scaling can transpire horizontally, by replicating successful practices in new set up areas or target groups. Vertical scaling up encompasses harmonizing policy and institutional amendment transversely by altering project elements, formation or approach in a rejoinder to a surfacing realism (World Bank, Citation2003). Up-scaling can be effected either directly when an institution is forthwith liable for change, or indirectly when it controls the changes. This paper, presents the CCAFS’s input in conveying CSA technologies and practices to scale using the CSV approach and scaling out in non-CSVs in Kenya’s Nyando basin. It contributes to knowledge by identifying existing gaps hindering a clear scalable approach that is inclusive.
2. Materials and methods
2.1. Research area
This study took place in Nyando basin which traverses through Kisumu and Kericho counties. The villages represent different land uses in the commune, within a similar context to the CSV. This then creates an ideal situation to test the scalable, comprehensive and impartial framework for CSA implentation. The key geographical characteristics for the study sites were dissimilar, such that Kisumu is drier than the Kericho area, but has more water points as it lies along the lowland Nyando basin. Kericho on the other hand is in higher altitude, more remote and less served by transport. It experiences less floods during rainy seasons because of the slope. Agriculture contribution to livelihoods in the area accounts for 95% in incomes and over 80% employment and food from a combination of mixed system namely crop/livestock, cash crops/livestock and or all combined (Godino, Citation2021). Nyando basin is considered 99% cultivable (Ogada et al., Citation2020), however it encounters food shortfall partially due to unreliable rainfall, varying temperatures ranging 9–35 °C (County Government of Kisumu, Citation2013). During the commencement of every rain season soils swell, split and seal rendering water in-ability to further permeate deeper and therefore forces it to overflow as floods to the plain landscape (Murono et al., Citation2018). Run-off develops profound gorges destroying approximately 40% of field crops (Kinyangi et al., Citation2015). Farms are not diverse and exhibit barely any farming modernization (Nyakundi et al., Citation2010; Kinyangi et al., Citation2015). Comparing scaling out CSA among respondents in Nyando CSV with non CSVS, the main difference was observed to be on the resource use by type of agricultural investment. Whereby, in Kisumu relatively larger share of rice was prevalent and while, sugar cane plantations in Kericho was observed. This provides opportunities to develop climate-smart rice and sugar cane models. represents map of the study area.
Figure 1. Location of the study Site. Source: Climate Change Agriculture and Food Security (CCAFS), site of Nyando/KatukuOdeyo, Kenya, in Sijmons et al. (Citation2013).
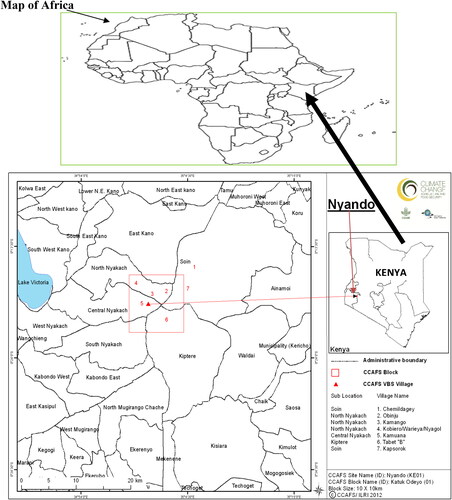
2.2. Data collection, sampling methods, data analysis and limitation of the study
Data was drawn from 2019 to 2020 baseline, end line and financial diaries surveys. A simple random sampling technique was used to select 216 households residing in CSV from the 2017 survey that had been observed by CCAFS during a Monitoring and Evaluation (M&E) routine. To identify the required sample size of 122 it was determined proportionate to methodology by Yamane (Citation1967).
(1)
(1)
Where n is the sample size, N is the population, and e is the level of precision.
(2)
(2)
Further, purposive sampling was used to identify ninety households who comprised members of Community Based Organizations (CBOs) who took part in CCAFS activities (overseen via these CBOs) and thirty two households residing in non-CSV but within the vicinity of the CSVs. The non-CSV respondents assisted to shade light on the CSA horizontal replication/up-scaling in new set up areas or target groups other than in CSV. All sites selected had similar geographical characteristics to the CSVs namely; temperatures, rainfall, soil type, landscape and socio-economic attributes (namely crops planted like maize, sorghum and beans, ownership of livestock and applied husbandry practices, and market activities). STATA 14 data analytical tool was used thus descriptive statistics and regression models results presented in form of tables and figures.
2.2.1. Approach
To determine the CSA dis-adoption trends (2017–2020) for agricultural production systems into climate-smart interventions in Nyando basin, secondary data was extracted from a technical report by Oostendorp et al. (Citation2021) who were part of this study. The analysis was done to confirm the scope to which the practical outline replicated underlying association. To determine the spatial scaling pathways of different systems in the commune, baseline and financial diaries panel data were used. Field observations and participation in group meetings organized by CCAFS shed light on some of the issues presented.
2.2.2. Analytical framework
This study used Engel curves to analyze household head and spouse farm expenditures for adopters and non-adopters of CSA practices. From an economic outlook there exists a bond between farming expenditures and household decisions on adoption of a technology within a constrained household budget. Given that household expenditures are determined by demand, prices per unit and total earnings. Engel curves display the reliance of expenditure (E) on the sum of households’ and spouses’ wages, Yh+Ys, taking prices and other demographic characteristics (Δ) ceteris paribus.
(1)
(1)
EquationEquation (1)(1)
(1) is a restriction of the more general Engel curve
(2)
(2)
EquationEquation (3)(3)
(3) is a general specification of Equationequation (2)
(2)
(2) that can be attributed to any collective model of household behavior and contains a quadratic specification of the Engel curve:
(3)
(3)
Δi, i = 1…., n are demographic variables reported, namely household annual incomes from household head and spouse, access to credit, saving patterns, and household size.
The term e in Equationequation (3)(3)
(3) is a random error term. It was assumed that farm expenditure results from restrictions of Equationequation (2)
(2)
(2) such that βs = βh and βww = βhh = βhw/2. This meant that each income (from household head and spouse) had the same effect on expenditures. EquationEquation (3)
(3)
(3) then was re-written in a restricted manner giving:
(4)
(4)
EquationEquation (4)(4)
(4) in a restricted form indicate that farm expenditures were pooled, on the other hand a rejection of these restrictions suggested that farm expenditures were not pooled.
2.2.3. Limitation of the study
Several limitations were encountered during data collection which included bad weather conditions and poor road infrastructure, enumerators had a challenge of language barrier where respondents not literate could not answer questions correctly. The weekly visits to households for 362 days became too monotonous that some respondents opted out. In some instances respondents found it difficult to disclose income sources, actual amounts, and expenditures.
2.3. Binary regression model on determinants of CSA adoption/dis-adoption
This gave an overview of the nature of variables that influenced adoption of CSA practices. The binary independent variables were then computed to form the overall CSA dichotomous variables, hence, yes (1/adopting) and no (0/not adopting) for the function of the logistic regression. Focus was on farm level economic aspects on incomes and farming expenditures which were analysed against the overall CSA adoption. Important variables considered were socio- economic characteristics (i.e. household size, off-farm incomes and CSA incomes). Further, institutional characteristics namely access to credit, group membership, husbands and wife’s savings were also well thought-out. The variables were computed into the model as follows.
(5)
(5)
Where CSA denotes uptake; (B) CSA budget; (I) lncomes, (SE) Social- economic; IL, Institutional (banks, farmer groups, NGOs, donors, government) and ε the error margin. Variables used in the model are shown in .
Table 1. Descriptive statistics of variables used in the Logit regression model.
3. Results and discussions
3.1. Descriptive statistics
3.1.1. Shocks affecting CSA adoption and farm productivity by respondents
In this study shocks referred to climatic risks caused by unpredictable weather events and other uncertainties that destroy the sustainability and livelihood of a rural household/community. Respondents were asked whether they had experienced a shock within 2 waves (January to June and July to December). Results on indicated that unpredictable emergence of new pests and diseases (in crops and livestock) was noted by (80%) of farmers, little/excess rainfall (66%), sickness and deaths of household members (34%), and increase in input prices (19%) for both adopters and non-adopters. Similarly, high price on farm produce was reported by (59%) of the respondents within CSVs and (45%) in non-CSVs. Weather shocks related to climate variability like droughts, floods and rising temperatures Godino, (Citation2021); Ogallo et al. (Citation2019) are a driver to emergence of new pests, disease infestation and related stress on crops, livestock, as well as farmers and laborers (FAO, Citation2016). These are often unpredictable and result in major losses of produce and death of livestock thus affecting gains achieved in scaling up CSA practices. As a result of this Kenyan farmers lost Ksh 3 billion losses of maize due to army worm infestation in 2017 (De Groote et al., Citation2020). This scenario explains why there has been an improved adoption of pesticides and use of a range of drought tolerant crop varieties. On the other hand, little rainfall leads to stunted growth of crops and fodder leading to low yields, starvation and malnutrition of both people and livestock due to over reliance on rain-fed agriculture. Field observations showed that there was less than (1%) of investment in water conservation and irrigation measures among CSVs and non-CSVs respondents. These observation present similar proof attained in Tanzania and Uganda by (Mwongera et al., Citation2017; Belay et al., Citation2017; and Elum et al., Citation2017).
Figure 3. (a–e) Engel curves on wife and husband household expenditure ratios. Source: Author (2020).

This study found that high food prices in CSV was attributed to high cost of inputs such as; pesticides/fungicides, labor, fertilizer and veterinary drugs which was forcing farmers to sell their produce at a higher cost to recoup their production costs. According to Heeb et al. (Citation2019) the prevalence of pests has escalated leading to losses of up to 100% if not managed due to variation in weather patterns. Additionally, over-use of pesticides and exposure to high temperatures linked to climate change has reduced their effectiveness (Mulwa et al., Citation2017; IPCC, Citation2018; Mujeyi et al., Citation2019). The repercussions of these reflects low productivity and less households’ earnings. This subsequently affects food prices in the markets including other numerous nodes along the food value chain (e.g., storage, food quality, and safety). Likewise, institutional related shocks reported like inputs and high food prices due to market failures as a result to inaccessibility to markets can affect scaling up of CSA practices. This findings agree with FAO (2011) and Barnard et al. (Citation2015), that poor infrastructure to markets may hinder farmers accessing information, business and food losses due to market related drivers. Loss of family members from related stresses, especially those providing cash to invest on CSA may also have affected adoption and scaling up.
3.1.2. Socio-economic activities of CSVs and non-CSVs respondents (T-test results)
Results in showed that slightly more than (41%) of non-CSV farmers engaged in off-farm jobs compared to (39%) of CSA adopters in CSVs. The off-farm activities consisted of small businesses requiring very low capital investments. A major challenge to these start-ups was their survival and take-off which mainly depended on household needs, availability of working capital and business skills. Partaking off-farm jobs was positive and significant at (p < 0.05) compared to households that entirely depended on farming. The extra cash proceeds from off-farm activities may have enabled farmers to purchase the needed inputs and implementation of high investment CSA practices. The finding concur with Ojo and Baiyegunhi (Citation2020), who found out that households earning extra cash from off-farm were more likely to adopt most CSA technologies, especially those which call for huge financial commitment. The findings suggested that off-farm incomes as an alternative source, was likely to heighten use of CSA practices.
Table 2. ‘t-test’ Results on demographics in CSVs and NON-CSVs by respondents.
Additionally, up-take of several CSA technologies within a season favoured farmers with financial ability. However, divergent views were observed by Mathenge et al. (Citation2014) who found that taking up off-farm activities abstracted attention away from farming activities. This is also a true scenario in the villages where women and men took part in agri-business. They exhibit dis-interest in investment on new farm strategies and divert crucial labor from farms to their small businesses.
Results in revealed that saving decisions by CSA adopters had a mean of (70) compared to non-adopters at (50). They saved mainly in Self-help groups (SHGs), CBOs, micro-finance institutions like banks and Kenya Women Finance Trust (KWFT) a women based financial institution. The savings were necessary since they were the basis for ability to borrow loans from the financial institutions. The study found out that many smallholder farmers fail to comply and fall out in this regard. According to Chitakira and Ngcobo (Citation2021), farmers should perhaps acquire affordable credits with negligible interest rates, flexible terms of repayment, insurance, subsidy and waiver during events of poor production due to adverse weather variations like prolonged drought, pests and diseases. There has been an arising awareness on need for future security in form of savings to cushion unforeseen shocks example death of family members, sickness, and weather related risks. According to Sethi (FAO), (Citation2013) this may be an influencing factor that can lead farmers towards tendency to save. The findings on saving decision by farmers may act as a “pull” as an enabler to credit access towards adoption of CSA and should therefore be emphasized.
Table 3. Education level of respondents affecting CSA adoption rates of respondents.
Access to credit was significant (p < 0.05) and had a positive effect on households adoption rates of CSA practices with means of (61) and (51) for adopters and non-adopters respectively. The major informal credit lenders to over 40% of the smallholder were CBOs and SHGs through table banking, family members, friends, and through mobile money platforms. Other sources of credit included formal banks, and micro finance institutions. Loans assists in boosting cash required to facilitate and embark on innovative practices. The study found out that majority of smallholder farmers lack collateral and or savings to enable them access loans. Therefore, CCAFS introduced a kind of (merry-go-round) as an innovative fund in form of table banking to develop a culture of saving, to enable borrowing and investment and thus boost the financial strength of smaller village groups. According to Mulwa et al. (Citation2017) and Wekesa et al. (Citation2018), they found out that lack of financial access may hinder uptake of low or high capital CSA practices. Financial services, policies and government programs should focus specifically on marginalized farmer groups in the poorest income quantile who are often the worst affected by weather pattern changes. Access to credit by farmers through institutions, groups and intermediaries could enhance the “pull” effect to adoption of CSA. Financial literacy and lending in groups will however need to be considered to reduce repayment defaults (Ruben et al., Citation2018; Omore et al., Citation2019).
3.1.3. Education level of respondents affecting knowledge smart scaling pathway
Descriptive statistics on indicate that (28) of household heads had completed primary level with only (27) spouses achievement. At least (13) of the respondents had completed secondary and just (9) of spouses. This implied that higher level of education did not play a significant role in acceptance of CSA. This result implied that since trainings are usually carried out by CBOs to members, in field schools, radio and public gatherings the medium of communication i.e. use of local language was key. This enabled farmers to participate with ease and ask questions in areas not understood. Contrary, Bernier et al. (Citation2015) had earlier found that education level was interrelated with improved up-take of proficient application of fertilizer and high yielding varieties, but with a decline in use of compost and cover crops. Though these practices may be deemed simple their adoption rates varied. The more sophisticated a strategy is, the requirement for technical know- how on its implementation is paramount for example water pans construction, green houses, hydroponics, bio-gas production amongst others. This findings agree with Mujeyi et al. (Citation2019) that higher levels of education increases ability to comprehend, attain and grasp easily new technologies within a short time. This is also in line with results by Gido et al. (Citation2015) who stated that a build on farmers’ innovativeness and improved information access is enhanced by higher levels of education which are vital in the adoption of better farming methods.
According to results by Oostendorp et al. (Citation2021), dis-adoption rates were high in fertilizer use and improved breeds in 2017–2020. On the other hand, there was more adoption of high yielding varieties in 2020. Field observations revealed that majority of farmers with low education level relied more on indigenous knowledge, cultural beliefs and often related conventional knowledge with costs. Therefore, these may have contributed to increased dis-adoption rates in fertilizer and improved breeds adoption. According to Mathews et al. (Citation2018), unreliable weather patterns has disrupted the traditional farming practices, livelihoods and earnings of smallholder communities who practice semi-subsistence agriculture. Encouraging adult education is key as a means to achieving basic literacy amongst small scale farmers. This will inform them on importance of CSA interventions in the wake of climate change and thus a “pull” towards adoption to enhance resilience.
3.1.4. Markets accessibility affecting adoption of CSA practices by respondents
Distance (measured by walking in km) to serviced road, and open agricultural markets were variables considered on participation in CSA practice. Results in show that distance to food market for adopters was found to have a mean of (2.9) and for non-adopters was (3.2) km. Livestock markets seemed to be relatively far with a mean of (8.8) and (8.9) Km for adopters and non-adopters. Kassie et al. (Citation2013) observed that not only does distance affect access to the market, but also the accessibility of new technologies, information and credit institutions thus having a negative correlation. Cooperatives and creation of collecting centers for picking livestock could reduce transportation challenges, middlemen and brokers along the value chain. This finding concur Iskandar and Gatzweiler (Citation2016) and Mujeyi (Citation2019) who found that during rainy seasons rural gravel/murram feeder roads are often impassable resulting to disruption in transport services and costly charges. Good infrastructure by county governments is also necessary in order for produce to reach markets on time and hence reduce on losses from spoilt produce. All these could act as “pull” aspects to enhance farmers to adopt CSA practices for higher yields and income gains where markets are accessible.
Table 4. Distance to various markets.
3.2. Household decision-making mechanisms on CSA adoption and scaling up
3.2.1. Engel curves indicating household decisions on CSA adoption and scaling up expenditures
The budget share Engel curves were used to describe how the proportion of household income spent on various goods varies with income. Engel curves were found to be suitable since they clearly display how real expenditure varies with household income. The study sought to find out whether rural household members cooperated on budget share decisions impacting on CSA adoption and scaling up. Using the local weighted estimate scatter plot (LOWES), results in indicate that spouse/wife’s contribution to scaling up expenses significantly went down as the husband’s income increased. This indicated cooperative decision making between wife and household head/husband in graphs a and b (). Although wife’s contribution to farming activities increased as her income increases, it was only to a certain point as presented in the Engel graph (c). The same trend was observed in graph (b) for wife’s farm expenditure against the total household income. The results are expected since wife may reduce their participation in farm expenditures (CSA) adoption and scaling up and boost utilization of personal and children’s goods (health, clothes, group savings). This leaves farming expenditures to men and their responsibility. The results agree with findings by Bjornlund et al. (Citation2019) who found out that all financial decisions were the responsibility of men in Tanzanian. Men proposed that women were wasteful and often engaged in needless expenses such as buying luxurious items and new clothes. On the other hand, women viewed their expenditures as a need and necessary and often secretly sold crops to attain cash. Graph (e) shows that as household’s welfare improved with more incomes, wife’s contribution to expenditures rose up-to a certain level then it started to decline. These results concludes that cooperative decisions within rural households could boost adoption and scaling up of CSA practices.
3.3. Determinants of adoption/dis-adoption of CSA strategies in Nyando basin
Results in illustrate that important determinants of dis/adoption of CSA practices were household head’s income, household expenditure, household size, and household’s savings patterns. Household head’s cash significantly (p < 0.001) was associated with contribution to farm expenditure. The coefficient was negative (−.227) suggesting that as incomes increased his contributions declined by 22%. Household expenditures significantly (p < 0.001) and positively (0.240) affected contributions to farm expenditures by 24%. This signified that as household’s expenditures increased more resources were required to ease budget constraints on consumption, CSA input costs, school fees, health and thus cooperative decisions were necessary. Household size significantly (p < 0.05) and positively (0.0189) impacted on contribution to farming expenses rising by 18%. The results suggests that a large family demands a higher expenditure on their various needs which may constrain budget against CSA adoption. However, their contribution to farm labour may influence adoption rates. Husband’s savings significantly (p < 0.1) and negatively (−0.09) affected contributions to expenditures by a decline of 9%. Interestingly as his savings increased contribution to farm expenses reduced. The findings suggest that as husband’s savings and incomes increase he shifts focus to large investments like building a house, machinery and even marrying a second wife.
Table 5. Binary Regression model results on determinants of CSA adoption/dis-adoption.
On the other hand, wife’s savings significantly (p < 0.05) and positively (0.107) influenced contributions 10%. This finding put forward the fact that spouse’s savings which often enabled borrowing from SHGs which supported some farm expenses. Spouse’s savings accrued from social-capital endowment (merry-go rounds from groups and financial help- gifts/remittances from relatives, friends) was observed as a livelihood diversification strategy. However, worth to note was the result that CSA adopt was negative (p < −0.0163) and not significant (0.735), suggesting that being an adopter of the practices did not influence adoption rates of households slightly by1%. On the other hand, CSA farm incomes significantly (p < 0.001) and positively (1.566) influenced expenditures thus resulting to adoption of various practices. This informed that, as incomes from CSA practices more investment on farming was feasible which increased adoption rate.
This observation concluded that as benefits from the practices rose, up/out scaling was viable through CSA adoption. As observed by Milder et al. (Citation2011), CSA is normally more lucrative in the long-run in contrast to convectional agriculture. However, achieving the long-term gains necessitate high start-up cash injection, which regularly is unaffordable and uncertain for a majority vulnerable smallholder farmers to embark on (Aggarwal et al., Citation2018). This often is the case especially for farmers that are risk averse in relation to their food security distress. Additionally, according to Hobbs (Citation2007) many farmers earn profits within 1st year of adopting CSA, others do not experience higher yields or success until after 3–7 years. Therefore, due to long time lag farmers may decide to abandon CSA for other alternative income earning activities. Therefore, long term adoption and scaling up is more desirable to most farmers when CSA offers noteworthy gains in the 1st or 2nd year (Reij et al., Citation2009). The deficiency of or scarce financial resources to obtain farm inputs represent a significant hurdle to smallholder farmer adoption of CSA especially if implentation costs are high.
3.4. Pathways to scaling up CSA practices
3.4.1. Integrating CSA adoption in extension services capacity building
Extension services provides capacity building needs to farmers in the form of training and information. However, this was identified as the single major barrier to CSA adoption across all regions in 33 countries in Africa, Asia and Latin America CSA sites (Vernooy & Bouroncle, Citation2019). Results in showed that in CSVs (69%) of the respondents accessed extension services compared to 57% in the non-CSVs. Major roles played by extension agents were creating awareness and demonstrating recent CSA technologies. The main objective being promoting sustainable farming which requires an entire new component of expertise, inspection, observing and risk evaluation. The County governments in the study area offer demand driven extension services. Other extension service providers are One Acre Fund, Kenya Agriculture and Livestock Research Organization (KALRO), CCAFS, (International Livestock Research Institute (ILRI) amongst others. However, it was noted that farmers preferred NGOs and donor agencies’ extension support than from the county government’s staff. Mulwa et al. (Citation2017) and Maguza-Tembo et al. (Citation2017) concluded that many contacts with extension service agents enhances indigenous skills, knowledge which encourages uptake of a range of farm technologies with ease. This concurs with observations by Eshetu et al. (Citation2020) that, farmers’ actions while implementing various practices, maybe insufficient without government extension services in order to merge both adaptive knowhow and technical support. A major inhibiting factor in extension service delivery in Kenya is that it is a function devolved to the 47 counties under (2010 constitution) and its effectiveness has been declining due to lack of field officers, and lack of commitment by government. This has resulted to Kenya rated as an importer of food (GoK, 2023). Heightened campaigns through extension service should be undertaken as a matter of urgency to counter high dis-adoption rates across almost all CSA practices as a “pull” mechanism to promote their scaling.
Table 6. Access to extension services (% of respondents).
3.4.2. Integrating CSA adoption to group membership and welfare of respondents
As indicated on group membership (pooled) was (46.36)% for the household head while 50% for the spouse. Mixed groups were preferred at (82.35) and (70.91)% for household head and spouse respectively. The study findings suggested that farmers who participated in collective action (i.e. through farmer groups/organizations), had a higher probability to adopt CSA practices, such as use of high yielding and drought resistant crop varieties, pesticide/herbicides application against pests. The results agree with Ngigi et al. (Citation2017), that group-based strategies and involvement promoted execution of CSA practices.
Table 7. Group membership of respondents.
In the study area CSA is advocated and promoted by CBOs whose highest membership percentage and leadership positions are occupied by women. Nichols (Citation2021), adds that there is heightened deployment of women-led self-help groups (SHGs) as an avenue for conveying development projects by donor agencies and non-governmental organizations. Nonetheless, presently very negligible thoughtfulness about SGHs exists on how effective or if they are equitable platforms for delivering CSA and livelihoods intercessions.
These associations offer linkages to credit and extension services providers which are important elements for adoption of CSA technologies. The findings agree Bizikova et al. (Citation2020, that farmer organizations enables members to get farming skills, climate information, and market linkages as a means to alleviate resilience to cope with climate change. Groups also offer interactions, exchange of ideas, farm demonstrations and trainings including dissemination of important research findings. Gido et al. (Citation2015) highlighted that groups ease effort by extension agents to access group members which lowers cost of service delivery. This ensures economies of scale by ensuring many contacts with members are achieved per learning session. Further, from the findings youths seem not to be interested in group membership and thus the laxity in engaging in farming activities. This, therefore hampers on the “pull” of youths in learning through interaction in groups for adoption of CSA. For adult group members within communities learning interface through extension agents is made favorable. Groups promote active participation in activities via ideal communication styles using local languages, simple illustrations and learning by action (demonstrations), this making the learning sessions a welfare activity thus a “pull” factor to farmers to upscale CSA.
In findings indicate that (40)% of respondents were affiliated to independent groups while (36%) to CBOs. Church groups also seem to be important with (10.9)% of respondents being members. In a research by Nichols (Citation2021), he stated that group gatherings are avenues where women partake formal procedures (such as opening hyms/prayer, deposit cash, loan repayment and requests) and explicitly discuss pertinent agendas. Social capital is supposedly fortified through the habitual of these meetings, and a cooperative is created that is pro-active in securing other benefits for members. As a guide to SHG-routed development, participation based on learning intercessions may have positive implications on CSA adoption rather than credit/loans or handouts access which may be less feasible. Work burdens and illiteracy levels often constrains involvement, thus these may hinder the key goal of SHGs as an empowerment tool for women. In order to “pull” farmers and permit them to implement CSA practices, caution should be observed not to create undue burden on group member’s time in the name of SHG activities. More emphasis should focus on investment on basic literacy provided by adult education programmes.
3.4.3. Integrating CSA to key commodity value chains in the study area
Observations in Sub-Sahara Africa Totin et al. (Citation2018), show that institutions in particular private sector support for agricultural development and for CSA technologies (e.g. markets) is weak and often regarded as a bad business practice. Nonetheless, resilient public–private partnerships seem to be an encouraging alternative for upscaling CSA technologies to create business opportunities. On the perspective of the project, the information in was gathered from field observations and attendance of group meetings. This study found poor market environment and limited value addition of farm produce, as the most critical challenges inhibiting better prices for actors especially the producers in the area. This is in agreement with Kirui et al. (Citation2018), who concluded that many farmers in rural Kenya are inhibited by poor marketing facilities characterized by high cost of transportation, poor storage, and massive waste and losses.
Various actors performed different functions which comprised of; input supplying, production, collecting/transporting, merchandising, wholesaling and retailing to various consumers. Small-scale farmers directly supplied/sold at farm level to (rural) road side traders or at the local markets, devoid of association with co-operatives or Ministry of Agriculture, livestock and Irrigation (MoAL&I). At the farm gate level outputs were traded through barter trade or via cash or mobile money transaction, whilst the remaining produce was sold at the roadsides. The results further revealed that other producers directly supplied local rural retailers/shops who bulked and sold directly to traders within and beyond the vicinity.
Additionally, enablers were actors identified in the value chain process under the umbrella of institutions/organizations and cooperatives who were not part of the CSA project. Their mandate is to oversee farmers’ progression and their activities run proficiently. They provided trainings, credit facilitation, market information and projects facilitation. These enablers included sugar and rice boards, Ministry of Agricultural extension Officers, (Kenya Agriculture and Livestock Research Organization (KALRO) field staffs and CCAFS project staffs. The boards majorly, performed the task of linking farmers with the consumers and national markets in the major cities of the country. Intermediaries namely middlemen, brokers and transporters also participated as enablers. Meanwhile, the extension officers performed the role of providing technical skills and knowledge to producers along the value chain process.
The evaluation from field observations was unable to further analyze the flow of the farm produce along the value chain map owing to lack of data on the actual quantities transacted in each node of the value chain. The assessment further noted that apart from sugarcane, rice and maize, very little of other food produce was exported to other regional markets and neighboring country (Uganda). Food scarcities and low fertilizer use impacted on low productivity which informed why respondents were a net importer of food from other counties. Key to note was the potential linkages that were lacking namely processors, farmers and traders to farmer/trade groups or co-operatives. The farmer cooperatives could be avenues for farmers to access loans, subsidized inputs, market information and export markets. The commercialization/market based pathway is majorly a “pull approach” to scaling. This uses monetary based inducements and market-driven approaches to “pull” farmers into implementing the CSA technologies by using contract farming where private organizations and institutions are involved. Providing crop insurance and credit too may permit farmers to be well set to implement new technologies (Bui & Vu, Citation2020; Reyes et al., Citation2015). These guarantees markets for their outputs assurance however, processing and value addition were observed as prevailing gaps/aspects requiring urgent consideration.
Westermann et al. (Citation2015), in a CCAFS working paper reiterates that value chains can link many actors around a common objective. They can create a conducive environment for exchange of knowledge, discussion, capacity building and create negotiation capacities. Mapping value chain dynamics, especially for production-oriented agricultural technologies, can highlight the scope and prospects for diversification or added value and create interest of players who build market demand (Neufeldt et al., Citation2015). Value chains can also be used by government and private entities to deliver subsidy programmes, credit and extension services ().
4. Conclusion and policy implications
The adverse effects of changing weather patterns have been exhibited in Sub-Saharan Africa leading smallholder farmers to abandon farming activities and turn to other off-farm diversification strategies. This has led to decreased production and challenges in scaling-up of technologies within and outside the scope area. Study findings indicate that some of determinants affecting scaling up adopted CSA practices were prevalence of pests and diseases, high food and input prices. On socio-economic aspects off-farm incomes was found to be significant and positive especially in the non-CSVs. Education level did not play a key role in CSA adoption, however, indigenous knowledge, cultural beliefs and technical knowledge access had an effect on dis-adoption rates. There was gender equity and pooling of resources in the CSVs which could contribute to pathway to scaling-up. Likewise, farmers in the CSVs had more contacts with extension agents thus encouraging knowledge smartness on CSA practices. On market value chains aspects, actors and agents seem not to exhibit collaboration and information sharing was absent. The market environment was not attractive with poor road networks, scarce market infrastructures like buildings/sheds and poor sanitation. Majority of the small-holder farmers preferred selling goods at farm gate or at road sides. The enablers in the value chain like government officers, financial institutions and NGOs were absent.
The study recommends agronomists and breeders to consider agronomic biofortication technologies under climate change and test under different geographical localities in the near future. Most convectional breeding approaches are time consuming and breeders have to wait for a long time for results. Therefore use of Omics could offer many advantages over traditional breeding at crop level and artificial insemination for livestock as best options in future.
Environmental hazards can be limited by application of quantitative framework that creates a platform to assess location-specific vulnerability. However, to exploit this framework, policy makers should choose their most favorable approaches in policy formulation. Financial institutions should come up with favorable credit facilities to assist farmers continue adopting CSA technologies and practices and up-scale. Smart marketing strategies should be implemented such that there is free flow of information, value chain actors’ collaboration, good infrastructure and value addition to act as pull factors to farmers to increase food production. In this entire scenario, there is need to apply and test this study’s findings and knowledge on similar site specific regions and countries. This may input a comprehensive policy approaches to guide farmers on importance and need to up-scaling CSA in order to overcome food production challenges due to changing weather patterns encountered by most countries now and in future.
6. Area for further research
More research should be conducted to test modern approaches and frameworks at site specific locations, to evaluate and advice policy on best strategies to apply under current climate change challenges.
Acknowledgements
Appreciation and thanks goes to the respondents interrogated and the enumerators who lent a hand in data collection. Thanks to CCAFS, ILRI, NWO, VI agro and other stakeholders for allowing use of their study site in Nyando basin. Gratitude to all the community leaders, who led the enumerators in locating the respondents for this study.
Disclosure statement
No potential conflict of interest was reported by the author(s).
Additional information
Notes on contributors
Josephine W. Njogu
Josephine W. Njogu works with the Ministry of Agriculture and Livestock Development, State Department for Livestock Development.
George Karuku
George Karuku is a Professor at the University of Nairobi, College of Agriculture and Veterinary sciences. Department of Land Resource Management Agricultural Technologies.
John Busienei
John Busienei is a senior lecturer at the University of Nairobi, College of Agriculture and Veterinary Sciences, Department of Agricultural Economics.
John Kamau Gathiaka
John Kamau Gathiaka is a senior lecturer at the University of Nairobi, College of Business and Economics Studies.
References
- Aggarwal, P. K., Jarvis, A., Campbell, B. M., Zougmoré, R. B., Khatri-Chhetri, A., Vermeulen, S. J., Loboguerrero, A. M., Sebastian, L. S., Kinyangi, J., Bonilla-Findji, O., Radeny, M., Recha, J., Martinez-Baron, D., Ramirez-Villegas, J., Huyer, S., Thornton, P., Wollenberg, E., Hansen, J., Alvarez-Toro, P., … Yen, B. T. (2018). The Climate-Smart Village approach: Framework of an integrative strategy for scaling up adaptation options in agriculture. Ecology and Society, 23(1), 1. https://doi.org/10.5751/ES-09844-230114
- Ahmed, I., Ullah, A., Rahman, M. H., Ahmad, B., Wajid, S. A., Ahmad, A., & Ahmed, S. (2019). Climate change impacts and adaptation strategies for agronomic crops. In Climate change and agriculture (pp 1–20). IntechOpen.
- Amanullah, S., Khalid, Imran, H., A., Khan, M., Arif, A., Rahman, A., Muhammad, A., Shah Faha, A., Shah., & B., Parmar. (2020). Effects of Climate Change on Irrigation water quality. Department of Agronomy, The University of Agriculture Peshawar.
- Andati, P., Majiwa, E., Ngigi, M., Mbeche, R., & Ateka, J. (2022). Determinants of adoption of climate smart agricultural technologies among potato farmers in Kenya: Does entrepreneurial orientation play a role? Sustainable Technology and Entrepreneurship, 1(2), 100017. https://doi.org/10.1016/j.stae.2022.100017
- Aryal, J. P., Sapkota, T. B., Rahut, D. B., & Jat, M. (2020). Agricultural sustainability under emerging climatic variability: The role of climate-smart agriculture and relevant policies in India. International Journal of Innovation and Sustainable Development, 14(2), 219–245. https://doi.org/10.1504/IJISD.2020.106243
- Asseng, S., Ewert, F., Martre, P., Rötter, R. P., Lobell, D. B., Cammarano, D., Kimball, B. A., Ottman, M. J., Wall, G. W., White, J. W., Reynolds, M. P., Alderman, P. D., Prasad, P. V. V., Aggarwal, P. K., Anothai, J., Basso, B., Biernath, C., Challinor, A. J., De Sanctis, G., … Zhu, Y. (2014). Rising temperatures reduce global wheat production. Nature Climate Change, 5(2), 143–147. https://doi.org/10.1038/nclimate2470
- Autio, A., Johansson, T., Motaroki, L., Minoia, P., & Pellikka, P. (2021). Constraints for adopting climate-smart agricultural practices among smallholder farmers in Southeast Kenya. Agricultural Systems, 194, 103284.
- Barnard, J., Manyire, H., Tambi, E., & Bangali, S. (2015). Barriers to scaling up/out climate smart agriculture and strategies to enhance adoption in Africa Forum for Agricultural Research in Africa. FARA.
- Barzola Iza, C. L., & Dentoni, D. (2020). How entrepreneurial orientation drives farmers’ innovation differential in Ugandan coffee multi-stakeholder platforms. Journal of Agribusiness in Developing and Emerging Economies, 10(5), 629–650. https://doi.org/10.1108/JADEE-01-2020-0007
- Bayala, J., Ky-Dembele, C., Dayamba, S. D., Somda, J., Ouédraogo, M., Diakite, A., Chabi, A., Alhassane, A., Bationo, A. B., Buah, S. S. J., Sanogo, D., Tougiani, A., Traore, K., Zougmoré, R. B., & Rosenstock, T. S. (2021). Multi-actors’ co-implementation of climate-smart village approach inWest Africa: achievements and lessons learnt. Frontiers in Sustainable Food Systems, 5, 120. https://doi.org/10.3389/fsufs.2021.637007
- Belay, A., Recha, J. W., Woldeamanuel, T., & Morton, J. F. (2017). Smallholder farmers’ adaptation to climate change and determinants of their adaptation decisions in the Central Rift Valley of Ethiopia. Agriculture & Food Security, 6(1), 1–13. https://doi.org/10.1186/s40066-017-0100-1
- Bell, L. W., Harrison, M. T., & Kirkegaard, J. A. (2015). Dual-purpose cropping-capitalising on potential grain crop grazing to enhancemixedfarming profitability. Crop and Pasture Science, 66(4), I–IV. https://doi.org/10.1071/CPv66n4_FO
- Bernier, Q., Meinzen-Dick, R., Kristjanson, P., Haglund, E., Kovarik, C., Bryan, E., Ringler, C., & Silvestri, S. (2015). Gender and institutional aspects of climate-smart agricultural practices: Evidence from Kenya. CCAFS Working Paper No. 79. CGIAR Research Program on Climate Change, Agriculture and Food Security (CCAFS). Available online at: www.ccafs.cgiar.org
- Biswas, S., Li, R., Yuan, Z., Zhang, D., Zhao, X., & Shi, J. (2019). Development of methods for effective identification of CRISPR/Cas9-induced indels in rice. Plant Cell Reports, 38(4), 503–510. https://doi.org/10.1007/s00299-019-02392-3
- Bizikova, L., Nkonya, E., Minah, M., Hanisch, M., Turaga, R. M. R., Speranza, C. I., Karthikeyan, M., Tang, L., Ghezzi-Kopel, K., Kelly, J., Celestin, A. C., & Timmers, B. (2020). A scoping review of the contributions of farmers organizations to smallholder agriculture. Nature Food, 1(10), 620–630. https://doi.org/10.1038/s43016-020-00164-x
- Bjornlund, H., Zuo, A., Wheeler, S. A., Parry, K., Pittock, J., Mdemu, M., & Moyo, M. (2019). The dynamics of the relationship between household decision-making and farm household income in small-scale irrigation schemes in southern Africa. Agricultural Water Management, 213, 135–145. https://doi.org/10.1016/j.agwat.2018.10.002
- Blary, A., & Jenczewski, E. (2019). Manipulation of crossover frequency and distribution for plant Breeding. TAG. Theoretical and Applied Genetics. Theoretische und angewandte Genetik, 132(3), 575–592. https://doi.org/10.1007/s00122-018-3240-1
- Bui, L. V., & Vu, T. B. (2020). A systematic review of Climate-Smart Agriculture (CSA) practices and recommendations for adoption in the implementation of Nong Thon Moi in the 2021–2030 Strategy. Climate Change and Sustainable Agricultural Development, Special Issue of November 2020, 154–166. in Vietnamese. English available at: https://cgspace.cgiar.org/handle/10568/111530
- Callaway, E. (2018). CRISPR plants now subject to tough GM laws in European Union. Nature, 560(7716), 16–16. https://doi.org/10.1038/d41586-018-05814-6
- CCAFS (2016). Climate-smart villages. An AR4D approach to scale up climate- smart agriculture. CGIAR Research Program on Climate Change, Agriculture and Food Security (CCAFS).
- Chitakira, M., & Ngcobo, N. Z. P. (2021). Uptake of climate smart agriculture in peri-urban areas of South Africa’s economic hub requires up-scaling. Frontiers in Sustainable Food Systems, 5, 706738. https://doi.org/10.3389/fsufs.2021.706738
- County Government. (2013). First county integrated development Plan 2013–2017. County Government of Kisumu.
- Descheemaeker, K., Oosting, S. J., Homann-Kee Tui, S., Masikati, P., Falconnier, G. N., & Giller, K. E. (2016). Climate change adaptation and mitigation in smallholder crop–livestock systems in sub-Saharan Africa: A call for integrated impact assessments. Regional Environmental Change, 16(8), 2331–2343.
- Dey, R. K. (2023). Advances and challenges in developing climate-resilient crops: A review. Advances in Crop Science and Technology, 11, 582.
- De Groote, H., Kimenju, S. C., Munyua, B., Palmas, S., Kassie, M., & Bruce, A. (2020). Spread and impact of fall armyworm (Spodoptera frugiperda J.E Smith) in maize production areas of Kenya. Agriculture, Ecosystems & Environment, 292, 106804. https://doi.org/10.1016/jagee.2019.106804.
- Dhungana, N., Silwal, N., Upadhaya, S., Khadka, C., Regmi, S. K., Joshi, D., & Adhikari, S. (2020). Rural coping and adaptation strategies for climate change by Himalayan communities in Nepal. Journal of Mountain Science, 17(6), 1462–1474. https://doi.org/10.1007/s11629-019-5616-3
- Elum, Z. A., Modise, D. M., & Marr, A. (2017). Farmer’s perception of climate change and responsive strategies in three selected provinces of South Africa. Climate Risk Management, 16, 246–257. https://doi.org/10.1016/j.crm.2016.11.001
- Eshetu, G., Johansson, T., Garedew, W., & Yisahak, T. (2020). Determinants of smallholder farmers’ adaptation options to climate change in a coffee-based farming system of Southwest Ethiopia. Climate and Development, 13(4), 318–325. https://doi.org/10.1080/17565529.2020.1772706
- Fahad, S., Muhammad, Z. I., Abdul, K., Ihsanullah, D., Saud, S., Saleh, A., Wajid, N., Muhammad, A., Imtiaz, A. K., Chao, W., Depeng, W., & Jianliang, H. (2018). Consequences of high temperature under changing climate optima for rice pollen characteristics-concepts and perspectives. Archives of Agronomy and Soil Science. 64(11), 1473–1488. https://doi.org/10.1080/03650340.2018.1443213
- Fahad, S., Rehman, A., Shahzad, B., Tanveer, M., Saud, S., Kamran, M., Ihtisham, M., Khan, S. U., Turan, V., & Rahman, M. H. U. (2019a). Rice responses and tolerance to metal/metalloid toxicity. In M. Hasanuzzaman, M. Fujita, K. Nahar, & J. K. Biswas (Eds.), Advances in rice research for abiotic stress tolerance (pp. 299–312). Woodhead Publ Ltd.
- Food and Agriculture Organization (FAO). (2013). Climate-smart agriculture sourcebook. Food and Agriculture Organization of the United Nations. www.fao.org/docrep/018/i3325e/i3325e.pdf
- Food and Agriculture Organization (FAO). (2016). The state of food and agriculture: Climate change, agriculture, and food security. Food and Agriculture Organization of the United States.
- Gido, E. O., Sibiko, K. W., Ayuya, O. I., & Mwangi, J. K. (2015). Demand for agricultural extension services among small-scale maize farmers: micro-level evidence from Kenya. The Journal of Agricultural Education and Extension, 21(2), 177–192. [Google Scholar] https://doi.org/10.1080/1389224X.2013.872045
- Godino, M. M. (2021). Assessing psycho-social, socioeconomic and institutional characteristics that influence adoption of climate smart agriculture in Taita Taveta County, Kenya [Doctoral dissertation]. Strathmore University.
- Gul, F., Ahmed, I., Ashfaq, M., Jan, D., Fahad, S., Li, X., … & Shah, S. A. (2020). Use of crop growth model to simulate the impact of climate change on yield of various wheat cultivars under different agro-environmental conditions in Khyber Pakhtunkhwa, Pakistan. Arabian Journal of Geosciences, 13, 1–14.
- Gunjal, K. (2016). Agricultural risk management tools resource for the e-learning curriculum course on “agricultural risk assessment and management for food security in developing countries”. 25(3/4), 236. https://doi.org/10.1504/IJRAM.2022.130527
- Harrison, M. T., Cullen, B. R., Mayberry, D. E., Cowie, A. L., Bilotto, F., Badgery, W. B., Liu, K., Davison, T., Christie, K. M., Muleke, A., & Eckard, R. J. (2021). Carbonmyopia: The urgent need for integrated social, economic and environmental action in the livestock sector. Global Change Biology, 27(22), 5726–5761. https://doi.org/10.1111/gcb.15816
- Heeb, L., Jenner, E., & Cock, M. J. W. (2019). Climate-smart pest management: Building resilience of farms and landscapes to changing pest threats. Journal of Pest Science, 92(3), 951–969. https://doi.org/10.1007/s10340-019-01083-y
- Hobbs, P. R. (2007). Conservation agriculture: What is it and why is it important for future sustainable food production? The Journal of Agricultural Science, 145(02), 127–137. https://doi.org/10.1017/S0021859607006892
- Hunt, J. R., Lilley, J. M., Trevaskis, B., Flohr, B. M., Peake, A., Fletcher, A., Zwart, A. B., Gobbett, D., & Kirkegaard, J. A. (2019). Early sowing systems can boost Australian wheat yields despite recent climate change. Nature Climate Change, 9(3), 244–247. https://doi.org/10.1038/s41558-019-0417-9
- IPCC. (2018). Global Warming of 1.5 °C. An IPCC Special Report on the impacts of global warming of 1.5 °C above pre-industrial levels and related global greenhouse gas emission pathways, in the context of strengthening the global response to the threat of climate change. IPCC.
- Iskandar, D. D., & Gatzweiler, F. W. (2016). An optimization model for technology adoption of marginalized smallholders. In F. W. Gatzweiler & J. V. Braunvon (Eds.), Technological and institutional innovations for marginalized smallholders in agricultural development. Springer.
- Kangogo, D., Dentoni, D., & Bijman, J. (2021). Adoption of climate-smart agriculture among smallholder farmers: Does farmer entrepreneurship matter? Land Use Policy, 109, 105666. https://doi.org/10.1016/j.landusepol.2021.10566
- Kassie, M., Jaleta, M., Shiferaw, B., Mmbando, F., & Mekuria, M. (2013). Adoption of interrelated sustainable agricultural practices in smallholder system: Evidence from rural Tanzania. Technological Forecasting and Social Change, 80(3), 525–540. https://doi.org/10.1016/j.techfore.2012.08.007
- Kinyangi, J., Recha, J., Kimeli, P., & Atakos, V. (2015). “Climate - smart villages and the hope of food security in Kenya”. Preliminary results from climate change adaptation and mitigation initiatives in Nyando climate-smart villages. CGIAR Research Program on Climate Change, Agriculture and Food Security (CCAFS).
- Kirina, T., Groot, A., Shilomboleni, H., Ludwig, F., & Demissie, T. (2022). Scaling climate smart agriculture in East Africa: Experiences and lessons. Agronomy, 12(4), 820. https://doi.org/10.3390/agronomy12040820
- Kirui, V., Kisang, A., & Kiptum, E. (2018). Investigating farmer’s participation in value–added activities in rural Kenya: Case of Kapkamak-Kabonon irrigation project in arror ward, Elgeyo Marakwet County. Journal of Small Business and Entrepreneurship Development, 6(1), 59–66. https://doi.org/10.15640/jsbed.v5n2a6
- Kurgat, B. K., Lamanna, C., Kimaro, A., Namoi, N., Manda, L., & Rosenstock, T. S. (2020). Adoption of Climate-Smart Agriculture Technologies in.
- Linn, J. F. (Ed.), (2012). Scaling up in agriculture, rural development, and nutrition | IFPRI. http://www.ifpri.org/publication/scaling-agriculture-rural-development-and-nutrition
- Liu, K., Harrison, M. T., Yan, H., Liu, D. L., Meinke, H., Hoogenboom, G., Wang, B., Peng, B., Guan, K., Jaegermeyr, J., Wang, E., Zhang, F., Yin, X., Archontoulis, S., Nie, L., Badea, A., Man, J., Wallach, D., Zhao, J., … Zhou, M. (2023). Silver lining to a climate crisis in multiple prospects for alleviating crop waterlogging under future climates. Nature Communications, 14(1), 765. https://doi.org/10.1038/s41467-023-36129-4
- Maguza-Tembo, F., Abdi-Khalil, E., & Mangisoni, J. (2017). Determinants of climate smart agriculture technology adoption in the drought prone districts of Malawi using a multivariate probit analysis Lilongwe University of Agriculture and Natural Resources, Malawi. Asian Journal of Agricultural Extension, Economics & Sociology, 16(3), 1–12. https://doi.org/10.9734/AJAEES/2017/32489
- Makate, C. (2019). Effective scaling of climate smart agriculture innovations in African smallholder agriculture: A review of approaches, policy and institutional strategy needs. Environ. Sci. Policy,.96, 37–51.
- Makate, C., Wang, R., Makate, M., & Mango, N. (2016). Crop diversification and livelihoods of smallholder farmers in Zimbabwe: adaptive management for environmental change. SpringerPlus, 5(1), 1135. https://doi.org/10.1186/s40064-016-2802-4
- Mathenge, M. K., Smale, M., & Olwande, J. (2014). The impacts of hybrid maize seed on the welfare of farming households in Kenya. Food Policy, 44, 262–271. https://doi.org/10.1007/978-3-319-92798-5_14
- Mathews, J. A., Kruger, L., & Wentink, G. J. (2018). Climate-smart agriculture for sustainable agricultural sectors: The case of Mooifontein. Jàmbá: Journal of Disaster Risk Studies, 10(1), 1–10. https://doi.org/10.4102/jamba.v10i1.492
- Martinez-Baron, D., Orjuela, G., Renzoni, G., Loboguerrero Rodríguez, A. M., & Prager, S. D. (2018). Small-scale farmers in a 1.5 °C future: The importance of local social dynamics as an enabling factor for implementation and scaling of climate-smart agriculture. Current Opinion in Environmental Sustainability, 31, 112–119. https://doi.org/10.1016/j.cosust.2018.02.013
- Milder, J. C., Majanen, T., & Scherr, S. J. (2011). Performance and potential of conservation agriculture for climate change adaptation and mitigation in sub‐Saharan Africa. Ecoagriculture Discussion Paper No. 6. EcoAgriculture Partners, Washington, DC.
- MoALF. (2016). Climate risk profile for Taita Taveta: Kenya County climate risk profile series (Nairobi).
- Mulwa, C., Marenya, P., Rahut, D. B., & Kassie, M. (2017). Response to climate risks among smallholder farmers in Malawi: A multivariate probit assessment of the role of information, household demographics, and farm characteristics. Climate Risk Management, 16, 208–221. https://doi.org/10.1016/j.crm.2017.01.002
- Mujeyi, A., Mudhara, M., & Mutenje, M. J. (2019). Adoption determinants of multiple climate smart agricultural technologies in Zimbabwe: Considerations for scaling-up and out. African Journal of Science, Technology, Innovation and Development, 12(6), 735–746.
- Murono, D. A., Wabuyele, E. M. I. L. Y., Muoria, P. K., & Abuto, J. O. (2018). Distribution of Parthenium hysterophorus Linn and its Impacts on Biodiversity in Nyando Sub-County, Kisumu County, Kenya. Eur Acad Res, 6, 1599–1621.
- Mwongera, C., Shikuku, K. M., Twyman, J., Läderach, P., Ampaire, E., Van Asten, P., Twomlow, S., & Winowiecki, L. A. (2017). Climate smart agriculture rapid appraisal (CSA-RA): A tool for prioritizing context-specific climate smart agriculture technologies. Agricultural Systems. 151, 192–203. https://doi.org/10.1016/j.agsy.2016.05.009
- Neufeldt, H., Negra, C., Hancock, J., Foster, K., Nayak, D., & Singh, P. (2015). Scaling up climate-smart agriculture: Lessons learned from South Asia and pathways for success. ICRAF Working Paper No. 209. World Agroforestry Centre. https://doi.org/10.5716/WP15720.PDF
- Ngigi, M. W., Mueller, U., & Birner, R. (2017). Gender differences in climate change adaptation strategies and participation in group-based approaches: An intrahousehold analysis from rural Kenya. Ecological Economics, 138, 99–108. https://doi.org/10.1016/j.ecolecon.2017.03.019
- Nichols, C. (2021). Self-help groups as platforms for development: The role of social capital University of Iowa. World Development, 146, 105575.
- Njuguna, J. W. (2020). Factors affecting the adoption of climate smart agricultural practices among smallholder farmers in Bungoma County, Kenya [MSc thesis]. Moi University.
- Nyakundi, H., Mogere, S., Mwanzo, I., & Yitambe, A. (2010). Community perceptions and response to flood risks in Nyando District, Western Kenya. Journal of Disaster Risk Studies, 3(1).
- Nyasimi, M., Kimeli, P., Sayula, G., Radeny, M., Kinyangi, J., & Mungai, C. (2017). Adoption and dissemination pathways for climate-smart agriculture technologies and practices for climate-resilient livelihoods in Lushoto, Northeast Tanzania. Climate, 5(3), 63. https://doi.org/10.3390/cli5030063
- Ogada, M. J., Raob, E. J. O., Radenyc, M., Rechac, J. W., & Solomon, D. (2020). Climate-smart agriculture, household income and asset accumulation among smallholder farmers in the Nyando basin of Kenya. World Development Perspectives, 18, 100203.
- Ogallo, L., Omay, P., Kabaka, G., & Lutta, I. (2019). Report on historical climate baseline statistics for Taita Taveta, Kenya. (Vol. 1). IGAD Climate Prediction and Application Centre.
- Ojo, T. O., & Baiyegunhi, L. J. (2020). Impact of climate change adaptation strategies on rice productivity in South-west, Nigeria: An endogeneity corrected stochastic frontier model. The Science of the Total Environment, 745, 141151. https://doi.org/10.1016/j.scitotenv.2020.141151
- Omore, A., Kidoido, M., Twine, E., Kurwijila, L., O’Flynn, M., & Githinji, J. (2019). Using “theory of change” to improve agricultural research: recent experience from Tanzania. Development in Practice, 29(7), 898–911. https://doi.org/10.1080/09614524.2019.1641182
- Oostendorp, R., Asseldonk, M. v., Gathiaka, J., Mulwa, R., Radeny, M., Recha, J. W., Wattel, C., & Wesenbeeck, L. V. (2021). Adoption of CSA practices in Nyando Basin, Western Kenya. CCAFS Technical Report. CGIAR Research Program on Climate Change, Agriculture and Food Security (CCAFS).
- Rahman, M. H. u., Ahmad, A., Wang, X., Wajid, A., Nasim, W., Hussain, M., Ahmad, B., Ahmad, I., Ali, Z., Ishaque, W., Awais, M., Shelia, V., Ahmad, S., Fahd, S., Alam, M., Ullah, H., & Hoogenboom, G. (2018). Multi-model projections of future climate and climate change impacts uncertainty assessment for cotton production in Pakistan. Agricultural and Forest Meteorology. 253–254, 94–113. https://doi.org/10.1016/j.agrformet.2018.02.008
- Reij, C., Tappan, G., & Smale, M. (2009). Re-greening the sahel: Farmer-led innovation in Burkina Faso and Niger. In D. Spielman & R. Pandya-Lorch (Eds.), Millions fed: Proven successes in agricultural development. IFPRI.
- Reyes, C. M., Mina, C. D., Gloria, R. A. B., & Mercado, S. J. P. (2015). Review of design and implementation of the agricultural insurance programs of the Philippine Crop Insurance Corporation (PCIC). Discussion Paper Series No. 2015-07. Philippine Institute for Development Studies. https://pidswebs.pids.gov.ph/CDN/PUBLICATIONS/pidsdps1507_rev2.pdf
- Ruben, R., Verhagen, J., & Plaisier, C. (2018). The challenge of food systems research: What Difference does it make? Sustainability, 11(1), 171. https://doi.org/10.3390/su11010171S
- Ruto, V. K. (2020). Shocks and coping mechanisms in climate-smart villages of the Nyando Basin, Kenya: Findings from a climate-smart agriculture (CSA) survey in Nyando Basin, Kenya. CCAFS Info Note. CGIAR Research Program on Climate Change, Agriculture and Food Security (CCAFS).
- Saj, S., Torquebiau, E., Hainzelin, E., Pages, J., & Maraux, F. (2017). The way forward: An agro-ecological perspective for Climate-Smart Agriculture. Agriculture Ecosystems and Environment, 250, 20–24. https://doi.org/10.1016/j.agee.2017.09.003
- Sándor, R., Ehrhardt, F., Grace, P., Recous, S., Smith, P., Snow, V., Soussana, J.-F., Basso, B., Bhatia, A., Brilli, L., Doltra, J., Dorich, C. D., Doro, L., Fitton, N., Grant, B., Harrison, M. T., Kirschbaum, M. U. F., Klumpp, K., Laville, P., … Bellocchi, G. (2020). Ensemble modelling of carbon fluxes in grasslands and croplands. Field Crops Research, 252, 107791. https://doi.org/10.1016/j.fcr.2020.107791
- Scherr, S. J., Shames, S., Friedman, R., Beddington, J., Asaduzzaman, M., Fernandez, A., Clark, M., Guillou, M., Jahn, M., & Erda, L. (2012). From climate-smart agriculture to climate-smart landscapes. Agriculture & Food Security, 1, 12.
- Schut, M., Leeuwis, C., & Thiele, G. (2020). Science of Scaling: Understanding and guiding the scaling of innovation for societal outcomes. Agricultural Systems, 184, 102908.
- Shirsath, P. B., Aggarwal, P. K., Thornton, P. K., & Dunnett, A. (2017). Prioritizing climate-smart agricultural land use options at a regional scale. Agricultural Systems, 151, 174–183.
- Sijmons, K., Kiplimo, J., Forck, W., Thornton, P. K., Radeny, M., & Kinyangi, J. (2013). CCAFS site Atlas-Nyando/Katuku Odeyo. CCAFS sitematlas series. The CGIAR Research Program on Climate Change, Agriculture and Food Security (CCAFS), Copenhagen, Denmark. www.ccafs.cgiar.org.
- Sloat, L. L., Davis, S. J., Gerber, J. S., Moore, F. C., Ray, D. K., West, P. C., & Mueller, N. D. (2020). Climate adaptation by crop migration. Nature Communications, 11(1), 1243. https://doi.org/10.1038/s41467-020-15076-4
- Taylor, M. (2018). Climate-smart agriculture: What is it good for? The Journal of Peasant Studies, 45(1), 89–107. https://doi.org/10.1080/03066150.2017.1312355
- Thakuri, S., Dahal, S., Shrestha, D., Guyennon, N., Romano, E., Colombo, N., & Salerno, F. (2019). Elevation-dependent warming of maximum air temperature in Nepal during 1976–2015. Atmospheric Research, 228, 261–269. https://doi.org/10.1016/j.atmosres.2019.06.006
- Totin, E., Segnon, A. C., Schut, M., Affognon, H., Zougmoré, R. B., Rosenstock, T., & Thornton, P. K. (2018). Institutional perspectives of climate-smart agriculture: A systematic literature review. Sustainability, 10(6), 1990. https://doi.org/10.3390/su10061990
- Ullah A, Ahmad I, Ahmad A, Khaliq T, Saeed U, M Habib-Ur-Rahman, Hussain J, Ullah S, Hoogenboom G (2019a) Assessing climate change impacts on pearl millet under arid and semi-arid environments using CSM-CERES-Milletmodel. Environmental Science and Pollution Research International, 726:6745–6757. https://doi.org/10.1007/s11356-018-3925-7
- Vernooy, R., & Bouroncle, C. (2019). Climate-smart agriculture: In need of a theory of scaling. CCAFS Working Paper no. 256. CGIAR Research Program on Climate Change, Agriculture and Food Security (CCAFS). www.ccafs.cgiar.org
- Wekesa, B. M., Ayuya, O. I., & Lagat, J. K. (2018). Effect of climate-smart agricultural practices on household food security in smallholder production systems: Micro-level evidence from Kenya. Agriculture & Food Security, 7, 1–14. https://doi.org/10.1186/s40066-018-0230-0.
- Wester, P., Mishra, A., Mukherji, A., & Shrestha, A. B. (2019). The Hindu Kush Himalaya assessment: Mountains, climate change, sustainability and people (p. 627). Springer Cham. https://doi.org/10.1007/978-3-319-92288-1
- Westermann, O., Thornton, P. K., & Förch, W. (2015). Reaching more farmers. Innovative approaches to scaling up climate-smart agriculture. CCAFS Working paper 135. CGIAR Research Program on Climate Change, Agriculture and Food Security. https://cgspace.cgiar.org/rest/bitstreams/60041/retrieve
- World Bank. (2003). Scaling-up the impact of good practices in rural development: A working paper to support implementation of the World Bank’s rural development strategy. World Bank Group.
- Xu, J., Badola, R., Chettri, N., Chaudhary, R. P., Zomer, R., & Pokhrel, B. (2019). Sustaining biodiversity and ecosystem services in the Hindu Kush Himalaya. In P. Wester, A. Mishra, A. Mukherji, & A. B. Shrestha (Eds.), The Hindu Kush Himalaya assessment, mountains, climate change, sustainability and people (pp. 127–165). Springer Nature.
- Yamane, T. (1967). An introductory analysis (2nd ed.). Harper and Row.