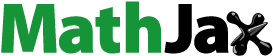
Abstract
Contract farming was implemented in Tigray to improve access to support and marketing services and ensure consistency in the volume of supplied products. However, its impact has not been examined yet. Therefore, this study estimates the impact of contract farming on sesame productivity farmers in western Tigray. The study uses cross-sectional farm household-level data collected in 2021 with a sample of 397 household heads and those household heads were selected using a multistage stratified random sampling technique. Propensity Score Matching and Endogenous Switching Regression models were employed to estimate the impact of contract farming on Sesame productivity and to check the robustness of the results. Our findings show that participation in contract farming corroborates important improvements for contract farmers via the chosen output indicators. The number of workers used, farming experience, inorganic fertilizer use, seed variety, and farm size allocated to sesame were positively associated with both contract and non-contract farmers. However, years of schooling were positively correlated while the age of household heads was negatively associated with contract farmers. On the other hand, access to extension services was negatively correlated with the non-contract farmers. The endogenous switching regression model predicts two results coincidentally for the contract and non-contract farmers. The result indicates that 400 kg per hectare would reduce sesame productivity if the farmers would not adopt contract farming while non-contract farmers could improve their sesame productivity by 150 kg per hectare if they would be part of the program.
REVIEWING EDITOR:
1. Introduction
In many developing African countries, agriculture is a crucial sector as it encourages economic growth, reduces poverty, and improves livelihoods (Sarkar et al., Citation2023). Contract farming offers opportunities for better access to support services like financing and credit as well as marketing facilities such as sorting, grading, and packaging services facilities for small-scale farmers (Pili & Ncube, Citation2022). Additionally, for the contracting firms contract farming offers enhanced consistency in the quantities and quality of supplied products (Pili & Ncube, Citation2022). Although agriculture is the backbone of low-income African countries, its productivity is still low compared to that of the non-agricultural sector in similar countries (Sifundza, Citation2019). This is due to diverse reasons, such as limited access to new agricultural inputs, soil infertility, limited access to credit institutions, and limited improved extension services, which are important inputs for enhancing productivity (Buehren et al., Citation2019). Besides, there are also factors that limit farmer’s adoption of private and public credit institutions, those are due repayment period, the amount of interest rate, off-farm income, extension contact, lending procedure, and group lending (Sifundza, Citation2019).
Contract farming was introduced as a means to improve the productivity of agriculture and the livelihoods of households in low-income countries (Bidzakin et al., Citation2020). In contract farming, typically, contractors agree with target farmers directly or through farmers’ unions for one calendar year (Kabwe et al., Citation2018). Under this agreement, the contractor provides agricultural inputs while the farmers provide specific products (Kabwe et al., Citation2018). Furthermore, the contractor also provides a guarantee to purchase the products at the best price (Kabwe et al., Citation2018). Contract farming involves a legally binding agreement between two parties, where they pre-determine the terms for conducting agricultural business. This includes the agreed-upon volume, quality, and indicative price before the producer begins the production process.
Although the Ethiopian government introduced contemporary agricultural inputs to improve sesame productivity, few farmers knew how to use those (Shikur, Citation2023). This awareness gap is mainly caused by a lack of economic scale, poor market access, and inadequate extension worker performance in farmers’ fields (Mezgebo et al., 2021). The cost of new agricultural input is also another constraint for those who have adequate evidence regarding its significance in sesame productivity (Shikur, Citation2023). Contract farming is the best policy that can solve the above constraints (Federgruen et al., Citation2019). Currently, contract farming is widely introduced in sub-Saharan countries, and it is supposed to be an instrument for farming transformation in these countries due to its ability to solve productivity and farming marketing challenges simultaneously (Bezabeh et al., Citation2020). Many studies indicated that contract farming supports farmers through immediate participation in the profitable market, modern agricultural inputs and credit institutes to enhance their crop productivity (Adam & Agegnehu, Citation2023; Hoang, Citation2021).
Chen and Chen (Citation2021) revealed that adoption of contract farming has a significant impact on crop productivity. On the other hand, Mazwi et al. (Citation2020) reported that adoption of contract farming also pursues risk-sharing amongst agribusiness and crop producers. A study suggests that contract farming can reduce market imperfections for contract farmers by providing the required agricultural inputs, credit services, and evidence that reduces transaction costs (Khanal et al., Citation2020). Another study revealed that contract farming played a key role in increasing crop productivity and adopting new farming inputs, with a focus on legume crops (Bezabeh et al., Citation2020). It was further elaborated that adopting contract farming has a toll cost of production compared to non-contract farmers in Ethiopia.
Schoneveld (Citation2022) explained that there are several obstacles that prevent Ethiopian farmers from adopting contract farming. One of the constraints is that contract farming does not allow for direct communication between agribusiness firms (such as exporters and processors) and farmers. Additionally, dealing costs can be high when agribusinesses provide credit, allocate inputs, and participate in crop gathering. Additionally, According to Yeshitila et al. (Citation2020), farmers who adopt contract farming tend to be more efficient than those who do not adopt. However, it is important to note that data envelopment analysis, which is used to determine efficiency, has its limitations such as measurement errors. Additionally, this technique is not suitable for testing hypotheses and is highly sensitive to the selection of input and output variables. This study also had a contextual research gap. Moreover, most of the current literature focuses on the impact of contract farming on production efficiency and smallholder welfare, as well as on perishable crop types (Bezabeh et al., Citation2020).
Several studies in Ethiopia have examined the impact of contract farming on the productivity and production efficiency of various crops. However, the impact of contract farming on sesame productivity in western Tigray has not been widely studied. In an effort to improve sesame productivity, contract farming was introduced in western Tigray, but its effect on sesame productivity has not yet been evaluated, specifically in the Kafta Humera district. Therefore, the objective of this study was to estimate the impact of contract farming on sesame productivity and identify the factors that prevent smallholder farmers in the Kafta Humera district from adopting contract farming. The results of this study will serve as primary evidence for agricultural experts, researchers, and policymakers, as it evaluates the impact of policy. Moreover, this study provides primary evidence of the impact of contract farming on sesame productivity and the factors that hinder contract farming adoption at the micro-level.
2. Materials and methods
2.1. Study area
The study was conducted in the Tigray region of the western zone, particularly in the Kafta Humera district, in which sesame is typically cultivated. The district has an annual mean temperature of 27 °C and an annual mean rainfall of 510 mm (Hailu & Mezegebo, Citation2021). The economy of the district relies heavily on the production of high-value edible oil from sesame. Local farmers, who cultivate an average of 12 hectares each, contribute to 42% of the total farmland in the district, as reported (Hailu & Mezegebo, Citation2021). The production of sesame typically involves labor-intensive techniques, especially during harvesting, threshing, and weeding (Kassie et al., Citation2023). Central Statistical Agency (Dori, Citation2008) of Ethiopia, the total population size of the district is 90,256 out of which 41,374 are women and the remaining 48,882 are men. The density of the population is 18.16; the total households of the district were 21,483; and 2842.20 square kilometres of land was devoted to sesame cultivation (Hailu & Mezegebo, Citation2021).
2.2. Sampling frame
To make this study inclusive and representative, we employed multi-stage sample collection methods in selecting sesame farmers. First, the Kafta Humera district was purposively selected depending on sesame production volume. Second, from the eighteen (18) kebeles of the district, we randomly selected five, namely Adebay, Adi-goshu, Maycadra, Rawyan and Bereket because they are found in the same ecological zone. Third, the rain-fed is the only production ecology, and this production ecology is divided into non-contract and contract farmers strata. After determining the total number of household heads in the kebeles, we divided them into two sub-groups based on whether they adopted contract farming or not. Additionally, after we divided them into sub-groups, we randomly selected representative sample sizes from each group. Therefore, the target population was purposively divided into two sub-groups during the representative sample selection process. Next, we utilized simple random sampling techniques to obtain a representative sample of farmers from each group. A total of 397 representative household heads were selected, including 149 non-contract farmers and 248 contract farmers.
2.3. Sources and types of data
Both primary and secondary sources of data were used for this study. The primary data was collected from both contract and non-contract farmers households using survey methods. To triangulate the cross-sectional quantitative data secondary sources of data were also collected from research reports, journal articles, and from focus group discussion and key informant interviews. Besides, sesame production characteristics and descriptions of the study site were also gathered from the agricultural office of Kafta Humera District.
Our household survey was conducted by face-to-face interviews. A semi-structured questionnaire was developed for the household survey. Data collected from the household survey includes information on sesame production status, access to extension services, farm characteristics, and adoption of contract farming.
2.4. Ethical considerations
Appropriate ethical approval to conduct this research was obtained from the College of Dryland Agriculture and Natural Resource, Research and Community Engagement Committee, Mekelle University, Ethiopia (RPDO/MU/03/2020). The guidelines are in line with the international standards declaration of Helsinki (Adopted by the 18th WMA General Assembly, Helsinki, Finland, June 1964, Amended October 2013) appropriate compensation and treatment for individuals who are harmed as a result of participating in research must be ensured. In this study, the researchers were prepared a consent sheet in written form. However, since most of the participants who participated in the survey were unable to read and write, the consent form was presented orally to them by the researcher. Each participant was provided with a detailed explanation of the study’s aims, the information that would be collected, and any potential risks involved. Participants were informed that they were free to withdraw from the research at any time and that the confidentiality, anonymity, and privacy of all information would be guaranteed. Additionally, administrative authorization from the head of the department was obtained.
2.5. Model specification of endogenous switching regression
The decision of whether to adopt contract farming (CF) is modeled using the Random Utility Theory (RUT). This theory assumes that farmers are risk-neutral and will choose to participate based on the utility they expect to receive. In the case of sesame farmers, they are assumed to choose the support and financial service option that will provide them with the maximum benefits. As such, their decision to adopt CF will be influenced by the utility they expect to derive from it (Abdulai & Huffman, Citation2014). Under the assumption that, the utility (yield and gross margin) farmers derive from CF adoption is YKCF, and the utility from non-adoption is represented as YKNCF for the endogenous switching regression.
The two regimes can be specified as follows:
Where XK is a vector of variable factor, βCF and βNCF are the parameter estimates for CF and NCF, respectively, and UKCF and UKNCF are the error terms, which are assumed to be i.i.ds. given that households choose to adopt contract farming or not to adopt contract farming, the observed net benefit produces the following two equations:
YKNCF and YKCF represent the outcome variable (sesame productivity) for non-contract farmers and contract farmers, respectively, while XK is the vector of variables.
The process of estimating ESR involves two steps. In the first step, a probit regression is used to determine the probability of adoption in contract farming and to estimate the parameters mentioned in the above regimes. However, this two-step estimation mechanism has a limitation. It produces heteroscedastic residuals, which means that they cannot be used to obtain consistent standard errors without a complex adjustment process (Lokshin & Sajaia, Citation2004). To overcome this problem, the full information maximum likelihood method is suggested, which involves a simultaneous estimation of the two equations, i.e. the outcome and adoption equations. Finally, the error terms are expected or assumed to have a trivariate normal distribution, with zero mean and covariate matrix.
Dagnew et al. (Citation2023) have been reported that estimating the impact of contract farming on agricultural productivity can lead to biased results, making it difficult to predict its actual impact on crop productivity. Although the study suggests that the issue is not about causal inference, the counterfactual situation is a significant concern. When dealing with cross-sectional data, it becomes difficult to identify the counterfactual problem and establish causal inference. However, by estimating the disparity in results between contract farmers and non-contract farmers, we can address these concerns and better understand the impact of contract farming adoption (Dagnew et al., Citation2023).
Benedetto et al. revealed that the propensity score matching (PSM) econometric model was used to estimate the impact of new agricultural input adoptions on farm productivity. Particularly, this model is relevant if self-selection bias is a concern. Nevertheless, the PSM model attempts to detect the distribution of covariates between the contract farmers and those non-contract farmers. Hence, employing a logit or probit model cannot control these problems. Propensity score matching (PSM) is a statistical method used to match treated and untreated groups in order to estimate causal effects. However, PSM has limitations when it comes to unobservable variables, such as a farmer’s interest, cooperation, consciousness, and innate ability endowment, which can affect the outcome. Moreover, PSM assumes that the baseline characteristics of the groups are homogenous, which may not always be the case. PSM also discards unmatched observations, which can result in a loss of statistical efficiency, and post-matching adjustments can sometimes lead to biased results.
A general form of Heckman’s selection correction model which is the endogenous switching regression model can control for selection bias by making selectivity an omitted variable problem (Heckman, Citation1979). Most significantly, the endogenous switching regression model shows farm productivity for both contract farmers and non-contract farmers and the factors determining them.
Therefore, the endogenous switching regression model was employed to estimate the impact of contract farming adoption on sesame productivity and to control the selection bias. Endogenous switching regression is a methodology used to address the limitations of propensity score matching. Specifically, ESR is useful for countering the issue of endogeneity and preventing selection bias caused by unobservable factors. However, there is a limitation to ESR in that the selection process requires the use of exclusion restriction, which can sometimes lead to bias. Additionally, ESR may not always account for unobservable factors such as talent, skill, consciousness, and perseverance.
2.6. Data analysis
The primary data collected from both contract and non-contract farmers such as their demographic and socio-economic status were presented through t-statistics below. In this study, it was analyzed the relationship between total farm size, total sesame harvested, and total land cultivated by contract and non-contract farmers with sesame productivity. The mean difference, standard deviation, and polynomial smoothing graphs to examine this association. Additionally, PSM was employed to determine the average treatment on the treated (contract farming adoption) in the area under study. While PSM provides unbiased results by manipulating only the observed bias, it may not account for unobserved biases.
Meanwhile, the endogenous switching regression model was used to account for both observed and unobserved bias in analyzing the impact of contract farming on sesame productivity. Bidzakin et al. (Citation2019) revealed that this model is the preferred method when assessing the impact of new agricultural inputs when the data is cross-sectional data. The study also examined the heterogeneity effect, through investigating whether the impact of contract farming differed between farmers who had adopted it and those who had not.
3. Results and discussions
3.1. Households description
As illustrated in , contract farmers had an average of 5.13 years of experience in sesame farming, while non-contract farmers had an average of 3.55 years of experience. The average farmer experience difference in sesame farming between the contract farmer and non-contract farmer was 1.58 years and it is also statistically significant at 1%. Looking at the mean age of the farmers it was 40.23 years for the contract farmer and 35.15 years for the non-contract farmers.
Table 1. Summary and test statistics of farmers.
The average age difference between the two groups was about 5 years. Based on the International Labor Organization (Blustein et al., Citation2019) these ages were categorized into economically active labor force groups. Further, this result implies that the district has an abundant active labor force and this is an advantage for local farmers as sesame cultivation requires a huge quantity of labor force.
In terms of the average productivity of sesame, contract farmer produces higher compared to non-contract farmers 379 kg per hectare. The result implies that farmers’ adopting of contract farming helped them to produce more sesame compared with those who didn’t adopt contract farming. According to the qualitative data gathered from focus group discussions and key informants, due to participation in contract farming, sesame productivity increased over time. This finding corroborates with the study of May et al. (Citation2021); Bidzakin et al. (Citation2019) found that contract farming had a positive impact on crop productivity.
Regarding the total gender distribution in both contract farmers and non-contract farmers, 65.49% of them were male farmers and the remaining 34.51% were female farmers. Bezabeh et al. (Citation2020) sated that the total distribution of female farmers in Ethiopia was 29%. The gender respondent distribution in the study area is greater than the total female farmer distribution in Ethiopia. However, it is lower compared to that of other developing countries, which is about 43.20%. Comparing the gender respondent distribution among contract farmers and the non-contract farmers, there was a big distribution (70%) in contracting farmers in the district, or male farmers have a higher number than female farmers.
The average arable landholding for respondents who adopting contract farming was 6.56 ha, while the landholding for a non-contract farmer was 4.25 ha. The average landholding by both contract and non-contract farmers is much higher compared to the Tigray regional average landholding of a small-scale farmer, which is 1.23 ha. The Focus Group Discussion and Key Informant Interviews, in the study area, household heads own large arable land; for two reasons, The first was that the district has a large potential for cultivation land which was prepared by extension farming. Second, the district has less population compared to other districts in the region. These two reasons are the advantages for the individual farmers to hold a large size of arable farm.
Furthermore, the quantity of inorganic fertilizer used by contract farmers is higher than that of non-contract farmers. Inorganic fertilizer adoption is among the modern agricultural input’s farmers use to enhance crop productivity. The quantity of inorganic fertilizer used in sesame farms cultivated by contract farmers was greater compared to sesame farms cultivated by non-contract farmers. This result agrees with the findings of Hailu and Mezegebo (Citation2021) who indicated that contract farmers used more inorganic fertilizers than non-contract farmers due to support services such as fertilizers and seed varieties from contracting firms. The average formal educational status of household heads, both the contract farmers and non-contract farmers, were 8.97 years of schooling and 8.18 years of schooling, respectively. This result revealed that household heads who attained higher educational levels were most likely to adopt contract farming to improve sesame productivity.
Looking at the quantity of labor input used for sesame cultivation, there was a significant difference between contract farmers and non-contract farmers. The average labor input employed by a contract farmer was four persons/day, while the average labor input employed by a non-contract farmer was about three persons/day. This result revealed that contract farmers employed higher daily labor input than non-contract farmers in their farm activity. In general, different studies by Prowse (Citation2022) and Schoneveld (Citation2022) employed different units to measure the role of various daily labour inputs in crop productivity. As small-scale farmers in developing countries mostly use household daily labor input without payment and it is difficult to calculate the household daily labor factor. This result is supported by the work of Prowse (Citation2022) indicated that small-scale producers who participated in contract farming hired more professional and unprofessional labor inputs.
As illustrated in (), local polynomial regression was adopted to establish an association between the total volume of sesame harvested with the size of land planted with sesame (Panel 1), and the yield of sesame obtained with land planted sesame (Panel 2). The total volume of sesame produced and the size of land planted under sesame were directly correlated for both contract and non-contract farmers. The above result shows that the graph is slightly constant when the farm size is below ten hectares. This indicates that farmers who cultivate small farm sizes produced more sesame. This result is consistent with the findings (Martey et al., Citation2019) that reported that farm size and rice productivity had a negative relationship due to farm management efficiency.
Figure 1. Local polynomial regression result of all households.
Panel 1: Volume of Sesame Harvested vs. Land Planted with Sesame, Panel 2: Productivity of Sesame vs. Land Planted with Sesame for contract farmers.
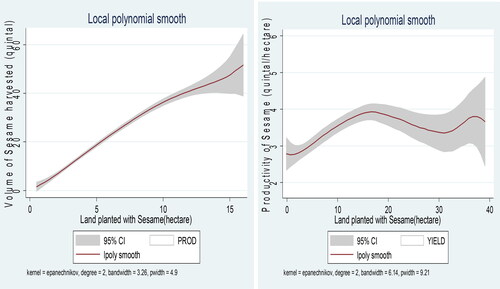
The adoption of contract farming aims to improve sesame productivity for small-scale farmers. On average, the result implied that there is a direct relationship between sesame produce and farm size planted. On average, there is a direct relationship for land size below twelve hectares, but beyond that point, they were an inverse relationship. This finding is consistent with the idea in developing countries; there is an inverse between crop productivity and the size of land cultivated. Further, this result indicates farmers with small farm sizes were more likely to participate in contract farming to enhance sesame productivity. This outcome agrees with the findings Dubbert et al. (Citation2023) that indicated that households who had small farm sizes were ready to work with the contracting firms to get support services and technical advice.
As depicted in (), the result of non-parametric local polynomial regression shows significant differences between the farmers who adopt contract farming to improve sesame productivity and farmers who didn’t adopt contract farming. In terms of contract farmers, the initial point of panel 3 implied that when the size of land deviated to sesame was small, sesame yield was high. Small farm size provides more sesame productivity compared to large farmland. This result shows that there was an inverse relationship between sesame yield and the size of land deviated for sesame. This argument corroborates the result from the focus group discussion and key informant interview indicating that the small size of a farm makes accomplishing farm activities very easy. On average, the augments of sesame were growing at an increasing rate, and it reached the maximum level of yield; after that point, sesame yield started to decline. In the same panel, (panel 3), when the farm size planted with sesame increased, the yield of sesame indicated a reduction. This is due to the requirement of better management skills for large farm activities than for small farm activities, and this has a negative influence on sesame productivity. In developing countries, there is an inverse relationship between the size of cultivated area and land productivity, and this result is consistent with that idea. This result is in line with the findings of Ayamga (Citation2023) who found that farm size and farm productivity had an inverse relationship due to management efficiency.
Figure 2. Local polynomial regression result of the two groups.
Panel 3: Productivity of Sesame VS. Farm Size Cultivated by Contract Farmer, Panel 4: Productivity of Sesame VS. Farm Size Cultivated by Non-Contract Farmer.

On the other hand, panel 4 in the above graph implied that the relationship between farm size cultivated by the non-contract farmer and sesame yield was differently likened to a contract farmer.
3.2. Matching results
PSM estimation includes nearest neighbour matching (NNM), Kernel-based matching method, radius matching, and calliper matching. In the second stage of PSM, the impact of contract farming adoption on the average outcome variables (Sesame productivity) was determined, and the ATT was estimated. The NNM method is used to pair contract farmers with non-contract farmers based on their propensity score distance. However, the NNM faces bad matches if the propensity score distance between two neighbours is large. This problem can be removed by imposing a tolerance level on the maximum propensity score, and calliper matching with 0.1 restrictions was specified for common support conditions (Ibarra Michel et al., Citation2023). The kernel matching method constructs the counterfactual outcome and produces the ATE on the treated using the kernel weighted average of the farmers in the adopter group. The key capability of PSM estimation is controlling selection bias, which is dependent on two conditions: the balancing performance of the given covariates and the absence of systematic household heterogeneity due to the household’s unobserved characteristics (Ibarra Michel et al., Citation2023). The balancing test in PSM estimation assumes that it will balance the variable distribution, reduce bias, and eliminate potential differences in the given covariates (Ibarra Michel et al., Citation2023). Moreover, the PSM addresses the systematic difference due to observable characteristics among households in two ways: first, by estimating the probability of propensity score for each observed characteristic using the logit or probit model, and second, by matching each contract farmers with non-contract farmers who have the same propensity score value to estimate the ATT.
As illustrated in , our results suggest that contract farming in the study area is playing a crucial role at enhancing sesame productivity among smallholder farmers. As indicated by the nearest neighbor the outcome difference between the treated and controlled group is positive. This implies that individual farmers who adopt contract farming increased their sesame productivity by 59 kg per hectare than those who didn’t adopt contract farming. Similarly, the average treatment impact on the treated for the radius the result difference between the treated and controlled group of farmers is positive. Additionally, the result indicates that sesame productivity enhances by 81 kg per hectare due to adoption of contract farming.
Table 2. Results of matching algorithm.
The average impact of treatment on the treated for the remaining matching methods, kernel, and stratification, indicates a positive correlation between sesame productivity and contract farming (13 kg and 126 kg per hectare, respectively). In other words, after matching the treated and controlled farmers, it was found that the impact of the contract farming program led to an increase in sesame productivity by about 13 kg per hectare to 126 kg per hectare, using one period of data. This means that individual farmers who adopt contract farming produce more than those who don’t. Overall, the adoption of contract farming is crucial in enhancing the productivity of sesame, especially for smallholder farmers in low-income countries (Sell & Minot, Citation2018 and Pili & Ncube, Citation2022).
The significant and positive factors of sesame productivity as shown in () above are; adult labor used in the cultivation of sesame, farming experience, cost of fertilizer, variety of seed used, and land planted for sesame. The significant and negative determinant of sesame productivity is access to extension services.
Table 3. Endogenous switching regression result for contract farming participation.
The age of farmers has a significant negative impact on sesame productivity for the contract farmers. However, the age of farmers has no significant impact on sesame productivity for non- contract farmers. This result agrees with the findings of (Andani et al, Citation2020; Hoang & Nguyen, Citation2023) who described that a rise in age correlated with a low level of adoption of contract farming to avoid the probability of negative outcomes.
The farm experience of farmers has a significant impact on sesame productivity for both subgroups, and they are positively associated. In higher numbers, contract farmers achieve higher sesame productivity from additional years of farming experience than the non-contract farmers. The reason why contract farmers produce more sesame compared with the non-contract farmers is that they have better access to agricultural inputs and technical supports from the contracting firms. Since experience alone does not necessarily mean greater productivity farmers need other agricultural inputs and other financial services. The result from key informant interviews also indicated that, better farm experience helps farmers in managing pests and weeds, decide on planting and harvesting time, and enhancing soil fertility, which has an impact on sesame productivity. This finding was in collaboration with the study of Hoang and Nguyen (Citation2023) who indicated that farming experience was positively correlated with adoption of contract farming.
Farm size has a significant impact on sesame productivity for the treated and controlled groups. As expected, there is a positive association between land productivity and the size of the farm owned by farmers. Farmers who have large arable land covered by sesame produce more output compared to the owners of small plots of land with effective management. however, farm size covered by sesame has a positive correlation with sesame productivity as a result of better access to agricultural inputs for the treated group. This is to mean that the treated group produce more output than the controlled group at the same plot of land. The outcome indicates that households who cultivated small farm size for sesame have high probability to adopting contract farming to augment sesame productivity compare to households who cultivated large farm size. This result is consistent with the findings of Sheng and Chancellor (Citation2019); Hailu and Mezegebo (Citation2021) indicated that there a positive association between size of farm and adoption of contract farming in their respective study areas.
Both groups of farmers experience a significant increase in sesame productivity when they use improved sesame seeds. Improved seeds are known to augment crop productivity, which is why it’s expected to have a positive impact on sesame productivity. According to the key informant, farmers have a good understanding of how important it is to use improved sesame seeds to enhance productivity. Generally, farmers are better aware of the benefits of adopting improved seed varieties to increase sesame productivity compared to traditional ones. However, the impact of sesame seed variety on sesame productivity was greater for contract farmers than non-contract farmers. This is due to the fact that the contract farmers use other additional inputs that the sesame seed variety helps them to produce more than that of the non-contract farmers. This finding is consistent with studies of Meselu (Citation2019) and Maity et al. (Citation2023) who found that using improved seed variety had big contribution on contract farmers than non-contract farmers due to the reason that contract farmers have the opportunity to access other support services which help them to enhance sesame productivity.
Additionally, inorganic fertilizer adoption has a significant impact on sesame yield for both subgroups. The association between inorganic fertilizer and sesame productivity was positive and was as expected. Fertilizer application plays a crucial role by enriching the content of soil nutrients which helps crops to grow faster and bigger; this finally results in higher yields. Despite, the impact of fertilizer use was not the same for the two subgroups; its impact was more significant for the contract farmers compared to the non-contract farmers. This implies that contract farmers had better access to inorganic fertilizer and improved seed. These farmers were efficient and wise in applying the agricultural inputs. Moreover, as result from the group discussion and key informant interview indicated these farmers who were engaged in contract farming has a better access to marketing services like packaging, grading and sorting because they were producing better quantity and quality products. This result was in line with the studies of Kassie et al. (Citation2020) who indicated that inorganic fertilizer adoption and crop productivity had positive relationship.
As illustrated in , quantity of daily labour has a significant impact on sesame productivity for both contract and non-contract farmers. Besides, there is a positive correlation between land productivity and the number of labors. Notwithstanding, the amount of labor has an impact on sesame yield; as illustrated in , the impact was more on non-contract farmers than on contract farmers. This implies that non-contract farmers employed more labor input to come up with the same produce with the contract farmers. Sesame productivity depends upon the types of labor employed whether the farmer used more unpaid family daily labour and self-employed labour or hired labour. In the district, the farmers need massive manpower starting from land preparation, sowing, weeding, harvesting and trashing; for these purposes, they hire laborers who come from all over the country. Even though agricultural mechanization was introduced in the district, it was more limited to large-scale farmers and demonstration sites. Results from qualitative data shows that unpaid family labors were more productive with less supervision while the hired laborers were less productive but they required more supervision. This result is consistent with the works of Emerick (Citation2018) and Ruml et al. (Citation2022) who highlighted that number of labor employed by farmers plays a lot of contributions to augment crop productivity.
Furthermore, the results of the switching regression analysis, indicating that the level of education of contract farmers has a significant positive impact on sesame productivity. On the other hand, it has an insignificant yet positive impact on non-contract farmers. This result suggests that farmers with a better educational background are more likely to adopt new agriculture-related technologies than those who are not literate. Additionally, the extension service has a negative but significant impact on non-contract farmers. This means that farmers who do not regularly visit the extension office are less likely to adopt newly introduced agricultural technology. Our finding is in line with the works of (Dagnew et al., Citation2023) who indicated that formal educational level of farmers and crop productivity had positive correlation in their respective study areas. The implication of rho_2 greater than rho_1 and sigma_1 greater than sigma_2 means that contract farmers have an absolute advantage in sesame productivity due to participation in contract farming compared to the non-contract farmers. The result obtained from ESR estimated via full information maximum likelihood indicates that the negative correlation between contract farming and some covariates, but significantly different from zero. This result implies that both unobserved and observed factors determine the decision to adopt contract farming.
presents the impact of contract farming on Sesame productivity. The results indicate that farmers who took part in contract farming had an actual sesame yield of 1414 kg per hectare, while their counterfactual yield would have been 1089 kg per hectare. In contrast, farmers who did not adopt contract farming had an actual yield of 1794 kg per hectare, which could have increased to 1842 kg per hectare if they had joined the contract farming. It is evident that the farmers who took part in contract farming had better yields than those who did not. Moreover, the result for transitional heterogeneity is positive, it means that those farmers who are part of the contract farming are better off compared to the non-contract farmers. Besides, the positive result of transitional heterogeneity indicates that there is no any selection bias in the model. The results of both base heterogeneities are negative implies that the counterfactual result is greater than the actual result for the contract farmers. Additionally, the actual result is greater than the counterfactual result for the non-contract farmers. This variation comes due to the household’s unobservable characteristics. Our findings are consistent with the results of (Bellemare & Bloem, Citation2018; Zhang et al., Citation2023) who revealed that new agricultural inputs have big contributions at enhancing crop productivity.
Table 4. Impact of contract farming on sesame productivity.
4. Conclusion
In Tigray region, particularly in the western Tigray zone, farmers are adopting various agricultural inputs to boost Sesame productivity, one of them is contract farming. The study aimed to assess the impact of contract farming on sesame productivity. To estimate the impact of the contract farming on sesame productivity, endogenous switching regression was used for both contract and non-contract farmers. The results revealed that adopting contract farming significantly increases sesame productivity. Moreover, factors such as household size, daily labour input during farm cultivation, farming experience of farmers, use of inorganic fertilizer, use improved sesame seed variety, landholding size, and the educational background of farmers have a positive influence on sesame productivity. On the other hand, farmer’s age and access to extension services have a negative correlation with sesame productivity.
The result demonstrates that the adoption of contract farming had a positive contribution to enhancing sesame productivity. It was found that sesame productivity would be reduced by 400 kg per hectare if the farmers would not participate in the program. On the other side, non-contract farmers could improve their sesame productivity by 150 kg per hectare if farmers would be part of the contract farming.
The outcome indicates the significance of contract farming for improving sesame productivity, particularly for the small-scale households in the study area. This study mainly emphasized the present tie between new agricultural inputs introduction and farmers’ willingness to adopt them. Therefore, the full application of contract farming can scale up the smallholder rural farmers by changing the agriculture sector in general.
Although cross-sectional studies are generally less costly to perform, easy, and not need a lot of time, they have certain limitations. The results obtained from these studies may be biased due to the continuous change social, economic, psychological, demographic characteristics, and temporal confusion or protopathic bias can be a problem. Moreover, it is less likely to establish the reverse causality for some and this may cause an endogeneity problem. Therefore, the findings of such studies may not be able to show extended duration and may have some limitations.
However, the results of the study remain relevant and applicable not only to the district used as representative but also to other regions in the country that share similar topographic setting, Agro-climatic conditions, and edaphic. The study is particularly valuable in illuminating the contribution of contract farming to Sesame productivity. Therefore, this study is significant for policymaking and adds to the current literature. However, it is also suggested that further research can be conducted in this area, such as using panel studies to better capture the overall impact of adoption on farmers’ livelihoods. In addition, a plot-level study may be useful to understand whether the adoption of contract farming varies depending on factors such as the number of daily laborers, slope of the farm, soil fertility of field plots, landholding size, livestock density, and distance to farmers farm, and whether these factors may affect the study’s findings.
5. Recommendation
The study has put forward policy recommendations to relevant stakeholders. The regional and local administration units should prioritize the all-inclusive expansion of the contract farming package in their agricultural transformation program and overcome obstacles hindering its expansion. Additionally, a plan of action that supports the introduction of new technology should be given high priority as it can improve crop productivity as a whole.
There is a need for close collaboration between private sectors, contract farmers, government institutions, and non-governmental organizations. The goal is to enhance business opportunities and improve coordination between contracting firms and small-scale producers.
There is a significant demand for partnerships between contracting firms and farmers, particularly to enhance capacity building, access to support services, technical advice and increase crop productivity.
In Ethiopia, contract farming is still in its early stages, as is the case in many sub-Saharan African countries. The weak policy strategies and lack of institutional support require critical attention to improve agricultural productivity, as the country is anticipating. Foster cooperation and partnerships between farmers and contracting firms, including private partnerships, to promote the adoption and transfer of agricultural inputs.
Authors’ contributions
Haileslasie Gereziher Hailu: Conceptualization, Data Curation, Methodology, Formal Analysis, Writing-Original Draft, Writing-Review, Editing, revising comments, And Supervision. Gidey Kidu Mezgebo: Formal Analysis, Writing-Original Draft, Writing-Review, Data Curation, Revising Comments, and Editing.
Ethical approval
The study was approved by the College of Dryland Agriculture and Natural Resources, Research and Community Engagement Committee, Mekelle University, Ethiopia (RPDO/MU/03/2020).
Acknowledgement
I would like to express my heartfelt gratitude to Mr. Gidey Kidu for his invaluable technical guidance in designing, analyzing, interpreting the result as well as support and unwavering patience throughout the entire process of this study. I would also like to extend my thanks to Mekelle University for providing funding for this research.
Disclosure statement
There is no any conflict of interest reported by the author(s).
Data availability statement
Both authors declare and agreed that data will be made available on request.
Additional information
Funding
Notes on contributors
Haileslasie Gereziher Hailu
Haileslasie Gereziher Hailu (MSc) in Economics is a Lecturer and Researcher at Mekelle University, College of Dryland Agriculture and Natural Resources, Department of Agricultural and Resource Economics. His research interest is in Agricultural Productivity and Welfare of households, Sustainable Agriculture, Food Economics and Policy, and Climate Change and Agriculture.
Gidey Kidu Mezgebo
Gidey Kidu Mezgebo (Asst prof) in Natural Resource Economic and Policy is Lecturer and Researcher at Mekelle University College of Dryland Agriculture and Natural Resource, Department of Agricultural and Resource Economics. His research area interest is in Natural Resource Policy, Agricultural Productivity, Agricultural Policy, and agricultural technology.
References
- Abdulai, A., & Huffman, W. (2014). The adoption and impact of soil and water conservation technology: An endogenous switching regression application. Land Economics, 90(1), 1–13. https://doi.org/10.3368/le.90.1.26
- Adam, A. G., & Agegnehu, A. W. (2023). Contract farming as an alternative to large-scale land acquisition and promoting inclusive and responsible agricultural investment: Evidences from Ethiopia. Corporate Social Responsibility and Environmental Management, 30(6), 2840–2851. https://doi.org/10.1002/csr.2519
- Andani, A., Moro, A. H. B., & Issahaku, G. (2020). Fertilizer subsidy policy and smallholder farmers crop productivity: The case of maize production in North-Eastern Ghana. Journal of Agricultural Extension and Rural Development, 12(2), 18–25.
- Ayamga, M. (2023). Contract farming and smallholder farmer productivity in northern Ghana: Does farm size matter? Ghana Journal of Science. Technology and Development, 9(1), 89–104.
- Bellemare, M. F., & Bloem, J. R. (2018). Does contract farming improve welfare? A review. World Development, 112, 259–271. https://doi.org/10.1016/j.worlddev.2018.08.018
- Bezabeh, A., Beyene, F., Haji, J., & Lemma, T. (2020). Impact of contract farming on income of smallholder malt barley farmers in Arsi and West Arsi zones of Oromia region, Ethiopia. Cogent Food & Agriculture, 6(1), 1834662. https://doi.org/10.1080/23311932.2020.1834662
- Bidzakin, J. K., Fialor, S. C., Awunyo-Vitor, D., & Yahaya, I. (2020). Contract farming and rice production efficiency in Ghana. Journal of Agribusiness in Developing and Emerging Economies, 10(3), 269–284. https://doi.org/10.1108/JADEE-11-2018-0160
- Bidzakin, J. K., Fialor, S. C., Awunyo-Vitor, D., & Yahaya, I. (2019). Impact of contract farming on rice farm performance: Endogenous switching regression. Cogent economics & finance.
- Blustein, D. L., Kenny, M. E., Di Fabio, A., & Guichard, J. (2019). Expanding the impact of the psychology of working: Engaging psychology in the struggle for decent work and human rights. Journal of Career Assessment, 27(1), 3–28. https://doi.org/10.1177/1069072718774002
- Buehren, N., Goldstein, M., Molina, E., & Vaillant, J. (2019). The impact of strengthening agricultural extension services on women farmers: Evidence from Ethiopia. Agricultural Economics, 50(4), 407–419.
- Chen, J., & Chen, Y. J. (2021). The impact of contract farming on agricultural product supply in developing economies. Production and Operations Management, 30(8), 2395–2419. https://doi.org/10.1111/poms.13382
- Dagnew, A., Goshu, D., Zemedu, L., & Sileshi, M. (2023). Impacts of contract farming on asset accumulation of malt barley farmers in Northwestern Ethiopia. Cogent Economics & Finance, 11(2), 2230724. https://doi.org/10.1080/23322039.2023.2230724
- Dori, B. (2008). Assessment for a relational database management system (RDBMS) for the Ethiopian central statistical agency (CSA). CRIS, 120, 352.
- Dubbert, C., Abdulai, A., & Mohammed, S. (2023). Contract farming and the adoption of sustainable farm practices: Empirical evidence from cashew farmers in Ghana. Applied Economic Perspectives and Policy, 45(1), 487–509. https://doi.org/10.1002/aepp.13212
- Emerick, K. (2018). Agricultural productivity and the sectoral reallocation of labor in rural India. Journal of Development Economics, 135, 488–503. https://doi.org/10.1016/j.jdeveco.2018.08.013
- Federgruen, A., Lall, U., & Şimşek, A. S. (2019). Supply chain analysis of contract farming. Manufacturing & Service Operations Management, 21(2), 361–378. https://doi.org/10.1287/msom.2018.0735
- Hailu, H. G., & Mezegebo, G. K. (2021). Estimating the impact of inorganic fertilizer adoption on sesame productivity: evidence from Humera, Tigray, Ethiopia. Cogent Food & Agriculture, 7(1), 1933798. https://doi.org/10.1080/23311932.2021.1933798
- Heckman, J. J. (1979). Sample selection bias as a specification error. Econometrica, 47(1), 153–161. https://doi.org/10.2307/1912352
- Hoang, V. (2021). Impact of contract farming on farmers’ income in the food value chain: A theoretical analysis and empirical study in Vietnam. Agriculture, 11(8), 797. https://doi.org/10.3390/agriculture11080797
- Hoang, V., & Nguyen, V. (2023). Determinants of small farmers’ participation in contract farming in developing countries: A study in Vietnam. Agribusiness, 39(3), 836–853. https://doi.org/10.1002/agr.21795
- Ibarra Michel, J., Dréon, J., Boccard, M., Bullock, J., & Macco, B. (2023). Carrier-selective contacts using metal compounds for crystalline silicon solar cells. Progress in Photovoltaics: Research and Applications, 31(4), 380–413. https://doi.org/10.1002/pip.3552
- Kabwe, S., Mutambara, J., Mujeyi, K., Blackmore, E., Vorley, B., & Weng, X. (2018). Contract farming and informality: drivers and governance responses in Zambia and Zimbabwe. International Institute for Environment and Development.
- Kassie, A. M., Abate, B. B., Kassaw, M. W., Aragie, T. G., Geleta, B. A., & Shiferaw, W. S. (2020). Impact of knowledge and attitude on the utilization rate of cervical cancer screening tests among Ethiopian women: A systematic review and meta-analysis. PLoS One, 15(12), e0239927. https://doi.org/10.1371/journal.pone.0239927
- Kassie, S. Y., Chereka, A. A., & Damtie, Y. (2023). Systematic review and meta-analysis of knowledge on PMTCT of HIV/AIDS and association factors among reproductive age women in Ethiopia, 2022. BMC Infectious Diseases, 23(1), 491. https://doi.org/10.1186/s12879-023-08461-z
- Khanal, A. R., Mishra, A. K., Mayorga, J., & Hirsch, S. (2020). Choice of contract farming strategies, productivity, and profits: Evidence from high-value crop production. Journal of Agricultural and Resource Economics, 45(3), 589–604.
- Lokshin, M., & Sajaia, Z. (2004). Maximum likelihood estimation of endogenous switching regression models. The Stata Journal: Promoting Communications on Statistics and Stata, 4(3), 282–289. https://doi.org/10.1177/1536867X0400400306
- Maity, A., Paul, D., Lamichaney, A., Sarkar, A., Babbar, N., Mandal, N., Dutta, S., Maity, P. P., & Chakrabarty Chakrabarty, S. K. (2023). Climate change impacts on seed production and quality: Current knowledge, implications, and mitigation strategies. Seed Science and Technology, 51(1), 65–96. https://doi.org/10.15258/sst.2023.51.1.07
- Martey, E., Kuwornu, J. K., & Adjebeng-Danquah, J. (2019). Estimating the effect of mineral fertilizer use on land productivity and income: Evidence from Ghana. Land Use Policy, 85, 463–475. https://doi.org/10.1016/j.landusepol.2019.04.027
- May, S. G., Huber, C., Roach, M., Shafrin, J., Aubry, W., Lakdawalla, D., Kane, J. M., & Forma, F. (2021). Adoption of digital health technologies in the practice of behavioral health: qualitative case study of glucose monitoring technology. Journal of Medical Internet Research, 23(2), e18119. https://doi.org/10.2196/18119
- Mazwi, F., Chambati, W., & Mudimu, G. T. (2020). Tobacco contract farming in Zimbabwe: power dynamics, accumulation trajectories, land use patterns and livelihoods. Journal of Contemporary African Studies, 38(1), 55–71. https://doi.org/10.1080/02589001.2020.1746752
- Meselu, Y. K. (2019). A review on the seed sector of Ethiopia: Prospects and challenges of faba bean seed supply. South Asian Journal of Development Research, 1(1), 44–54.
- Mukucha, P., Tsekea, S., Jaravaza, D. C., & Jaravaza, N. (2024). Contract farming: a comparison of production and marketing contracts as sourcing strategies in the fastfood restaurant industry. Journal of Foodservice Business Research, 10(1), 1–18. https://doi.org/10.1080/15378020.2024.2303540
- Pili, O., & Ncube, B. (2022). Smallholder farmer coping and adaptation strategies for agricultural water use during drought periods in the Overberg and West Coast Districts, Western Cape, South Africa. Water SA, 48(1), 97–109.
- Prowse, M. (2022). Tobacco contract farming, crop diversification and household relations in the central region of Malawi. Journal of Southern African Studies, 48(2), 355–374. https://doi.org/10.1080/03057070.2022.2043653
- Ren, Y., Peng, Y., Campos, B. C., & Li, H. (2021). The effect of contract farming on the environmentally sustainable production of rice in China. Sustainable Production and Consumption, 28, 1381–1395. https://doi.org/10.1016/j.spc.2021.08.011
- Ruml, A., Ragasa, C., & Qaim, M. (2022). Contract farming, contract design and smallholder livelihoods. Australian Journal of Agricultural and Resource Economics, 66(1), 24–43. https://doi.org/10.1111/1467-8489.12462
- Sarkar, S. M., Dhar, B. K., Crowley, S. S., Ayittey, F. K., & Gazi, M. A. I. (2023). Psychological adjustment and guidance for ageing urban women. Ageing International, 48(1), 222–230. https://doi.org/10.1007/s12126-021-09467-1
- Schoneveld, G. C. (2022). Transforming food systems through inclusive agribusiness. World Development, 158, 105970. https://doi.org/10.1016/j.worlddev.2022.105970
- Sell, M., & Minot, N. (2018). What factors explain women’s empowerment? decision-making among small-scale farmers in Uganda. In Women’s studies international forum (Vol. 71, pp.46–55). https://doi.org/10.1016/j.wsif.2018.09.005
- Sheng, Y., & Chancellor, W. (2019). Exploring the relationship between farm size and productivity: Evidence from the Australian grains industry. Food Policy, 84(2019), 196–204. https://doi.org/10.1016/j.foodpol.2018.03.012
- Shikur, Z. H. (2023). Competitive agricultural input markets in Ethiopia: Theoretical promises versus reality. Regional Science Policy & Practice, 15(6), 1276–1298.
- Sifundza, S. B. (2019). Contract farming and access to formal credit in South Africa: A case of small-scale sugarcane growers in the Felixton Mill area of KwaZulu-Natal [Doctoral dissertation]. University of Pretoria.
- Yeshitila, M., Bunyasiri, I., Sirisupluxana, P., & Suebpongsakorn, A. (2020). The impact of contract farming on technical efficiency in Ethiopia’s smallholder sesame production. Journal of the Austrian Society of Agricultural Economics, 16(4), 57–66.
- Yitayew, A., Abdulai, A., & Yigezu, Y. A. (2022). Improved agricultural input delivery systems for enhancing technology adoption: evidence from a field experiment in Ethiopia. European Review of Agricultural Economics, 49(3), 527–556. https://doi.org/10.1093/erae/jbac006
- Zhang, W., Gao, L., Zolghadr, M., Jian, D., & ElHafsi, M. (2023). Dynamic incentives for sustainable contract farming. Production and Operations Management, 32(7), 2049–2067.