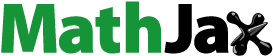
Abstract
Agricultural credit and subsidies are crucial policies for improving agricultural productivity. The main objective of this study is to examine the effects of agricultural credit and subsidies on agricultural productivity in Ethiopia from 1990 to 2021, taking into account both short- and long-term relationships. We obtain secondary data from the Food and Agriculture Organization and the World Bank databases. The autoregressive distributive lag model to co-integration is applied to estimate the long- and short-run effects of agricultural credit and subsidies on productivity. The bound test results indicate that the variables have a long-term relationship. The findings revealed that in the short-run, credit provision affected productivity negatively but subsidies to agricultural sector showed a positive significant effect. However, in the long run, the effect of credit turned out to be positive and significant, whereas subsidies affected productivity negatively. It is recommended that the government design and implement policy measures that create better access to agricultural financing to enjoy long-run improvements in productivity. Similarly, the government should offer subsidies to the agricultural sector only during short-run periods and need to lift them gradually in the long run as their provision retards productivity in the long run.
Reviewing Editor:
Subjects:
1. Introduction
Agriculture is the mainstay of a significantly large proportion of the rural population in many developing countries including Ethiopia. It is a source of household income, a means of rural livelihood and the cornerstone for realizing food security. At the country level, agriculture contributes significantly to GDP, foreign currency earning and a source of employment for the population in the country (Osman Nabay et al., Citation2022).
It is anticipated that increasing agricultural productivity through the removal of barriers to the adoption and use of contemporary agricultural practices will increase food security, mainly by giving farm households access to more food produced for their own consumption or more money to buy food through the commercialization of agricultural products (Cordonnier et al., Citation2022). Many agricultural policies have been put into place by the Ethiopian government, including market liberalization, structural adjustment, agricultural-led industrialization, programs for sustainable development and poverty reduction, accelerated and participatory sustainable development to end poverty, and successive growth and transformation plans I and II to increase agricultural productivity from 1991 to 2016 (Shakur, Citation2020).
Despite its importance in the overall development of the country and its role as an engine for economic growth, the level and pace of productivity growth are very low in most LDCS. Specifically, the pace with which productivity improvement takes place is a serious bottleneck in the development process of many countries. This calls for enhancing productivity and expanding the supply in the sector, which requires identifying the main factors contributing to this low level of productivity. Different studies identified several factors as the main drivers of agricultural productivity growth in developing countries. These may include, but are not limited to, the adoption of new technologies, the use of modern agricultural practices, commercialization, and the proper use and application of productivity enhancement techniques (Shakur, Citation2020; Alemu, Citation2022).
Agricultural credit enables producers to meet the cash needs imposed by the cycle of production that distinguishes farming: preparing the land, growing, cultivating, and collecting the crops usually takes place over a few months in which very little cash sales are generated, whereas expenses on resources, bought inputs, and usage require to be funded with money (Engida, Citation2022). Agricultural finance is required to buy better farm supplies when modernizing agriculture from a traditional farming method. Farmers must have access to financing to boost agricultural productivity (Urago and Bozoglu, Citation2021). However, the fundamental issue with agricultural credit is that when it is utilized for non-agricultural uses while the expenditure financing for farming is inadequate, the goals of policies aimed at boosting agricultural output and farmer incomes are never accomplished (Chaiya et al., Citation2023).
The adoption of technology to boost agricultural output is the primary goal of programs for input subsidies. In particular, input subsidies might start a “virtuous cycle” encouraging farmers to adopt new technology or improved farming methods. While some programs involve agricultural extension services to promote proper farming methods, others simply offer fertilizers and improved seeds to farmers so they can learn via trial and error (Nguyen et al., Citation2023). Generally speaking, the productivity of agriculture is significantly impacted by agricultural subsidies (Shakur, Citation2020). The particular difficulties the agricultural industry experiences and the significant role it plays in our society by maintaining food security are some of the reasons why agricultural subsidies are given. Agriculture is particularly vulnerable because it relies on the weather and susceptibility to unforeseen events like natural disasters.
Agricultural subsidies are a key government tool for boosting food production, farmer incomes, and social welfare in developing countries. Subsidy programs are important for farmers and rural communities, but their benefits vary depending on the crop, input, government program, and other factors (Badu and Lee, Citation2020). Long-term subsidy programs can be economically viable if they are efficient and fair. However, political and economic factors raise questions about the effectiveness of long-term initiatives. As a result, stakeholders have a strong interest in maintaining and expanding subsidies.
Prior studies have examined the impact of agricultural credit on productivity and output in Ethiopia using various models (Engida, Citation2022; Gebeyehu et al., Citation2020; Elias, Citation2019; Mukasa et al., Citation2017). However, most studies used cross-sectional data, and none considered the combined impact of credit and subsidies on agricultural productivity. This study aims to analyse the impact of agricultural financing and subsidies on productivity in agriculture in Ethiopia, a sector that makes a significant contribution to the country’s economy. Despite numerous factors affect agriculture’s productivity over the long and short terms. However, this paper is intended to provide some clues on how agricultural credit and subsidies affect agricultural productivity, which is the main issue that should be considered.
The remaining sections of the paper are organized as follows: Section 2 reviews the relevant literature, Section 3 describes the study methodology, Section 4 presents and discusses the research results, and Section 5 concludes the paper and discusses the policy implications.
2. Methodology
2.1. Source and description of data
This study used annual time series data from 1990 to 2021 from the World Development Indicators (WDI) and the Food and Agriculture Organization (FAO). The description of the variables used in this study is mentioned in .
Table 1. Definition, measurement and source of variables used in this study.
2.2. Model specification
The dependent variable of the model is agricultural productivity, and it is regressed on independent variables like credit to agriculture, agricultural subsidies, land area under cultivation, fertilizer consumption, agricultural employment, pesticide use, inflation rate, and foreign direct investment (FDI).
(1)
(1)
The log-linear version of the provided model is obtained from EquationEquation 1(1)
(1) as:
(2)
(2)
Where β1, β2, β3, β4, β5, β6, β7 and β8 are the estimated coefficients.
The log form of the model removes outliers and large coefficients. Elasticity values are important because they measure how agricultural productivity (AP) changes in response to changes in the independent variable.
2.3. Estimation technique
Essential checks on the variables are necessary to ensure accurate parameter estimates from the stated model when examining time series data. To ensure reliable estimates, we first examine the stationarity of all variables. We then use the Autoregressive Distributed Lag (ARDL) estimation approach of Onifade et al. (Citation2020) to examine the relationship between the variables. The ARDL technique is broadly suited for time series analysis, regardless of the order of integration, as long as none of the underlying variables are integrated in the second order. The ARDL model is the preferred econometric technique when the parameters are stationary at I(0) or integrated to order I (1). The ARDL model is an alternative tool for addressing the spurious regression problem (Ghouse et al., Citation2018). The autoregressive distributed lag (ARDL) model is one of the most widely used dynamic unconstrained models in the econometric literature.
2.3.1. Test of stationarity
Stationary data has a constant mean, variance, and covariance over time. When a series is differentiated using differencing operators, it generates multiple sets of observations. For example, the first-differenced values are Xt = Xt − Xt−1. A series is integrated of order 0 if it is stationary without differencing, and integrated of order 1 if it is stationary after first differencing. The Dickey-Fuller test was used to examine stationarity in the series (in levels or fractional differences). It is a well-known unit root test that improves on the Dickey-Fuller test by including additional lagged terms of the dependent variable to address autocorrelation. A simple ADF stationarity test can be described as follows:
(3)
(3)
EquationEquation (3)(3)
(3) compares the alternative hypothesis of no unit root to the null hypothesis of a unit root, where Y is the time series variable, t is time, α1, α2, and α3 are estimated parameters, Δ is the first difference operator, β1 are the estimated parameters of the differenced lag variables, and εt is the white noise error term. The series is stationary if the null hypothesis is rejected.
2.3.2. Autoregressive distributed lag (ARDL) model of the short-run and long-run
The ARDL estimation method examines the long-run relationship and short-run dynamic interactions among the variables under study. The study’s ARDL model is defined as follows:
(4)
(4)
Where Log represents natural logarithm and is the first difference operator, 𝛽0i, 𝛽1i, 𝛽2i, 𝛽3i, 𝛽4i, 𝛽5i, 𝛽6i, 𝛽7i and 𝛽8i show the model’s short-run dynamics, while,
,
,
,
,
,
,
,
and
indicate the ideal lag times,
the long-run association, and the stochastic error term at time t.
2.3.3. ARDL bounds test
The ARDL bounds test is a test for the existence of long-run equilibrium relationship between variables. It is determined by the overall test of significance of the lagged levels of the variables. Pesaran et al. (Citation2001) argue that this methodology is preferred for small samples and produces reliable results. Therefore, this study evaluates the F-relevance statistics. The study compares the alternative hypothesis of a long-run equilibrium relationship with the null hypothesis of no such relationship. The study concludes that the null hypothesis is more likely to be true.
This is done using EquationEquation (5)(5)
(5) .
(5)
(5)
The ARDL bounds test for cointegration compares the calculated F-statistic to the critical values provided by Pesaran et al. (Citation2001). If the F-statistic is greater than the upper bound, the null hypothesis of no cointegration is rejected, indicating a long-term equilibrium relationship between the variables. If the F-statistic is less than the lower bound, the null hypothesis cannot be rejected, and there is no evidence of a long-term equilibrium relationship. If the F-statistic falls between the lower and upper bounds, the result is inconclusive.
An ARDL model can be transformed into a dynamic error correction model (ECM) using a simple linear transformation. The ECM integrates short-run dynamics and equilibrium without losing long-run information. The following is a general outline of an ECM for accounting for increases in agricultural productivity:
(6)
(6)
Where X is a set of independent variables, illustrates the error correction factor.
are to be estimated for the short-run parameters. If there is any disequilibrium, the error correction term (ECT) from EquationEquation (6)
(6)
(6) indicates how quickly the variables will return to long-run equilibrium. A negative and significant ECT is expected, implying that the variables will restore equilibrium if there is any deviation from the long run. A positive ECT, on the other hand, indicates an explosive condition and suggests that the variables may not reach their equilibrium point.
3. Findings and discussion
3.1. Results from descriptive statistics
summarizes the descriptive statistics of variables used in the analysis. It reports the mean values, standard deviations, minimum, and maximum values that describe the nature of distribution of each variable. A 32-year time series from 1990 to 2021 is used for both the explanatory and dependent variables.
Table 2. Results of descriptive statistics.
3.2. Results of the stationary test
3.2.1. Plot of a time series
show the time series plots of the variables. All variables, except for inflation rate, appear to be non-stationary in their levels. However, they become stationary after first differencing. The ADF test is used to confirm the stationarity properties of the variables. The results of the ADF test are shown in .
3.2.2. The ADF test result
Before estimating the model, we must ensure that the variables are stationary. shows the results of the ADF test for stationarity. Only the inflation rate is stationary at the level and the other variables become stationary after first differencing implying that they are I(1).
Table 3. The augmented dickey-fuller unit root test.
3.3. The ARDL bounds test
Following the ARDL model’s estimation, the bounds test finds a long-term relationship at a 1% significance level between the dependent variable, agricultural productivity, and the other variables in the model. Compared to all of the critical values in , the F-statistic (95.661) is significantly higher. Therefore, there exists long-run relationship among variables. Co-integrated variables can establish or move towards a long-run equilibrium. However, short-run dynamics and fluctuations may cause disequilibrium. The error correction mechanism (ECM), estimated in , adjusts this disequilibrium. The large and statistically significant negative coefficient of EC (-1) indicates a high speed of adjustment.
Table 4. Bound test results for ARDL.
Table 5. Long-run and short-run estimates using ARDL (all variables are in log form).
3.4. Long-run coefficients and short-run dynamics
shows the long-run and short-run coefficients of the ARDL model for agricultural productivity and its determinants. In the long-run, agricultural credit has a positive and significant effect on agricultural productivity, but subsidies negatively affect agricultural productivity.
The findings indicate a strong and positive long-run relationship between agricultural credit and productivity which verifies the hypothesis. A 1% increase in agricultural credit increases productivity by 0.05%. This is because in the long run, credit could help farmers increase their productivity by allowing them to invest in new technologies and inputs, to expand their operations and to adopt new farming practices, all of which help to enhance productivity.
In addition to these direct effects, agricultural credit can also have indirect effects on productivity. For example, credit can help farmers to improve their access to markets and to obtain better prices for their products. The long-run relationship between agricultural credit and productivity is particularly important for countries like Ethiopia where agriculture is often a major source of employment and income, and it plays a vital role in food security. By providing farmers with access to credit, governments can help them to boost their productivity, improve their livelihoods, and contribute to economic growth. This finding aligns with previous research by Akinrinola and Okunola (Citation2020), Florence and Nathan (Citation2020), and Degu and Bekele (Citation2019), which also found a positive long-run relationship between agricultural credit and productivity.
Agricultural subsidies have a significant negative impact on agricultural productivity in the long run where, a percent increase in subsidies reduces agricultural productivity by 0.05% by rejecting the hypothesis. Agricultural subsidies can have a significant negative impact on agricultural productivity in the long run for some reasons. First, subsidies can discourage innovation and efficiency. When farmers receive subsidies for their products, they are less likely to invest in new technologies and practices that could improve their productivity. Second, subsidies can lead to overproduction. When farmers receive subsidies, they are incentivized to produce more crops than the market can absorb. This can lead to lower prices for farmers and can make it difficult for them to compete with farmers in other countries who do not receive subsidies. Our findings align with those of Chandio et al. (Citation2022), Danish et al. (Citation2017), and Yang et al. (Citation2023), who found that agricultural subsidies have a negative relationship with agricultural productivity.
The funding further shows that, as confirmed by the hypothesis, agricultural employment has a positive and significant long-run impact on agricultural productivity. A 1% increase in agricultural employment increases agricultural productivity by 1.2%. This finding is consistent with Ngalawa and Derera (Citation2020), who found that agricultural productivity, has grown rapidly due to rising labour productivity.
Pesticide use has a positive and significant long-run impact on agricultural productivity which verifies the hypothesis. A 1% increase in pesticide use increases agricultural productivity by 0.13%, because pesticides help to control pests and weeds, which can lead to higher yields. It implies that pesticides are employed to ward off pest infestations and manage weeds, leading to an increase in output. The outcome was confirmed by Zafeiriou et al. (Citation2023) who suggest that farmers were able to increase production and productivity by using more pesticides.
Inflation has a negative and significant long-run impact on agricultural productivity in Ethiopia where a 1% increase in inflation reduces agricultural productivity by 0.01% in the long run. The hypothesis supported the findings. This is because inflation increases input costs, such as labour, land, capital, fertilizer, and pesticides, which leads to uncertainty and restricts the growth of agricultural output. Mekonen (Citation2020) and Befikadu (Citation2022) also found that inflation hurts rather than helps the expansion of agricultural sectors.
Foreign direct investment (FDI) has a significant negative long-run impact on agricultural productivity (AP). The hypothesis validated the results. A 1% increase in FDI reduces AP by 0.07% in the long run. This finding is consistent with previous research by Ogbanje and Salami (Citation2022), Nyiwul and Koirala (Citation2022).
also shows the short-run results of the estimated model. The error correction term (EC) is negative (-0.81), indicating that that 81% of the disequilibrium of the previous year is corrected in the current year, which is a fairly fast adjustment speed. As shown in the table, agricultural credit has a negative significant effect on agricultural productivity in the short run, but subsidies have a positive effect. A 1% increase in agricultural credit reduces agricultural productivity by 0.04% in the short run. This may be due to the misuse of agricultural financing by farmers, who may use it for non-productive purposes such as household expenses, children’s education, or leisure activities. However, as time goes on (in long-run), farmers may have used it to invest in more productive farm businesses. This finding is consistent with Bahşi and Çetin (Citation2020) and Sogules and Nkoro (Citation2016).
Agricultural subsidies have a strong short-run positive relationship with agricultural productivity (AP). A 1% increase in agricultural subsidies increases AP by 0.05% in the short run. This is likely because farmers are unable to divert subsidies to non-agricultural uses in the short term. Li et al. (Citation2022) and Aragie et al. (Citation2016) also found that agricultural subsidies significantly increase farm productivity in the short run.
Similarly, employment in agriculture has a positive and significant short-run impact on agricultural productivity, in which 1% increase in employment in agriculture increases agricultural productivity by 4.01%. This finding is consistent with Zhang and Diao (Citation2020), who found out that rising agricultural employment, has led to rapid growth in agricultural productivity.
Inflation has a significantly positive effect on agricultural productivity in the short term. For every 1% increase in inflation rates, agricultural productivity rises by 0.01%. This suggests that input costs change more slowly in the short term than in the long term, and therefore have less of an impact on agricultural productivity. In the short term, agricultural productivity may increase due to the need to increase yields to respond to rising prices. This finding is consistent with Catherine Mbah et al. (Citation2022).
Finally, the findings also reveal that foreign direct investment (FDI) has a negative short-term relationship with agricultural productivity (AP). This suggests that a 1% increase in FDI will result in a 0.01% decrease in AP in the short term. This finding is consistent with Malikane and Chitambara (Citation2018), who found a similar negative short-term relationship.
3.5. Diagnostic tests
To further confirm the robustness of the model, we test its stability. This requires the absence of autocorrelation, heteroscedasticity, and non-normality, as well as accurate model specification. For a robust econometric model, all test statistics should be expected to be negligible. The test results are shown in .
Table 6. Results of diagnostic tests.
The stability of the parameter estimates over time was assessed using the Cumulative Sum (CUSUM) test. This test detects systematic changes in the regression coefficients and is plotted using the critical limits of 5% levels of significance. If the plot remains within the critical boundaries, there is no evidence against the null hypothesis that all the coefficients are stable. The CUSUM test results are shown in . The findings demonstrate that there is no coefficient instability because the plot of the CUSUM statistics falls within the critical bands of the 5% confidence intervals of parameter stability (Pesaran et al., Citation2001).
4. Conclusions and policy implications
This study investigated the effects of agricultural credit and subsidies on agricultural productivity in Ethiopia from 1990 to 2021 using ARDL model. The studies short data life span, however, was a limitation brought on by the lack of available data. The findings reveal several important conclusions and policy implications.
4.1. Conclusion
The study found that agricultural credit initially had a negative impact on productivity but showed positive effects in the long run, while subsidies had a positive effect in the short run but a negative effect in the long run. These imply the need for the government to create better access to agricultural financing and offer subsidies only during short-run periods, gradually reducing them in the long run to avoid retarding productivity.
4.2. Policy implications
Several policy implications can be derived from the result. First, the government should design and implement policies that improve access to agricultural financing to promote long-run improvements in productivity. Second, credit allocation should be monitored to ensure that it is used for agricultural purposes and not diverted to non-agricultural objectives. Third, collaboration with financial institutions is crucial to develop specialized finance techniques for farmers and address challenges in obtaining credit for agriculture. Fourth, subsidies should be provided consistently and without interruption in the short run; however, in the long run, the government should emphasize on building the capacity of farm household to be self-reliance rather than continue to subsidize them.
One of the limitations of the study is that it focused on the effects of agricultural credit and subsidies on agricultural productivity. Nevertheless, there are other factors influencing agricultural productivity. Hence, future studies should examine the influence of public investment in agriculture on agricultural productivity.
Author contribution
All authors in this paper have variously contributed to the writing and editing of the manuscript. The manuscript was produced, examined, evaluated and the final report was written by the first author -Lemane Gebeyehu. The second author - Amsalu Bedemo participated significantly in the analysis and oversaw, reviewed, and provided feedback on the draft manuscript.
Acknowledgment
Not applicable.
Disclosure statement
There are no conflicts of interest between authors.
Data availability
Data available on request from the authors.
References
- Akinrinola, O. O., & Okunola, A. M. (2020). Performance analysis of Nigerian agricultural credit guarantee scheme: Bounds test approach to cointegration. Journal of Development and Agricultural Economics, 12(2), 1–11.
- Alemu, F. M. (2022). Amplification of agriculture factor productivity, food price and exchange rate on societal welfare spiralling in Ethiopia. Heliyon, 8(9), e10675. https://doi.org/10.1016/j.heliyon.2022.e10675
- Aragie, E. A., McDonald, S., Ferrari, E., & Dudu, H. (2016). Investment in agricultural productivity vs rural commercialization: Which way to rapid poverty reduction in Ethiopia?
- Badu, D. B., & Lee, Y. (2020). Government agricultural subsidy programmes impact on rice production in Ghana from 2005 to 2018. African Journal of Food, Agriculture, Nutrition and Development, 20(05), 16278–16289. https://doi.org/10.18697/ajfand.93.19545
- Bahşi, N., & Çetin, E. (2020). Determining of agricultural credit impact on agricultural production value in Turkey. Ciência Rural, 50(11), e20200003. https://doi.org/10.1590/0103-8478cr20200003
- Befikadu, A. T. (2022). An Empirical Analysis of the Effects of Population Growth on Economic Growth in Ethiopia Using an Autoregressive Distributive Lag (ARDL) Model Approach. Journal of the Knowledge Economy, 1–22.
- Chaiya, C., Sikandar, S., Pinthong, P., Saqib, S. E., & Ali, N. (2023). The impact of formal agricultural credit on farm productivity and its utilization in Khyber Pakhtunkhwa, Pakistan. Sustainability, 15(2), 1217. https://doi.org/10.3390/su15021217
- Chandio, A. A., Akram, W., Sargani, G. R., Twumasi, M. A., & Ahmad, F. (2022). Assessing the impacts of meteorological factors on soybean production in China: What role can agricultural subsidy play? Ecological Informatics, 71, 101778. https://doi.org/10.1016/j.ecoinf.2022.101778
- Catherine Mbah, C., Orjime, S. M., & Mgbemena, E. M. (2022). Agricultural productivity, food prices and inflation in Nigeria.
- Cordonnier, V., Covarrubias, K. A., De la, O., & Campos, A. P. (2022). Agricultural interventions and food security in Ethiopia–What is the role of adjusting livelihood strategies?.
- Degu, A. A., & Bekele, D. T. (2019). Macroeconomic determinants of total factor productivity and its trend in Ethiopia. International Journal of Research in Business and Social Science (2147- 4478),), 8(6), 219–228. https://doi.org/10.20525/ijrbs.v8i6.553
- Danish, M. H., Tahir, M. A., Azeem, H. S. M., & Anwar, M. (2017). Impact of Agriculture Subsidies on Productivity of Major Crops in Pakistan and India.
- Elias, H. (2019). Impact of credit constraints on agricultural productivity in the face of climate variability. Ethiopian Journal of Economics, 28(2), 27–62.
- Engida, B. T. (2022). Bank credit to agricultural sector and its productivity in Ethiopia. Journal of International Trade, Logistics and Law, 8(2), 168–182.
- Florence, N., & Nathan, S. (2020). The effect of commercial banks’ agricultural credit on agricultural growth in Uganda. African Journal of Economic Review, 8(1), 162–175.
- Gebeyehu, L., Emana, B., & Mitiku, F. (2020). Impact of agricultural credit on maize productivity among smallholder farmers in Hababo Guduru district. Oromia, Ethiopia. Ethiopian Journal of Applied Science and Technology, 11(1), 23–35.
- Ghouse, G., Khan, S. A., & Rehman, A. U. (2018). ARDL model as a remedy for spurious regression: problems, performance and prospectus.
- Li, C., Sha, Z., Sun, X., & Jiao, Y. (2022). The effectiveness assessment of agricultural subsidy policies on food security: Evidence from China’s poverty-stricken villages. International Journal of Environmental Research and Public Health, 19(21), 13797. https://doi.org/10.3390/ijerph192113797
- Malikane, C., & Chitambara, P. (2018). Foreign direct investment, productivity and the technology gap in African economies. Journal of African Trade, 4(1-2), 61–74. https://doi.org/10.1016/j.joat.2017.11.001
- Mekonen, E. K. (2020). Agriculture sector growth and inflation in Ethiopia: evidence from autoregressive distributed lag model. Open Journal of Business and Management, 08(06), 2355–2370. https://doi.org/10.4236/ojbm.2020.86145
- Mukasa, A. N., Simpasa, A. M., & Salami, A. O. (2017). Credit constraints and farm productivity: Micro-level evidence from smallholder farmers in Ethiopia. African Development Bank, 247, 1–40
- Ngalawa, H., & Derera, E. (2020). Agricultural production, employment and gender vulnerability: Covid-19 implications. African Journal of Governance & Development, 9(1.1), 200–225.
- Nguyen, L., Russ, J., & Triyana, M. (2023). The Effect of Agricultural Input Subsidies on Productivity.
- Nyiwul, L., & Koirala, N. P. (2022). Role of foreign direct investments in agriculture, forestry and fishing in developing countries. Future Business Journal, 8(1), 50. https://doi.org/10.1186/s43093-022-00164-2
- Ogbanje, E. C., & Salami, A. O. (2022). Impact of foreign direct investment on Nigeria’s agricultural sector (1981 to 2019). International Journal of Environment, Agriculture and Biotechnology, 7(4), 161–171. https://doi.org/10.22161/ijeab.74.18
- Onifade, S. T., Çevik, S., Erdoğan, S., Asongu, S., & Bekun, F. V. (2020). An empirical retrospect of the impacts of government expenditures on economic growth: new evidence from the Nigerian economy. Journal of Economic Structures, 9(1), 1–13. https://doi.org/10.1186/s40008-020-0186-7
- Osman Nabay, P. V., Bangura, R., Jr,., & Singh, D. R. (2022). Impact of agricultural investment in the economic growth of Sierra Leone (2001-2020. American Journal of Economics, 12(1), 1–5.)2022, pp. https://doi.org/10.5923/j.economics.20221201.01
- Pesaran, M. H., Shin, Y., & Smith, R. J. (2001). Bounds testing approaches to the analysis of level relationships. Journal of Applied Econometrics, 16(3), 289–326. https://doi.org/10.1002/jae.616
- Sogules, I. W., & Nkoro, E. (2016). Bank credits and performance of agricultural and manufacturing sectors in Nigeria, 1970-2013. Business, Management and Economics Research, 2(5), 90–95.
- Shakur, Z. H. (2020). Agricultural policies, agricultural production and rural households’ welfare in Ethiopia. Journal of Economic Structures, 9(1), 1–21.
- Urago, G. G., & Bozoglu, M. (2021). Literature Review on Farmers’ Agricultural Credit Access in Ethiopia. Anadolu Tarım Bilimleri Dergisi, 37(2), 301–316.
- Yang, T., Chandio, A. A., Zhang, A., & Liu, Y. (2023). Do farm subsidies effectively increase grain production? Evidence from major grain-producing regions of China. Foods (Basel, Switzerland), 12(7), 1435. https://doi.org/10.3390/foods12071435
- Zafeiriou, E., Karelakis, C., Martínez-Zarzoso, I., Galanopoulos, K., & Gkika, D. (2023). Economic development and pesticide use in EU agriculture: A nonlinear panel data autoregressive distributed lag approach. Agriculture, 13(9), 1693. https://doi.org/10.3390/agriculture13091693
- Zhang, Y., & Diao, X. (2020). The changing role of agriculture with economic structural change–the case of China. China Economic Review, 62, 101504. https://doi.org/10.1016/j.chieco.2020.101504