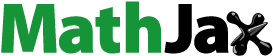
Abstract
Poultry production technologies adoption, such as improved Indigenous Chicken (IC) breeds and fabricated brooders, remain a viable option for enhancing IC productivity. However, the uptake of improved IC technologies remains low, especially in developing countries. This study investigated the adoption and intensity of the use of IC technologies in Machakos County, Kenya. The study adopted a cross-sectional survey to collect data from 374 households selected through a multi-stage sampling technique. A structured questionnaire and focus group discussion were employed, and data were analyzed using descriptive statistics and the Double-Hurdle model. The double hurdle results revealed that farmer experience, land size, non-farm activities, group membership, access to credit, awareness of IC technologies, and use of intensive/semi-intensive production systems were positively associated with IC technology adoption. However, household size was negatively associated with IC technology adoption. The intensity of use of IC technology was positively associated with gender, active labor, credit access, distance to the weather roads, and intensive production system. The study recommends that there is a need for agricultural stakeholders to promote membership in farmer associations and credit access, create awareness of IC technology, and improve opportunities for non-farm activities to help improve the adoption and intensity of the use of IC technologies.
PUBLIC INTEREST STATEMENT
The integration of improved Indigenous Chicken (IC) technologies plays an essential role in bridging the existing demand for white meat in developing nations. The present popularity of the indigenous chicken is due to the ease of feed conversion, the organic nature of production, scavenging ability, and delicious products. Despite the increased demand, the rate of uptake of the improved IC technologies remained low. The findings underscore the crucial role of human-specific, economic, and institutional factors in determining the decision and intensity of IC technologies in the arid and semi-arid lands of Kenya. The focus should be on targeted interventions such as programs suited to the requirements of the region’s farmers, increasing access to credit and extension services, and campaigning for supporting government policies and programs that stimulate the use of improved IC technologies.
REVIEWING EDITOR:
1. Introduction
The demand for indigenous chicken (IC) products, particularly meat and eggs, has surged in recent decades. This increase is attributed to factors such as population growth, increased per capita income, the proliferation of food service outlets, urbanization, affordability of poultry meat, and health awareness due to dietary change that is limiting the consumption of red meat (FAO, 2018; Gerber et al., Citation2013; Willett et al., Citation2019). For example, the United Nations projects that approximately 70% of the world’s population will reside in urban areas by 2050, hence the increased demand for food (United Nations, 2017). By 2031, meat production will rise to approximately 37 million metric tons (Mts) in carcass weight, equivalent to the current 339Mt, due to the rising global demand (OECD/FAO, Citation2022). Accordingly, by 2030, the global consumption of poultry meat is anticipated to constitute 47% of the total meat consumption, followed by pig, sheep, and bovine (OECD, Citation2022). The growth in poultry meat will be a primary driver towards increased meat production at the global level (FAO, 2022; Henchion et al., Citation2021). Thus, integrating strategies targeting increased poultry productivity remains paramount.
The poultry industry is witnessing global flock expansion by integrating improved genotypes, crossbreeding birds, and other advanced technologies to meet changing supply and demand trends (Sariyev & Zeller, Citation2023; FAO, 2022). In developing countries, poultry production is mainly done in rural set-ups on a small-scale, with the venture significantly contributing to human nutrition, social and cultural functions, and income generation due to the ease of sale of poultry to meet precautionary demand for money (FAO, 2013; Passarelli et al., Citation2022; Shapiro et al., Citation2017; Wong et al., Citation2017). According to Dumas (Citation2017), poultry production plays a significant role in women’s empowerment through the sale of live birds and their products, thus enabling women to meet some of the frequent expenses at the household level. Moreover, the poultry subsector provides a potential avenue to reduce greenhouse gas emissions, pests, and weeds thus contributing towards crop productivity among smallholder farms (Bosshardt et al., Citation2022). Investing in the poultry subsector by farmers would play a vital role in building resilience in rural communities prone to the effects of climate change (OECD, Citation2022).
In Kenya, the chicken population accounts for 98% of the poultry subsector, with the indigenous chicken (IC) accounting for 70% of the total chicken population (Kenya National Bureau of Statistics, Citation2019). Over 80% of IC farmers dwell in rural areas (Patel et al., Citation2016). Indigenous chicken production is a crucial source of employment, food security, income generation, active pest and insect control, a source of manure, and helps to promote gender empowerment among smallholder farmers (Alders et al., Citation2018; FAO, 2018; Smith et al., Citation2015; Wong et al., Citation2017). IC products are also preferred for their organic production, health consciousness, good taste, and leanness (Bwalya & Kalinda, Citation2014; Gakige et al., Citation2015). It is evident from the wide range of potential benefits attached to IC production that the enterprise can ultimately lead to sustainable livelihoods among smallholder farmers in rural areas. However, the smallholders have not fully embraced the implementation of improved indigenous chicken technologies. IC farmers still experience some challenges, such as unimproved breeds, increased chicks’ mortality, and poor mothering ability, resulting in low productivity (Atela et al., Citation2016).
Over the last few decades, agricultural stakeholders such as research institutions, universities, county governments, donors, and international funding agencies have been at the forefront of promoting and disseminating IC technologies such as improved IC breeds (for example, Karlo-Kienyeji, Sasso, Rainbow Rosters, and Kuroiler among others.) and fabricated brooders (hay-boxes, charcoal, paraffin, hot water and perforated pot brooders among other management technologies in Machakos County. The poultry breeds developed serve dual purposes and exhibit the potential for faster maturation (20 weeks to reach market weight) and the capability to lay around 230 eggs per hen per year (KALRO, Citation2018). Brooding technologies serve a crucial role in reducing chicks’ mortality by providing regulated warmth, preventing prospects of predation and feeding competition with adult birds, and hence increasing the survival rate (FAO, 2015; Glatz, Citation2013). These interventions are pertinent as they aim to improve performance and productivity to bridge the region’s demand and supply of IC products. Machakos County is characterized by spatial and temporal variability; hence, crop production is highly unpredictable (MoALF, Citation2017). The climate variability subjects a major proportion of the rural population to the food insecurity trap, hence the rise in poverty levels. As a result, IC production is a major economic activity practiced by over 62% of households to diversify sources of income and nutrition (KNBS, Citation2019). Therefore, improved poultry technologies are one of the integrated efforts by the stakeholders that anticipate reducing poverty levels to 30% from the current 43% by 2025 (Machakos County Integrated Development Plan, Citation2018; Economic Survey, 2020). Despite the positive contributions of IC, the sector lags in terms of performance and productivity due to the low adoption of improved IC technologies, such as improved IC and fabricated brooders.
Several empirical studies have investigated socioeconomic factors influencing the uptake of technologies in the poultry sector. For example, Teklewold et al. (Citation2006) analyze the determinants of adopting exotic breeds in Ethiopia. Using a Double Hurdle (DH) approach, the study found that family size, age, access to credit, and extension services influenced the decision to adopt exotic breeds. Farmers’ experience, the age of the household head, market problems, and expected benefits affected the intensity of adopting exotic breeds. Idio and Okoro (Citation2017) investigated the adoption of feed technologies in Nigeria and found that awareness, gender of household head, education level, and group membership influenced the decision to adopt. Using a regression analysis, Singha et al. (Citation2016) explored determinants of poultry farming technologies such as feeds, health care, and housing. Results revealed extension services, farm size, and farming as a primary occupation as determinants of adoption. Tamir et al. (Citation2015) evaluated the determinants of adopting improved exotic breeds among smallholder farmers in the Amhara region. The study documented inadequate supply of breeds, limited awareness, and lack of extension services as the main factors of low adoption. (Ithika et al., Citation2013) found that the enterprise’s flock size influenced the adoption of scientific poultry practices among broiler farmers in India. Accordingly, the findings by (Adetomiwa, Citation2020) revealed that awareness, access to credit, farm size, off-farm activities, cost, and perception influenced the decision to adopt artificial intelligence among poultry farmers. Factors such as education, land ownership, extension services, and credit influenced the extent of AI adoption among poultry farmers.
The literature review identifies a gap in research related to the adoption and intensity of the use of IC technologies, specifically in areas designated as Arid and Semi-Arid Lands (ASAL). Most existing studies have focused on the decision to adopt poultry technologies using single equation approaches, neglecting the nuanced factors influencing adoption and intensity of use. The double hurdle (DH) econometric framework is suggested as a more comprehensive method to analyze the adoption and intensity of the use of IC technologies. This framework allows for a detailed examination of whether the decision to adopt and the intensity of use are made jointly or separately, providing a more nuanced understanding compared to classic models like the Tobit model. There is a call for more empirical research specifically focused on the adoption and intensity of IC technologies among smallholder farmers in regions like Machakos County. Understanding the diverse socioeconomic settings, institutional factors, and experience levels of farmers is crucial for effectively managing IC production and designing appropriate interventions. Most studies on poultry adoption have focused on exotic breeds such as layers and broilers, while neglecting indigenous chicken. The study aims to fill this gap by identifying the determinants of IC technology adoption decisions and understanding what influences the extent or level of adoption among smallholder farmers in Machakos County, Kenya. The study contributes to the existing literature by employing a DH econometric framework to analyze the adoption and intensity of the use of improved IC technologies. By addressing the identified research gap, the study intends to provide actionable insights for designing appropriate interventions to support smallholder farmers in the region effectively.
2. Materials and methods
2.1. The study area
The study was conducted in Machakos County (), the lower eastern region of Kenya. The county lies between latitudes 0°45’ and 1°31’South and longitudes 36°45’ and 37°45’ East. The area has a total coverage area of 6,208.2 Km2. The county comprises nine (9) sub-counties, namely Athi River, Kalama, Kangundo, Kathiani, Machakos, Masinga, Matungulu, Mwala, and Yatta (KNBS, Citation2019). The population distribution in the county is estimated at 1,421,998, with 710,707 males and 711,191 females, respectively. The population density is 235 per Km2, with good road networks traversing across the county (KNBS, Citation2019). Agriculture is the main economic activity practiced in the county, with livestock dominating the sector (Machakos County Integrated Development Plan,). Indigenous chicken is the most preferred venture, and they dominate the livestock sector due to their resilience to harsh climatic conditions in the county (CIDP, Citation2018). The subsector is also anticipated to reduce the poverty level from 43% to 30% by 2030 (MoALF, Citation2017; ADP, 2020). However, the adverse effect of climate change has had a significant setback on agricultural production in the county (Machakos County Climate Smart Profile, 2017). The temperature fluctuates between 18 °C and 29 °C with a bimodal rainfall pattern that reaches a maximum of 1250 mm and a minimum of 500 mm (CIDP, 2018). The county’s main agroecological zones (AEZ) resemble arid and semi-arid land with very low to moderate precipitation amounts and adequacy (CIDP, 2018).
2.2. Data and sampling procedure
The study adopted a multi-stage stratified sampling technique. Purposive sampling was applied to select Machakos County, one of Kenya’s leading indigenous chicken-producing counties in the Lower Eastern region. Approximately 62% of households in Machakos County produce indigenous chicken (KNBS, Citation2019). The second stage used stratified random sampling to select the sub-counties within Machakos County: Kangundo, Mwala, Yatta, and Machakos. The four sub-counties are categorized under Lower Midland - Agro-Ecological Zones, which portrays high potential and suitability for IC production (KNBS, Citation2019: MoALF, Citation2017). Subsequently, the study used a simple random sampling to obtain a sample of 374 households. The study adopted a structured questionnaire, checklist, key informant interviews, focused group discussion, and direct observations to collect the data from the respondents between April and June 2023.
2.3. Theoretical framework for Technological adoption of IC technologies
Adoption refers to the decision to apply and continue using a technology (Rogers and Shoemaker , Citation1971). The decision to adopt an agricultural technology is extended based on the assumption of farmer utility maximization theory (Adesina & Zinnah, Citation1993). The framework has guided many recent studies to evaluate decisions on the uptake and effect of improved technologies in various contexts (Ahmed et al., Citation2017; Khonje et al., Citation2015; Manda et al., Citation2019). This study utilized the random utility framework to model the household’s decision to adopt improved IC technologies. Given the different adoption constraints, households adopt a technology set y at time t, to maximize the utility (Max uit = f(xit) where x represents the set of independent variables affecting the adoption decision of the farmer. Let R* denote the difference in the expected utilities between the adopters E(uit1) and non-adopters E(uit0) of improved IC technologies. The farmer will decide to choose to adopt an improved technology only if the utility derived from adoption supersedes the utility of the non-adopters (R*= {E (uit1) – E (uit0 > 0) or (uit1) > uit0}. It is worth noting that the two utilities are non-observable, thus the net benefit R*, which is a latent model predicted by observed and unobserved variables, can be expressed as;
(1)
(1)
Where R is the observable variable that equals 1 if the IC farmer adopts a single technology or set of technologies and 0, otherwise; β denotes the estimable parameters; X is a vector of explanatory variables such as socio-economic characteristics, and ɛi is the stochastic term.
2.4. Econometric model specification for Technological adoption among smallholder
This study adopted a Double Hurdle (DH) approach to quantify the intensity of the adoption of IC technologies. The approach emphasizes two tiers that farmers face during the adoption process. The adoption decision is simulated in the first tier while adoption intensity is determined using the second tier (Cragg, Citation1971). Berhanu and Swinton (Citation2003) hypothesize the two tiers (decision and intensity) have a connection. Similar studies have utilized the framework to analyze this hypothesized association (Anik and Salam, Citation2015; Awotide et al., Citation2016; Okoffo et al., Citation2016).
The two tiers are denoted by the following EquationEquations (2)(2)
(2) and Equation(3)
(3)
(3) as follows;
(2)
(2)
(3)
(3)
Where Wi = {1, if Wi* >0; 0 if Wi* ≤ 0} and Di = {D*, if Di> 0 and Wi*> 0; 0, if otherwise}. Wi* - the latent variable that makes the value of 1, if the IC farmer adopts; 0 otherwise; Zi—socioeconomic drivers of decision to adopt; Xi—explanatory variables considered to model the intensity of technological adoption. The study employed an average fractional index which is an aggregated adoption of different dimensions of agricultural technology to quantify intensity, and Ui and Vi—deterministic terms.
2.5. Estimation procedure for Technological adoption among smallholder IC farmers
According to Wooldridge (Citation2002), the truncated model distribution consists of the Tobit model that enables tests of the Tobit hypothesis. The model assumes joint decisions on use and intensity of adoption. The study, therefore, compared the Tobit model results alongside the DH model results based on the likelihood ratio tests, denoted as (LR). The idea was to establish whether the two models differed significantly and which was superior when measuring adoption. The maximum log-likelihood test technique is denoted as:
(4)
(4)
Where Σ/0 = summation over the zero observations; Σ/+ denotes summation over positive observations, and are the standard normal cumulative distribution functions and probability distribution functions, respectively. This study hypothesized that the adoption decision and intensity of IC technologies is made separately, and that a feedback effect exists from the 2nd tier (on intensity) to the 1st tier (on decision). The double hurdle assumes that the disturbance terms in EquationEquations (5)
(5)
(5) and Equation(6)
(6)
(6) are independent. As a result of this, the random and independent distribution is expressed by Vi ∼ N (0, 1) and Ui ∼ N (0, σ^2), respectively. The Tobit model, on the other hand, can be written as;
(5)
(5)
Accordingly, the Likelihood ratio statistic as proposed by (Greene, Citation2000) was computed as shown in EquationEquation (6)(6)
(6) ;
(6)
(6)
Where LT = Tobit model likelihood; LP=Probit model likelihood; LTR=Truncated Model likelihood; and k = the explanatory variables in the equation. Based on this, the test hypothesis is denoted as;
(7)
(7)
In this study, H0 was rejected on a prespecified significance level if Г > 𝜒2k. The results of the likelihood ratio test between the Tobit and DH model are presented in (Annex 1). Test statistics was Г= 46.446 and above the tabulated value [χk2 (15) =24.996] at 5% level of significance. Thus, the double hurdle fitted the data better in comparison to the Tobit Model. This implied that a farmer’s decision to adopt IC technologies and the intensity of the use of IC technologies were two independent processes.
2.6. Measurement of variables and the hypothesized effect
The variables listed above () empirically show the relationship between the dependent variable (Improved IC breeds, and brooders) and the listed explanatory variables.
Table 1. Definitions of variables and the expected signs for the double hurdle model.
3. Results and discussion
3.1. Descriptive statistics
presents the descriptive statistics of the smallholder farmers sampled in the study area. The results capture the farmers’ characteristics based on their IC technology adoption status (adopters versus non-adopters). Approximately 54% of the sampled households had adopted at least one improved IC technology (Annex 2). The results indicate that the average family size of the adopters and non-adopters of IC technologies was 3.9 and 4.2, respectively. Family size is considered a proxy for labor availability, specifically for labor-intensive IC technologies. Generally, IC production is a capital-intensive enterprise, hence the importance of labor. On average, adopters of IC technologies had 15.93 years of experience in poultry farming, compared to 12.73 years of experience for their non-adopter counterparts, with the difference being significantly different at the 1% significance level. A higher level of production experience among IC technology adopters enables them to evaluate the risks and economic value associated with IC technology, hence increasing the likelihood of its adoption. Adopters of IC technologies owned 2.88 acres of land, compared to their non-adopters, who owned 2.01 acres of land, and this variable was statistically significant at the 1% significance level. The farm size signifies that IC adopters had large farm sizes and were able to devote some of their land to IC production, given the potential of indigenous chicken to scavenge.
Table 2. Descriptive statistics for the continuous variables.
The results revealed that adopters of IC technologies had an average of 37 IC birds compared with their non-adopters, who owned 25 birds on average, and this variable was significantly different at the 1% significance level. The flock size points to a possible link between adoption status and the number of birds owned by the adopters of IC technologies. The findings show that adopters of IC technologies were generally aware of the existing IC technologies. The awareness score of the IC technology adopters was 1.93 compared to the 1.41 of their non-adopter counterparts, and the score was significantly different at the 1% significance level. The years of experience signify that adopters have gained greater knowledge about the existence and successful use of IC technologies, lowering the uncertainty associated with them. The distance to the tarmac road measured in walking minutes, was significant at the 10% significance level. The results revealed that adopters of IC technologies covered slightly longer distances compared to non-adopters, with adopters walking on average for 21 minutes compared to 16.75 minutes for non-adopters. The statistical difference in the above continuous variables suggests possible associations between household successes in IC technology adoption and these variables (). The results show that the farmers owned 1.8 herds of livestock, 2.8 workers, had at least 3.04 contacts with the extension agents, and had 11.87 years of schooling, respectively. However, these variables were not significantly different among the adopters and non-adopters of IC technology.
presents descriptive statistics for the dummy variables used in the empirical analysis. Participation in non-farm activities was higher for adopters (52.2%) compared to non-adopters (37.4%). Non-farm activities participation enabled the adopters to overcome the financial constraints that discouraged the adoption process. Membership in an agricultural association showed that participation was higher among the adopters (43.8%) than the non-adopters (22.2%). Association membership enabled the IC adopters to build social capital through trust and knowledge exchange on IC technologies. The proportion of household heads who accessed credit for poultry production was higher for adopters (54.2%) than non-adopters (38%). Access to credit by the adopters reduced the liquidity constraints associated with IC technology adoption.
Table 3. Descriptive statistics for binary and categorical variables (chi2-test).
Similarly, the results revealed that training on poultry production in the last year of research was higher for adopters of the IC technologies (43.8%) compared to the non-adopters (22.8%). Training on poultry production implied that the adopters had amassed more knowledge on the benefits of IC technology, thus stimulating the adoption. Moreover, higher proportions of households with title deeds were observed among the adopters (65.5%) than non-adopters (44.4%). Title ownership is considered a proxy of wealth and serves as collateral for technology adoption. This implies a link between IC technology adoption and land ownership by the households. The result predicts a significant relationship between the production system and the adoption of IC technology. The findings show that the majority of non-adopters (77.7%), preferred free-range systems over other poultry production systems. Free-range systems which are characterized by low input investment were utilized as the reference category in this study. This shows that a considerable proportion of non-adopters chose a farming system with few inputs. The proportion of use of both semi-intensive (SIS) and intensive system (IS) was higher for adopters (38.8 and 14.3%, respectively), compared to non-adopters (19.9 and 0.02%). Semi-intensive (SIS) and intensive systems occur in a controlled environment, which is why they are preferred by IC technology adopters for control and trial basis.
3.2. Determinants of adoption and intensity of use of IC technologies
The results of the determinants of adoption and intensity of use of IC technologies are presented in . The results of the first hurdle indicate that household size, experience in poultry production, cultivated land size, participation in off-farm activities, membership to agricultural associations, access to credit, awareness of IC technologies, semi-intensive and intensive systems of production were statistically significant variables influencing the probability of adoption of poultry production technologies in the study area. The marginal effects estimates depict changes in the likelihood of adoption of poultry technologies for an additional unit increase in the explanatory variables. On the other hand, the truncated results revealed that the gender of the household head, active labor force, access to credit, distance to the weather roads, and intensive system of poultry production had a positive and significant effect on the intensity of use of IC technologies. An average fractional (IC) index quantifies the intensity of adoption of the IC technologies, which ranges from 0 to 1.
Table 4. A double hurdle (probit + truncated model) versus the Tobit model estimates.
The household size variable was statistically significant at the 5% significance level, indicating its importance in influencing farmers’ decisions to adopt IC technologies. The variable implied that an increase in household size by one member led to, on average, a 3.32% decrease in the probability of farmers adopting IC technologies, suggesting that larger households are less likely to adopt these technologies. The plausible explanation is that farmers with larger households may prioritize using their revenue to support their family’s financial needs rather than investing in IC technologies. Passarelli et al. (Citation2022) indicate that in such cases, the decision to adopt IC technologies may be motivated by a precautionary, transactional or speculative demand for money, where meeting the family’s needs takes precedence. Second, IC production among smallholder farmers may be less labor-intensive, considering the number of birds per household, implying that factors other than labor requirements influence adoption decisions. Previous studies have reported a negative association between household size and adoption decisions (Tran et al., Citation2019; Okoffo et al., Citation2016; Awotide et al., Citation2016; and Uhunamure et al., Citation2019). The study contrasts with the findings of Abadi et al. (Citation2018), which reported a positive relationship between household size and the adoption of exotic chicken breeds in Ethiopia. The household size variable thus illustrates how complex and possibly context-dependent the relationship between family size and the uptake of technology is in different scenarios.
Participation in an agricultural group is associated with a higher likelihood of adopting IC technologies. The variable is significant at the 5% significance level. The marginal effect results indicate that being a member of a farmer’s association increases the probability of adopting IC technology by 9.2%. The justification in support of the finding is that farmers in an agricultural association benefit from increased exposure to new technologies through information exchange and dialogue, hence facilitating peer learning. A farmer can therefore analyze the various benefits and risks associated with the new technology. Group membership also augments access to institutional support such as credit and inputs, which promotes technology adoption (Mango et al., Citation2020; Mutenje et al., Citation2016). Agricultural associations have a role in changing farmers’ attitudes towards technology adoption. The finding is in line with existing theory and empirical evidence from previous related studies. Specifically, Tasie et al. (Citation2019) investigated the adoption of climate change adaptation techniques among poultry farmers in Nigeria, while Nanyeenya et al. (Citation2013) conducted research on the technology adoption of village chicken producers in Uganda. The study affirms that farmers’ adoption of technology is facilitated by belonging to agricultural groups, a conclusion that is evident in the existing literature.
Farmer access to agricultural credit significantly and positively affects the intensity and probability of adoption of IC technologies at 5% significance level. The marginal effects of this variable showed that farmers with access to credit increased the likelihood of adopting IC technologies and their intensity of use by 12.67% and 8.5%, respectively. The result implies that access to credit enables farmers to procure improved IC technologies and other complementary inputs, thus bridging the financial barriers attributed to the technology adoption process. Interested farmers who face a financial gap will be willing to adopt new IC technologies once provided with credit to bridge that gap. Access to credit, thus helps households overcome budget limitations associated with technology adoption by stimulating households’ risk-bearing capacity. This result is consistent with previous studies, such as Mulungu and Kangogo (Citation2022), who found a positive relationship between access to credit and adoption of IC technologies in Eastern Kenya; Khan et al. (Citation2022), on the adoption of poultry technology in Pakistan; and Teklewold et al. (Citation2006), on the adoption of poultry technology in Ethiopia.
Results also demonstrated a positive relationship between farm size and the adoption of IC technologies. Specifically, adding one acre of land increased the probability of IC technology adoption by 3% while holding the other factors constant. This implies that the larger the farm the more likelihood of adopting the IC technologies. A farmer is always willing to utilize land for improved IC technologies given their ability to scavenge for feeds. Additionally, farmers with larger farms can dedicate part of their land to experiment with new IC technologies. Large tracts of land constitute part of physical capital endowment, thus incentivizing farmers to adopt IC technologies. This finding is consistent with prior research by Quddus (Citation2022), who found a positive relationship between farm size and the adoption of technological innovations of livestock in Bangladesh. However, the result contradicts Sariyev and Zeller (Citation2023), who reported a negative relationship between farm size and the decision to adopt crossbred poultry in Ethiopia. These differences in results could be due to differences in contextual factors and institutional support, which differ between different agricultural sectors and technologies.
The results also revealed a positive association between farmers’ participation in off-farm activities and the adoption of IC technologies, at the 5% significance level. The marginal effect results indicate that farmers who benefitted from off-farm income were 13.1% more likely to adopt improved IC technologies than their non-adopter counterparts who do not engage in off-farm activities. The plausible justification is that engagement in off-farm activities helps reduce farmer liquidity constraints, thus increasing their ability to adopt IC technologies. The income generated from non-farm engagement is a proxy for household financial capacity to invest in agricultural technologies. A farmer can afford the improved breeds and brooders, among other required inputs. In the context of this study, income diversification plays a crucial role in increasing farmers’ capacity to afford the improved IC technology. Farmer participation in non-farm pursuits encourages the adoption of labor-saving technologies like IC technologies, hence enhancing agricultural productivity and efficiency. The result of this study is consistent with Wondmeneh et al. (Citation2014), who also found a positive relationship between participation in on-farm activities and the adoption of exotic chicken breeds among farmers in Horro and Ada districts, Ethiopia. However, this contradicts with findings from other studies, namely - (Ng’ombe et al., Citation2014), Issahaku and Abdulai (Citation2020), and Awotide et al. (Citation2016), which found a negative association between off-farm activities and technology adoption.
The study also revealed a positive correlation between farmers’ experience in poultry production and the adoption of IC technologies, with a statistical level of 5%. The result suggests that an additional year of experience increased the probability of adoption of IC technologies by 0.7%. The finding suggests that farmers with more years of experience in poultry production are more likely to adopt IC technologies. The possible justification is that household heads with more years of experience are more likely to have accumulated knowledge and skills of IC technologies in terms of risks, challenges, and benefits. Their knowledge of different technology solutions enables them to evaluate and justify implementing new practices more successfully. According to Kaliba et al. (Citation2018), farmers often require sufficient time to make informed decisions about shifting from old to new agricultural technologies. Therefore, the accumulated experience over the years enables farmers to evaluate the potential advantages of adopting IC technologies and curb against the risks associated with technology dis-adoption. This finding is in tandem with prior research by Tsadik et al. (Citation2015) and Tadelech & Mesfin (Citation2020), who investigated the adoption of village poultry technologies and improved chicken packages among smallholder farmers in Ethiopia. These studies showed a significant correlation between the technology’s farmers used to raise chickens and their degree of expertise. Thus, there is a need to empower farmers through knowledge transfer on IC technologies, which will play a role in persuading farmers to accept new technologies.
The study also found a positive and statistically significant relationship between awareness and the adoption of IC technologies at the 1% significance level. The marginal effect revealed that farmers’ awareness of either of the IC technologies increased the probability of adoption by 26.1%. The relationship implies that farmers who have heard or read about IC technologies during the preceding years are better informed on their embedded advantages, thus increasing the likelihood of adoption. Similarly, awareness reduces the perceived risks and uncertainties associated with adoption, which allows farmers to make more objective assessments of IC technologies, leading to a higher probability of adoption. According to Kaliba et al. (Citation2018), awareness is a pre-condition for informing the adoption of a technology. Various channels such as media, mobile applications, and contact with researchers, extension officers, family and friends have been identified as a source of promoting awareness regarding technology (Uhunamure et al., Citation2019). Through various platforms, farmer awareness is enhanced, thus increasing the probability of adopting IC technologies that help to bridge the unmet demand for IC products, such as meat and eggs. This result is in line with the previous research by Campbell et al. (Citation2018) and Mwololo et al. (Citation2020), which also reported a positive association between awareness and adoption of different IC technologies.
The results also showed a positive and significant association between the type of production system and the adoption of IC technologies. The marginal effect showed that the semi-intensive and intensive systems increased the probability of adoption of IC technologies by 15.38 and 30.56%, respectively, compared to the free-range production system. However, only the intensive system qualified for the second hurdle, affecting the intensity of the use of IC technologies by 14.32%. The positive relationship depicts the preference by the farmers for the adoption of the technologies was dependent on the type of production systems. Farmers using the intensive production system were more inclined to adopt genetically improved breeds and brooders among other interventions. Similarly, farmers with non-free-range systems tend to separate their flocks, given the higher levels of confinement and management, necessitating the integration of IC technologies such as improved breeds and brooders to optimize production efficiency. Alders et al. (Citation2018) emphasize the importance of rearing improved IC breeds or intermediate crossbreeds under the intensive system to improve performance and increase income, ultimately leading to increased profit margins.
The gender of the household head significantly affected the intensity of adopting IC technologies at a 5% statistical level, with male-headed households showing a higher intensity of use than female-headed households. Results showed a 6.95% increase in the intensity of use of IC technologies. The finding suggests that male-headed households are more likely to allocate resources to IC technologies than their female-headed counterparts. This finding is fascinating since studies have linked IC production to women-dominated investment (Alemayehu et al., Citation2018; Nduthu, Citation2015). However, the possible justification includes constrained economic status, derailed access to inputs and credit, and lack of information regarding IC technologies due to social position among female-headed households in rural settings (Nkhoma et al., Citation2017). Based on the traditional roles, Fara (Citation2015) reported gender as a significant setback in adopting agricultural technologies in developing countries. Men make production decisions, creating opportunities for them to control productive farm resources. The factors above of production are crucial and inform the adoption process. This result is in line with Ansah and Frimpong (Citation2015), who found a positive relationship between the gender of the household head and the intensity of the use of technology.
The study found that the number of contacts with extension significantly and positively affected the intensity of IC technology usage among farmers. Access to farmer’s training led to a substantial increase of IC technology usage by 17.59%. A reasonable justification is that access to training and frequent interactions with extension providers contribute to a better understanding of IC technologies. The interactions likely provided farmers with comprehensive information and guidance on adopting and utilizing IC technologies. Extension services serve as a catalyst for technology adoption among smallholder farmers. Through the promotion of modern agricultural technologies and providing support and guidance, extension service providers facilitate the adoption process. According to Asfaw et al. (Citation2012), farmers have become more progressive and are more likely to experiment with new technologies due to frequent visits by extension agents. Farmers’ human capital also tends to be strengthened when exposed to novel information on agricultural technologies by quality extension agents (Khonje et al., Citation2015; Teklewold et al., Citation2017). In the context of this study, farmers’ contact with extension personnel has strengthened pathways toward access to better information, and advising on IC technologies will enhance farmers’ integration into their IC production system. Ngeno (Citation2018) confirmed that shared information helps farmers mitigate technologically associated risks and uncertainties. Belachew et al. (Citation2020) also assert that extension services are diligent in raising awareness of poultry technologies and the likelihood that a farmer may choose to intensify their adoption. This finding aligns with those reported by Singha et al. (Citation2016), who found that extension agents had a positive and significant influence on the extent of adoption of poultry technologies among farmers in the North Eastern States of India.
The active workforce was a statistically significant variable at 5%, indicating its importance in influencing the intensity of IC technology use. There is a positive relationship between the number of active workforce members and the intensity of IC technology use. Specifically, an increase in the workforce by one person is associated with a 3% increase in the intensity of IC technology use. The results underline how important family labor is to rural households, especially when it comes to capital replenishment for IC production. It would seem from this that families with more involved family members are more suited to accept and employ IC technology. Households with a higher proportion of members participating in non-farm engagements may generate additional income, which can be used to facilitate the procurement of IC technologies. This implies that diversified sources of income can support technological advancement in rural areas. The intensity of use of IC technologies is perceived to be relatively labor-intensive, emphasizing the importance of having a large number of active family members involved in the production process (Gregory and Sewando, Citation2013). Adjin et al. (Citation2020) support that active workforce members increase the likelihood of households participating in farmers’ groups where information about agricultural technologies, including IC technologies, is shared. This highlights the role of social networks and collective learning in technology adoption. Previous studies by Gregory & Sewando (2013), Adjin et al. (Citation2020), Gebre-Selassie and Bekele (Citation2014), Teshome and Tegegne (Citation2020), and Beshir (Citation2014) have also found that an active labor force is a driver of agricultural technology adoption and intensity of use.
Distance to tarmac roads had a positive and significant relationship at a 1% statistical level. The model results revealed that as the distance to all-weather roads increases by one unit (kilometre), the intensity of the use of IC technologies increases by 0.14% keeping all the other factors constant. The possible justification is that households in the study area remain sparsely distributed, with IC production being predominant in remote areas. Due to the long distances to roads, farmers are more likely to adopt nearby technologies like fabricated brooders made of timber or other local materials to reduce production costs. Another plausible justification is that the farmer may aim to reduce transportation costs by procuring more improved IC breeds, which would lead to reduced visits to nearby towns located along tarmac roads. Breeding stock may be the primary emphasis of some IC farmers’ bird sales. Reducing transportation expenses by remaining closer to producing locations could be the focus due to limited supply in remote areas. The findings align with studies conducted by Beshir et al. (Citation2022) in North Western Ethiopia and Awotide et al. (Citation2016) in Nigeria, indicating a consistent trend across different regions.
4. Conclusion and policy recommendation
This article aimed to investigate the socioeconomic and demographic aspects influencing smallholder farmers in Machakos County, Kenya, to embrace IC technologies (improved IC breeds and brooders). The adoption rate was relatively low for each technology IC breeds and brooding technologies). However, a majority had accepted at least one of the two categories of technologies, notwithstanding the low adoption rate. The T-test results indicated that the following household characteristics—family size, expertise in IC production, farm size, awareness level, distance to the tarmac road, and flock size—showed significant differences between the adopters and non-adopters. Chi-square tests revealed significant differences for the following dummy variables: ownership of a title deed, group membership, availability to credit, training in poultry production, and kind of IC production system. Specifically, a Double Hurdle (DH) approach was deemed more suitable than the Tobit Model. This suggests that farmer decisions about the adoption and the intensity of the use of IC production technology were made independently. This led to the null hypothesis rejection that the farmer decisions on adoption and intensity of the use of IC technology were joint.
Consistent with the empirical evidence from the literature on adoption, farmer’s experience in IC production, household size, land size, non-farm activities, group membership, credit availability, awareness, and use of intensive and semi-intensive production systems were important determinants of IC adoption decisions. Conversely, the gender of the household head, labor force participation, access to credit, distance to the weather roads, and intensive system of poultry production were significant drivers of IC technology intensity of the use. Several recommendations are made in light of the findings to enhance the acceptance and usage of IC technologies. First, agricultural stakeholders should actively encourage the formation of farmer associations at the grassroots to strengthen social capital in order to enhance information sharing on improved IC technologies. Second, financial institutions should also provide more preferential interest rates on credit to enable smallholder farmers to purchase IC technologies and other inputs such as housing materials, feeds, and vaccines. Finally, the results emphasize the need for practitioners and agricultural stakeholders to promote membership in farmer associations and credit access, create awareness of IC technology, and improve opportunities for non-farm activities to help improve the adoption and intensity of the use of IC technologies. The strategy would be to improve the training materials and content that give farmers more access to knowledge. More farmer training programs will thereby raise awareness, which will help boost the uptake and usage of the IC technologies.
Ethics approval and consent to participate
The data collection approach adhered to the ethical principles of research. Participating in this research was voluntary, with informed consent obtained from the farmers before conducting the interviews. The farmers were aware of their involvement and rights in the study. The National Commission for Science, Technology, and Innovation, Kenya, approved this study under License No: NACOSTI/P/23/23976.
Authors’ contributions
Christopher Njuguna Kamau generated the idea and study design, collected data, carried out data analysis, and wrote up the first draft of the manuscript. Dr. Eucabeth B. Majiwa, Prof. Lucy W. Kabuage, and Dr. Geoffrey O. Otieno supervised data collection, provided constructive suggestions and statistical assistance, and read, edited, and revised the manuscript.
Acknowledgments
The authors are grateful to the enumerators who helped collect the data used in this paper and all the farmers who participated in the survey. Many thanks to the anonymous reviewers for their constructive feedback that helped improve the quality of this paper.
Disclosure statement
The authors declare no conflicts of interest in writing this paper. The opinions expressed in this paper are those of the authors and do not reflect the position of the funding agency.
Data availability statement
The data used for this study is available from the corresponding author upon request.
Additional information
Funding
Notes on contributors
Christopher N. Kamau
Christopher N. Kamau is a Tutorial Fellow in Kenyatta University and a PhD candidate at Jomo Kenyatta University of Agriculture and Technology (JKUAT). He has an extensive research experience with; Kenya Climate Smart Agricultural Projects (KCSAP); Kenya Agricultural Productivity and Agribusiness Project (KAPAP); The Research Foundation for the State University of New York (SUNY); African Agricultural Technologies Foundation (AATF); his professional interest focuses on; agricultural interventions and impact assessment.
Eucabeth B. Majiwa
Eucabeth Majiwa is a Lecturer in the Department of Agricultural and Resource Economics, Jomo Kenyatta University of Agriculture and Technology (JKUAT) with over fifteen years’ experience in agricultural economics and agribusiness development.
Geoffrey O. Otieno
Geoffrey Otieno is a Lecturer in the Department of Agricultural and Resource Economics, JKUAT with over ten years’ experience in agricultural economics and agribusiness development.
Lucy W. Kabuage
Lucy. W. Kabuage is an Associate Professor in the Department of Animal Science, Kenyatta University with over thirty years of experience in agricultural research and development and a specialist in animal production.
References
- Abadi, T., Gezahegn, M., & Teklehaimanot, A. (2018). Assessment of factors affecting adoption of exotic chicken breed production in North Western Zone of Tigray, Ethiopia. International Journal of Livestock Production, 9(11), 1–16. https://doi.org/10.5897/IJLP2017.0392
- Adesina, A. A., & Zinnah, M. M. (1993). Technology characteristics. Farmers’ perceptions and adoption decision: A Tobit model application in Sierra Leone. Agricultural Economics, 9(4), 297–311. https://doi.org/10.1111/j.1574-0862.1993.tb00276.x
- Adetomiwa, K. ( 2020). Adoption and utilization of artificial intelligence (Ai) in poulty production: Evidence from smart agricultural practices in Nigeria. SSRG InternationalJournal of Agriculture & Environmental Science, 7(3), 46–54. https://doi.org/10.14445/23942568/IJAES-V7I3P106
- Adjin, K. C., Goundan, A., Henning, C. H. C. A., & Sarr, S. (2020). Estimating the impact of agricultural cooperatives in Senegal: Propensity score matching and endogenous switching regression analysis. Working Papers of Agricultural Policy, No. WP2020-10. Kiel University, Department of Agricultural Economics, Chair of Agricultural Policy. http://nbn-resolving.de/urn:nbn:de:gbv:8:3-2021-00299-3. https://doi.org/10.1016/0169-5150(93)90019-9
- Ahmed, M. H., Geleta, K. M., Tazeze, A., & Andualem, E. (2017). The impact of improved maize varieties on-farm productivity and wellbeing: Evidence from the East Hararghe zone of Ethiopia. Development Studies Research, 4(1), 9–21. https://doi.org/10.1080/21665095.2017.1400393
- Alders, R. G., Dumas, S. E., Rukambile, E., Magoke, G., Maulaga, W., Jong, J., & Costa, R. (2018). Family poultry: Multiple roles, systems, challenges, and options for sustainable contributions to household nutrition security through a planetary health lens. Maternal & Child Nutrition, 14(Suppl 3), e12668. https://doi.org/10.1111/mcn.12668
- Alemayehu, T., Bruno, J., Getachew, F., & Dessie, T. (2018). Socio-economic, marketing and gender aspects of village chicken production in the tropics: A review of literature. ILRI Project Report International Livestock Research Institute (ILRI).
- Aliyi, I., Faris, A., Ayele, A., Oljirra, A., & Bayessa, M. (2021). Determinants of market participation among smallholder vegetable producers in Southwest Ethiopia. Ethiopian Journal of Applied Science and Technology, 12(2), 24–37.
- Amare, F., & Beyene, A. D. (2019). Climate-smart agricultural practices and welfare of rural smallholders in Ethiopia: Does planting method matter? Land Use Policy, 85, 387–396. https://doi.org/10.1016/j.landusepol.2019.04.020
- Anik, A., &Salam, A. (2015). Determinants of adoption of improved onion variety in Bangladesh. Journal of Agriculture and Environment for International Development, 109(1), 71–78. DOI: 10.12895/jaeid.20151.259.
- Ansah, Y. B., & Frimpong, E. A. (2015). Impact of the adoption of BMPs on social welfare: A case study of commercial floating feeds for pond culture of tilapia in Ghana. Cogent Food & Agriculture, 1(1), 1048579. https://doi.org/10.1080/23311932.2015.1048579
- Asfaw, S., Shiferaw, B., Simtowe, F., & Lipper, L. (2012). Impact of modern agricultural technologies on smallholder welfare: Evidence from Tanzania and Ethiopia. Food Policy. 37(3), 283–295. https://doi.org/10.1016/j.foodpol.2012.02.013
- Atela, J. A., Ouma, P. O., Tuitoek, J., Onjoro, P. A., & Nyangweso, S. E. (2016). Comparative performance of indigenous chicken in Baringo and Kisumu Counties of Kenya for sustainable agriculture. Int. J. Agric. Policy Res, 4, 97–104.
- Awotide, B. A., Karimov, A. A., & Diagne, A. (2016). Agricultural technology adoption, commercialization and smallholder rice farmers’ welfare in rural Nigeria. Agric. Agricultural and Food Economics, 4(3), 1–24. https://doi.org/10.1186/s40100-016-0047-8
- Belachew, A., Mekuria, W., & Nachimuthu, K. (2020). Factors influencing adoption of soil and water conservation practices in the northwest Ethiopian highlands. International Soil and Water Conservation Research, 8(1), 80–89. https://doi.org/10.1016/j.iswcr.2020.01.005
- Berhanu, G., & Swinton, S. M. (2003). Investment in soil conservation in Northern Ethiopia: The role of land tenure security and public programme. Journal of. Agricultural Economics, 29(1), 69–84. https://doi.org/10.1111/j.1574-0862.2003.tb00148.x
- Beshir, H. (2014). Factors affecting the adoption and intensity of use of improved forages in north east highlands of Ethiopia. American Journal of Experimental Agriculture, 4(1), 12–27. https://doi.org/10.9734/AJEA/2014/5481
- Beshir, M., Tadesse, M., Yimer, F., & Brüggemann, N. (2022). Factors affecting adoption and intensity of use of Tef-Acacia decurrens-charcoal production agroforestry system in Northwestern Ethiopia. Sustainability, 14(8), 4751. https://doi.org/10.3390/su14084751
- Bosshardt, S., Sabatier, R., Dufils, A., & Navarrete, M. (2022). Changing perspectives on chicken-pastured orchards for action: A review based on a heuristic model. Agricultural Systems, 196, 103335. https://doi.org/10.1016/j.agsy.2021.103335
- Bwalya, R., & Kalinda, T. (2014). An analysis of the value chain for indigenous chickens in Zambia’s Lusaka and Central Provinces. Journal of Agricultural Studies, 2(2), 32–51. https://doi.org/10.5296/jas.v2i2.5918
- Campbell, Z. A., Marsh, T. L., Mpolya, E. A., Thumbi, S. M., & Palmer, G. H. (2018). Newcastle disease vaccine adoption by smallholder households in Tanzania: Identifying determinants and barriers. PloS One, 13(10), e0206058. https://doi.org/10.1371/journal.pone.0206058
- Cragg, J. G. (1971). Some statistical models for limited dependent variables with application to the demand for durable goods. Econometrica, 39(5), 829–844. https://doi.org/10.2307/1909582
- Dumas, S. E. (2017). Evaluating the impact of poultry interventions on maternal and child nutrition outcomes in the Luangwa Valley, Zambia. [Doctoral dissertation]. Cornell University.
- Endiris, A., Brehanie, Z., & Ayalew, Z. (2021). The impact of off-farm activities on rural households’ food security status in Western Ethiopia: The case of Dibatie district. Cogent Food & Agriculture, 7(1), 1879425. https://doi.org/10.1080/23311932.2021.1879425
- FARA. (2015). State of knowledge on CSA in Africa: Case Studies from Ethiopia, Kenya and Uganda.
- Food and Agriculture Organization of the United Nations. (2013). The state of food and agriculture 2013. Food Systems for Better Nutrition.
- Food and Agriculture Organization of the United Nations. (2015). Family poultry development: Issues, opportunities, and constraints. Animal production and health working paper no. 12.
- Food and Agriculture Organization of the United Nations. (2018). Building stronger partnerships for resilience Opportunities for greater FAO engagement in realizing the goals of the DFID Humanitarian Policy. (Accessed from www.fao.org/publications).
- Gakige, J. K., King’ori, A. M., Bebe, B. O., & Kahi, A. K. (2015). Effects of targeted phase supplementary feeding on gut morphology of scavenging ecotypes of indigenous chickens in Kenya. Livestock Research for Rural Development, 27.
- Gebre-Selassie, A., & Bekele, T. (2014). A review of Ethiopian agriculture: Roles, policy and small-scale farming systems. Retrieved June 25, 2016, from http://global-growing.org/sites/default/files/GGC_Ethiopia.pdf.
- Gerber, P. J., Steinfeld, H., Henderson, B., Mottet, A., Opio, C., Dijkman, J., Falcucci, A., & Tempio, G. (2013). Tackling climate change through livestock: A global assessment of emissions and mitigation opportunities. Food and Agriculture Organization of the United Nations (FAO).
- Glatz, P. (2013). Improving the profitability of village broiler production in Papua New Guinea. Australian Centre for International Agricultural Research (ACIAR).
- Grabowski, H., Long, G., Mortimer, R., & Boyo, A. (2016). Updated trends in US brand-name and generic drug competition. Journal of Medical Economics, 19(9), 836–844.https://doi.org/10.1080/13696998.2016.1176578
- Greene, W. H. (2000).Econometric analysis. 4th Edition. Prentice Hall, Englewood Cliffs.
- Gregory, T., &Sewando, P. (2013). Determinants of the probability of adopting of quality protein maize (QPM) technology in Tanzania: A logistic regression analysis. International Journal of Development and Sustainability, 2(2), 729–746.
- Habtamu, R., & Krishna, N. (2021). Determinants of decision to use herbicides by smallholder farmers: The case of Dale Sadi district Kelem Wolega Zone, Oromia, Ethiopia. Journal of Economics and Sustainable Development, 12(13), 5–11. https://doi.org/10.7176/JESD/12-13-02
- Henchion, M., Moloney, A. P., Hyland, J., Zimmermann, J., & McCarthy, S. (2021). Review: Trends for meat, milk and egg consumption for the next decades and the role played by livestock systems in the global production of proteins. Animal: An International Journal of Animal Bioscience, 15(Suppl 1), 100287. https://doi.org/10.1016/j.animal.2021.100287
- Idio, A. D., &Okoro, G. I. (2017). Adoption of Improved Feed Production Technologies byPoultry Farmers in Akwa Ibom State, Nigeria. International Journal of Agricultural Extension and Rural Development Studies, 4(1), 1–22.
- Issahaku, G., & Abdulai, A. (2020). Adoption of climate-smart practices and its impact on farm performance and risk exposure among smallholder farmers in Ghana. Australian Journal of Agricultural and Resource Economics, 64(2), 396–420. https://doi.org/10.1111/1467-8489.12357
- Ithika, C. S.,Singh, S. P., &Gautam, G. (2013). Adoption of scientific poultry farming practices by the broiler farmers in Haryana. Iranian Journal of Applied Animal Science, 3(2), 417–422.
- Kaliba, A. R., Mazvimavi, K., Gregory, T. L., Mgonja, F. M., & Mgonja, M. (2018). Factors affecting adoption of improved sorghum varieties in Tanzania under information and capital constraints. Agricultural and Food Economics, 6(1), 1–21. https://doi.org/10.1186/s40100-018-0114-4
- KALRO. (2018). Kenya Agricultural and Livestock Research Organization. Annual report 2017-2018.
- Kenya National Bureau of Statistics. (2019). The Kenya Population and housing census. Distribution of Population Distribution by Socio-Economic Characteristics, Vol. 4.
- Khan, K., Saeed, A. F., Khattak, S. W., & Liaqat, S. (2022). Determinants of poultry technology adoption: Evidence from District Lasbela, Balochistan, Pakistan. Sarhad Journal of Agriculture, 38(3), 960–967. https://doi.org/10.17582/journal.sja/2022/38.3.960.967
- Khonje, M., Manda, J., Alene, A. D., & Kassie, M. (2015). Analysis of adoption and impacts of improved maize varieties in Eastern Zambia. World Development, 66, 695–706. https://doi.org/10.1016/j.worlddev.2014.09.008
- Knight, J.,Weir, S., &Woldehanna, T. (2003). The role of education in facilitating risk-taking and innovation in agriculture. Journal of Development Studies, 39(6), 1–22. doi:10.1080/00220380312331293567
- Machakos County Integrated Development Plan (CIDP). (2018). Accessed from. https://www.cog.go.ke/downloads/category/106-county-integrated-development-plans2018-2022.
- Manda, J., Alene, A. D., Tufa, A. H., Abdoulaye, T., Wossen, T., Chikoye, D., & Manyong, V. (2019). The poverty impacts of improved cowpea varieties in Nigeria: A counterfactual analysis. World Development, 122, 261–271. https://doi.org/10.1016/j.worlddev.2019.05.027
- Mango, N., Makate, C., Tamene, L., Mponela, P., & Ndengu, G. (2020). Impact of the adoption of conservation practices on cereal consumption in a maize-based farming system in the Chinyanja Triangle, Southern Africa. Sustainable Futures, 2, 100014. https://doi.org/10.1016/j.sftr.2020.100014
- Melkamu, T., Kumar, N., Gebrekidan, B., Kebede, E., & Tkue, T. (2017). Performance of Bovans Brown chickens under intensive and backyard management system in Mekelle, Ethiopia. Ethiopian Journal of Veterinary Science and Animal Production, 1, 73–80.
- MoALF. (2017). Climate risk profile for Machakos County. Kenya County climate risk profile series. The ministry of agriculture, livestock and fisheries (MoALF).
- Mulungu, K., & Kangogo, D. (2022). Striving to be resilient: the role of crop-poultry integrated system as a climate change adaptation strategy in semiarid eastern Kenya. Heliyon, 8(11), e11579. https://doi.org/10.1016/j.heliyon.2022.e11579
- Mutenje, M., Kankwamba, H., Mangisonib, J., & Kassie, M. (2016). Agricultural innovations and food security in Malawi: Gender dynamics, institutions and market implications. Technological Forecasting and Social Change, 103, 240–248. https://doi.org/10.1016/j.techfore.2015.10.004
- Mwololo, H. M., Nzuma, J. M., Ritho, C. N., Ogutu, S. O., & Kabunga, N. (2020). Determinants of actual and potential adoption of improved indigenous chicken under asymmetrical exposure conditions in rural Kenya. African Journal of Science, Technology, Innovation and Development, 12(4), 505–515. https://doi.org/10.1080/20421338.2019.1636489
- Nanyeenya, W. N., Mugisha, A., Musinguzi, S. P., Magambo, R., & Senoga, M. (2013). Constraints, livelihoods and technology adoption of village chicken producers in Uganda. Livestock Research for Rural Development, 25. Retrieved January 17, 2023, from. http://www.lrrd.org/lrrd25/11/nany25188.html
- Nduthu, P. W. (2015). Social-economics influence on indigenous poultry production project in Kenya. A Case of Machakos Indigenous Poultry. International Journal of Education and Research, 3(1).
- Ng’ombe, J.,Kalinda, T.,Tembo, G., &Kuntashula, E. (2014). Econometric analysis of the factors that affect adoption of conservation farming practices by smallholder farmers in Zambia.. Journal of Sustainable Development, 7(4), 124–138. https://doi.org/10.5539/jsd.v7n4p124.
- Ngeno, V. (2018). Impact of dairy hubs on small-holder welfare: Empirical evidence from Kenya. Agricultural and Food Economics, 6(1), 9–13. https://doi.org/10.1186/s40100-018-0107-3
- Nkhoma, S., Kalinda, T., & Kuntashula, E. (2017). Adoption and impact of conservation agriculture on smallholder farmers ‘crop productivity and Income in Luapula Province, Zambia. Journal of Agricultural Science, 9(9), 168–181. https://doi.org/10.5539/jas.v9n9p168
- OECD. (2022). Agricultural policy monitoring and evaluation 2022: Reforming agricultural policies for climate change mitigation. OECD Publishing. https://doi.org/10.1787/7f4542bf-en.
- OECD/FAO. (2022). OECD-FAO agricultural outlook 2022-2031. OECD Publishing. https://doi.org/10.1787/f1b0b29c-en
- Okoffo, E. D., Denkyirah, E. K., Adu, D. T., & Fosu-Mensah, B. Y. (2016). A double-hurdle model estimation of cocoa farmers’ willingness to pay for crop insurance in Ghana. SpringerPlus, 5(1), 873. https://doi.org/10.1186/s40064-016-2561-2
- Organization for Economic Co-operation and Development, OECD Agriculture Statistics [Datasets], https://doi.org/10.1787/agr-outl-data-en
- Passarelli, S., Abdelmenan, S., Tewahido, D., Demmu, Y. M., Abreham, H., Ambikapathi, R., Gunaratna, N. S., Berhane, Y., & Fawzi, W. (2022). Nutrition-sensitive chicken production in Ethiopia: A qualitative evaluation. Agriculture & Food Security, 11(1), 1–12. https://doi.org/10.1186/s40066-022-00363-8
- Patel, S. J., Patel, A. S., Patel, M. D., & Patel, J. H. (2016). Significance of light in poultry production: A review. Advances in Life Sciences, 5(4), 1154–1160.
- Quddus, M. A. (2022). Dissemination of technological innovations of livestock in Bangladesh: Adoption levels and behavioral precision. Proceedings of the National Academy of Sciences, India Section B: Biological Sciences, 92(2), 461–472. https://doi.org/10.1007/s40011-022-01357-z
- Rogers, E. M., & Shoemaker, M. M. (1971). Communication of Innovations. New York, The Free Press.
- Sariyev, O., & Zeller, M. (2023). Crossbred poultry adoption and impact: Evidence from Ethiopia. Social Sciences & Humanities Open, 7(1), 100394. https://doi.org/10.1016/j.ssaho.2022.100394
- Shapiro, B., Gebru, G., Desta, S., Negassa, A., Nigussie, K., Aboset, G., & Mechale, H. (2017). Ethiopia livestock sector analysis: A 15-year livestock sector strategy. ILRI project report. https://cgspace.cgiar.org/bitstream/handle/10568/92057/LSA_Ethiopia.pdf?sequence=3.
- Singha, A. K., Jat, P. C., Bordoloi, R., Singha, J. K., Devi, M., & Bhuyan, P. C. (2016). Determinants of adoption of poultry technology by the farmers of adopted and non-adopted villages in North Eastern states of India. Economic Affairs, 61(4), 633–640. https://doi.org/10.5958/0976-4666.2016.00079.6
- Smith, B. A., Todd, R., Erik, S., Heather, A., Neil, C., Ian, Y., Anna, M. L., & Aamir, F. (2015). A risk modeling framework to evaluate the impacts of climate change and adaptation on food and water safety. Food Research International, 68, 78–85. https://doi.org/10.1016/j.foodres.2014.07.006
- Tadelech, W., & Mesfin, T. (2020). Determinants of adoption of improved chicken packages: The case of Wolaita zone, southern Ethiopia. International Journal of Current Research, 12(1), 9158–9163.
- Tamir, S.,Fessiha, M.,Tilahun, Y., &Haile, M. (2015). Determinants of adoption of exotic poultry breeds among smallholder poultry producers in North Western Amhara Region, Ethiopia. Global Science Research Journals, 3(6), 162–168.
- Tasie, L. S. O., Sanou, A., & Tambo, J. A. (2019). Climate change adaptation among poultry farmers: evidence from Nigeria. Climate Change, 157(3-4), 527–544. https://doi.org/10.1007/s10584-019-02574-8
- Teklewold, H., Dadi, L., Yami, A., & Dana, N. (2006). Determinants of adoption of poultry technology: A double-hurdle Approach. Journal of Rural Development, 18(3).
- Teklewold, H., Mekonnen, A., Kohlin, G., & Di Falco, S. (2017). Does adoption of multiple climate-smart practices improve farmers climate resilience? Empirical evidence from the Nile Basin of Ethiopia. Clim. Chang. Econ, 8, 30.
- Teshome, S., & Tegegne, B. (2020). Determinants of adoption of improved teff varieties by smallholder farmers: The case of Kobo District, North Wollo Zone, Amhara Region, Ethiopia. International Journal of Agricultural Economics, 5(4), 114–122. https://doi.org/10.11648/j.ijae.20200504.14
- Tran, N. L. D., Rañola, R. F., Ole Sander, B., Reiner, W., Nguyen, D. T., & Nong, N. K. N. (2019). Determinants of adoption of climate-smart agriculture technologies in rice production in Vietnam. International Journal of Climate Change Strategies and Management, 12(2), 238–256. https://doi.org/10.1108/IJCCSM-01-2019-0003
- Tsadik, E., Berhan, T., & Zemelak, S. (2015). Determinants of village poultry technology package adoption; limitations, constraints and opportunities in the central Oromia Region, Ethiopia. International Journal of Development Research, 5, 4436–4443.
- Uhunamure, S., Nethengwe, N., & Tinarwo, D. (2019). Correlating the factors influencing household decisions on adoption and utilisation of biogas technology in South Africa. Renewable and Sustainable Energy Reviews, 107, 264–273. https://doi.org/10.1016/j.rser.2019.03.006
- United Nations Development Partners (UNDP). (2017). Africa and foresight: Better futures in development. UNDP Global Centre for Public Service Excellence. UNDP.
- Willett, W., Rockström, J., Loken, B., Springmann, M., Lang, T., Vermeulen, S., Garnett, T., Tilman, D., DeClerck, F., Wood, A., Jonell, M., Clark, M., Gordon, L. J., Fanzo, J., Hawkes, C., Zurayk, R., Rivera, J. A., De Vries, W., Majele Sibanda, L., … Murray, C. J. L. (2019). Food in the anthropocene: The eat–lancet commission on healthy diets from sustainable food systems. Lancet (London, England), 393(10170), 447–492. https://doi.org/10.1016/S0140-6736(18)31788-4
- Wondmeneh, E., van Der Waaij, E. H., Tadelle, D., Udo, H. M. J., & van Arendonk, J. A. M. (2014). Adoption of exotic chicken breeds by rural poultry keepers in Ethiopia. Acta Agriculturae Scandinavica, Section A—Animal Science, 64(4), 210–216. https:// https://doi.org/10.1080/09064702.2015.1005658
- Wong, J. T., de Bruyn, J., Bagnol, B., Grieve, H., Li, M., Pym, R., & Alders, R. G. (2017). Small-scale poultry and food security in resource-poor settings: A review. Global Food Security, 15, 43–52. https://doi.org/10.1016/j.gfs.2017.04.003
- Wooldridge, J. M. (2002). Econometric analysis of cross section and panel data. The MIT Press.
Annexes
Annex 1. T-statistics on double hurdle (probit + truncated model) versus Tobit model.
Annex 2. Status of adoption of ic production technologies.