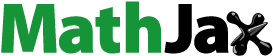
Abstract
The objective of this study is to predict unemployment in Indonesia in the wake of the demographic dividend. The sample used in this study is the unemployment data from 1990 to 2022 from the Indonesian Central Bureau of Statistics database. Using non-seasonal ARIMA (Autoregressive Integrated Moving Average) modeling, this study projected unemployment. It was predicted using six alternative models. With a mean absolute percent error (MAPE) of 9.56% (MAPE ≤10%), the predictions were quite accurate. It indicates that the ARIMA model has a good forecasting capability. According to the dynamic method’s unemployment projection, there will be less unemployment between 2023 and 2050. For Indonesia, maximizing the demographic dividend is both a challenge and an opportunity presented by the decline and stable number in unemployment. The demographic dividend will cause a substantial increase in employment and the creation of various new jobs. Several factors will support the demographic dividend. Thus, it could help governments to make decisions on labor issues. It also highlights a policymaker’s direction to pursue labor development, including employment trends.
IMPACT STATEMENT
The purpose of this study is to forecast Indonesia’s unemployment rate following the demographic dividend. As a result, an Autoregressive Integrated Moving Average (ARIMA) was suggested for a univariate time-series analysis. Based on dynamic and static methodologies, my results demonstrate that the ARIMA model could predict Indonesia’s unemployment rate. The execution of sixteen economic policy packages, improvements to the human resources quality policy, and an accelerated infrastructure development strategy are some factors that promote the demographic dividend. A robust investigation shows that GDP has a positive and significant effect on the labor force between the ages of 15 and 19, as well as the influence of COVID-19 periods on unemployment in Indonesia. It could be helpful for government decision-making about labor issues. It also highlights the course that a policymaker wishes to pursue to achieve labor development.
1. Introduction
Indonesian Central Bureau of Statistics has predicted that from 2035 to 2045, Indonesia will experience the end of the demographic dividend. This means that the size of the elderly population will increase, while the number of young people will be less or unable to keep up with the growth of the elderly group (Kaneko & Sato, Citation2013; Ogawa et al., Citation2005, Citation2010). As a result, youth groups must work to bear the burden of meeting the needs of the older generation. When the youth group is unable to meet the needs of the older group, this triggers a window of opportunity, which is the cause of the socio-economic crisis (Han-Hyung, Citation2009; Kim et al., Citation2019; Oku et al., Citation2017; Stephen, Citation2012; Usman & Tomimoto, Citation2013).
The changing age structure of a population can provide a powerful incentive for economic growth and family welfare. Indonesia’s current demographic conditions are ready to take advantage of such a demographic dividend. Favorable conditions have existed for some time, but the window of opportunity will begin to close after another decade. Several studies conducted by Dritsaki (Citation2016), Dritsakis and Klazoglou (Citation2018), Floros (Citation2005), Huang (Citation2015), Johnes (Citation1999), Kurita (Citation2010), Nkwatoh (Citation2012), Romero-Ávila and Usabiaga (Citation2007), Rublikova and Lubyova (Citation2013), Wilson and Perry (Citation2004), Montgomery et al. (Citation1998), Etuk et al. (Citation2012), Larson and Sinclair (Citation2022), Naccarato et al. (Citation2018), Nagao et al. (Citation2019), Simionescu and Cifuentes-Faura (Citation2022), Larson and Sinclair (Citation2022), González-Fernández and González-Velasco (Citation2018), D’Amuri and Marcucci, (Citation2017), Caperna et al. (Citation2022), Aaronson et al. (Citation2022) intend to either develop econometric models and Google Trends to forecast unemployment but little research has explicitly focused related to unemployment forecasting and demographic dividend. In Indonesia, the International Monetary Fund (Citation2019), Mahmudah et al. (Citation2017), and Fajar et al. (Citation2020) have predicted the unemployment rate, but they do not consider the demographic dividend factor in their results. Only a few studies in Indonesia explore the demographic dividend and working-age population projection (e.g., Ulhaq & Wahid, Citation2022).
Despite some of these studies offering a strong foundation for developing an econometric model on the subject, there is not much literature on the relationship between Indonesian demographic dividends and unemployment forecasting. Consequently, the current paper seeks to contribute to the existing literature. First, it offers a comprehensive analysis using ARIMA to predict unemployment in Indonesia. In other words, it was a comprehensive study in Indonesia that provided a model and predicted the future of unemployment. Second, the ARIMA model is necessary to observe the dynamic pattern (e.g., annual basis) of unemployment in Indonesia. Third, very few research studies in Indonesia explore unemployment prediction and demographic dividend. This study fills this gap by demonstrating that Indonesia’s government needs to manage labor (wages, job retraining, up-skilling programs, etc.), health, education, and infrastructure spending to optimize the demographic dividend. Based on these arguments, I propose a research question: can the ARIMA model predict Indonesia’s unemployment rate in the wake of demographic dividend? Therefore, the objective of this study is to predict unemployment in Indonesia in the wake of the demographic dividend by using the dynamic and static method of the Autoregressive Integrated Moving Average model.
The remainder of this paper is structured as follows. Section 2 describes the study background. Section 3 develops the theoretical literature review. Section 4 summarizes the empirical literature review and the development of the hypotheses. Section 5 highlights the research design. Section 6 posits the empirical results and discussion. Section 7 presents summary and conclusion remarks.
2. Background
Indonesia, the fourth most populated country in the world, is expected to experience its ‘demographic dividend’. This circumstance indicates that the proportion of the population that is working age will reach its highest point and that there will theoretically be the greatest opportunity for higher output per capita and, consequently, more fruitful investment. However, realizing this potential will depend on several social and economic factors, such as gender equality and other important concerns related to human resource capacity, which will decide how well-positioned Indonesia is to handle the challenges involved (Oey-Gardnier & Gardnier, Citation2013).
This contribution begins by reviewing the transition of demographics in Indonesia. The term ‘demographic transition’ describes how a population’s age distribution shifts (Adioetomo, Citation2004). It began with at least some focus on bettering health conditions in the years after independence. Still, it didn’t pick up speed until the New Order Government took the national family planning program seriously. It was in line with the government’s heightened focus on development, which included investments in social development in vital sectors like health and education in addition to attaining rapid economic growth (Adioetomo, Citation2004).
The family planning program in Indonesia has a long history of success, as documented by Hull et al. (Citation1977). Indonesia was fortunate to have windfall income from abruptly soaring oil prices when the National Family Planning Program began, enabling social spending. Welfare was enhanced by the provision of jobs for women, especially young women, as well as for men. This happened in the public and commercial sectors, particularly in health and education, as Indonesia implemented a labor-intensive import substitution strategy to create domestic commodities. As a result of the growth of community health clinics catering to the wealthy and the underprivileged, health services became more widely available and easily accessible. According to the education policy, a primary school should be present in every village in rural and urban areas.
The East Asian populations and the developing world grew rapidly (e.g., in 1960). Consequently, several governments in East Asia and Southeast Asia, such as China, South Korea, Taiwan, Singapore, Thailand, and Indonesia (except Malaysia following a pro-natalist course), pursued policies to lower childbearing rates and slow population growth (Mason & Kinugasa, Citation2008). As a developing country, Indonesia has a development agenda outlined in the triple-track strategy, including pro-growth, pro-jobs, and pro-poor. It can be understood that economic growth is a result of increased production capacity, which is a derivative of increased investment. Therefore, economic growth is closely related to an increase in the use of labor as well as investment (Todaro & Smith, Citation2015). Of course, an increase in investment will increase labor demand. It means that economic growth caused by increased investment will affect the decline in the unemployment rate, assuming investment is labor-intensive.
The global COVID-19 pandemic has slowed the world’s economy, including Indonesia’s. This economic slowdown led to fewer job prospects and many layoffs in 2020. In May 2020, 1.7 million workers were affected by the COVID-19 pandemic. Until the end of 2020, this pattern persisted. 29.12 million working-age persons were impacted by the pandemic starting in August 2020, with 2.56 million losing their jobs, 0.76 million giving up looking for work, 1.77 million experiencing temporary unemployment, and 24.03 million having their work hours reduced. The COVID-19 pandemic-related economic slowdown also increased the open unemployment to 7.07%, translating to 9.77 million unemployed individuals. With a 13.55% share, graduates from technical schools continue to dominate this high open unemployment. The percentage of informal workers increased by 4.59% from 2019 to 60.47%, or 77.68 million workers (SMERU Research Institute, Citation2021). According to the SMERU Research Institute (Citation2021), most laid-off workers were young (15-24 years old). Construction, trade, restaurant, and accommodation services had the highest layoffs. Indonesia is predicted to join the demographic dividend period between 2012 and 2035, with the window of opportunity occurring between 2020 and 2030. The fact that there are twice as many working-age people as there are children and the elderly indicates that this problem exists. The vast population of people of working age has the potential to supply labor, business actors, and potential customers who play a critical role in quickening development (Central Bureau of Statistics, Citation2022). Predicting open unemployment in Indonesia is an important issue due to the 2030 demographic dividend. This study accurately highlights the Indonesian economy and the sakes of investment. Therefore, the 2030 demographic dividend is the path to becoming one of the Top Five world economic giants.
3. Theoretical literature review
3.1. Demographic dividend
The demographic dividend refers to the accelerated economic growth that begins with changes in the age structure of the population of a country as its transitions from high to low rates of birth and death (Gribble & Bremner, Citation2012). Recent demographic dividend studies have investigated groups of countries with different income levels (Lee & Mason, Citation2012). Low, upper-middle-income countries already face the end of this opportunity window. Only Russia, India, and China benefited from the demographic transition in emerging countries (Brito & Carvalho, Citation2015; Stampe et al., Citation2013). However, Sub-Saharan African countries have not taken advantage of the demographic dividend as they need reforms to improve human capital (Drummond et al., Citation2014; Harkat & Driouchi, Citation2017; Loewe, Citation2007).
The robust and effective fertility control program in Indonesia has reduced overall population growth and altered the country’s age distribution. Due to decreased fertility, the broad pyramid of children has shrunk to include a growing percentage of people in their prime working years. As the nation advances into the future, this proportion will eventually rise more quickly among the elderly (Oey-Gardnier & Gardnier, Citation2013). These demographic patterns are reflected in the United Nations’ (United Nations, Citation2009) population predictions. Following independence, the nation saw a baby boom that increased the percentage of children in the general population. Specifically, the percentage of people aged 0–14 climbed from 39% in 1950 to 42% in 1970.
The dependence ratio, defined as the ratio of individuals aged 0–14 and 65 and over to the working-age population aged 15–64, reflects the so-called burden on society represented by the young and old. It symbolizes the ‘weight’ carried by individuals in their most fruitful years, supporting people typically perceived as consuming more than they generate (Mason & Kinugasa, Citation2008). The age pyramid shifts towards the working age due to a prolonged fertility drop. This is accompanied by a decrease in overall dependency brought on by a comparatively large number of children and the elderly. But eventually, there comes the point at which the weight of the old begins to increase at an ever-faster rate while the load of the children keeps decreasing. What demographers refer to as ‘the demographic dividend’ is the time when the dependency burden is at its lowest, and society may most effectively take advantage of the opportunity provided by their productive forces. The term ‘dividend’ refers to this phenomenon because of a recent macroeconomic analysis that examines the impact of population dynamics on economic development. Furthermore, Asia’s economic success bears witness to the significant role of the demographic dividend in driving economic growth (Oey-Gardnier & Gardnier, Citation2013).
The UN’s century-long demographic estimates for 1950–2050 tell the general picture of an early dependency spike followed by a reduction as family planning became more popular and fertility rates decreased. The proportion of the working-age population has increased recently in response to the dropping proportion of children, and the proportion of the elderly will only start to rise noticeably in the second quarter of the twenty-first century. Although the demographic shift’s nature and the timing of this ‘window of opportunity’ are obvious, numbers by themselves may not be enough to establish the favorable welfare conditions necessary to take advantage of this shift (United Nations, Citation2009).
Indonesia’s high rate of youth unemployment is one of its issues. The people who live in Indonesia are young. Nearly half of the population is under thirty. This may suggest that there is a substantial labor force in the country. However, the demographic dividend could become tragic if job opportunities are insufficient to support this labor force. Therefore, it is imperative to look ahead, particularly given that Indonesia is predicted to witness a demographic dividend.
3.2. Unemployment prediction
Economic growth, employment, industrial transitions, and other concerns are among the numerous that face us in economics. Unemployment is among contemporary civilisation’s most significant problems (Boga, Citation2020). When predicting future employment performance, unemployment is a significant factor. When predicting effective employment, the unemployment rate is commonly considered one of the most significant macroeconomic indicators (Caperna et al., Citation2022; Jo et al., Citation2023; Naccarato et al., Citation2018). The stock-flow concept can also be understood by understanding unemployment (Byrialsen & Raza, Citation2018; Jo et al., Citation2023). The analysis of a change in outflow concerning inflow is employed as an indication in a stock-flow model to predict the unemployment rate (Barnichon & Nekarda, Citation2012). A nonlinear unemployment rate forecast model that converges to a conditional steady-state with a three to five-day lag was presented by Barnichon and Nekarda (Citation2012). Through a stock-flow model that reduces practical complexity and offers potential remedies, their work explains an unemployment issue. Google Trends has also been used in numerous studies to estimate unemployment and has shown to be a trustworthy source of trend data for online searches to predict various macroeconomic trends. Effective techniques to enhance unemployment prediction with possible explanatory variables from Google Trends are showcased (Caperna et al., Citation2022; Naccarato et al., Citation2018; Simionescu, Citation2015). A robustness or additional analysis demonstrates the framework of Okun law. Okun (Citation1962) discovered the inverse link between economic growth and the unemployment rate. When the real output growth rate was high, Okun (Citation1962) saw a decline in unemployment and vice versa.
4. Empirical literature review and hypotheses development
Previous studies in several countries around the world investigated the forecasting of unemployment. In Australia, the quarterly unemployment rate from 1978 Q2 to 2002 Q3 was investigated by Wilson and Perry (Citation2004). They proved that spectral analysis models achieve higher predictive accuracy levels than autoregressive integrated moving average counterparts (e.g., the accuracy of turning point forecast). The spectral analysis showed that the Australian unemployment rate for individuals, males, and females continues to decline over the forecast period. Also, Romero-Ávila and Usabiaga (Citation2007) used individual Lagrange multiplier (LM) to investigate the annual unemployment rate from 1976 to 2004. They proved that U.S. unemployment is closer to the natural rate paradigm than the hysteresis paradigm. The fact showed that the unemployment rate trend declined after the first oil shock. It can be understood that stabilization policies and labor market reforms have been implemented in the right direction.
In addition, the quarterly data from Q1 1948 to Q2 1968 were predicted by Montgomery et al. (Citation1998). They demonstrated an asymmetric cyclical movement (e.g., during the severe downward cycles) that dominates the behavior of the unemployment rate over time (e.g., except during the long business expansions of the 1960s and 1980s). The unemployment movement was affected by general contractions in business (e.g., long and gradual declines in business expansion). In addition, unemployment dropped slowly and irregularly (e.g., after the Second World War). Mahmudah et al. (Citation2017) predicted Indonesia’s annual unemployment rate data from 1986 to August 2015. This study showed an ongoing decline in the unemployment rate in Indonesia. A low unemployment rate indicates a good shape for the national economy.
In Japan, the monthly unemployment rate data from January 1995 to August 2005 were estimated by Kurita (Citation2010). This study showed that the fractionally integrated autoregressive and moving average (ARFIMA) model is considered a reliable predictive device for the unemployment rate in Japan. Moreover, the prediction results revealed the growing unemployment rates for the next period. Furthermore, from 1999 to August 2008, Etuk et al. (Citation2012) predicted the monthly unemployment rate data. They demonstrated that the appropriate model is an autoregressive integrated moving average of orders 1 and 2 and ARIMA(1,2,1). The prediction results revealed Nigeria’s increasing unemployment rate in Nigeria between January and March 2009.
From January 1955 to July 2017, Dritsakis and Klazoglou (Citation2018) investigated the monthly unemployment rate in the United States. They demonstrated that the Seasonal Autoregressive Integrated Moving Average (1,1,2)(1,1,1)12 and Generalized Autoregressive Conditional Heteroskedasticity (1,1) are the best models for predicting unemployment in the U.S. The forecast result revealed that the expected unemployment value is close to the actual value. Therefore, investigating this trend and proposing solutions is important for researchers. However, Huang (Citation2015) used an autoregressive integrated moving average with exogenous variables to analyze the monthly unemployment rate from January 1948 to October 2014. This study showed that the index of job openings has much greater predictive power than the initial job insurance claim. It means that the index of job openings can be used as one of the leading indicators to improve the unemployment forecast. In Slovakia, Rublikova and Lubyova (Citation2013) investigated the monthly unemployment rate between January 1999 and May 2013. The results showed that the ARIMA (0,1,2)(0,1,1)12 and GARCH(1,1) model was the best to forecast both the conditional mean and the conditional variance. In Nigeria, the quarterly data from 1967Q1 to 2011Q4 was predicted by Nkwatoh (Citation2012). Based on the results of the root mean square error (RMSE), mean absolute percent error (MAPE), mean absolute error (MAE) criteria and Theil’s coefficient, it has been shown that the ARIMA(1,1,2) or ARCH(1) model is the most suitable for forecasting unemployment in the particular period. In Greek, Dritsaki (Citation2016) used monthly data from 1998 to 2015 to predict the unemployment rate for the dynamic and static processes. Using the Box-Jenkins methodology and SARIMA models, it has been shown that the SARIMA(0,2,1)(1,2,1)12 model is the best forecasting model, while the mean squared error (MSE), the MAE criteria and Theil’s coefficient have been more predictive in the static process.
Johnes (Citation1999) predicted the monthly data in the United Kingdom from January 1960 to August 1996. He proved that more general alternative specifications dominate the simple linear AR model. Additionally, the nonlinearities are present in the data under investigation. The linearity hypothesis cannot be rejected for other important macroeconomics (e.g., output). The monthly unemployment rate data from January 1971 to December 2002 was also predicted by Floros (Citation2005). This study showed that MA(1) and AR(4) are the best models to predict the unemployment rate. Such results showed that the forecasting approaches were similar to the reality of the UK labor market. This showed a close relationship between the forecasting theory and labor market conditions.
Some recent studies try to forecast unemployment with several econometric models. To estimate the unemployment rate, Yamacli and Yamacli (Citation2023) examine the accuracy of the Auto-Regressive Integrated Moving Average (ARIMA) and Artificial Neural Networks (ANN) approaches. According to the comparison results, the ARMA (2,1) model is the most appropriate for estimating the unemployment rate. This outcome confirms that there are enduring causes for unemployment in Turkey. On the other hand, during the severe pandemic in 2020–2021, the ARMA (2,1) model’s unemployment rate forecasting error was greater than that of the ANN model. The results of the neural network model have fewer errors during the economic uncertainty brought on by the COVID-19 pandemic than those of the autoregressive moving average model. As a classifier, neural networks outperform alternative scoring models regarding prediction accuracy (Abdou et al., Citation2016). Artificial neural network modeling of the unemployment rate offers different insights for economic forecasting. Using regional unemployment rates recorded for the Romanian counties, Simionescu (Citation2015) predicts the national unemployment rate using the spatial autoregressive process (SAR). The outcome demonstrates that county unemployment in the current period will be impacted by neighboring changes in the unemployment rate from the previous period. Most counties’ 2013 unemployment rates fell between 5.36 and 6.54%. The SAR method with random effects estimates for 2014 indicated that the country’s unemployment rate would be approximately 4.7%. Yet, there are strong chances to be an underestimated value from 2011 to 2013. The duration distribution of unemployment is predicted using a three-step factor-flows simulation-based approach by Chodorow-Reich and Coglianese (Citation2021). They discovered that although increasing in a more gloomy recovery scenario due to a high level of labor market churn, long-term unemployment remains below the Great Recession level. The findings advise how many people are impacted by various extension lengths. For instance, in the baseline scenario, a large percentage of potentially eligible (e.g., laid off) people may experience unemployment for longer than the 26 weeks covered by ordinary state benefits, totaling more than 4.2 million at the peak.
Several studies recommend using data from online searches to enhance the forecasting and nowcasting of official economic indicators to speed up the dissemination of these data. When the economy undergoes a sudden transformation, near-term projections (nowcasts) are the most challenging but important (Larson & Sinclair, Citation2022). Naccarato et al. (Citation2018) determine whether big data and time series models can help anticipate Italy’s monthly young unemployment rate. The Google Trends keyword search volume for ‘job offers’ and the official labor force survey data for ‘young unemployment’ in Italy are the time series that were used. There are two estimated models. A VAR model that combines the former series with the Google Trends query share and an ARIMA model that uses only the official young unemployment rate series. The findings demonstrate that using Google Trends data reduces forecast error on average. Nagao et al. (Citation2019) compare the traditional AR model to search intensity data from Google Trends to nowcast the unemployment rate in the United States. For some circumstances, using Google Trends doesn’t necessarily help to increase forecast accuracy. According to this evidence, adding a particular phrase’s search volume to the forecast model may be limited. The first problem is the modification to Google Trends’ definition, which restricts the time frame for which the information on search intensity may be accessed weekly. The second concern is the possibility of a change in the endpoint value of a seasonal series depending on the date of the seasonal adjustment, which could pose problems when performing a real-time forecast. Based on information from Google Trends, Simionescu and Cifuentes-Faura (Citation2022) predicted the unemployment rate in Spain and Portugal. The forecast is based on macroeconomic statistics that have a greater time lag. The outcomes showed how Google Trends’ unemployment-related data might be used to estimate unemployment rates across regions in Spain accurately. To accurately predict US initial unemployment claims in the spring of 2020 during the COVID-19 epidemic, Larson and Sinclair (Citation2022) examine the performance of models using various information sets and data structures. A state-level panel model that adds dummy variables to describe the heterogeneity in the timing of state-of-emergency declarations is the best model, particularly close to the structural break in claims. Initial results from autoregressive models are poor, but they quickly improve. The state-level panel model outperforms models using Google Trends by taking advantage of the diversity in the timing of state-of-emergency announcements. The findings imply that there is a bias-variance tradeoff during structural change. Forecasts are improved by straightforward methods that take advantage of relevant data in the cross-sectional dimension, but the efficiency of autoregressive models dominate in later periods.
González-Fernández and González-Velasco (Citation2018) investigate whether Google econometrics can be used to predict unemployment in Spain. The findings show that Google searches and unemployment have a strong relationship. According to the findings, Google data search volumes enhance unemployment prediction. As a leading indicator for forecasting the monthly US unemployment rate, they evaluate the effectiveness of a Google job-search intensity index (D’Amuri & Marcucci, Citation2017). Google-based models produce more precise forecasts than models based on labor force flows, typical nonlinear models, and models from the Survey of Professional Forecasters. Google-based models performed particularly well at the critical juncture at the beginning of the Great Recession, while their relative prediction powers remained steady afterwards. Caperna et al. (Citation2022) developed a search-based unemployment index using machine learning techniques. They are also investigating the impact of lockdowns during the first wave of the COVID-19 epidemic. Using a Difference-in-Differences study, they demonstrate that the indicator increased dramatically and consistently after lockdowns. Using a state-level event analysis, Aaronson et al. (Citation2022) use Google Trends to assess the elasticity of initial unemployment insurance claims concerning search volume. The findings demonstrate that Google Trends elasticity caused by hurricanes produces better real-time predictions of initial unemployment insurance claims than other widely used models. The method is also suitable for forecasting on a state and federal level. In its estimate of the degree of uncertainty for its out-of-sample forecasts during the COVID-19 pandemic, it is demonstrated to be well-calibrated. The COVID-19 outbreak is substantially influencing Indonesia’s economy, which is contracting and leading to a rise in unemployment (Fajar et al., Citation2020). Using an Autoregressive Integrated Moving Average with Explanatory Variable (ARIMAX) with Google Trends data query share for the keyword of job termination, Fajar et al. (Citation2020) forecast the unemployment rate during the COVID-19 pandemic. The results MAPE number of 13.46% indicates that the ARIMAX model has good predicting capabilities. The outcomes of utilizing ARIMAX to forecast the open unemployment rate over the COVID-19 phase produce projected numbers consistent with reality. Although some of these studies provide a solid base to develop an econometric model on unemployment forecasting, an analysis regarding unemployment forecasting and demographic dividends in Indonesia is still limited. Based on the prior studies, I propose the following hypotheses:
Ho: The ARIMA model can’t predict Indonesia’s unemployment rate in the wake of the demographic dividend.
H1: The ARIMA model can predict Indonesia’s unemployment rate in the wake of the demographic dividend.
5. Research design
5.1. Sample and data sources
In this study, I use a univariate time-series analysis. It means that only one variable varies over time. A time-series design was utilized to assess the future open unemployment rate. This study uses open unemployment data from 1990 to 2022 provided by the Indonesian Central Bureau of Statistics. The procedures for collecting, organizing, and analyzing data are the basic principles of statistics. The techniques used to reach broader conclusions or inferences about populations based on samples are known as inferential statistics. Purposive sampling was employed to choose the samples. The following criteria choose the sample. Indonesia made significant progress in education and health during the 1990s, albeit from a relatively low base, due to the authoritarian New Order regime, a booming economy, and a highly centralized set of development policies spreading access to basic services more widely across the nation. The World Bank (Citation1990) even acknowledged this in its well-known study on the Asian economic miracle. Second, I consider these periods due to the COVID-19 pandemic from 2020 until 2022. Third, since the 1990–1995 economic boom, the official open unemployment rate has gradually risen. But throughout the crisis, it had remarkably increased. The National Labor Force Survey was not conducted in 1995. So, I employed predictions of missing values/imputation. The three most common methods to make these predictions (imputation) are prior knowledge, average (mean) value imputation, and regression (Çokluk & Kayri, Citation2011; Mertler et al., Citation2021). This prediction is carried out due to the MA (moving average) estimation requires a continuous sample.
5.2. Variable measurement
When someone is willing to work and has an education but is unable to find employment, they are said to be experiencing open unemployment. In the society, this type of unemployment is readily apparent. In this study, the open unemployment rate is the percentage of total unemployed to the labor force. I use several variables for robustness analysis, such as gross domestic product and labor forces based on age groups. I also provide other control variables, such as COVID-19 (dum2020, dum2021, and dum2022), lag 1 of open unemployment (AR1), and economic growth.
5.3. Box-Jenkins methodology
ARIMA (non-seasonal) model analysis can be explained through Box-Jenkins’ methods. In ARIMA modeling, the Box-Jenkins approach consists of the following stages ().
highlights the steps of a valid basis for forecasting. Box et al. (Citation1994) suggested that a non-seasonal version of ARIMA is symbolized as ARIMA (p, d, q). This methodology takes into consideration the historical data extracted and decomposed during the process of Autoregressive (AR), Moving Average (MA), Autoregressive Moving Average (ARMA) and Autoregressive Integrated Moving Average (ARMA) (Ljung & Box, Citation1979). EquationEquations (1)(1)
(1) and Equation(2)
(2)
(2) below demonstrate the common AR model of order p and the lag operator L.
(1)
(1)
where,
y is open unemployment rate.
εt ∼N(0,σ2) is the white noise.
(2)
(2)
Further, a common MA model of order q and the lag operator L can be seen in EquationEquations (3)(3)
(3) and Equation(4)
(4)
(4) .
(3)
(3)
(4)
(4)
In addition to AR and MA, the orders p and q (e.g., ARMA(p, q)) and lag operator L can be seen in EquationEquations (5–7).
(5)
(5)
(6)
(6)
(7)
(7)
Where,
Theoretically, an ARMA(p,q) model following differences of the d order required to make the series stationary is known as ARIMA(p,d,q). Thus, the ARIMA(p,d,q) model with the lag operator L can be seen in EquationEquation (8)(8)
(8) .
(8)
(8)
We can use the differential equation form to analyze the polynomial, as seen in EquationEquation (9)(9)
(9) .
(9)
(9)
EquationEquation (9)(9)
(9) takes the form of EquationEquation (10)
(10)
(10) .
(10)
(10)
Finally, we can provide an inversion form of the polynomial and random shock form, as seen in EquationEquations (11–13).
(11)
(11)
(12)
(12)
(13)
(13)
where:
ψ(L)=1 + ψ1L + ψ2L2+… is the random shock.
ψi is the ith parameter of ψ(L).
In addition, Mean Absolute Percentage Error (MAPE) is a popular model prediction accuracy metric. MAPE calculates the average magnitude of error produced by a model. The average absolute percentage difference between expected and actual values is defined as MAPE. Lewis (Citation1982) created a table with typical MAPE values ().
Table 1. Interpretation of MAPE value.
Automatic ARIMA forecasting is an ARIMA model-based approach for predicting values for a single series. EViews (Econometric Views) software provides an automated ARIMA set of forecasts that enables researchers to forecast possible open unemployment in Indonesia.
6. Empirical results and discussion
6.1. Descriptive statistics
Descriptive statistics summarize the data set that can represent a sample or population. shows the descriptive statistics.
Table 2. Descriptive statistics.
highlights the annual number of open unemployment, which comprised an average of 6.475, with the lowest number of cases being 2.550 and the highest number of open unemployment being 11.240. Meanwhile, the standard deviation of open unemployment data was 2.279. This implies that the number of open unemployment data was not stationary because it fluctuated on average and variance.
6.2. Stationarity test
I employ a stationarity test using trend and intercept. The open unemployment data transformation is noted in Dlog(unemployment). The results of the stationary test are shown in .
Table 3. Stationarity test.
demonstrates that the number of open unemployment in Indonesia is not stationary at the level order or I(0). This can be seen from the p-value of 0.8125, which is greater than the significance level (1, 5, and 10%). Nonetheless, Indonesia’s number of open unemployment has first-order or I(1) stationary. It can be seen from the p-value of 0.0005, which is smaller than the significance level (1, 5, and 10%). The results of the first-order transformation of data showed a relatively stable variance. Therefore, this data can be used to predict unemployment.
6.3. ARIMA model identification
The ARIMA system uses the Autocorrelation Function (ACF) and Partial Autocorrelation Function (PACF), which defines logarithmic distinction. shows the results of the ACF and PACF.
Table 4. ACF and PACF results.
shows the ACF plots had fallen to zero, and the PACF was out of interval on the small lags (lag 1, 2, and 4). This means the three lags were the best for modeling the open unemployment data. Thus, several alternative models [AR(1), AR(2), AR(4), ARMA(1,1), ARMA(1,2), ARMA(2,2)] can be used for forecasting as follows:
(14)
(14)
(15)
(15)
(16)
(16)
(17)
(17)
(18)
(18)
(19)
(19)
The equivalent models are:
Model 14 is the ARIMA model (1,1,0) for log(unemployment) data
Model 15 is the ARIMA model (2,1,0) for log(unemployment) data
Model 16 is the ARIMA model (4,1,0) for log(unemployment) data
Model 17 is the ARIMA model (1,1,1) for log(unemployment) data
Model 18 is the ARIMA model (1,1,2) for log(unemployment) data
Model 19 is the ARIMA model (2,1,2) for log(unemployment) data
6.4. Parameter estimation
To estimate the parameters, the six alternative ARIMA models must pass the normality and white noise assumption. The results of the assumption of normality and white noise will be shown in .
Table 5. Normality assumptions and white noise assumptions.
Looking at the statistics, it is noted that the six models have a normal distribution of error normality. This resulted in the overall model’s Jarque-Bera likelihood of being lower than the significance rate (5%). In addition, the six models have white noise and are suitable. The probability of the six models in the Ljung-Box (Q) was higher than the meaning level (5%). Selecting the best model based on normality and white noise assumptions is possible.
6.5. Selection of the best model
Model selection is choosing a statistical model based on data from a set of candidate models. shows the modeling results summary and the best model selection.
Table 6. ARIMA models summary.
The results of the overview models show that the AR(4) model is the best. The decision was based on the assumption that the minimum AIC and SBC values are present in the AR(4) model.
6.6. Static forecast method
For 2023, the AR(4) method was also used to estimate the number of open unemployment. The result was a visually stable forecast shown in .
presents three curves (upper, middle, and lower). The vertical axis reflected the unemployment rate, and the horizontal axis highlighted the periods. I use the middle curve as the static predicted value. Based on this Figure, there was 5.86 open unemployment in 2023. This indicates a decrease and stable number of open unemployment compared to 6.49 and 5.86 open unemployment in 2021 and 2022. The mean absolute percent error (MAPE) of 9.558923 (MAPE ≤10%) indicated highly accurate forecasting (Lewis, Citation1982). It demonstrates that the ARIMA model can predict Indonesia’s unemployment rate in the wake of demographic dividend (H1 supported).
6.7. Dynamic forecast method
In the subsequent 28 years, the AR(4) model was also used to predict the open unemployment data. As shown in and , the model can generate a visually dynamic forecast for the future.
Table 7. The predicted value for unemployment data.
The last three curves indicate the predicted upper, middle, and lower values. The vertical axis presented the unemployment rate, and the horizontal axis revealed the periods. We use the middle curve as the static predicted value. Based on , open unemployment decreased and stabilized from 2023 to 2050. also shows that Indonesia is facing a declining trend with open unemployment.
6.8. Discussion
The findings are reported from the Indonesian annual open unemployment rate series’ predictive between AR(1), AR(2), AR(4), ARMA(1,1), ARMA(1,2), and ARMA(2,2). This study showed that AR(4) was the best model to predict Indonesia’s future open unemployment between 2023 and 2050. The AR(4) model forecasting showed that open unemployment in Indonesia tends to decrease and stabilize continuously until 2050. My empirical findings support the hypothesis that the ARIMA model can predict Indonesia’s unemployment rate in the wake of the demographic dividend. This result was supported by Johnes (Citation1999), who said that linear autoregressive was the best model to predict the UK monthly unemployment rate rather than GARCH, threshold autoregressive and neural network models. Mahmudah et al. (Citation2017) supported this study, which found that Indonesia’s unemployment rate tends to decrease. Similarly, Ulhaq and Wahid (Citation2022) also support the current findings that Indonesia is set to experience a demographic dividend starting in 2025, according to the population projections produced by system dynamics modeling. According to Oey-Gardnier and Gardnier (Citation2013), a demographic dividend is simply waiting to happen (Oey-Gardnier & Gardnier, Citation2013). Although more deterministic economic-demographic models ensure a favorable result, a body of research indicates that a sizable economic dividend might not always occur and that the dividend would be better understood as a window of opportunity (de Carvalho & Wong, Citation1998; Pool, Citation2007). Indeed, it might even be a ‘curse’ if the nation faces many young people without jobs (Oey-Gardnier & Gardnier, Citation2013).
However, the empirical results different with several study findings (e.g., Dritsaki, Citation2016; Dritsakis & Klazoglou, Citation2018; Huang, Citation2015; Johnes, Citation1999; Kurita, Citation2010; Mahmudah et al., Citation2017; Montgomery et al., Citation1998; Nkwatoh, Citation2012; Romero-Ávila & Usabiaga, Citation2007; Wilson & Perry, Citation2004; Montgomery et al., Citation1998; Etuk et al., Citation2012; Larson & Sinclair, Citation2022; Naccarato et al., Citation2018; Nagao et al., Citation2019; Simionescu & Cifuentes-Faura, Citation2022, Larson & Sinclair, Citation2022, González-Fernández & González-Velasco, Citation2018; D’Amuri & Marcucci, Citation2017; Caperna et al., Citation2022; Aaronson et al., Citation2022; Fajar et al., Citation2020) because they only focused to forecast but my study focused on open unemployment forecasting related to demographic dividend and test the validity of Okun law during COVID-19 pandemic periods.
Indonesia was predicted to have a demographic dividend by 2030. The demographic dividend focuses on how the working-age group youth will dominate the population of Indonesia in 2030. In addition, the country was also expected to be one of the world’s economic giants. Population census data in 2018 shows that the population aged 15–64 reached 179.13 million, or about 67.6% of the total population in Indonesia (Central Bureau of Statistics, Citation2018). Indonesia’s economic development from 2014 until the present has shown positive changes. The fact shows that economic growth has risen from 4% (2015) to 5.2 (2018), Gross Domestic Product per capita has increased from USD 3,332 (2015) to USD 3,894 (2018), poverty has fallen from 11.22% (March 2015) to 9.41% (March 2019), and inflation has fallen from 3.4% (2015) to 3.1% (2018) (Indonesian Ministry of National Development Planning, Citation2019). In addition, the sixteen economic policy packages to enhance the competitiveness of the national industry, exports and investment are summarized in .
Table 8. The sixteen economic policy packages.
The role of the young generation is closely related to economic growth and development (Mason & Kinugasa, Citation2008). Youth between 15 and 24 make up one-third of all productive-age workers. In addition, the young generation is predicted to increase real output by 5% in developing countries (Febyolla, Citation2019). The demographic dividend could be if a better education is available to the younger generation (Kaneko & Sato, Citation2013; Mason & Kinugasa, Citation2008; Ogawa et al., Citation2005, Citation2010; Stephen, Citation2012). Interestingly, the increased labor force implies the achievement of expansion labor programs implemented by the Indonesian government.
In addition, Indonesia’s government could also focus on investing in human resources. It can be understood that a country could move forward when it has a good human resource investment (Mason & Kinugasa, Citation2008; Ogawa et al., Citation2005, Citation2010). In 2020, Indonesia’s expenditure regarding increased productivity and competitiveness of human resources (e.g., expanding access to education, growing skills, entrepreneurship, mastering Information Communication Technology, and supporting research activities) was IDR 508.1 trillion. Regarding human resource investment, Indonesia’s government also pays attention to the health sector (Kim et al., Citation2019; Mason & Kinugasa, Citation2008; Ogawa et al., Citation2005, Citation2010). In the same year, the government of Indonesia provided IDR 132.2 trillion to accelerate stunting reduction, preventive promotive strengthening, and the continuation of the national health insurance program (Indonesian Ministry of Finance, Citation2019).
Concerning Indonesia’s 2030 demographic dividend, the government also focuses on infrastructure. Infrastructure for welfare (20 October 2014, to present) became Indonesian government jargon. Indonesia’s government provides IDR 423.3 trillion to increase investment, export competitiveness, industry transformation (e.g., connectivity, food, energy, and water), and anticipate urban social problems (e.g., clean water, sanitation, waste management & mass transportation). This policy is aimed at improving infrastructure to compete with other countries. Such policies (investment in human resources and infrastructure) should be implemented from now on. Therefore, Indonesia could be part of the world economy’s Top Five (Indonesian Ministry of National Development Planning, Citation2019). In addition, the World Bank (Citation2014) also suggested that policymakers focus on improving cities by improving infrastructure and enhancing services for urbanizing economies, such as China, Indonesia, the Philippines, and Vietnam.
A robustness analysis demonstrates how my findings support the hypothesis testing. In this case, I ran a regression regarding the impact of the gross domestic product on labor forces based on age groups. It can be seen on .
Table 9. The impact of GDP on labor forces based on age groups (1990–2022).
demonstrates that GDP positively and significantly impacts labor forces aged between 15 and 19 years. It can be understood that when GDP increases, the number of labor forces in this age group tends to increase (holding other independent variables constant). In other words, the 15 to 19 age group prefer not to continue studying in universities instead of entering the labor market. These labor forces opt not to continue their education to tertiary levels because they consider this option will increase their future productivity and outputs.
I also provided a sensitivity test using economic growth, autoregressive (AR1), and COVID-19 dummies to explain the impact of COVID-19 periods on unemployment in Indonesia ().
Table 10. The impact of COVID-19 periods on unemployment.
At the end of 2019, the world is facing big problems. It started with the emergence of a disease outbreak caused by the coronavirus so called COVID-19. My empirical results show that the biggest impact of COVID-19 on unemployment comes from 2020 (DUM2020). The data of Indonesia’s open unemployment rate at 7.07, 6.49 and 5.86% from 2020 to 2022 confirmed it. Compared to February 2020, the open unemployment rate has grown dramatically to 1.32% due to the COVID-19 pandemic (Central Bureau of Statistics, Citation2021). This will undoubtedly impact Indonesia’s economic growth. Since society’s low production of labor and goods will slow Indonesia’s economy’s expansion rate. Furthermore, the regression results did not support the Okun law’s validity for COVID-19 periods.
7. Summary and conclusion
The six alternative models, AR(1), AR(2), AR(4), ARMA(1,1), ARMA(1,2) and ARMA(2,2) were used to estimate the number of open unemployment in Indonesia. The model summary provided evidence that from 1990 to 2022 the AR(4) was the best model for forecasting the number of open unemployment in Indonesia. ARIMA forecasting using AR(4) revealed that open unemployment tends to decrease from 2023 to 2050. Forecasting of open unemployment plays an important role in supporting the demographic dividend faced in 2030. Several factors support the demographic dividend, such as the realization of sixteen economic policy packages, improvement of the human resources quality policy, and acceleration of infrastructure development policy. In addition, I provided evidence that the increased labor force implies the achievement of labor expansion programs implemented by Indonesia’s government. An attractive investment prospect is a country with a young population. The demographic dividend will create various new jobs and increase the workforce significantly. Therefore, the demographic dividend in 2030 is the way to becoming one of the economic giants of the Top Five worlds.
The decrease and stabilize open unemployment are opportunities and challenges for Indonesia to optimize the demographic dividend that will end in 2045 (Indonesia’s Golden Generation). The demographic dividend will create various jobs (leisure economy) and significantly increase the workforce. At the same time, Japan and South Korea are experiencing an aging population (Han-Hyung, Citation2009; Kim et al., Citation2019; Oku et al., Citation2017; Stephen, Citation2012; Usman & Tomimoto, Citation2013). Therefore, an attractive investment prospect is a country with a young population, particularly in Indonesia.
7.1. Implications
This study proved the number of open unemployment tends to decrease and stable from 2023 to 2050. The decrease and stable number of open unemployment is an opportunity and a challenge for Indonesia to optimize the demographic dividend in 2030. However, a demographic dividend can become a disaster if employment opportunities cannot absorb the workforce. Thus, Indonesia’s government must manage labor, health, and infrastructure spending well to face the demographic dividend in 2030. This demographic dividend has two sides, like a two-edged blade. It will be a terrible demographic disaster if we can’t control it. If we manage this demographic dividend effectively, it will become a remarkable economic resource.
The quality of the workers is equally as important as the quantity when it comes to realizing Indonesia’s potential because productivity is directly correlated with dividend realization. The education system in Indonesia needs more attention from the government since it is still growing and improving, at least in quantity. Education has improved due to the dynamics between an increasing number of youths, creating a demand for education that is continually raised and affecting both economic growth and the quality of labor. Changes in the labor force may also impact the ability to benefit from the demographic dividend. In addition, the Indonesian government needs to increase funding for job retraining and up-skilling programs for workers who have lost their jobs during the crisis (e.g., COVID-19). My findings contribute to the body of knowledge on unemployment prediction, demographic dividend, and the validity of Okun law during the COVID-19 pandemic periods.
7.2. Limitation and future research
Along with its contributions to the literature concerning unemployment prediction, demographic dividend, and the validity of Okun law during the COVID-19 pandemic periods, this study also had some limitations. First, this study only focuses on forecast open unemployment data as a whole (national). So, future studies could propose a data-driven procedure linked to the phenomenon of interest in a cross-province or cross-country setting. Second, this study only focuses on forecasting the general open unemployment rate. Therefore, future studies could analyze the demographic dividend related to youth unemployment. Future studies could also consider forecasting based on gender (e.g., male and female unemployment) and age (e.g., youth unemployment). Third, the short-term forecasts can be used with ARIMA models. Thus, future studies should have more extended forecasts for random time-series disruptions. Future studies are necessary to consider various models such as Spectral analysis, Long Short-Term Memory (LSTM), Convolutional Neural Network (CNN), Convolutional Long Short-Term Memory (ConvLSTM), etc.
Disclosure statement
No potential conflict of interest was reported by the author(s).
Data availability statement
Data is available upon reasonable request.
Additional information
Funding
Notes on contributors
Andrian Dolfriandra Huruta
Andrian Dolfriandra Huruta is an Assistant Professor at the Department of Economics, Faculty of Economics and Business, Satya Wacana Christian University, Indonesia. His primary research interests are macroeconomics, econometrics, and international trade. He got his PhD from Chung Yuan Christian University, Taiwan. He has been selected for membership in Beta Gamma Sigma as the international honor society for collegiate school of business and The Phi Tau Phi Scholastic Honor Society of the Republic of China.
References
- Aaronson, D., Brave, S. A., Butters, R. A., Fogarty, M., Sacks, D. W., & Seo, B. (2022). Forecasting unemployment insurance claims in realtime with Google Trends. International Journal of Forecasting, 38(2), 1–21. https://doi.org/10.1016/j.ijforecast.2021.04.001
- Abdou, H. A., Tsafack, M. D. D., Ntim, C. G., & Baker, R. D. (2016). Predicting creditworthiness in retail banking with limited scoring data. Knowledge-Based Systems, 103, 89–103. https://doi.org/10.1016/j.knosys.2016.03.023
- Adioetomo, S. R. (2006). Age-structural transitions and their implications: the case of Indonesia over a century, 1950–2050. In: I., Pool, L. R., Wong, & E. Vilquin (Eds.), Age-structural Transitions: Challenges for Development (pp. 129–157). Paris: CICRED.
- Barnichon, R., & Nekarda, C. J. (2012). The ins and outs of forecasting unemployment: Using labor force flows to forecast the labor market. Brookings Papers on Economic Activity, 2012(1), 83–131. https://doi.org/10.1353/eca.2012.0018
- Boga, S. (2020). Investigating the asymmetry between economic growth and unemployment in Turkey: A hidden cointegration approach. Pressacademia, 7(1), 22–33. https://doi.org/10.17261/Pressacademia.2020.1178
- Box, G. E. P., Jenkins, G. M., & Reinsel, G. C. (1994). Time series analysis; forecasting and control (3rd ed.). Prentice Hall.
- Brito, R. D., & Carvalho, C. (2015). Macroeconomic effects of the demographic transition in Brazil. In J. M. Fanelli (Ed.), Asymmetric demography and the global economy (pp. 151–185). Palgrave Macmillan.
- Byrialsen, M. R., & Raza, H. (2018). Macroeconomic effects of unemployment benefits in small open economies: A stock–flow consistent approach. European Journal of Economics and Economic Policies: Intervention, 15(3), 335–363. https://doi.org/10.4337/ejeep.2018.0032
- Caperna, G., Colagrossi, M., Geraci, A., & Mazzarella, G. (2022). A babel of web-searches: Googling unemployment during the pandemic. Labour Economics, 74, None. https://doi.org/10.1016/j.labeco.2021.102097
- Central Bureau of Statistics. (2018). Statistik Indonesia dalam Infografis 2018. Central Bureau of Statistics. https://www.bps.go.id/publication/2018/12/24/39b2ed48b00f0e785046d37d/statistik-indonesia-dalam-infografis-2018.html
- Central Bureau of Statistics. (2021). Berita Resmi Statistik: Keadaan Ketenagakerjaan Indonesia Februari 2021. Central Bureau of Statistics.
- Central Bureau of Statistics. (2022). Analysis of Indonesia population profile. In W. Winardi, (Ed.), Badan Pusat Statistik. Central Bureau of Statistics.
- Chodorow-Reich, G., & Coglianese, J. (2021). Projecting unemployment durations: A factor-flows simulation approach with application to the COVID-19 recession. Journal of Public Economics, 197, 104398. https://doi.org/10.1016/j.jpubeco.2021.104398
- Çokluk, Ö., & Kayri, M. (2011). The effects of methods of imputation for missing values on the validity and reliability of scales. Kuram ve Uygulamada Egitim Bilimleri, 11(1), 303–309.
- D’Amuri, F., & Marcucci, J. (2017). The predictive power of Google searches in forecasting US unemployment. International Journal of Forecasting, 33(4), 801–816. https://doi.org/10.1016/j.ijforecast.2017.03.004
- de Carvalho, J. A. M., & Wong, L. R. (1998). Demographic and socioeconomic implications of rapid fertility decline in Brazil: A window of opportunity. In G. Martine, M. Das Gupta, & Chen, L (Eds.), Reproductive change in India and Brazil (pp. 208–240). Oxford: Oxford University Press.
- Dritsaki, C. (2016). Forecast of Sarima models: Αn application to unemployment rates of Greece. American Journal of Applied Mathematics and Statistics, 4(5), 136–148. https://doi.org/10.12691/ajams-4-5-1
- Dritsakis, N., & Klazoglou, P. (2018). Forecasting unemployment rates in USA using Box–Jenkins methodology. International Journal of Economics and Financial Issues, 8(1), 9–20. https://www.econjournals.com/index.php/ijefi/article/view/5550/pdf.
- Drummond, P., Thakoor, V., & Yu, S. (2014). Africa rising: Harnessing the demographic dividend (WP/14/143; IMF Working Paper). https://www.imf.org/external/pubs/ft/wp/2014/wp14143.pdf.
- Etuk, E. H., Uchendu, B., & Victor-Edema, U. (2012). ARIMA fit to Nigerian unemployment data. Journal of Basic and Applied Scientific Research, 2(6), 5964–5970. https://pdfs.semanticscholar.org/3482/6072fe6205740fd4ab2ced3488d4e0fe3b25.pdf?_ga=2.61529260.1831424978.1570262485-1772847037.1568539219.
- Fajar, M., Rizky Prasetyo, O., Fajar, M., Prasetyo, O. R., & Nonalisa, S. (2020). Forecasting unemployment rate in the time of COVID-19 pandemic Using Google Trends data (case of Indonesia). International Journal of Scientific Research in Multidisciplinary Studies, 6(11), 29–33.
- Febyolla. (2019). Demographic bonus: Threat or opportunity? Faculty of Economics and Business – Gadjah Mada University. https://feb.ugm.ac.id/en/news/2625-demographic-bonus-threat-or-opportunity.
- Floros, C. (2005). Forecasting the UK unemployment rate: Model comparisons. International Journal of Applied Econometrics and Quantitative Studies, 2(4), 57–72. https://core.ac.uk/download/pdf/52395341.pdf.
- González-Fernández, M., & González-Velasco, C. (2018). Can Google econometrics predict unemployment? Evidence from Spain. Economics Letters, 170, 42–45. https://doi.org/10.1016/j.econlet.2018.05.031
- Gribble, J. N., & Bremner, J. (2012). Achieving a demographic dividend. Population Bulletin, 67(2), 1–15. https://assets.prb.org/pdf12/achieving-demographic-dividend.pdf.
- Han-Hyung, P. (2009). South Korea’s looming aging crisis “demographic change & challenges of graying ahead”. Japan Spotlight, 164, 26–27.
- Harkat, T., & Driouchi, A. (2017). Demographic dividend & economic development in Arab Countries. Munich personal RePEc archive: Vol. November (Munich Personal RePEc Archive, Issue 82880). https://mpra.ub.uni-muenchen.de/82880/.
- Huang, X. (2015). Forecasting the US unemployment rate with a Google job search index [open access Master’s Theses]. University of Rhode Island. https://pdfs.semanticscholar.org/da65/14fe2a4858dc957f6bec1bdc9fa22deadff1.pdf.
- Hull, T. H., Hull, V. J., & Singarimbun, M. (1977). Indonesia’s family planning story: Success and challenge. Population Bulletin, 32(6), 1–52.
- Indonesian Ministry of Finance. (2019). APBN 2020 APBN Akselerasi Daya Saing melalui Inovasi dan Penguatan Kualitas Sumber Daya Manusia. Jakarta: Indonesian Ministry of Finance.
- Indonesian Ministry of National Development Planning. (2019). Perjalanan Perekonomian Indonesia. Jakarta: Indonesian Ministry of National Development Planning.
- International Monetary Fund. (2019). Indonesia unemployment forecast 2019–2024, data and charts. International Monetary Fund. https://knoema.com/stvidq/indonesia-unemployment-forecast-2019-2024-data-and-charts.
- Jo, C., Kim, D. H., & Lee, J. W. (2023). Forecasting unemployment and employment: A system dynamics approach. Technological Forecasting and Social Change, 194, 122715. https://doi.org/10.1016/j.techfore.2023.122715
- Johnes, G. (1999). Forecasting unemployment. Applied Economics Letters, 6(9), 605–607. https://doi.org/10.1080/135048599352709
- Kaneko, R., & Sato, R. (2013). Entering the post-demographic transition phase in Japan: Dynamic social changes toward new population regime. XXVII IUSSP International Population Conference, 1–8. International Union for the Scientific Study of Population. https://iussp.org/sites/default/files/event_call_for_papers/ExtendedAbstract(KanekoSato20121030).pdf.
- Kim, J., Jang, M., & Shin, D. (2019). Examining the role of population age structure upon residential electricity demand: A case from Korea. Sustainability, 11(14), 3914. https://doi.org/10.3390/su11143914
- Kurita, T. (2010). A forecasting model for Japan’s unemployment rate. Eurasian Journal of Business and Economics, 3(5), 127–134. https://doi.org/10.5539/mas.v7n7p10
- Larson, W. D., & Sinclair, T. M. (2022). Nowcasting unemployment insurance claims in the time of COVID-19. International Journal of Forecasting, 38(2), 635–647. https://doi.org/10.1016/j.ijforecast.2021.01.001
- Lee, R. D., & Mason, A. (2012). Lower-income countries and the demographic dividend. NTA Bulletin, 5, 1–8. https://www.eastwestcenter.org/system/tdf/private/ntabulletin005_1.pdf?file=1&type=node&id=33908.
- Lewis, C. D. (1982). Industrial and business forecasting methods. Butterworths.
- Ljung, G. M., & Box, G. E. P. (1979). The likelihood function of stationary autoregressive-moving average models. Biometrika, 66(2), 265–270. https://doi.org/10.1093/biomet/66.2.265
- Loewe, M. (2007). A demographic dividend for the developing countries? Consequences of the global aging process (6/2007). https://www.die-gdi.de/uploads/media/6_2007_EN.pdf.
- Mahmudah, U., Universiti Malaysia Terengganu, Malaysia. (2017). Predicting unemployment rates in Indonesia. Economic Journal of Emerging Markets, 9(1), 20–28. https://doi.org/10.20885/ejem.vol9.iss1.art3
- Mason, A., & Kinugasa, T. (2008). East Asian economic development: Two demographic dividends. Journal of Asian Economics, 19(5–6), 389–399. https://doi.org/10.1016/j.asieco.2008.09.006
- Mertler, C. A., Vannatta, R. A., & LaVenia, K. N. (2021). Advanced and multivariate statistical methods: Practical application and interpretation. Routledge.
- Montgomery, A. L., Zarnowitz, V., Tsay, R. S., & Tiao, G. C. (1998). Forecasting the U.S. unemployment rate. Journal of the American Statistical Association, 93(442), 478–493. https://doi.org/10.1080/01621459.1998.10473696
- Naccarato, A., Falorsi, S., Loriga, S., & Pierini, A. (2018). Combining official and Google Trends data to forecast the Italian youth unemployment rate. Technological Forecasting and Social Change, 130, 114–122. https://doi.org/10.1016/j.techfore.2017.11.022
- Nagao, S., Takeda, F., & Tanaka, R. (2019). Nowcasting of the U.S. unemployment rate using Google Trends. Finance Research Letters, 30, 103–109. https://doi.org/10.1016/j.frl.2019.04.005
- Nkwatoh, L. S. (2012). Forecasting unemployment rates in Nigeria using univariate time series models. International Journal of Business and Commerce, 1(12), 33–46. http://www.ijbcnet.com/1-12/IJBC-12-11202.pdf
- Ntim, C. G., English, J., Nwachukwu, J., & Wang, Y. (2015). On the efficiency of the global gold markets. International Review of Financial Analysis, 41, 218–236. https://doi.org/10.1016/j.irfa.2015.03.013
- Ntim, C. G., Opong, K. K., Danbolt, J., & Dewotor, F. S. (2011). Testing the weak-form efficiency in African stock markets. Managerial Finance, 37(3), 195–218. https://doi.org/10.1108/03074351111113289
- Oey-Gardnier, M., & Gardnier, P. (2013). Indonesia’s demographic dividend or window of opportunity? Masyarakat Indonesia: Jurnal Ilmu-Ilmu Sosial Indonesia, 39(2), 481–504.
- Ogawa, N., Kondo, M., & Matsukura, R. (2005). Japan’s transition from the demographic bonus to the demographic onus. Asian Population Studies, 1(2), 207–226. https://doi.org/10.1080/17441730500317451
- Ogawa, N., Mason, A., Chawla, A., & Matsukura, R. (2010). Japan’s unprecedented aging and changing intergenerational transfers. In T. Ito, & A. Rose (Eds.), The economic consequences of demographic change in East Asia (Vol. 19, Issue August, pp.131–160). University of Chicago Press.
- Oku, A., Ichimura, E., & Tsukamoto, M. (2017). Aging population in Asian countries ‘lessons from Japanese experiences’. PRI discussion paper series (No. 17A-12; PRI Discussion Paper Series, Vol. 17A, Issue 12). https://www.mof.go.jp/pri/research/discussion_paper/ron299.pdf.
- Okun, A. M. (1962). Potential GNP: Its measurement and significance (No. 190; Cowles Foundation Paper). https://milescorak.files.wordpress.com/2016/01/okun-potential-gnp-its-measurement-and-significance-p0190.pdf.
- Pool, I. (2007). Demographic dividends: Determinants of development or merely windows of opportunity? Ageing Horizons, 7, 28–35.
- Romero-Ávila, D., & Usabiaga, C. (2007). Unit root tests, persistence, and the unemployment rate of the U.S. States. Southern Economic Journal, 73(3), 698–716. https://www.jstor.org/stable/20111919 https://doi.org/10.1002/j.2325-8012.2007.tb00797.x
- Rublikova, E., & Lubyova, M. (2013). Estimating Arima – Arch model rate of unemployment in Slovakia. Prognostické Práce, 5(3), 275–289. http://www.prog.sav.sk/sites/default/files/2018-03/PP_5_2013_3_clanok3_Rublikova_Lubyova.pdf.
- Simionescu, M. (2015). Predicting the national unemployment rate in Romania using a spatial auto-regressive model that includes random effects. Procedia Economics and Finance, 22, 663–671. https://doi.org/10.1016/s2212-5671(15)00281-6
- Simionescu, M., & Cifuentes-Faura, J. (2022). Can unemployment forecasts based on Google Trends help government design better policies? An investigation based on Spain and Portugal. Journal of Policy Modeling, 44(1), 1–21. https://doi.org/10.1016/j.jpolmod.2021.09.011
- SMERU Research Institute. (2021). Study on the impact of COVID-19 pandemic on the creation of sectoral employment opportunities, national development planning program. The SMERU Research Institute. https://smeru.or.id/en/research/study-impact-covid-19-pandemic-creation-sectoral-employment-opportunities-national.
- Stampe, M. Z., Porsse, A. A., & Portugal, M. S. (2013). Demographic change and economic growth in Brazil: An exploratory spatial data analysis. Simpósio Internacional de Pós-Graduação Em Enfermagem, 1–36. https://pdfs.semanticscholar.org/a9c8/245177798af313f9cd6eb343eaf6365ed6fe.pdf?_ga=2.161739324.1013202980.1573055444-1772847037.1568539219.
- Stephen, E. H. (2012). Bracing for low fertility and a large elderly population in South Korea. KEI Academic Paper Series. http://www.keia.org/sites/default/files/publications/aps_doc_elizabeth_stephens.pdf.
- Todaro, M. P., & Smith, S. C. (2015). Economic development (12th ed.). Pearson.
- Ulhaq, M. D., & Wahid, A. (2022). System dynamics modeling for demographic bonus projection in Indonesia. IOP Conference Series: Earth and Environmental Science, 1039(1), 012031. https://doi.org/10.1088/1755-1315/1039/1/012031
- United Nations. (2009). World population prospects: The 2008 revision. United Nations.
- Usman, M., & Tomimoto, I. (2013). The aging population of Japan: Causes, expected challenges and few possible. Research Journal of Recent Sciences, 2(11), 1–4.
- Wilson, P. J., & Perry, L. J. (2004). Forecasting Australian unemployment rates using spectral analysis. Australian Journal of Labour Economics, 7(4), 459–480. https://businesslaw.curtin.edu.au/wp-content/uploads/sites/5/2016/05/AJLE-v7n4-wilson.pdf.
- World Bank. (1990). The East Asian miracle: Economic growth and public policy. World Bank.
- World Bank. (2014). East Asia Pacific at work: employment, enterprise & well-being. World Bank. https://www.indonesia-investments.com/news/news-columns/world-bank-report-east-asia-pacific-at-work-employment-enterprise-and-well-being/item1976.
- Yamacli, D. S., & Yamacli, S. (2023). Estimation of the unemployment rate in Turkey: A comparison of the ARIMA and machine learning models including Covid-19 pandemic periods. Heliyon, 9(1), e12796. https://doi.org/10.1016/j.heliyon.2023.e12796