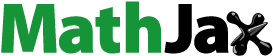
Abstract
Modernisation of the sugar industry in Indonesia, particularly at the off-farm level, has been slow, with most sugar factories still using old factory machinery. However, this can be improved through the use of technology to build a sustainable sugar industry. Technological advances in the Industry 4.0 era require the preparation of professional human resources, ready to compete in the global arena. In relation to these conditions, this study aims to evaluate technology in the sugarcane production process and to map the competency units of human resources in the sugarcane production process. The research method to evaluate the technology in the sugarcane production process uses two decision-making methods, namely non-numerical and numerical decision-making. The recommendation obtained is that the cut-load-transport section is a priority technology to be replaced by a new technology. The results of the evaluation were then used to map the competence units of human resources in sugar production. Competency unit mapping was carried out in the cut-load-transport and milling sections and then combined by adopting the existing refined crystal sugar work competency standards. The results of the competency mapping design can be used by sugar factories to increase the productivity of human resources in sugar factories, which does not yet exist in Indonesia.
1. Introduction
The decline of the sugar agro-industry was due to inefficiencies in cane growing, sugar factories and distortions in the international sugar market (Heryanto et al., Citation2020). However, future workers in the cane sugar agro-industry need to be considered with regard to certification in the Industry 4.0 era. In order to ensure the effectiveness of the sugar production process, it is strategic to manage individual competencies and map their development (Kipper et al., Citation2021; Rout et al., Citation2018; Takey & Carvalho, Citation2015) Human Resources (HR) in the Sugar Agro-Industry in the Industry 4.0 Era. In terms of HR certification and the cane sugar industry, there are no HR competency standards in the cane sugar sector in Indonesia (Mursiti et al., Citation2021). Because to produce quality sugar that meets the standards requires human resources who have the knowledge, skills, and work attitudes in operating equipment in the sugar production process. For this reason, efforts are needed to improve human resources in the industrial era 4.0. In order to ensure that HR competencies are in accordance with what is expected by the industry, it is necessary to evaluate the application of production process technology used by sugar factories today. This reason is one of the triggers for researchers to evaluate sugar production process technology and sugar cane competency standards.
In Indonesia, there is a competency standard for refined crystal sugar issued by the Decree of the Minister of Manpower of the Republic of Indonesia No. 178 of 2000 on the Determination of the Indonesian National Work Competency Standards for the Processing Industry Category of the Food Industry Principal Group of the Refined Crystal Sugar Industry in the Quality Control and Production Sub-Sector. This research aims to evaluate the sugar production process technology that needs to be replaced with new technology and to map the human resource competency units in the sugar production process. Rangnekar (Citation2020) emphasises the understanding of different skills and how these skills are used by employees to contribute to the organisation. Given the importance of HR associated with certifications such as certifications in graphic design schemes, computer operators and others. The researcher proposes here the process of designing human resource competency mapping in the cane sugar production process.
The method used to evaluate the sugar production process technology to be replaced by new technology uses 2 methods, namely non-numerical and numerical decision making. The results of both methods are used to compare the results of the recommendations obtained. The non-numerical decision making used is multi-expert multi-criteria decision making (MEMCDM). The results of both non-numerical and numerical decision-making methods show that cut-load-transport is a priority process technology in sugar production that needs to be replaced by new technology. The methodology for formulating competency standards is at least proven by a training certificate for formulating Indonesian national competency standards. The technical content is proven by relevant experience; company recognition or recommendation; certificate of competence. This research is limited to competency mapping, which is arranged in an order of job functions that include main objectives, key functions, main functions, and basic functions or units of competence. The formulation can be done by field research, adaptation or adoption. The formulation of competency mapping is verified and validated. The purpose of competency mapping is to identify and map the units of competence of an industry sector that need to be compiled into competency standards.
2. Literature review
2.1. Agriculture 4.0
Agriculture 4.0 is an inevitable trend in modern agriculture. The foundations of Agriculture 4.0 are the Internet of Things, big data, artificial intelligence, cloud computing and connectivity (Albiero et al., Citation2020). Agriculture 4.0 is expected to increase agricultural yields, save input costs, and in many situations reduce human resources. Agriculture 4.0 is expected to increase agricultural yields, reduce input costs and, in many situations, reduce human resources. Agriculture 4.0 is also expected to improve environmental health by increasing food production on existing land and improving eco-efficiency (Ehlers et al., Citation2022). In addition, the contribution of the agricultural sector to Indonesia’s GDP is quite high at 13.22% and has shown the ability to maintain positive growth during the Covid-19 pandemic (BPS, Citation2022).
The development of national industry in 2020–2024 is geared towards the implementation of Making Indonesia 4.0, which focuses more on five industrial sectors, namely: (1) food and beverages; (2) textiles and fashion; (3) automotive; (4) chemicals; and (5) electronics (Bappenas, Citation2020). Mastering science and technology and innovation in line with Industry 4.0 is the key to a nation’s global competitiveness in the future. According to Alhloul and Kiss (Citation2022), Industry 4.0 has ten main technologies that drive this revolution as follows (1) Industrial Internet of Things (Araújo et al., Citation2021; Fatimah et al., Citation2023; Harry, Citation2020; Raj et al., Citation2021); (2) industrial cloud computing (Bello et al., Citation2021; Oruma et al., Citation2021); (3) big data (Chowdhury et al., Citation2020); (4) simulation (Asrol et al., Citation2021; Raj et al., Citation2021; Zhai et al., Citation2020); (5) augmented reality (Alam et al., Citation2023; Kipper et al., Citation2021; Liu et al., Citation2023); (6) additive manufacturing (Alcácer & Cruz-Machado, Citation2019; Blayone & VanOostveen, Citation2021; Fonseca, Citation2018; Hahn, Citation2020); (7) horizontal and vertical system integration (Fuertes et al., Citation2022; Hasbullah et al., Citation2021; Pérez-Lara et al., Citation2019); (8) artificial intelligent (Fatimah et al., Citation2023; Fachrunnisa & Hussain, Citation2020; Flores et al., Citation2020; Zhang & Lu, Citation2021); (9) cybersecurity (Aldhyani & Alkahtani, Citation2023; Stachová et al., Citation2020; Silva et al., Citation2023); (10) cyber-physical system (Kadir & Broberg, Citation2021; Luthra et al., Citation2020).
2.2. Skills 4.0 for human resources
Competence is an individual’s ability to work and includes aspects of knowledge, skills and attitudes that are in line with expected standards (Jaskiran Kaur et al., Citation2023). Another definition is that competence is something related to the ability and skills of individuals to achieve expected results (Lambert & Bouchamma, Citation2019). Competence is a combination of a person’s attributes, abilities, skills, knowledge and experience required to perform roles in life and work (Hecklau et al., Citation2016). Based on this definition, some important things related to competence are knowledge, attitudes, understanding, values, talents or skills and interests. Competences can also be defined as individual characteristics that can be measured and determined to indicate certain behaviour and work performance in an individual. Competence is therefore a guide for organisations to indicate the right job function for an employee. Competencies are related to attitudes (what a person says and does) that indicate a person’s performance is good or bad.
The rapid advancement of communication and information technologies in the psycho-social structure of societies, cities and industries through smartphones, IoT and AI is due to their perceived ease of use and cost savings. This has led to their widespread use, resulting in an increasing dependency on these functions. It can be said that people are now in an almost symbiotic synergy with digital technology (Albiero et al., Citation2020). For this reason, improving the skills of the workforce is important for increasing industrial productivity in the Industry 4.0 era (Chryssolouris et al., Citation2013). Competence is a combination of a person’s attributes, abilities, skills, knowledge and experience required to perform a job (Meyer et al., Citation2015). According to Miao et al. (Citation2017) that digital skills and emotional intelligence have a proven impact on job performance. According to Flores et al. (Citation2020) that HR competencies include (1) soft competencies, (2) hard competencies, (3) cognitive competencies, (4) emotional competencies, and (5) digital competencies. Soft competence is a collection of traits that reflect a person’s social gifts in a particular environment (Haeffner & Panuwatwanich, Citation2018). Hard skills, also known as technical skills, are the skills and knowledge required to carry out a trade, craft or occupation that requires special skill, training or experience (Robles, Citation2012). Cognitive ability, or intelligence quotient (IQ), is the intellectual ability that enables learning to support the mastery, skill and performance of specific tasks (Kautz et al., Citation2014). Emotional skills or emotional intelligence is the ability to understand and regulate one’s emotions, which is expected to lead to success through better ability to cope with new challenges and resilience to frustration and stress (Rezvani et al., Citation2016). Digital literacy is the ability to understand and use digital content, devices and systems to carry out activities.
Vuorikari et al. (Citation2016) has developed a framework that addresses five areas of digital literacy development, as shown in . The framework provides a very comprehensive view, covering all digital skills and activities, primarily in five areas and two dimensions.
Table 1. DigComp 2.0 framework for five digital skill areas.
In relation to the certification of personnel in the cane sugar agro-industry, it was found that there is no regulation on the standards of work competence in the cane sugar agro-industry, let alone the levelling of positions in sugar factories (Mursiti et al., Citation2021). This is important to ensure the competence of the workforce to perform their duties correctly and well. In relation to work competency standards in Indonesia for the sugar industry, the government has issued Decree of the Minister of Manpower of the Republic of Indonesia No. 178 of 2020 on the determination of Indonesian national competency standards category of processing industry main group of food industry in the field of refined crystal sugar industry in the subfield of quality control (Decree of the Minister of Labour RI No 178 Year 2020 about the Determination of Indonesian national competency standards Category Processing Industry Main Group Food Industry Refined Crystal Sugar Industry Sector, Citation2020). Cane sugar and refined sugar have similarities and differences, the similarity being as a sweetener. The difference lies in the production process, product standards and labelling. In the production process, cane sugar has a longer stage, there is sugar cane harvesting and sugar cane milling, but the production process after milling is the same. In terms of quality, cane sugar has a lower colour standard than refined sugar. In Indonesia, cane sugar is consumed by households and refined sugar by the food and beverage industry.
Previous research on competency mapping has proposed a seven-step method for project management competency maps (Takey & Carvalho, Citation2015). Explore the extent to which competency mapping can assist in analysing gaps in required skills and be followed up to improve competency levels (Jaideep Kaur & Kumar, Citation2013), learn how competency mapping can be used to analyse the mix of skills of different people to create the most cohesive teams and produce higher quality work (Jaskiran Kaur et al., Citation2023), describes a new mapping software that simplifies and standardises the competency mapping process (Holmes et al., Citation2018), discuss the implications of the recent competency mapping project for education and training programmes for public health workers (Coombe et al., Citation2022), explore the integrated relationship between talent acquisition competency mapping and performance management and appraisal in the Indian context (Rangnekar, Citation2020), no standardised benchmarks for achieving competence and open to individual interpretation (Perera et al., Citation2013).
3. Research methods
The research began with an evaluation of sugar production process technology in one sugar factory and all Indonesian sugar factories. This was done to compare the research results of the two research objects regarding the similarities and differences between them. The data processing techniques used are non-numerical multi-expert multi-criteria decision making and numerical decision-making methods. The data obtained is primary data through distributing questionnaires with different instruments for both. The preparation of the instrument is based on the research objectives and has been verified by experts. The experts selected here have predetermined qualifications. The qualifications include (1) the expert has experience in the sugar industry for at least 10 years, (2) has a position equivalent to manager and above, (3) has competence recognition in the sugar industry from external parties. Furthermore, the research results obtained from the two objects were used as a consideration to compile the mapping of sugar cane agro-industry competency units. Other considerations used to compile this unit of competency mapping are Indonesian national competency standards Refined Crystal Sugar Bidang Quality Control and Production which includes the process of purification, evaporation, crystallisation, separation and completion.
In , before conducting the competency mapping, the researchers first evaluated the technology of the sugar production process to find out which part of the technology requires new technology. The evaluation was carried out on sugar factories that have not implemented 4.0 technology and all sugar factories throughout Indonesia using 2 (two) different methods, namely non-numerical multi-expert multi-criteria decision making and numerical decision making through questionnaires with different instruments with the same purpose. The questionnaire was sent to experts in sugar factories with predetermined criteria. The criteria are (1) having at least a managerial position, (2) having at least 10 years of experience in the sugar sector, and (3) having sugar-related expertise recognised by others. Questionnaires were distributed to 3 (three) experts in August 2022 and to all 62 Indonesian sugar factories that have not implemented 4.0 technology, and 34 questionnaires were returned in January 2023.
In addition, the data obtained were recapitulated, processed and analysed for comparison in terms of population, methods and results obtained. The comparison of the results of the two surveys showed that cut-load-transport is a priority that requires new technology. The next analysis is of the SKKNI in the area of refined crystal sugar. The Indonesian National Competency Standard (SKKNI) is a formulation of work ability that includes aspects of knowledge, skills and/or expertise as well as work attitudes that are relevant to the performance of the duties and requirements of the specified position. The analysis of standards in the field of refined crystal sugar begins with an analysis of the mapping of units of competence in the field of refined crystal sugar. Competency mapping is a comprehensive description of each function in a business area that is used. The results of the refined crystal sugar competency mapping analysis show that the sugar production process that has been compiled starts from refining, evaporation, crystallisation, separation and completion. While cut-load-transport and milling are not included in the refined crystal sugar standard because it does not go through the process. The two parts, cut-load-transport and milling, are the differences in the production process between refined crystal sugar and white crystal sugar. These two parts will be the focus of this research to map HR competences in slash load transport and milling in the field of white crystal sugar or cane sugar.
Research on the mapping of cane sugar competence units was conducted in a cane sugar factory that has implemented 4.0 technology. The research object is located in the Blitar area, East Java, Indonesia. The research object is adapted to the research objectives, namely to map the competency units of the HR of the cane sugar agroindustry in the industrial era 4.0 with a focus on the cut-load-transport and milling sections. The research on competence mapping starts with the creation of a group or working team involved in the preparation of the mapping of competence units. The working team consisted of department heads from the cut-load-transport, milling, quality control, refining, evaporation, crystallisation, separation and white crystal sugar finishing departments. Each department was then asked to write down all the activities carried out as a starting point for the draft competency mapping. Furthermore, the draft competency mapping was verified and validated through Focus Group Discussion (FGD) activities in a sugar factory in Blitar, East Java, Indonesia.
4. Results and discussion
4.1. Assessment of cane sugar production technology
Prior to the mapping of the competence units, the researchers carried out an evaluation of the sugar production process technology in need of new technology. The evaluation was conducted using two (2) methods, namely (1) non-numerical multi-expert multi-criteria decision making and (2) numerical decision making through questionnaires. The research object was conducted for two (2) research objects, namely (1) 1 sugar factory in Eastern Indonesia and (2) 34 sugar cane factories throughout Indonesia. There are two (2) methods used, namely (1) Non-numerical Multi Expert Multi Criteria Decision Making (ME-MCDM) used for PG in Eastern Indonesia, and (2) Numerical decision making used for 34 sugar mills in Indonesia using questionnaires. ME-MCDM was chosen because it is one of the non-numerical methods and is suitable with the advantages of using multiple experts and multiple criteria to become an aggregation decision. The numerical decision-making method is used in contrast to the non-numerical decision-making method. The aim is to convince the researchers that the results of the evaluation of the cane sugar production technology from both methods have differences and similarities in different aspects.
4.1.1. Non-numerical multi-expert multi-criteria decision making
The basic logic of Yager’s MEMCDM method is that criteria with low importance will have little effect on the total value, while criteria with high importance will contribute significantly to the total value (Verna et al., Citation2023). This decision-making technique is used to determine alternative prioritisation of cane sugar production process technology that requires new technology. This method begins with the formulation of a problem that will be discussed first through literature review and discussion with experts who know the cane sugar agro-industry. Alternatives and assessment criteria are also developed together with experts. below illustrates the stages of research using the non-numerical ME-MCDM method.
Figure 2. Stages of research into recommendations for cane sugar production processes requiring new technology.

4.1.1.1. Data analysis techniques
The expert evaluation of the criteria is carried out by determining the degree of importance of the criteria for each alternative sugar production process using EquationEquation (1)(1)
(1) .
(1)
(1)
: negation of the weight value of criterion k
k: index (1, 2, 3, 4, 5)
q: number of scales
The aggregation process on the criteria uses EquationEquation (2)(2)
(2) .
(2)
(2)
: the value of the i-th alternative by the j-th expert
: the value of the i-th alternative by the j-th expert on the k-th criterion
The value weight is determined using EquationEquation (3)(3)
(3) .
: k-value weight
Int: integer
R: number of experts
The process of aggregating experts is done using EquationEquation (4)(4)
(4) .
(4)
(4)
: The process of aggregating experts is done using equations.
: j value weight
: The order of the highest value from the jth expert judgement.
4.1.1.2. Data collection and processing
Expert opinion was used to generate recommendations for prioritised production processes requiring new technology. The experts used were 3 experts from the sugar factory under study in Eastern Indonesia with pre-determined qualifications. The qualifications of the experts used are (1) at least a managerial position; (2) has 10 years of cane sugar experience; (3) has recognition related to cane sugar. The following is of alternative cane sugar production processes that are the subject of research.
Table 2. Alternative for cane sugar production process.
In addition, the rating scale for the evaluation of the seven alternatives is determined with 5 ratings, namely Perfect (P), Very High (VH), High (H), Average (A), Low (L). The negation of the rating scale is calculated using Equation (1) P to L, VH to A, H to H, S to VH and L to P. The level of importance of the criteria is an assessment given by 3 (three) experts for each criterion, then the final assessment is averaged to obtain the final results of the assessment of each criterion, which can be seen in and .
Table 3. Criteria level of importance and negation of level of importance.
Table 4. Results of the expert assessment of alternative cane sugar production processes.
Based on expert opinions, the aggregation of criteria for each alternative cane sugar production process is calculated using Equation (2) to obtain the calculation for each alternative.
1. Alternative: cut-load-transport
Aggregation of criteria
Weight value by using Equation (3)
Expert aggregation by using Equation (4)
The final score of alternative 1. cut-load-transport is H (high).
The same calculation is also carried out for alternative 2. milling up to alternative 7. completion, which is done in the same way. The final results for each alternative are shown in .
Figure 3. Diagram of final judgement by experts on production process technologies that require new technologies.

above shows that the technological priorities of the sugar factory production process requiring new technology with category H (high) there are 4 namely (1) cut-load-transport, (2) milling, (3) refining and (4) evaporation; category A (equal) there are 3 namely (1) crystallisation, (2) separation, (3) finishing. The results of the expert evaluation of the alternative sugar production processes resulted in 2 out of 5 categories. For category H (high), 4 alternatives are obtained, but among the 4 alternatives, it is not clear which order has priority, which means that the 4 alternatives have to be run simultaneously. Similarly, for category A (equal), 3 alternatives were obtained, but among the 3 alternatives it is not clear which order has priority, which means that they are equal, although there is actually a slight difference. However, the results of the recommendations obtained are still up to the sugar factory to decide, as the limitations of the sugar factory are not included in this calculation.
4.1.2. Numerical decision making
Data collection in research must be done scientifically and systematically. This study conducted a survey by distributing questionnaires or surveys as research instruments, where the questionnaire is an effective and efficient forum for collecting data to be measured numerically.
4.1.2.1. Data collection and processing
Expert opinion was used to develop recommendations for priority production processes requiring new technology. Data was collected using a questionnaire distributed to 62 sugar factories in Indonesia, and 34 questionnaires were returned to be used as research data. The qualifications of the experts who completed the questionnaire were determined by the following conditions: (1) at least a managerial position; (2) 10 years of cane sugar experience; (3) recognition related to cane sugar. Microsoft Excel was used for data analysis. The following results of data collection and processing from 34 cane sugar factories can be seen in .
Figure 4. Technological prioritisation of cane sugar production in Indonesia, which requires new technologies.

From the above , it can be seen that the priority of cane sugar production technology requiring new technology is as follows, in order: (1) cut-load-transport by 62%, (2) crystallisation by 47%, (3) refining by 41%, (4) milling by 35%, (5) refining by 29%, (6) completion by 29% and (7) separation by 18%. Based on the data processing and analysis visualised in , the state of sugar mills in Indonesia shows that cut-load-transport technology is the priority requiring new technology. This is followed by crystallisation, evaporation, milling, finishing, evaporation and separation. However, the results of the recommendations obtained are still up to the sugar factories to decide, as the limitations of the sugar factories are not taken into account in this calculation.
Furthermore, data processing and analysis was carried out between the two research objects, the priority of sugar production processes to be replaced by new technology is the same, namely cut-load-transport. The results of the comparison of the cane sugar production process technology to be replaced by new technology of the two research objects together with the Indonesian national competency standards for refined crystal sugar in the context of competence mapping in the cane sugar agro-industry can be seen in .
Table 5. Comparison of sugar production process evaluation results with 2 (two) methods.
shows a comparison of the results of the evaluation of the sugar production process using 2 (two) methods (non-numerical decision making and numerical decision making) with 2 (two) research objects, namely 1 (one) sugar mills in Eastern Indonesia and 34 (thirty-seven) sugar mills throughout Indonesia. It was found that the same results were obtained with both methods with both research objects, that is, that the slab transport section is a priority that requires new technology. This result was also confirmed by experts, and the cut-load-transport section does require new technology compared to other parts of the sugar production process. This is because the status of sugarcane land in Indonesia consists of 3 ownership statuses, namely (1) large state plantations, (2) large private plantations and (3) smallholder plantations. In 2021, Indonesia’s sugarcane area will be 449 hectares (BPS, Citation2022) with the largest composition of smallholder plantations (59%), large private plantations (30%) and large state plantations (11%). As a result, the majority of cane harvesting activities is carried out by farmers using manual rather than mechanised equipment.
In terms of loading activities, the sugar cane production line during harvesting, where the sugar cane fields are generally in the middle of the field and have to be moved to the lane to be loaded onto the truck, is also done manually and few use technological aids such as three-wheeled conveyors or lorries to transport sugar cane by rail. For transport activities, the problems are more related to the road infrastructure, which needs to be improved and repaired, which is considered to increase sugar production. According to Freitas et al. (Citation2019). In his research on cut-load-transport, he suggested a 40% reduction in the time spent waiting for sugarcane to be transported. The cause of the long waiting time for sugarcane to be milled at the mill terrace is the variability of the land, which results in inefficient use of felling and transport labour. There are 5 proposed solutions, namely (1) integration of computer system and traffic control system; (2) integration of harvester on-board computer with transporter; (3) application of truck filling volume sensor; (4) command-based management; (5) assignment of one person with different tasks; (5) assignment of one person with different tasks.
4.1.3. Mapping competency units in sugar cane factories with 4.0 technology
The purpose of this section is to design a mapping of HR competency units for the cane sugar agro-industry in the industrial era 4.0. The considerations used before mapping the HR competence units in the cane sugar agroindustry are (1) the results of the situation analysis from the HR aspect in the form of the need for competence infrastructure in the cane sugar agroindustry, (2) the results of the evaluation from the technological aspect that cut-load-transport as a priority technology that requires new technology, and (3) the analysis and adoption of Indonesian national competency standards in the field of refined crystal sugar that the milling part is a part of the production process that refined crystal sugar does not have due to differences in raw materials in the production of sugar.
From the results of the evaluation of cane sugar production technology, it is found that cut-load-transport is a priority technology that requires new technology. This reason encourages the author to conduct a mapping of HR competency units in the cane sugar agro-industry with a focus on the cut-load-transport and milling sections. While the part outside the cut-load-transport and milling adopts Indonesian national competency standards in the field of refined crystal sugar which includes quality control and production (purification, evaporation, crystallisation, separation, and completion). The research was conducted in one of the sugar factories in Blitar which is considered to have implemented 4.0 technology. Some considerations why this sugar factory is the subject of research because this factory is one of the new factories that use modern machinery such as the use of (1) tandem mill sets; (2) process stations; (3) robotic bagging; (4) boilers and turbine generators; (5) quality control laboratories using digital equipment.
The stages of competency unit mapping are (1) perception equalisation by providing a comprehensive understanding of the competency mapping programme, (2) conducting competency mapping by conducting sector/business group analysis and productive function analysis (main objectives, key functions, main functions and basic functions), (3) draft competency unit mapping of cane sugar agroindustry HR work competencies, (4) verification of draft competency unit mapping in FGDs, and (5) draft competency unit mapping that has been verified. Competency mapping will be carried out through the formation of a working group whose role and function will be to carry out the competency mapping activities in accordance with the terms of reference. The members of the working group in this study are the factory manager and his subordinate managers in a WhatsApp group to facilitate coordination and communication. The formulation of the unit competency mapping design was carried out using field research, adaptation and adoption methods. The role of the researcher here is from a methodological aspect by having a certification of training in the preparation of work standards, while the team members from the sugar factory from the content aspect of unit competency mapping.
Supporting data needed such as organisational structure, number of employees and company profile, distribution of questionnaires in the form of google forms to find out the duties and functions of each position in cut-load-transport and milling, and the need to form a WhatsApp group for coordination. The format of the competency unit mapping is based on the format of the Indonesian Ministerial Regulation No. 3 of 2016 on Procedures for Determining Indonesian National Work Competency Standards. Verification of the draft competency unit mapping was conducted through focus group discussions (FGD) at the sugar factory. The following results of the verified competency unit mapping are shown in .
Table 6. Competency unit mapping.
The positions in the On Farm Department of the cut-load-transport section are Zone Operator, Logistics Operator, Harvest Operator, Zone Shift Head, Logistics Shift Head, Section Head Zone, Plant Zone Manager, and Logistics Manager. Positions in the quality control department are Process Control Analyst, Product Analyst, Water Treatment Analyst, Incoming Material Analyst, Waste Analyst, Microbiological Analyst, Regular Foreman, Shift Foreman, Shift Head, Super Intendent, Assistant Manager, Manager. Positions contained in the production department of the milling section are DCS Mill Operator, Field Mill Operator, Lubricant Operator, Tipper Operator, Scrapper Operator, Cane Preparation Operator, Shift Head, Super Intendent, Assistant Manager, Manager. Positions contained in the production department of the process section are Afination Operator, Carbonation Operator, Filtration Operator, IER Operator, Evaporator Operator, Vacuum Pan Operator, Centrifugal Operator, Drying and Cooling Operator, and Packing Operator, Afination Foreman, Carbonator-Filtration-IER Foreman, Evaporator & Vacuum Pan Foreman, Centrifugal-Drying-Cooling Foreman, Packing Foreman, Decolorisation Supervisor, Centrifugal Supervisor, Boiling/Vacuum Pan/Crystallisation Supervisor, Bagging Supervisor.
Based on above, the role of the On-Farm cut-load-transport Department is to ensure that manual cut-load-transport activities comply with standards and are carried out effectively and efficiently. The Quality Control Department inspects raw materials, process materials, process materials and finished products. The Mill Production Department operates the mill unit to milk the cane as much as possible to separate it from the pulp and to minimise the sugar content in the pulp. The process section of the production department effectively and efficiently manages all production management in the refining, evaporation, crystallisation, separation and completion sections. The unit of competence mapping contains basic functions, hereafter referred to as units of competence. The unit of competence is the output of the position in the department.
illustrates the number of units of competency in the cut-load-transport and milling section. The number of units of competence in the cut-load-transport and milling section is 57 titles of units of competence that have been verified and validated by the internal sugar cane factory which is the object of research. This draft list of competency units can also be used by internal sugar factories that are the object of research as well as an initial design for the white crystal sugar industry to continue as a unit of competence into a national standard where several sugar cane factories are needed to make the mapping of competency units into national standards for the white crystal sugar sector. The draft competency map for the white crystal sugar field is a comprehensive description of the competencies of each function in a business field that is used as a reference in compiling competency standards.
Table 7. List of cut-load-transport and milling units of competence.
This competency mapping can be used by organisations as an employee assessment tool, allowing them to maximise the potential of employees by assigning them to tasks with the highest probability of success. In addition, the information can be used to focus talent on relevant tasks and to draw on employees’ expertise. As a result, organisations are likely to see results such as increased productivity and improved profits. Companies or sugar factories can also use competency mapping to write thorough job descriptions and attract talented people with the right skills. In addition, competency mapping can boost morale by outlining expectations and ensuring that employees understand how to achieve a good result. Employees may find it easier to gain the support of their employer if both parties are aware of the expectations. In addition, the transparency created by competency mapping facilitates career development. Building industry-relevant skills will encourage innovation and prepare employees for more advanced positions within the organisation.
5. Conclusions and suggestions
5.1. Conclusions
The results of the evaluation of the sugar production process technology using the non-numerical multi-expert multi-criteria decision-making method on two different objects have the same result that the cut-load-transport section is a priority that requires new technology. This is because Indonesia’s sugarcane area is 449 hectares in 2022, with the largest composition of 59% smallholder plantations, 30% large private plantations and 11% large state plantations. As a result, the majority of cane harvesting activities is carried out by farmers using manual equipment rather than mechanisation.
Various considerations in the design of competency unit mapping are by evaluating sugar production process technology and identifying related Indonesian national competency standards. The design of this competency unit map can also be used by internal sugar factories that are the subject of research as well as an initial design for the white crystal sugar industry to continue as a competency unit in a national standard where several sugar cane factories are needed to make the mapping of competency units in national standards for the field of white crystal sugar.
5.2. Suggestions
The role of stakeholders in the availability of competency infrastructure, one of which is national work competency standards, is needed and can be used as a government programme to facilitate and other parties support by participating according to their respective roles. Further research of this study is to look for the effect of the relationship between competency infrastructure and cane sugar productivity.
Author’s contributions
Conceived and designed the experiments: Alex Denni, Illah Sailah & M. Marimin; performed the experiments: Illah Sailah & M. Mursiti; analyed and interpreted the data: Illah Sailah, M. Marimin, M. Romli & M. Mursiti; contribute reagents, materials, analysis tools or data: M. Marimin, Alex Denni & M. Mursiti; wrote the paper: Illah Sailah, M. Marimin, M. Romli & M. Mursiti.
Acknowledgements
We would like to thank the supervisors inside and outside IPB who provided constructive input to enrich this paper.
Disclosure statement
The author(s) declared no potential conflicts of interest with respect to the research, authorship, and/or publication of this article.
Data availability
The data that support the findings of this study are available on request from the corresponding author upon reasonable request.
Additional information
Funding
Notes on contributors
M. Mursiti
M. Mursiti, graduated Bachelor of Industrial Engineering at STMI Jakarta (2000), Master of Industrial Engineering & Management at ITB Bandung (2008), and the final process of completing Ph.D. at IPB University Bogor (2024). Mursiti has been working at the Industrial Human Resources Development Agency, Indonesian Ministry of Industry since 2001.
Illah Sailah
Illah Sailah, graduated her Ph.D. from the University of Queensland in 1994. Prof Sailah has been teaching a course on human capital development since 2016, and her research interests are agro-industrial technology development and human resource development. She is a member of the Indonesian Institute of Engineers.
M. Marimin
M. Marimin, graduated B.Sc.H, M.Sc. and Ph.D. degrees from IPB University, Western University and Osaka University (1984, 1990, 1997) respectively. Prof. Marimin’s research interests are intelligent decision support and sustainable supply chain management. He is a member of Indonesian Society of Engineers and Institute of Electrical and Electronic Engineer (IEEE).
Muhammad Romli
Muhammad Romli, graduated his bachelor’s degree from Bogor Agricultural University, Indonesia (1984), and his master’s degree in biotechnology and Ph.D. in chemical engineering from the University of Queensland, Australia, (1990 and 1993), respectively. He is a professor at IPB University. His research interests include life cycle assessment of agro-industrial products.
Alex Denni
Alex Denni, is a dynamic Principal Learning Partner. Armed with a PhD in Human Capital from IPB (2011) and a Master’s from Atma Jaya University (1997), he has decades of experience. As Chairman of CEGOS Indonesia, he’s committed to empowering minds and shaping futures for unparalleled success in today’s dynamic world.
References
- Alam, M. F. B., Tushar, S. R., Zaman, S. M., Gonzalez, E. D. R. S., Bari, A. B. M. M., & Karmaker, C. L. (2023). Analysis of the drivers of Agriculture 4.0 implementation in the emerging economies: Implications towards sustainability and food security. Green Technologies and Sustainability, 1(2), 1. https://doi.org/10.1016/j.grets.2023.100021
- Albiero, D., Paulo, R. L. D., Junior, J. C. F., Santos, J. D. S. G., & Melo, R. P. (2020). Agriculture 4.0: A terminological introduction. Revista Ciência Agronômica, 51(5), 1–24. https://doi.org/10.5935/1806-6690.20200083
- Alcácer, V., & Cruz-Machado, V. (2019). Scanning the Industry 4.0: A literature review on technologies for manufacturing systems. Engineering Science and Technology, an International Journal, 22(3), 899–919. https://doi.org/10.1016/j.jestch.2019.01.006
- Aldhyani, T. H. H., & Alkahtani, H. (2023). Cyber security for detecting distributed denial of service attacks in Agriculture 4.0: Deep learning model. Mathematics, 11(1), 233. https://doi.org/10.3390/math11010233
- Alhloul, A., & Kiss, E. (2022). Industry 4.0 as a challenge for the skills and competencies of the labor force: A Bibliometric review and a survey. Science, 4(3), 34. https://doi.org/10.3390/sci4030034
- Araújo, S. O., Peres, R. S., Barata, J., Lidon, F., & Ramalho, J. C. (2021). Characterising the Agriculture 4.0 landscape—Emerging trends, challenges and opportunities. Agronomy, 11(4), 667. https://doi.org/10.3390/agronomy11040667
- Asrol, M., Marimin, M., Machfud, M., Yani, M., & Taira, E. (2021). Risk management for improving supply chain performance of sugarcane agroindustry. Industrial Engineering & Management Systems, 20(1), 9–26. https://doi.org/10.7232/iems.2021.20.1.9
- Bappenas. (2020). Annex I to Presidential Decree No 18 of 2020 on the National Medium-Term Development Plan 2020-2024 (p. 20).
- Bello, S. A., Oyedele, L. O., Akinade, O. O., Bilal, M., Davila Delgado, J. M., Akanbi, L. A., Ajayi, A. O., & Owolabi, H. A. (2021). Cloud computing in construction industry: Use cases, benefits and challenges. Automation in Construction, 122, 103441. https://doi.org/10.1016/j.autcon.2020.103441
- Blayone, T. J. B., & VanOostveen, R. (2021). Prepared for work in Industry 4.0? Modelling the target activity system and five dimensions of worker readiness. International Journal of Computer Integrated Manufacturing, 34(1), 1–19. https://doi.org/10.1080/0951192X.2020.1836677
- BPS. (2022). Indonesian sugar cane statistics. BPS RI/BOS-Statistics Indonesia.
- Chowdhury, M. T., Sarkar, A., Paul, S. K., & Moktadir, M. A. (2020). A case study on strategies to deal with the impacts of COVID-19 pandemic in the food and beverage industry. Operations Management Research, 15(1–2), 166–178. https://doi.org/10.1007/s12063-020-00166-9
- Chryssolouris, G., Mavrikios, D., & Mourtzis, D. (2013). Manufacturing systems: Skills & competencies for the future. Procedia CIRP, 7(May 2014), 17–24. https://doi.org/10.1016/j.procir.2013.05.004
- Coombe, L., Severinsen, C. A., & Robinson, P. (2022). Mapping competency frameworks: Implications for public health curricula design. Australian and New Zealand Journal of Public Health, 46(5), 564–571. https://doi.org/10.1111/1753-6405.13253
- Ehlers, M. H., Finger, R., El Benni, N., Gocht, A., Sørensen, C. A. G., Gusset, M., Pfeifer, C., Poppe, K., Regan, Á., Rose, D. C., Wolfert, S., & Huber, R. (2022). Scenarios for European agricultural policymaking in the era of digitalisation. Agricultural Systems, 196, 103318. https://doi.org/10.1016/j.agsy.2021.103318
- Fachrunnisa, O., & Hussain, F. K. (2020). Blockchain-based human resource management practices for mitigating skills and competencies gap in workforce. International Journal of Engineering Business Management, 12, 184797902096640. https://doi.org/10.1177/1847979020966400
- Fatimah, Y. A., Kannan, D., Govindan, K., & Hasibuan, Z. A. (2023). Circular economy e-business model portfolio development for e-business applications: Impacts on ESG and sustainability performance. Journal of Cleaner Production, 415(April), 137528. https://doi.org/10.1016/j.jclepro.2023.137528
- Flores, E., Xu, X., & Lu, Y. (2020). Human Capital 4.0: A workforce competence typology for Industry 4.0. Journal of Manufacturing Technology Management, 31(4), 687–703. https://doi.org/10.1108/JMTM-08-2019-0309
- Fonseca, L. M. (2018). Industry 4.0 and the digital society: concepts, dimensions and envisioned benefits. Proceedings of the International Conference on Business Excellence, 12(1), 386–397. https://doi.org/10.2478/picbe-2018-0034
- Freitas, L. D., De Melo Duarte Borges, T., Martins, A. S., Panaino, R. C., Neto, J. B. N., & Filho, M. G. (2019). Analysis and proposal of reduction of lead time in the process of cutting, loading and transportation in a sugar cane factory: A study case. Gestão & Produção, 26(3). https://doi.org/10.1590/0104-530x3446-19
- Fuertes, G., Zamorano, J., Alfaro, M., Vargas, M., Sabattin, J., Duran, C., Ternero, R., & Rivera, R. (2022). Opportunities of the technological trends linked to Industry 4.0 for achieve sustainable manufacturing objectives. Sustainability (Switzerland), 14(18), 11118. https://doi.org/10.3390/su141811118
- Haeffner, M., & Panuwatwanich, K. (2018). Perceived impacts of industry 4.0 on manufacturing industry and its workforce: Case of Germany. Lecture Notes in Mechanical Engineering, 0(9783319741222), 199–208. https://doi.org/10.1007/978-3-319-74123-9_21
- Hahn, K. (2020). Opportunities for socially responsible Industry 4.0. IEEE Engineering Management Review, 48(1), 63–70. https://doi.org/10.1109/EMR.2020.2969407
- Harry, J. (2020). European journal of human resource management studies stress management and employee performance. European Journal of Human Resource Management Studies, 0(0), 28–39. https://doi.org/10.5281/zenodo.3375458
- Hasbullah, H., Bareduan, S. A., & Hasibuan, S. (2021). Developing I4.0 Readiness Index for factory operation in Indonesia to enhance INDI 4.0. International Journal on Advanced Science, Engineering and Information Technology, 11(4), 1668–1677. https://doi.org/10.18517/ijaseit.11.4.14280
- Hecklau, F., Galeitzke, M., Flachs, S., & Kohl, H. (2016). Holistic approach for human resource management in Industry 4.0. Procedia CIRP, 54, 1–6. https://doi.org/10.1016/j.procir.2016.05.102
- Heryanto, M. A., Suryatmana, E. R., Sosial, D., Pertanian, E., Pertanian, F., & Padjadjaran, U. (2020). The dynamics of the Indonesian sugar agro-industry: Introduction The sugar industry has played an important and strategic role in the Indonesian economy since the Dutch colonial era until now. (Arifin, 2008 ; Magfiroh et al., 2017). 5, 194–210.
- Holmes, D. W., Sheehan, M., Birks, M., & Smithson, J. (2018). Development of a competency mapping tool for undergraduate professional degree programmes, using mechanical engineering as a case study. European Journal of Engineering Education, 43(1), 126–143. https://doi.org/10.1080/03043797.2017.1324404
- Kadir, B. A., & Broberg, O. (2021). Human-centered design of work systems in the transition to industry 4.0. Applied Ergonomics, 92(November 2020), 103334. https://doi.org/10.1016/j.apergo.2020.103334
- Kaur, J., & Kumar, V. (2013). Vol. 1 No. 1 January 2013 Competency mapping: A Gap analysis. International Journal of Education and Research, 1(1), 1–9.
- Kaur, J., Madaan, G., Qazi, S., & Bhalla, P. (2023). An explorative factor analysis of competency mapping for IT professionals. Administrative Sciences, 13(4), 98. https://doi.org/10.3390/admsci13040098
- Kautz, T., Heckman, J. J., Diris, R., ter Weel, B., & Borghans, L. (2014). Fostering and measuring skills: Improving cognitive and non-cognitive skills to promote lifetime success. SSRN Electronic Journal, 110. https://doi.org/10.2139/ssrn.2543890
- Ketenagakerjaan, K. (2020). Keputusan Menteri Ketenagakerjaan RI No 178 Tahun 2020 Tentang Penetapan SKKNI Kategori Industri Pengolahan Golongan Pokok Industri Makanan Bidang Industri Gula Kristal Rafinasi.
- Kipper, L. M., Iepsen, S., Dal Forno, A. J., Frozza, R., Furstenau, L., Agnes, J., & Cossul, D. (2021). Scientific mapping to identify competencies required by Industry 4.0. Technology in Society, 64(October 2020), 101454. https://doi.org/10.1016/j.techsoc.2020.101454
- Lambert, M., & Bouchamma, Y. (2019). Leadership requirements for school principals: Similarities and differences between four competency standards. Canadian Journal of Educational Administration and Policy, 188, 53–68. https://cdm.ucalgary.ca/index.php/cjeap/article/view/43249
- Liu, L., Song, W., & Liu, Y. (2023). Leveraging digital capabilities toward a circular economy: Reinforcing sustainable supply chain management with Industry 4.0 technologies. Computers & Industrial Engineering, 178(April 2022), 109113. https://doi.org/10.1016/j.cie.2023.109113
- Luthra, S., Kumar, A., Zavadskas, E. K., Mangla, S. K., & Garza-Reyes, J. A. (2020). Industry 4.0 as an enabler of sustainability diffusion in supply chain: an analysis of influential strength of drivers in an emerging economy. International Journal of Production Research, 58(5), 1505–1521. https://doi.org/10.1080/00207543.2019.1660828
- Mell, P., & Grance, T. (2012). The NIST definition of cloud computing: Recommendations of the National Institute of Standards and Technology. Public Cloud Computing: Security and Privacy Guidelines, 97–101.
- Meyer, C., Kreft, H., Guralnick, R., & Jetz, W. (2015). Global priorities for an effective information basis of biodiversity distributions. Nature Communications, 6(1), 8221. https://doi.org/10.1038/ncomms9221
- Miao, C., Humphrey, R. H., & Qian, S. (2017). A meta-analysis of emotional intelligence and work attitudes. Journal of Occupational and Organizational Psychology, 90(2), 177–202. https://doi.org/10.1111/joop.12167
- Ministry of Labour. (2020). Decree of the Minister of Manpower of the Republic of Indonesia No. 178 of 2020 on the determination of the SKKNI category of processing industry in the food industry main group in the field of refined crystal sugar industry.
- Mursiti, S., Marimin, I., Romli, M., & Denni, A. (2021). Competitive and sustainable human resources development strategy in sugarcane agroindustry: Literature review and future research agenda. Jurnal Teknologi Industri Pertanian, 31(2), 129–142. https://doi.org/10.24961/j.tek.ind.pert.2021.31.2.129
- Oruma, S. O., Misra, S., & Fernandez-Sanz, L. (2021). Agriculture 4.0: An implementation framework for food security attainment in Nigeria’s Post-Covid-19 Era. IEEE Access, 9, 83592–83627. https://doi.org/10.1109/ACCESS.2021.3086453
- Perera, S., Pearson, J., Ekundayo, D., & Zhou, L. (2013). Professional, academic and industrial development needs: A competency mapping and expert opinion review. International Journal of Strategic Property Management, 17(2), 143–160. https://doi.org/10.3846/1648715X.2013.806966
- Pérez-Lara, M., Saucedo-Martínez, J. A., Salais-Fierro, T. E., Marmolejo-Saucedo, J. A., & Vasant, P. (2019). Vertical and horizontal integration systems in Industry 4.0. EAI/Springer Innovations in Communication and Computing, 2, 99–109. https://doi.org/10.1007/978-3-030-03898-4_11
- Raj, M., Gupta, S., Chamola, V., Elhence, A., Garg, T., Atiquzzaman, M., & Niyato, D. (2021). A survey on the role of Internet of Things for adopting and promoting Agriculture 4.0. Journal of Network and Computer Applications, 187(August), 103107. https://doi.org/10.1016/j.jnca.2021.103107
- Rangnekar, S. R. S. (2020). Understanding relationship between talent acquisition, competency mapping and performance management and appraisal. International Journal of Management, 11(5), 1735–1749.
- Rezvani, A., Chang, A., Wiewiora, A., Ashkanasy, N. M., Jordan, P. J., & Zolin, R. (2016). Manager emotional intelligence and project success: The mediating role of job satisfaction and trust. International Journal of Project Management, 34(7), 1112–1122. https://doi.org/10.1016/j.ijproman.2016.05.012
- Robles, M. M. (2012). Executive perceptions of the Top 10 soft skills needed in today’s workplace. Business Communication Quarterly, 75(4), 453–465. https://doi.org/10.1177/1080569912460400
- Rout, S. S., Misra, B. B., & Samanta, S. (2018). Competency mapping with Sugeno fuzzy inference system for variable pay determination: A case study. Ain Shams Engineering Journal, 9(4), 2215–2226. https://doi.org/10.1016/j.asej.2017.03.007
- Silva, F. T. D., Baierle, I. C., Correa, R. G. D. F., Sellitto, M. A., Peres, F. A. P., & Kipper, L. M. (2023). Open innovation in agribusiness: Barriers and challenges in the transition to Agriculture 4.0. Sustainability (Switzerland), 15(11), 8562. https://doi.org/10.3390/su15118562
- Stachová, K., Stacho, Z., Cagáňová, D., & Stareček, A. (2020). Use of digital technologies for intensifying knowledge sharing. Applied Sciences (Switzerland), 10(12), 4281. https://doi.org/10.3390/app10124281
- Takey, S. M., & Carvalho, M. M. D. (2015). Competency mapping in project management: An action research study in an engineering company. International Journal of Project Management, 33(4), 784–796. https://doi.org/10.1016/j.ijproman.2014.10.013
- Verna, E., Genta, G., & Galetto, M. (2023). A new approach for evaluating experienced assembly complexity based on multi expert-multi criteria decision making method. Research in Engineering Design, 34(3), 301–325. https://doi.org/10.1007/s00163-023-00409-3
- Vuorikari, R., Punie, Y., Carretero, S., & Van Den Brande, L. (2016). DigComp 2.0: The digital competence framework for citizens. JRC-IPTS, (June). https://doi.org/10.2791/11517
- Zhai, Z., Martínez, J. F., Beltran, V., & Martínez, N. L. (2020). Decision support systems for agriculture 4.0: Survey and challenges. Computers and Electronics in Agriculture, 170(August 2019), 105256. https://doi.org/10.1016/j.compag.2020.105256
- Zhang, C., & Lu, Y. (2021). Study on artificial intelligence: The state of the art and future prospects. Journal of Industrial Information Integration, 23(May), 100224. https://doi.org/10.1016/j.jii.2021.100224