Abstract
This research investigates the ethical and privacy issues arising from using AI andML in neuromarketing, framed by rule utilitarianism. It assesses the impact of these technologies on consumerprivacy and human rights through a combination of literature review, bibliometric analysis, and empirical data fromsurveys and interviews with experts in the US and Spain. The study reveals the tensions between the efficacy ofneuromarketing techniques and the imperative to protect consumer privacy, particularly in light of the GDPR’sinfluence on global practices. It emphasizes the need for internationally consistent ethical standards and consumerdata regulations, drawing from the comparative analysis of policies in the US and EU. The outcomes include policyrecommendations to minimize ethical risks and promote the responsible progression of neuromarketing. Theserecommendations guide companies and managers toward ethical transparency and accountability. Additionally, theresearch offers a policy framework for crafting ethical neuromarketing practices that reconcile technological progresswith consumer well-being, thereby contributing to broader discussions on embedding ethics within technological innovation.
IMPACT STATEMENT
Advances in neurotechnology are revolutionizing marketing strategies, allowing businesses to predict and influence consumer behavior with remarkable accuracy. This paper investigates the ethical challenges and privacy concerns associated with using artificial intelligence (AI) and machine learning (ML) in neuromarketing. Through a comprehensive literature review and expert interviews, it explores how these technologies intersect with consumer rights and offers policy recommendations to address ethical dilemmas. This research examines the balance between marketing innovation and the safeguarding of individual privacy, contributing to the ongoing debate on the ethical use of AI in understanding consumer preferences.
1. Introduction
Neuromarketing is a quickly evolving research sector. Marketers now recognize that brain and biometric research can lead to a more in-depth understanding of consumer behavior and preferences (Fisher et al., Citation2010; de Oliveira & Giraldi, Citation2017; Srivastava et al., Citation2020; Pluta-Olearnik & Szulga, Citation2022; Sheth, Citation2021). While the sector is fast growing internationally, the US is one of the most significant regions in the global neuromarketing market, owing to end-user industries’ growing number of US-based market vendors and high investment in digital marketing (GMarket Data Forecast, Citation2022; Sengur & Goncalves, Citation2023). In this regard, neuromarketing strategies and applications are applied in several fields, such as market and marketing research, consumer behavior and preferences, and criminal justice.
Although neuromarketing research has developed, the current studies need more comprehensive insights into neuromarketing and marketing mix. Scholars (Alsharif et al., Citation2022; Goncalves et al., Citation2022; Alsharif et al., Citation2023a) have contributed significantly to the field, providing a comprehensive overview of neuromarketing, classification of neuroimaging and physiological tools that are currently used in the marketing mix, and highlighted neural responses of consumer’s behavior, such as emotions, attention, motivation, reward processing, and perception, to be considered in the marketing mix (Kaul, Citation2022). Their findings confirm the reliability of several tools in studying the marketing mix, such as advertising, brand, price, and product, including but not limited to electroencephalography, eye tracking, galvanic skin reaction (GSR), face coding, and many others. It is known today that the frontal and temporal gyri are correlated with pleasure/displeasure and high/low arousal. At the same time, the occipital lobe is linked to attention processes, and the hippocampus relates to long and short-term memory. Such findings provide valuable insights into the neural responses in marketing mix research.
This paper defines neuromarketing as the scientific study of the nervous system applied to marketing. It measures physiological and neural signals to gain insight into consumers’ behavior, motivations, preferences, and decisions, which can inform innovative advertising, product development, pricing, and other marketing areas. It utilizes brain imaging, scanning, or other brain measurement technologies to collect consumers’ reactions to marketing stimuli and evade the challenges of depending on consumers’ self-reports (Harrell, Citation2019; Brenninkmeijer et al., Citation2020; Parrish et al., Citation2020; Frederick, Citation2022; Halkiopoulos et al., Citation2022). Despite the large array of marketing research methods, through the use of neuroscience lab techniques, scientists, academics, and now marketers have realized that the answers given by the consumers are likely biased or skewed, consciously or unconsciously, due to the influence of stereotypes, cognitive biases, emotions, social and moral norms, and many other factors (BrandWatch, Citation2019; Casado-Aranda & Sanchez-Fernandez, Citation2022; Strieder, Citation2022).
Artificial intelligence (AI) has been used since the first half of the twentieth century. Many scholars have delineated technologies emulating the human brain to perform complex data analysis and decision-making tasks (Benbya et al., Citation2021; Berente et al., Citation2021; Nishant et al., Citation2023; Sengur & Goncalves, Citation2023). Over the decades, AI has been boosted and adjusted to perform more specific tasks like flying airplanes, driving cars, and analyzing complex data (Bhalla et al., Citation2020). Machine learning (ML) is an artificial intelligence subset related to statistics and data analysis (Ongsulee, Citation2017). ML algorithms are capable of learning patterns from training data sets, which can then be applied to various settings and targets such as populations, internet search engines, email filters, web-based services personalization and recommendations, banking and financial applications, and an ever-growing large array of applications running on digital devices. These algorithms are disseminated widely in the advertisement and marketing sectors, assisting throughout the consumer life cycle, learning continuously, much like humans, absorbing more knowledge, and becoming more intelligent. The more unstructured data the AI/ML algorithms process, the more precise the output they provide to users (Kietzmann et al., Citation2018; Neal et al., Citation2023).
Greater availability of neuromarketing technologies and often embedded AI software enables marketers to reduce the time spent analyzing consumer behavior and preferences, segmentation, and organizing targeting campaigns, thus increasing the marketing department’s productivity ratio. The general belief is that neuromarketing technologies and AI algorithms improve marketing and sales operations, consumer service, and personalization (Galli, Citation2022). AI and its elements are widely utilized throughout neuromarketing development and applications, from segmentation through direct marketing and targeting campaigns to relying on AI shopping assistants and chatbots. However, ML algorithms are mainly applied in the phases of segmentation and targeting due to their predictive and optimization abilities (Ma & Sun, Citation2020; Chang & Fan, Citation2023). Despite comprehensive studies on undermining social values and ethical considerations posed by chatbots and interactive AI/ML algorithms, they are occasionally underrated since they remain pretty hidden from the broader audience, users, and consumers.
Consumer emotion recognition-based experiments observe a tendency to investigate frontal and prefrontal cortex signals (Mouammine & Azdimousa, Citation2019). Many researchers found the use of electroencephalogram (EEG) favorable over functional magnetic resonance imaging (fMRI) in video advertisement-based neuromarketing experiments (Khurana et al., Citation2021; Yarosh et al., Citation2021; Alsharif et al., Citation2022; Alsharif et al., Citation2023b; Goncalves et al., Citation2023) due to its low cost and high time resolution advantages. Physiological response measuring techniques such as eye-tracking (ET), galvanic skin reaction (GSR) recording, heart rate (HR) monitoring, and facial mapping have also been found in these empirical studies exclusively or parallel with brain recordings (Kaheh et al., Citation2021; Şik & Soba, Citation2021; Goncalves et al., Citation2022). In consumer response prediction and classification, Artificial Neural Networks (ANN), Support Vector Machine (SVM), Linear Discriminant Analysis (LDA), Naïve Bayes, k-Nearest Neighbor (KNN), and Hidden Markov Model (HMM) have been performed with the highest average accuracy among other ML algorithms (Rawnaque et al., Citation2020; Raj et al., Citation2022; Kabanda, Citation2023). Corporations like Coca-Cola, Nestle, Proctor and Gamble, General Motors, Campbell, Frito-Lay, PayPal, Walmart, Home Depot, IKEA, and Target, to name a few, have all been noted as organizations that are actively engaging in neuromarketing research and AI/ML implantations. Such technologies and algorithms raise ethical concerns despite only a few public case studies exist to inform the public about them (Gurgu et al., Citation2020; Goncalves et al., Citation2022).
Despite AI/ML’s ubiquity across various technologies, industries, and healthcare, no comprehensive federal legislation regulates it in the US (Forrest, Citation2021). The executive branch continues to adopt directives and rulemaking that will impact the use of AI/ML. The US Congress has enacted and is considering several legislations regulating certain aspects of AI. In February 2020, the Electronic Privacy Information Center (EPIC) petitioned the Federal Trade Commission (FTC) to conduct rulemaking concerning AI in commerce to define and prevent consumer harm from AI/ML products (Zhu & Lehot, Citation2022). Much of the governing legal framework is through cross-applicating rules and regulations governing traditional disciplines such as product liability, data privacy, intellectual property, discrimination, and workplace rights. Self-regulation and standards groups also contribute to the governing framework. In the context of recent advances in AI and the launch of policy documents and ethics guidelines worldwide, regulations must be developed in the US (Zhu & Lehot, Citation2022).
The European Union (EU), since 2017, has been working on its approach to AI (Ulnicane, Citation2022), developing key policy documents and strategically positioning it vis-à-vis other global players. The EU General Data Protection Regulation (GDPR) will affect AI companies that meet the established criteria for the EU. Article 22 of the GDPR states that a ‘data subject shall have the right not to be subject to a decision based solely on automated processing, including profiling, which produces legal effects concerning him or her or similarly significantly affects him or her’ unless certain conditions are present. One permitted setting is based on express and informed consent by the data subject. GDPR’s Article 22 will likely affect how companies approach AI transparency and bias (Zhu & Lehot, Citation2022).
Following Cambridge Analytica’s Facebook crisis in 2018, emphasis has shifted to the ethics of algorithms (Confessore, Citation2018), as the adoption of AI technologies for neuromarketing is rising continuously in all industries (McKinsey, Citation2021). Thus, if marketing professionals do not consider the ethical risks of algorithms in consumer behavior, preferences, segmentation, and targeting (Zulaikha et al., Citation2020), they may damage the brand’s reputation or lead to a substantial monetary loss for its implementations. An example is the lawsuit against Alphabet Inc., amounting to 5 billion US dollars. Google is blamed for consumer profiling even in private mode (Reuters, 2020) and then again in incognito (Southern, Citation2021). Hence, since neither neuromarketing nor AI has matured in research and theoretical frameworks, both enjoy disbelief and unethical behavior from certain groups (Rakić, Citation2021; Petrillo et al., Citation2022). As they include algorithms capable of collecting and interpreting data to mimic cognitive decisions and predict future behavior, it is becoming imperative to determine which ethical aspects should be considered and regulated when combining neurotechnologies and neuromarketing.
This study seeks guidelines to assist market researchers, marketing professionals, data scientists, and developers in using neuromarketing and AI/ML algorithms ethically for consumer behavior and preferences, consumer segmentation, and buyer targeting while maintaining the benefits provided to consumers and businesses across industries. It also seeks to promote a more morally sound use of neuromarketing algorithms by offering viable solutions to address its challenges, enhancing understanding by raising awareness of the concerns in applying neuromarketing methods, and providing direction for future studies and innovations. The findings and recommendations can help practitioners, researchers, and stakeholders make better decisions about utilizing and implementing neuromarketing.
The researchers’ major difficulties and challenges were rooted in the complex ethical implications of using AI/ML algorithms in neuromarketing. One challenge was navigating the intricate balance between leveraging advanced neurotechnology for marketing effectiveness and ensuring consumer privacy and autonomy. The research sought to understand how these technologies could be applied ethically within the bounds of differing international data protection laws.
The research’s achievements included a comprehensive analysis that synthesized insights from a systematic literature review, expert surveys, and interviews. This multi-pronged approach allowed the researchers to craft nuanced policy recommendations to mitigate the ethical issues identified. Moreover, by comparing practices across the U.S. and Spain, the research made strides in understanding the global impact of neuroethics in neuromarketing, positioning its contributions at the forefront of the international discourse on AI ethics in consumer marketing.
2. Literature review
In the US, little is known regarding how consumers cope with privacy and data security risks, especially in the neuromarketing field. It is known that such algorithms have been finding their way into e-commerce, social media, the Internet of Things (IoT), and national security (Jin, Citation2018), as the threat associated with neuromarketing algorithms, privacy, and data security is fundamental. Primarily data-driven, such threats can directly or indirectly relate to AI/ML algorithms and other data technologies. For instance, as the expected value of data generated by neuromarketing algorithms is enhanced as it accumulates, companies are prompted to gather, collect, and store even more data, regardless of whether they train ML algorithms or use AI. In addition, another primary concern today is the need for internationally accepted principles regulating a responsible and ethical attitude to processing and using personal data for training systems with AI/ML algorithms that use these systems in the interests of business (Camilleri, Citation2023; Ozmen Garibay et al., Citation2023).
2.1. Privacy and data security in the US and the EU
The US has no overarching legislation on consumer privacy or data security. Currently, neuromarketing AI/ML algorithms’ consumer protection policies are a patchwork of local and federal regulations, with a few federal laws explicitly addressing privacy protection. They all tend to be industry-specific. For instance, the Children’s Online Privacy Protection Act of 1998 (COPPA) regulates online services for children under 13. The Health Insurance Portability and Accountability Act of 1996 (HIPPA) supplies data privacy and security provisions for medical records. The Gramm-Leach-Bliley Act (GLBA) regulates how financial institutions deal with personal data (Jin, Citation2018).
Privacy is subject to federal regulation by sectors. The Department of Health and Human Resources (DHHS) enforces HIPPA in health care. The Federal Communication Commission (FCC) regulates telecommunication services. The Federal Reserve System monitors the financial sector, while the Security and Exchange Commission (SEC) focuses on public firms and financial exchanges. The Department of Homeland Security (DHS) deals with terrorism and cybercrimes related to national security. The Federal Trade Commission (FTC) addresses privacy violations and inadequate data security as deceptive and unfair practices following the 1914 FTC Act. FTC’s privacy enforcement focuses on ‘notice and choice’, emphasizing how firms’ data practice deviates from the privacy notice they disclose to the public. This enforcement authority covers almost every industry and overlaps with many sector-specific regulators.
For sectors not subject to GLBA, HIPPA, or COPPA, no legislation mandates privacy notice, but many firms provide it voluntarily and seek consumer consent before purchase or consumption. Some industries also adopt self-regulatory programs to encourage certain privacy practices. For instance, the Digital Advertising Alliance (DAA), a non-profit organization led by advertising and marketing trade associations, establishes, and enforces privacy practices for digital advertising (Gehl, Citation2016). While privacy notice is something that consumers can access, read, and consent to, most data security practices are only visible once someone exposes the data vulnerability (via data breach or white-hat discovery). Accordingly, FTC enforcement on data security focuses on whether a firm has adequate data security, not whether the firm has provided sufficient information to consumers (Jin, Citation2018).
The EU has followed the ‘human rights’ approach, which curtails transfer and contracting rights often assumed under a ‘property rights’ approach. The EU recognized individual rights of data access, processing, rectification, and erasure in the new legislation. With its broad and transnational scope of application, the impact of GDPR, which entered into effect in May 2018, will also affect numerous companies outside the EU. Still, its effectiveness in protecting data privacy remains to be seen. However, two challenges are worth mentioning: First, for many data-intensive products (say self-driving cars), data do not exist until the user interacts with the product, often under third-party support (say GPS service and car insurance). Should the data belong to the user, the producer, or third parties? Second, even if property rights over data can be clearly defined, it does not imply perfect compliance. Both challenges could deter data-driven innovations if the innovator obtains the right to use data from multiple parties beforehand.
Data protection standards are becoming increasingly high, and companies face the more complex task of evaluating whether their data processing activities are legally compliant, especially internationally. Data can easily cross borders and play a vital role in the global digital economy. The processing of personal data occurs in various spheres of economic and social activity. The progress in information technology and digital business transformations makes processing and exchanging such data considerably easier (Voigt & Dem Bussche, Citation2017; Dąbrowska et al., Citation2022). In this context, the EU adopted the GDPR to harmonize further the rules for data protection within the EU Member States and raise privacy for the affected individuals.
2.2. Ethical concerns with neuromarketing strategies and applications
As consumer behavior has always been a marketer’s goal, the emergence of consumer neuroscience promising to shed light on consumers’ preferences and motivations, businesses have been increasingly attracted to this field. For over a decade, most of the discussion regarding neuromarketing applications and ethics concerns has concentrated on its commercial implementations as consumers fear that the data compiled can be utilized unethically (Hensel et al., Citation2017a, Citation2017b; Casado-Aranda & Sanchez-Fernandez, Citation2022; Tiutiu & Dabija, Citation2023). Consumer manipulation and the lack of transparency have been the primary concern, as big data and AI are reshaping consumer privacy and data security risks (Hensel et al., Citation2017a; Trettel et al., Citation2017; Wang, Citation2022). There is an increasing number of FDA-regulated AI/ML algorithms in the US but insufficient public information on validation datasets. These algorithms make it difficult to justify clinical applications since their generalizability and bias cannot be inferred (Ebrahimian et al., Citation2022).
2.2.1. Lack of transparency
Neuromarketing-based methodologies on relevant marketing stimuli lack transparency and reliability. Different companies offer services based on proprietary computational methods, algorithms, and approaches that are not entirely available to the scientific community or validated. This opacity in the methodologies employed by some companies and their results makes it difficult for researchers and scientists to separate supported and unsupported claims of validity of the services offered by those companies. Such ambiguity reflects common misconceptions toward public opinion and users of these methodologies regarding the effectiveness of these technologies (Trettel et al., Citation2017; Neuwirth, Citation2022).
Considering the perceptions and perspectives regarding these limitations, challenges, and potential solutions of neuromarketing implementations (Kaul, Citation2022; Alsharif et al., Citation2023b), a gap in the literature exists regarding the factors impeding the growth of neuromarketing, such as ethical and manipulation concerns, the high cost, the need for specialized expertise, lack of proper knowledge and understanding, the lack of financial resources, the lack of labs and facilities, and time requirements. Despite these obstacles, several scholars (Goncalves et al., Citation2022; Alsharif et al., Citation2023b) suggest potential solutions to enhance the application of neuromarketing, such as establishing collaborative solid networks, providing labs and facilities, increasing financial resources, complying with laws and regulations, and reducing tools and experiment costs.
The potential of neuromarketing can only be exploited effectively if trust in the field and industry rises, which correlates strongly with ethical conduct (Trettel et al., Citation2017; Wicinski, Citation2022). A significant downside of this opportunity is that, predominantly, several commercial studies confirm and need help with such ethical issues. Academics and business practitioners have hailed the development of a regulatory, ethical guideline in neuromarketing (Ulman et al., Citation2015; Briesemeister & Selmer, Citation2020). Most of the extant ethics codes are relatively too general, which can be helpful but does not elucidate specific essential issues for marketers (Hensel et al., Citation2017a, Citation2017b; Hula, Citation2022a). Consequently, Hensel et al. (Citation2016, Citation2017a, Citation2017b) developed an extended guideline known as the Ethical Guidelines in Neuromarketing (EGNM) as part of the Neuromarketing Science and Business Association (NMSBA) Code of Ethics, which supplies guidelines on steering neuromarketing research ethically.
2.2.1.1. Predicting consumer choice
The first commonly perceived potential ethical issue is the fear that neuromarketing may completely predict consumers’ choices. Similar criticisms regarding such prediction have been applied to traditional marketing research and practice but are perhaps most accentuated in neuromarketing (Hula, Citation2022b). Provocative research seems to herald the power of neuromarketing, as when fMRI has been used to predict individuals’ choices and purchase decisions (Schwarz, Citation2022; Kislov et al., Citation2023), like an EEG (Zhu et al., Citation2022), indicating that brain activity can foretell a consumer’s preference much better than self-reporting their preferences. Despite the narrowness of the assumptions drawn from these studies, all referring to data regarding brain predictors of preference in a single context, critics perceive such neuromarketing practices as a possible infringement of consumers’ rights to privacy (Penrod, Citation2022). It assumes that neuroscience techniques propose an outlet into consumers’ minds and extract data that consumers are unaware of, supplying a means to recognize consumers’ choices before making them.
Privacy concerns may arise for those accepting to participate in neuromarketing research, but these do not seem to be significant ethical hindrances for most (Saad, Citation2020; Penrod, Citation2022). This apprehension needs to be corroborated on numerous levels. First, most consumers do not have their brains scanned or provide hormone samples to investigators; that merely happens in the context of empirical research analyses (Verhulst et al., Citation2019). Thus, an individual consumer is not the explicit subject of a privacy infringement. Instead, findings are delineated based on inference to the general public from a small experimental population sample, as in existing marketing, biomedical, and behavioral research. Second, those who partake in scientific research are subject to an informed consent process in which they are apprised of the research’s goals and risks of participating in the experiment.
The general public occasionally interprets neuromarketing results as deterministic predictors of their behavior or preferences as prevenient from hard science. This perception may be exacerbated when results are exaggerated, as characteristic of many neuromarketing companies. Numerous scholars consider this view of consumers’ characterizations based on neuromarketing insights as belittling, dangerous, and immoral (Howard, Citation2020; Torbert, Citation2021; Mau et al., Citation2023). Another concern is that firms attempting to predict consumer choices will see and treat consumers as mere automata, or robots, without liberty to choose or dignity. The charge is that neuromarketers treat consumers as if they were only things to be used as mere means to the neuromarketers’ ends (Penrod, Citation2022; May, Citation2023).
Neuromarketing prognoses are probabilistic rather than deterministic, and firms engaged in such experiments need not claim that consumers’ preferences and behavior are entirely determined. Firms should recognize that consumers are at liberty to preclude whether to buy or not a product, regardless of their likes and dislikes or neurobias (Garofalo & Gallucci, Citation2021; Nguyen, Citation2021). Neuromarketing does not rely on the contemptible sentiment of consumers. Still, consumers can be predictable even if they are at liberty to choose (Suhler & Churchland, Citation2009; Liljevall & Lillskog, Citation2023). If consumers’ choices were predictable through traditional or neuromarketing investigation, they might be seen as sheer factors with relative value instead of individuals, undermining their dignity and respect.
Predicting consumer behavior and preferences significantly differs from compelling and manipulating consumers against their will. Such predictions must maintain the rationality and the dignity of the consumers’ preferences being predicted. Nevertheless, the neuromarketing domain is far from such a level of prediction. Even when neuromarketing companies anticipate consumers’ choices, they need not treat consumers as a means to an end. Instead, they can assist consumers in obtaining products or services with a more efficient rationale for why they want or need them (Keller, Citation2000; Taghikhah et al., Citation2021).
2.2.2. Influencing consumer choice
A commonly perceived potential ethical issue is the fear among consumers that neuromarketing can be used to go beyond prediction and influence consumer choice. Successful neuromarketing, it is argued, might rob consumers of control, and make the marketed goods irresistible (Garofalo & Gallucci, Citation2021; Närväinen, Citation2023). Of course, shaping consumers’ choices is the goal of marketing generally, but does neuromarketing offer firms a unique and novel ability to find a ‘buy button’ in the brain?
While neuroscience might help improve predictions of consumer choice, there is no current evidence of a ‘buy button’ in the brain. There are areas of the brain coded for value and reward (Clithero & Rangel, Citation2013; Garofalo & Gallucci, Citation2021; He et al., Citation2021), particularly the anticipation of reward (Ali et al., Citation2022). Things that are more rewarding or valued activate these areas more intensely, but this is not equivalent to a ‘buy button’. It should be noted that neuromarketing does not create the stimulus it tests (e.g. advertisement, promotional video, etc.), nor does it seek to make it unconscious. This discipline analyzes how the consumer responds to the stimulus to find out how it works, i.e. which areas are attended more or if it manages to excite, among other aspects (Halkiopoulos et al., Citation2022). Thus, neuromarketing provides no unique path—even in principle—for optimizing a marketing message to render consumers unable to control their actions; for example, neuromarketing could not create a menu description of an entrée that compels patrons to purchase that item any more than traditional marketing techniques could. In addition, even if it were plausible, targeting a person to define the optimal stimuli for their preferences would be impracticable (Martínez-López et al., Citation2020; Tirandazi et al., Citation2022).
Research has shown that supraliminal but unattended primes can significantly affect consumer behavior (Fitzsimons et al., Citation2002; Ferraro et al., Citation2009; Harmsel, Citation2021; Cheng et al., Citation2022). There is growing evidence that marketing information to which consumers are exposed can significantly affect their preferences, even when they were unaware that their choices and selections were being influenced or were exposed to brand information (Hayes et al., Citation2021; de Ridder et al., Citation2022; Goncalves et al., Citation2023). For example, a study by Goncalves et al. (Citation2023) manipulated the number of times consumers were exposed to photographs and videos of four leading road bicycles: Look, Specialized, Trek, and Pinarello. At the experiment’s end, consumers’ biosignals were analyzed to predict which brands consumers would prefer. The experiment analysis showed that consumers who participated in the experiment had different preferences depending on the age, gender, and aesthetics of each brand. While some consumers experienced surprise when exposed to a brand, others experienced disgust. Such an experiment clearly and causally demonstrated the power of marketing manipulation on consumers’ behavior that operates outside of conscious awareness. However, it could not predict consumers’ preferences, mainly due to neuro bias and the small population sample size (only nine subjects). However, it argued that consumers respond more favorably to a progressive combination of stimuli, from static posters and photos to videos, instead of only one type of stimuli. The study also showed that over-stimulation tends to lead to feelings of disgust.
While these studies demonstrate that behavioral research can elucidate strategies to influence consumers’ choices outside their awareness, they can also show that neuromarketing does not deserve any particular moral opprobrium and is certainly not the only way to influence consumers outside their conscious awareness (Carrington et al., Citation2010; Penrod, Citation2022; Reith, Citation2022). Critics might respond that all such unconscious influences—whether neuroscientific or not—are remote control. However, this reply conflates consciousness with control (Suhler & Churchland, Citation2009; Kalaganis et al., Citation2021), for example, as in the study by Goncalves et al. (Citation2023).
Such physiology-based marketing allows a better understanding of consumers’ decisions (Nanda et al., Citation2019; Mumtaz et al., Citation2021). Consumers decide freely, and neuromarketing tries to provide an answer to why they make specific choices by analyzing physiological factors that consumers cannot control, as well as their stated justifications (Bočková et al., Citation2021; Royo-Vela & Varga, Citation2022). This combination of data is relevant, given that consumers are unaware of all the factors that influence their choices. There is often a cognitive dissonance between the reasons they argue and the physiological responses triggered in their body. The current paradigm states that emotions are taken emotionally and justified a posteriori rationally (Mandolfo & Lamberti, Citation2021; Srinivasa, Citation2022; Seth & Mahato, Citation2023).
2.2.3. Brain and biometric data Overclaiming and manipulation
Some analysts argue that overclaiming, erroneous data sharing, and stealth marketing are significant causes for this industry’s lack of trust. Overclaiming is exaggerating brain and biometric data, which may emerge for the benefit of the company subsidizing the experiment where the outcomes are skewed in favor of their corporate objectives or adjusted based on a firm’s agenda (e.g. holding or maintaining consumers). Nonetheless, researchers (Thomas et al., Citation2017; Penrod, Citation2022a, Citation2022b) argued that overclaiming causes expectations to be inapplicable to what the research can reasonably deliver. In addition, falsely highlighted findings are often shared publicly to augment market share by employing anxiety or fear or the opposite, extremely optimistic (and inaccurate) data, which misleads the consumer into completing a purchase they otherwise would not have done. They argued that the ethical concern with the idea that neuromarketing is manipulative is that it arrests or overrides the consumer’s independence and autonomy. Even worse, stealth marketing, a marketing practice based on subtle persuasive cues provided by neuroscience insights, can potentially impact consumers below their conscious awareness (Mule, Citation2021; Penrod, Citation2022a, Citation2022b). Such practice is one of the top ethical concerns for the neuromarketing sector.
2.2.4. Protection of human subjects
Neuromarketing experiments demand human subjects, which can be challenging when the enrollment processes are ethical. There is a large and diverse number of potential human subjects, from individuals with disabilities, babies, and college students looking for some perks, all vulnerable and in an industry with almost no oversight or laws to protect them (Stanton et al., Citation2017; Thomas et al., Citation2017; Spence, Citation2020; Wicinski, Citation2022). Regarding consumer subjects, privacy is paramount (Belascu, Citation2020; McQuoid-Mason, Citation2022).
2.2.5. Reliability of results: Socioeconomic status and racism
Knowing that brain activity can be distinctively based on phenomenology, neuroscientists and neuromarketing scholars should be instructed to concentrate on a more diverse, multi-racial, human subjects experiment population sampling to achieve legitimate results, a rarely mentioned topic (Schibuk, Citation2022). Given that the typical participants in neuromarketing experiments are primarily from a white middle-class background, any insights drawn from these studies for global organizations targeting multicultural communities may be inadequate or misleading due to the limited racial diversity in the sample, as the subjects are drawn from societies that are Western, educated, industrialized, wealthy, and democratic. It is not appropriate to generalize the behavior of such individuals to the vast majority of humanity living under varying circumstances (Farah, Citation2017; Levrini & Jeffman dos Santos, Citation2021; Goncalves et al., Citation2022, Citation2023). Researchers incur the risk of reproducing scientific racism by omitting racial experiences that do not fit or are too tricky to understand in neurobiological calculations (Krieger, Citation2021; Rollins, Citation2021).
No human brain exists out of context; human brains are lived experiences that affect how they behave and how the body reacts (Greely, Citation2021; Nguyen et al., Citation2023). Because of this, neuromarketing investigators should assess how socioeconomic status (SES) and racism affect the brain and biometric activity (Agyeman et al., Citation2022; Goncalves et al., Citation2022). fMRI experiments have demonstrated that SES has an effect and encountered distinctions in how brain systems are engaged for executive functions that have not been observed in behavioral analyses (Farah, Citation2017; Merz et al., Citation2019; Saarikivi et al., Citation2023).
Using Diffusion Magnetic Resonance Imaging (dMRI), Assari and Boyce (Citation2021) showed that the impacts of SES indicators on cerebellum cortex microstructure and integrity are more fragile in black than white households. Such result aligns with Marginalization-related Diminished Returns (MDRs), defined as diminished effects in the SES index for blacks, minority groups, and other races compared to whites (Assari & Boyce, Citation2021). Other researchers (Masten et al., Citation2011; Atlas, Citation2021; Hamler et al., Citation2022) found that in reaction to being socially excluded by whites, black subjects seemed more distressed, significantly demonstrating more social pain-related neural activity and diminished emotion regulatory neural activity.
In addition to the lack of representation in lab population sampling, biometric analysis and secondary tools should be revisited for their effectiveness in testing multiracial diverse subjects; one such tool is AI/ML, often used as a secondary neuromarketing tool. Researchers use AI/ML algorithms to collect and analyze data from other neuro tool sources, enabling a more in-depth and reliable understanding of consumer behavior and preferences (Hawkins, Citation2022; Kliestik et al., Citation2022; Srivastava & Bag, Citation2023). However, some challenges are the proliferation of groupthink, insularity, and arrogance in the AI community, to name a few (Metz, 2021; Bedoya, Citation2022; Goncalves et al., Citation2022). AI/ML algorithms have not yet learned to recognize the differences and peculiarities among black faces because the images used to train it mainly had been of white faces, and neuromarketing researchers may not be accounting for this disparity (UN News Global Perspective Human Stories, Citation2020; Tippins et al., Citation2021).
2.3. Ethical concerns with ML algorithms in consumer behavior and preferences
One reason for introducing ML algorithms in neuromarketing is the increasing global market competition. Marketers seek a path to satisfy consumer needs while also delivering better service. However, understanding consumers’ demands requires greater spending. According to recent studies, almost half of the global corporations have already invested in developing ML algorithms, while nearly a quarter of them are in the learning stage and hope to integrate them into their business platforms accordingly (Lee & Shin, Citation2020; Hayes et al., Citation2021; Jaiswal et al., Citation2022). Hence, a significant strength of ML is its ability to manage a massive amount of unstructured data, such as text, video, and images (Adnan & Akbar, Citation2019; Ma & Sun, Citation2020; Velkova, Citation2023), very quickly and efficiently, saving time and money for marketers when analyzing thousands of consumer data segments.
These corporations investing and deploying ML algorithms benefit from comprehensive data analysis to align consumers’ needs and demands with market decisions (Adnan & Akbar, Citation2019; Velkova, Citation2023). Marketers best respond to consumer demands through segmentation (Velkova, Citation2023). As consumers are grouped and profiled, algorithms reduce the need for consumer analysis and observations (Velkova, Citation2023) by learning and making such information readily available to marketers. Another advantage of neuromarketing and ML algorithms is their predictive capabilities, as once an algorithm has recognized patterns in trained data, it can quickly forecast consumer product or service preferences (Ma & Sun, Citation2020; Velkova, Citation2023). ML algorithms can anticipate human behavior by modeling an extensive array of variables, matching products/services, and clients’ demands (Kotras, Citation2020). After segmenting a consumer base, targeting is the next step in the marketing strategy, requiring a thinking and more complex algorithm (Huang & Rust, Citation2022; Velkova, Citation2023), as the process requires features such as judgment, knowledge, decision-making skills, and, thus, more complex algorithms. Recommendation engines or predictive modeling are often adopted in these targeting processes (Liu & Chen, Citation2019; Huang & Rust, Citation2022).
Broadly, the focus surrounding AI/ML algorithm ethics has been on the opacity of how these algorithms reach decisions. No one knows how an algorithm makes a specific decision and why. While an algorithm makes decisions on a chess game or checkers, no one cares (Loi et al., Citation2021; Shin et al., Citation2022). However, when an ML algorithm decides whether a consumer obtains a loan or not or is racially or socioeconomically discriminated against, several ethical issues arise. Consumers deserve and potentially are entitled to an explanation of how decisions to accept or decline a loan were reached since such digitally automated decisions may harm the consumer whose loan has been declined. The same is true when a consumer is discriminated against. While not physical, such harms can significantly impact consumers’ lives, credit scores, and socioeconomic status due to an algorithm’s decision.
2.4. Principles of ethics
Whether neuromarketing algorithms are ethical or not, and whether these algorithms’ decision outputs concerning consumers are moral, correct, or wrong, such applications may be harmonized and able to be performed by comprehending the various viewpoints of ethics. The principles of ethics are split into four branches: meta, applied, descriptive, and normative. Meta-ethics is concerned with developing a broad understanding of ethics (Allan, Citation2015; Seif-Farshad et al., Citation2021; Poulsen & Christensen, Citation2023). Normative ethics focuses on developing ethical standards and responsible behavior (Copp, Citation2005; Laczniak & Murphy, Citation2019; Remišová et al., Citation2019; Liu et al., Citation2020). Applied ethics concentrates on pragmatic quandaries or controversial issues (Leese et al., Citation2019; Bang et al., Citation2022; Mason, Citation2023). Descriptive ethics concentrates on people’s behavior and moral standards (Hämäläinen, Citation2016; Laczniak & Murphy, Citation2019; Forbes et al., Citation2020; Jiang et al., Citation2021a, Citation2021b).
This study leans on the theories of normative ethics to assess which actions are ethical while responding to the research questions. The three broad types of normative ethics are consequentialism/utilitarianism, virtue ethics, and deontology (Harsanyi, Citation1977; Portmore, Citation2020; Ranganathan, Citation2022). Although within the same branch, each imbues distinct values and beliefs. Utilitarianism, similar to consequentialism, shares various tenets. While consequentialism does not specify any desired outcome, utilitarianism defines good as the outcome (Rachels & Rachels, Citation2012; Scheyer, Citation2019; Gesang, Citation2021; Andersen, Citation2022): an action is ethical if the overall benefits outweigh the widespread harm (Rogerson et al., Citation2017; Sætra, Citation2020). Deontology undervalues the outcome and questions the act’s morality instead. Nevertheless, virtue ethics drives the individual toward achieving the ultimate goal while maintaining high standards of excellence (Scheyer, Citation2019; Gal et al., Citation2020; Gesang, Citation2021; Penrod, Citation2022a) and practicing good traits such as compassion, respect, and generosity.
Several scholars have investigated the ethical risks of AI/ML algorithms (Stanton et al., Citation2017; Hensel et al., Citation2017b; Kritikos, Citation2018; Magalhães, Citation2018; Susser, Citation2019; Sample et al., Citation2020; Sætra, Citation2020; Attié et al., Citation2021; Sidorenko at al., Citation2021; Tsamados et al., Citation2021), but the emphasis has not explicitly been on those in neuromarketing (Clark, Citation2020). As a result, the literature on ethical issues of such algorithms and how they emerge in the neuromarketing industry is addressed in this study.
3. Objectives and research questions
The literature cited in this study is more about privacy and data security than neuromarketing AI/ML algorithms due to the scarcity of more specific data. The extant literature lacks explicit guidance on how neuromarketing AI/ML algorithms can continue to operate effectively and ethically. For this purpose, two regions were studied and compared: the US, for its lack of an ethical consumer protection guideline such as EU’s GDPR, and Spain, due to the close collaboration of research on the theme by three of the authors, and to assess how the EU’s GDPR impacted countries in the EU, such as Spain, in the protection of consumers’ privacy. Another goal was to draw a comparison between the two countries, one subject to GDPR and the other absent from any country-wide comprehensive guideline, intending to identify challenges in ethical frameworks and opportunities compared to the lack of similar regulations in the US. This research attempts to answer how neuromarketing algorithms can create and retain value within the ethical boundaries of consumer behavior and preferences, segmentation, and targeting.
3.1. Theoretical framework and conceptualization
Although many ethical theories exist, each with differing points of view, this study adopts rule utilitarianism as a framework for analysis. Its premise is that an act must maximize the overall good for society and adhere to specific regulations (Bowen, Citation2020). In this research context, a utilitarian framework can be operationalized by evaluating the consequences of neuromarketing practices powered by AI/ML algorithms in terms of overall benefit or harm to society. Descriptively, this framework would assess existing neuromarketing strategies to determine how they align with maximizing consumer welfare and protecting privacy. Normatively or prescriptively, it would guide the creation of policies and practices prioritizing actions yielding the greatest aggregate utility. Neuromarketing strategies should increase business profits and enhance consumer satisfaction and trust without infringing privacy rights. The utilitarian approach advocates for neuromarketing strategies that support the common good by being transparent, non-manipulative, and respectful of consumer autonomy and data protection norms.
When rule utilitarianism is applied to neuromarketing algorithms in Europe, the Charter of Fundamental Rights of the EU sets the limits, protecting universal values based on law and democracy (De Gregorio, Citation2018; Ivanova, Citation2021). In such a case, rule utilitarianism classifies these algorithms as unethical if they ignore human rights or if the benefits they deliver to individuals do not exceed the risks. Nonetheless, connecting distinct modes of profiling with Foucauldian thought on governing, pattern-based categorizations in data-driven profiling, safeguards such as the Charter of Fundamental Rights of the EU or its data-protection framework lose their applicability to a diminishing role of the tools of the anti-discrimination framework (Van Den Meerssche, Citation2022).
Some consumer protection laws and regulations apply to neuromarketing algorithms in the US. For instance, the Federal Trade Commission (FTC) enforces consumer protection laws and regulations prohibiting unfair or deceptive marketing practices, including some guidelines for using neuromarketing techniques (Savage, Citation2019; Alarcon & Ha, Citation2020; Galli, Citation2022). The FTC has also brought enforcement actions against companies that have used deceptive or unfair neuromarketing techniques (Wexler & Thibault, Citation2019; Bakir, Citation2020). Some examples include brainwave analysis and biometric data collection in advertising and marketing. Additionally, some states have consumer protection laws that apply to neuromarketing algorithms. For instance, California has a data privacy law, the California Consumer Privacy Act (CCPA), which gives consumers the right to know what personal information is being collected about them and opt out of selling their personal information (Baik, Citation2020; Mulgund et al., Citation2021). Nevertheless, no comprehensive federal law currently regulates neuromarketing algorithms in the US, allowing for gaps in consumer protections, particularly concerning emerging AI/ML algorithms, augmented and virtual reality, and other neuro technologies that existing laws and regulations may not cover (Luna-Nevarez, Citation2021; Kalkova et al., Citation2023).
Against this backdrop, this study endeavors to conduct a systemic review of the field of neurostrategy to scrutinize the isolated and fragmented attempts of conjoining neuromarketing and neuroethics in the extant literature and fill various gaps that are currently limiting the field, such as lack of (a) a formal research agenda; (b) knowledge about the concepts at the confluence of neuromarketing and neuroethics; and (c) clarity about what is ‘hot’ and what is ‘not’ in the field (Cristofaro et al., Citation2022). The following questions drove this study phase before conducting semi-structured interviews: a. What are the dominant concepts at the junction of neuromarketing and neuroethics? b. What are the broader links between these concepts? c. How has the scholarly discourse around neuroethics evolved? d. Which trends are gaining traction within neuroethics research? e. What propositions can be developed to advance empirical assessment in neuroethics related to AI/ML algorithms? A thematic (conceptual) and semantic (relational) analysis of the literature was conducted to attempt to answer these questions.
Investigating neuroethics through advanced bibliometric methods allows for a more robust, systematic, and comprehensive literature review (Kaur, Citation2023). Hence, this study contributes to the literature by laying down solid foundations of neuroethics based on machine-based identification of the core concepts and their relationships. Moreover, it offers a bibliometric mapping of the AI/ML-related neuromarketing and neuroethics research themes from 2019 through 2023, revealing the trends and bridging the gaps in this relatively novel field of scholarship.
4. Methodology
In addition to a survey and semi-structured interviews with expert academics and business practitioners from the field, both scientometric analysis and systematic literature review techniques have also been employed to analyze the literature thoroughly.
4.1. Study design
To provide further insight into the ethical issues concerning the combination of neuromarketing and AI, the design of this study is qualitative exploratory. Data collection methods were based on critical literature reviews, a survey, and semi-structured interviews with subject matter experts from the US and Spain to bridge academic and industry perspectives regarding the overarching question. The answers provided by these two groups were analyzed to understand best and compare the level of awareness of each group related to neuroethics, consumer privacy protection and legislation, and more internalized ethical guidelines adopted when considering neuromarketing initiatives and respective use of AI/ML algorithms. The findings from the literature review were adapted from similar research methods in this field (Xiao & Watson, Citation2019; Jöhnk et al., Citation2021), built upon during the interviews, fostering a critical thinking process and providing a comprehensive understanding of the status quo.
4.2. Participants
Several scholars (Coper Joseph, Citation2014; Adams, Citation2015; Galvin, Citation2015; Vogel et al., Citation2019; Gill, Citation2020) argue that an adequate number of interviews for qualitative research should range between five and 20, depending on its scope. Considering such ranges, using a random snowball sample selection approach, the authors invited several business and academic experts via LinkedIn or through professional connections at universities and corporations using or proficient in neuromarketing algorithms to answer the online survey and indicate if they would be willing to be interviewed. Few of those who answered the survey were willing to be interviewed. We ended up with 14 interviewees who were not gender-matched: Seven experts in the US and seven in Spain. They all had diverse backgrounds and years of experience in neuromarketing (see ). They were interviewed to maximize the diversity of opinions and find similarities and differences among them (Yin, Citation2014; Alamri, Citation2019). None of them had collaborated in the creation of an ethical code in neuromarketing.
Table 1. List of participants’ profile.
lists all participant’s profiles. Regarding the US interviewees, a total of seven interviews were carried out. The sample was not gender-matched (30% male, 70% female) aged 25 to 54 years (M = 36 years, SD = 9 years), 42% had a Ph.D., and 85% had more than five years of experience in the field. For Spaniard interviewees, a total of seven interviews were carried out. However, at the end of the session, one interviewee requested that the data be erased, which was promptly granted. The sample was also not gender-matched (33% male, 67% female) in an age range of 40 to 63 years (M = 51 years, SD = 11 years), 67% had a Ph.D., and 83% had more than five years of experience in the field.
4.3. Ethical aspects
Semi-structured interviews were conducted via Zoom in the interviews’ native language, English and Castilian, respectively, to create an atmosphere of trust and tranquility in which they felt free to express their opinions on such a sensitive subject as ethics and neuroethics (Jehn & Jonsen, Citation2010; Islam & Aldaihani, Citation2022). Informed consent was obtained from all participants, and their permission was sought for a voice recording of the session.
4.4. Data collection and analysis
The semi-structured interviews were conducted in May and June 2023 across Spain and the US, delivered in Castilian and English, correspondingly, through Zoom or Microsoft Teams. Each session lasted between 45-60 minutes. Participants were selected using purposive snowball sampling to ensure a diverse representation of the study’s target demographic, with ethical consent obtained digitally before the interviews. Digital recordings were meticulously transcribed and, where necessary, translated into English, followed by a retranslation to Castilian for cross-verification of translation accuracy. NVivo, a qualitative data analysis software, was used to identify themes, ensuring analytical rigor. Measures such as member checking and triangulation were incorporated to ensure reliability and validity. The researcher’s influence was minimized through reflexive practices, and all data were handled with strict adherence to confidentiality and data protection protocols. Follow-up interactions were scheduled as needed to clarify participant responses and deepen the understanding of emergent themes.
4.4.1. Methodical literature review and scientometric analysis
To minimize subjectivity and arbitrariness in the current systematic analysis and review, this study followed the guidelines and principles outlined by the Preferred Reporting Items for Systematic Reviews and Meta-Analyses (PRISMA) 2020 statement and checklist (Radua, Citation2021). The bibliometric mapping was conducted using VOSViewer, which was selected over R-tools and the like for the bibliometric analysis primarily due to its user-friendly interface and specialized capabilities tailored for bibliometric data. Unlike other tools, such as R, which requires data analysis and visualization programming skills, VOSViewer provides a more accessible platform, especially for less-skilled coding users. Its advanced visualization features allow for the creation of high-quality, interpretable bibliometric maps, simplifying the complex data interpretation process. VOSViewer’s design is optimized for handling bibliometric data sources like Web of Science and Scopus, enabling efficient data importation and network creation. Additionally, the support community for VOSViewer is dedicated to bibliometrics, offering focused assistance and updates that are particularly beneficial for this research field.
4.4.1.1. Eligibility criteria
The journal articles, theses, dissertations, editorials, reviews, books, book chapters, proceeding papers, and scientific texts that somehow address neuromarketing, neuroethics, and the application of AI/ML algorithms to neuromarketing formed the initial criteria for eligibility for this review. Along with published articles, early access articles in Web of Science’s business or management category were part of the studies under consideration. Moreover, the eligibility criteria included scientific texts written in English. Furthermore, studies published (or available as early review) at the confluence of neuromarketing and neuroethics were part of this review.
4.4.1.2. Information source
In conducting bibliometric analysis, including diverse document types such as full-text articles, reviews, editorials, proceedings, abstracts, technical papers, and chronologies is imperative for a comprehensive synthesis of academic discourse and scientific advancement. Full-text articles provide the core substance of research findings; reviews offer synthesized knowledge and identify trends; editorials present insights and scholarly opinions that reflect the field’s dynamics; proceedings capture the cutting-edge research presented in conferences; abstracts allow for rapid appraisal of research scope; technical papers present detailed technical findings; and chronologies map the historical progression and milestones in a discipline (Aria & Cuccurullo, Citation2017). This multifaceted approach enables a robust understanding of the research landscape, scholarly communication patterns, and the evolution of thought within a field, thereby supporting the identification of seminal works and emerging trends critical for academic and practical applications. As this research scope enwrapped fast-changing and ever-evolving technology updates, only resources from January 1, 2019, through May 31, 2023, were reviewed, including scientific papers, web-based articles, and secondary statistical data.
The Clarivate Analytics Web of Science Core Collection database was used to extract the most valuable and high-impact research data from other existing databases (such as Scopus, EBSCO, and Google Scholar). Web of Science database was specifically chosen for this study for five reasons: (1) It is the most updated database for bibliometric studies, which includes most of the papers published as well as recently accepted by reputable journals (Kaur, Citation2023; Rialti et al., Citation2019); (2) It covers wide variety of publication formats such as full-text articles, reviews, editorials, proceedings (journals and book-based), abstracts, technical papers, and chronologies. SCOPUS covers a wide range of document types; however, chronologies, timelines of historical events, or developments in a particular subject area are not standard document types cataloged in SCOPUS, as they focus on scientific documents and their bibliographic information rather than on providing historical timelines like a chronology (Kaur, Citation2023); (3) It includes more than 90 million records in comparison to 69 million records on Scopus (Moral-Muñoz et al., Citation2020); (4) It has broader temporal coverage than that of Scopus (Kaur, Citation2023); and (5) It provides consistent results for the same query used with similar search parameters over time (Kaur, Citation2023; Marzi et al., Citation2020). Thus, Web of Science is particularly suitable for this study as it provides the most updated, accurate, efficient, and reliable database with the broadest temporal coverage for performing a robust analysis of machine-readable scientometric data (Wilden et al., Citation2019).
Moreover, the choice of the database is consistent with prior bibliometric studies in business management research, such as Kaur (Citation2023), Marzi et al. (Citation2020), Rialti et al. (Citation2019), and Wilden et al. (Citation2019). In addition, Kaggle, Mordor Intelligence (Mordor Intelligence, Citation2021), and Statista were utilized to access secondary quantitative data, such as the adoption rates of AI/ML techniques in the neuromarketing field and public opinion survey results for data privacy concerns. Furthermore, this research relied on online blogs, reports, and articles to aggregate more detailed illustrated data about the algorithms’ application in neuromarketing and their inherently hidden ethical risks. The online papers help derive insights about the companies that have adopted automated targeting and personalization and recent scandals in the media arising from the data collection and analysis with neuromarketing’s ML algorithms. Language limitations have meant that the list of companies and media is far from exhaustive, but this article aims to contribute to a more extensive research process.
4.4.1.3. Search strategy
Following the best practices suggested in the literature to select a search query (Kaur, Citation2023), an in-depth analysis of the abstracts, author’s keywords, and literature reviews of published research articles at the junction of neuromarketing and neuroethics for AI/ML driven applications were conducted to assist in the identification of concepts that are being used in the scholarly discussion. After several iterations to define a broad research query, the following query was selected and implemented to capture the essence of neurostrategy effectively:
Written in English; keywords, title, and summary in English. These criteria were chosen to link the study objective to the research area, with a series of exclusion criteria applied to the latter. The inclusion criteria considered the following search queries:
‘neuromarket* algorithms AND marketing’, ‘artificial intelligence OR machine learning AND ethics’, ‘artificial intelligence OR machine learning AND neuromark*’, ‘personalized market* AND consumer privacy’, ‘ethical issues AND neuromark* algorithms’, ‘ethical AI’, ‘GDPR and neuromarket*’, ‘GDPR and AI’, ‘ethical theories and neuromarket* algorithms’, and ‘neuroethics and algorithms’.
4.4.1.4. Selection process
The above search query returned 587 results, with the language (English) and categories (business and marketing) filters in place. To refine these results, only the studies published in business, marketing, or neuromarketing journals were filtered out for further analysis. This selection process resulted in an initial sample of 195 studies. After that, a revision of the title and abstracts of these articles was conducted to determine the relevance of each article to the scholarly discourse about neuromarketing and neuroethics related to AI/ML algorithms applications. This manual review process weeded out 82 articles that were completely unrelated to the field (e.g. results for the search term market* leading to topics covering traditional marketing strategies, 4 P-7P strategies, robots, ML application strategy; or search for term neuroethics resulting into hits that involved studies on neurology studies; or search for the term neural* yielding results on studies dedicated to AI/ML neural networks). This process resulted in 113 studies that were considered for further screening. In the next step, four duplicate records were removed, which resulted in 109 unique studies. These studies were then analyzed in-depth based on the full text, and the following inclusion and exclusion criteria were applied to them:
Inclusion Criterion: The articles focused on the application of neuromarketing concepts, knowledge, or methods to investigate the application of AI/ML algorithms or neuroethics ramifications of such algorithms on consumer privacy, GDPR, consumer segmentation, selection and/or its outcomes, such as competitive advantage or enhanced neuromarketing strategies.
Exclusion Criterion: The exclusion criteria used to select the documents were research articles, review articles, letters to the editor, conference summaries, and lectures. No geographical restrictions were applied as studies from different continents relating to the variables and research areas found in the SCOPUS and Web of Science databases was considered in the review. Also excluded were studies focusing on the utilization of neuromarketing and neuroethics in other niche branches of management, such as NeuroIS, NeuroFinance, neurostrategy, neuro decision-making, etc., which were intentionally not included in the sample to dedicate this study.
After applying these inclusion and exclusion criteria to the initially identified data set of 109 studies, two did not meet the inclusion criterion and, thus, were not considered for further analysis. On the other hand, three studies were excluded based on the exclusion criterion. Furthermore, the reference lists of all articles retrieved from the search were subjected to manual review to identify additional studies that may fit the objectives of this study, but no additional relevant articles were identified. Hence, the final data set comprised 104 focal articles (86 journal articles, six book chapters, seven proceeding papers, three editorial materials, and two reviews), comparable to the sample size of similar studies in the field (Ascher et al., Citation2018). A flowchart depiction of this process is shown in .
4.4.2. Online survey
An online survey was set up in Qualtrics, containing 25 questions. Seven covered sociodemographic data, and four assessed the respondent’s perceived relationship between neuromarketing and AI/ML. Six evaluated the respondents’ general knowledge of ethical codes related to the discipline/industry, and seven assessed the ethical risks of AI/ML algorithms perceived at universities and the business environment. The last question asked respondents about their interest in being interviewed and, if so, their contact information.
Since this study relied on the EU’s GDPR as a consumer privacy guideline and aimed to compare best practices in the ethical use of AI/ML algorithms for neuromarketing in the US and the EU, data were collected in Spain and the US. The population selection criteria comprised Spaniards and American academic experts or business professionals in the digital and neuromarketing discipline/industry, directly or indirectly involved with AI/ML algorithms as developers or users (e.g. engaged with digital or neuromarketing, advertising), with at least three years of related experience and a member of a higher education institution or a company involved in the development or use of AI/ML algorithms for digital or neuromarketing applications. A total of 735 email invitations to complete the online survey were sent. These were gathered from prospects sourced from LinkedIn and the researchers’ connections with academic research institutions and business experts in the US and Spain. Then, a snowball approach was adopted by asking respondents to forward the survey link to other prospects they knew and met the survey criteria. The snowball sampling technique was chosen over other approaches, such as purposeful sampling, due to its effectiveness in reaching a specialized population that is difficult to access. This research delves into the niche intersection of neuromarketing, neuroethics, and AI/ML algorithms, so identifying subjects with the requisite expertise and experience was challenging. Snowball sampling allowed for utilizing the networks of initial participants to identify subsequent subjects, thereby capitalizing on the interconnectedness of professionals in this specialized field. This approach broadens the reach within a targeted community by leveraging personal connections. It increases the likelihood of including participants with the deep, nuanced knowledge necessary to contribute valuable insights to the study.
4.4.3. Semi-Structured interviews
The interviewees were selected based on their field of expertise, professional experience, and research activity. The selection criteria included expertise in neuromarketing algorithms or ML expertise, marketing, digital marketing, and ethics. The diverse respondents being interviewed were crucial in thoroughly answering the research questions, including researchers in these fields, corporate marketing representatives, data scientists, AI/ML professionals, ethics, and marketing professionals,
Since this study was conducted when the COVID-19 pandemic was still lingering, protecting the health and safety of respondents and researchers was among the top priorities. The interviews were conducted via Zoom. First, the interviewees were contacted via email. Then, a research prospectus, an invitation to the interview, an informed consent form, and a list of the main questions to be asked during the interview were provided. The respondents’ identity and confidentiality were protected by generating a code for each of them, but their current occupation/position and primary demographic data can be found in . The interviews were transcribed and anonymized. The interview transcripts were coded and analyzed using NVivo, which has robust tools to facilitate the systematic coding and analysis of semi-structured interviews, allowing the organization, categorization, and retrieval of text efficiently.
5. Research findings
The following are the results of various bibliometric analyses undertaken to unveil the field’s conceptual structure. Semantic relatedness (e.g. the frequency and proximity with which the concepts co-occur in the text) has been studied, and various maps of meaning have been generated to systematically answer this study’s research questions based on a few bibliometric indicators.
5.1. Dominant concepts at the junction of neuromarketing and neuroethics
and are word clouds extracted from the interview transcripts of the US and Spain interviewees. They underline the main themes in the minds of the interviewees when asked about their understanding and opinions about neuromarketing’s AI/ML algorithms’ applications at the junction of neuroethics. For American interviewees, the word ‘data’, followed by ‘algorithms’ and ‘consumer data’, were the highlights in the word cloud as it has the highest co-occurrence index in the sample. Nonetheless, the perimeter of the word cloud reveals the amplitude of other vital topics. It can be expected, therefore, that in the quest to develop a more ethical approach to neuromarketing AI/ML algorithms, awareness of what may be considered ‘unethical’, ‘consumer privacy’, ‘ethics’, and many other factors is warranted.
Figure 2. US Sample Word cloud: Data, followed by algorithms and consumer data, were the main themes with the highest co-occurrence index in the sample.

Figure 3. Spain Sample Word cloud: Ethics, followed by a concern with artificial intelligence, algorithms, guidelines, and neuromarketing, were the main themes with the highest co-occurrence index in the sample.
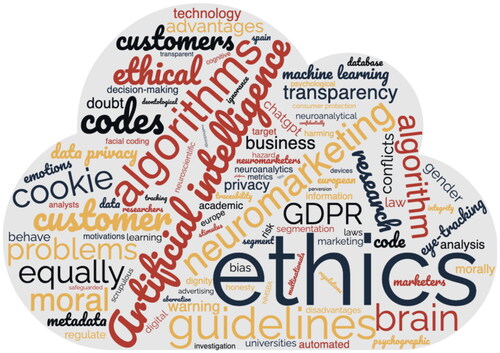
For the Spanish word cloud, the word ‘ethics’, not data as with the American sample, takes center stage, followed by a concern with ‘artificial intelligence’, ‘algorithms’, ‘guidelines’, and ‘neuromarketing’.
and are the result of a co-word analysis performed using VosViewer as an analytical tool to understand the conceptual structure of the field. Accordingly, the results are interpreted on the comparative positions of words and their dispersion along the dimensions, i.e. closer words are more similar in distribution. The co-word analysis depicted in , showing the association of strength of these major themes, suggests that the foundational concepts at the junction of neuromarketing AI/ML algorithms and ethics can be distinguished into nine different networks of concepts, listed in order of magnitude:
Figure 5. Neuromarketing and Neuroethics Bibliometric Analysis - Fractionalization of total link strength.
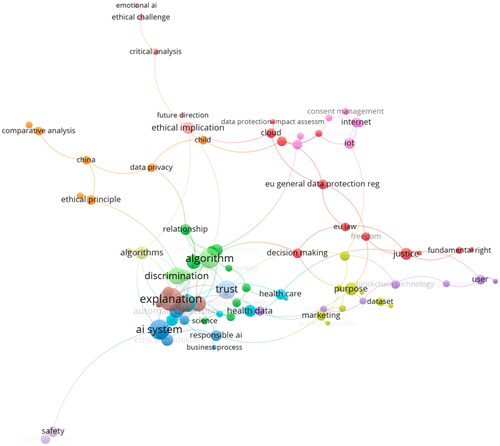
Network 1 focuses on constructs attempting to explain algorithm decisions and safety implications.
Network 2 is focused on trust, content analysis, data, marketing applications, and its purposes.
Network 3 emphasizes the vital interest of researchers in the issues related to algorithms, discrimination, relationships, and content analysis, among secondary interest in data and health applications.
Network 4 underlines researchers’ interest in neuromarketing and neuroethics, especially in neuromarketing methods, applications, protocols, and other minor interest themes such as content analysis and data management.
Network 5 highlights insights from related topics such as AI/ML algorithms, the purpose of such algorithms, marketing, and datasets.
Network 6 focuses on ethical implications, challenges, data governance, GDPR, the cloud, decision-making, freedom, and power.
Network 7 underscores consumer justice, focusing on fundamental rights, freedom, decision-making, data governance, and datasets. This network also includes analyzing the role of various significant factors it intersects, such as trust, risk, algorithms, methods, implicit knowledge, and gestures, in individual decision-making.
Network 8 is less significant than the first four networks. However, it concentrates on the more technological aspects of neuromarketing and AI/ML algorithms, including blockchain technology, privacy preservation, user/consumer privacy protection, protocols, and security analysis.
Network 9 embodies subtopics related to comparative analysis, ethical principles, data privacy, and children’s exposure to such technologies, with several interlinks to algorithms, trust, and ethical implications networks.
Another bibliometric angle of analysis, a thematic map analyzed through a fractionalization of total link strength, the total strength of the co-authorship links of a given researcher with other researchers, is shown in . It reveals additional critical neuromarketing, AI/ML algorithms, and neuroethics themes. This map reveals various research themes’ centrality (importance) and density (development). As shown, AI systems, responsible AI, emotional AI, business processes, data protection impact assessment, consent management, and automation represent important themes being researched. Hence, scholars in the field must continue to work towards further developing these themes, given their importance for foundational as well as future research in the field of neuromarketing’s use of AI/ML algorithms and neuroethics.
The bibliometric analysis also shows a thematic evolution of topics over recent years, demonstrating how the research in the field has evolved. It shows that even though the research on understanding AI/ML algorithms application in neuromarketing has been growing fast, the scholarly focus on neuroethics, including broad ethical considerations of such applications, possibly prompted by regulations, such as the EU’s GDPR and the new US’s CPRA, which became effective in January 2023. Moreover, increased interest can also be seen in interlinked topics such as neurotech (e.g. EEG, MEG, fMRI, GSR, eye-tracking, pupilometry, face coding, AI/ML algorithms, emotional AI, etc.), neuromanagement, IoT, cloud technologies, blockchain. Nevertheless, since consumer decision-making is a significant theme, scholars are encouraged to continue researching this field.
5.2. Online survey results
The survey dataset records responses from 60 digital and neuromarketing professionals from the US and Spain. The respondents’ gender distribution indicates that 40% (24) identified as male, while 56.67% identified as female. Additionally, 3.33% preferred not to disclose their gender. Regarding nationality, 12% were from the US, 27% were from Spain, and 3% chose the ‘Others’ category, while 58% did not specify their countries directly.
The survey aimed to gather insights into the participants’ opinions and awareness regarding the ethical dimensions of AI/ML and neuromarketing. Participants were asked for opinions regarding specific statements related to the research topic and select their level of agreement on a Likert scale ranging from 1-5, where 5 indicates strong agreement:
Neuromarketing and artificial intelligence are complementary and should not be used separately. – Out of 57 participants, 46% agreed, 11% strongly agreed, and 28% disagreed.
Neuromarketing benefits from artificial intelligence. – Of 57 responses, 60% agreed, 23% strongly agreed, and 9% disagreed.
Using machine learning algorithms for consumer/consumer preferences segmentation and targeting has many benefits. – Out of 57 responses, 82% agreed or strongly agreed, while 12% disagreed or strongly disagreed.
Participants were asked to select statements on which they agreed. The top five most selected statements, with each receiving more than 30 responses, included:
I understand how AI can be used in neuromarketing.
There are some disadvantages to using machine learning algorithms for consumer preference segmentation and targeting.
I understand how neuromarketing specifically benefits from AI.
The use of AI should have limits when applied to neuromarketing.
Artificial intelligence and machine learning are there to help the corporate operations and marketing teams have a wide neuromarketing scope to convey massive value to AI’s potential data.
For the second part of the survey regarding ethical considerations, participants were asked to select Yes or No to the following questions:
Are you aware of any codes of conduct or other written agreements for ensuring ethical businesses, or are the employees, for example, trained to behave morally? – Of 54 responses, 54% replied yes, 33% no, and the remaining were unsure.
Are you aware of current guidelines data scientists use to limit moral hazard to individuals? – Out of 54 responses, 61% replied no, 28% yes, and the remaining were unsure.
Are you aware of any code(s) of ethics for neuromarketing? – Out of 54 responses, 61% replied no, 20% yes, and the remaining were unsure.
Do you consider any of the ethical codes you are familiar with when doing research, whether academic or professional? – Of 54 responses, 59% replied yes, 35% no, and the remaining were unsure.
Can the ethical issues be traced back to the origin and determine who is responsible for them, both at companies and universities? – Of 54 responses, 52% replied yes, 20% no, and the remaining answered sometimes.
Are technical or ethical guidelines needed to ensure ethical data analysis? – Out of 54 responses, 94% replied yes, 4% no, and the remaining were unsure.
The survey also asked for opinions on other ethical risks for companies and universities:
Companies can ensure that they are intrinsically safe from the potential to harm consumers on a moral level when adopting AI and neuromarketing strategies. – Out of 54 responses, 61% disagreed or strongly disagreed, and 26% agreed or strongly agreed.
Academic researchers can ensure that they are intrinsically safe from the potential to harm participants on a moral level when adopting AI and neuromarketing strategies. – Of 54 responses, 43% agreed or strongly agreed, and 32% disagreed or strongly disagreed.
Marketers or AI algorithm developers can proactively limit ethical issues. – Out of 54 responses, 76% either agree or strongly agree, and 15% disagree or strongly disagree.
Are there any codes of conduct or other written agreements for ensuring ethical businesses, or are the employees, for example, trained to behave morally? – Out of 55 responses, 45% answered no, and 40% yes.
In response to questions regarding ethical issues and regulations:
Could ethical issues with negative impact arise from automated consumer targeting? – Of 53 responses, 60% answered sometimes, 38% don’t know, and 2% none.
The EU guidelines for ethical artificial intelligence and the GDPR usually require a higher level of compliance. Are there limitations to such strict guidelines? – Of 53 respondents, 28% answered sometimes, 64% didn’t know, and 8% none.
Do EU guidelines for ethical artificial intelligence and the GDPR limit marketers to offer better service levels and greater consumer personalization? – Out of 54 respondents, 78% answered don’t know, and 17% sometimes.
5.3. Semi-Structured interviews
During the semi-structured interviews with industry experts and scholars in Spain and the US, the benefits delivered by using AI/ML algorithms in neuromarketing were evident as most of the interviewees on both sides of the Atlantic underlined the ability of ML algorithms to manage massive amounts of unstructured data.
5.3.1. Ethical issues of AI/ML algorithms for segmentation and targeting
Most of the interviewees, mainly from Spain (83%), than the US (47%), expressed concerns about the hidden ethical issues presented by such algorithms, arguing that marketers and data scientists often overlook them. Most of them also expressed concerns, again mainly from Spain (83%) than the US (57%), with algorithms neuroethics including bias, invasion of privacy, nudging, and opacity, emphasizing that such algorithms all run counter to the ethical bounds established by the main theory employed in this study for ethical decision-making, rule utilitarianism. Such feedback finds support in the literature, as neuromarketing’s AI/ML algorithms for segmentation and targeting raise several ethical issues related to privacy, bias, autonomy, and opacity (Gandy, 2019; Wirtz et al., Citation2023). These concerns stem from the potential violation of human rights and can be analyzed through the lens of the utilitarian rule framework. These ethical risks and issues were evaluated using the utilitarian rule framework as they violate certain human rights. Furthermore, rule utilitarianism approaches the advantages provided to society holistically (Tolmeijer et al., Citation2021), in this case, the benefits generated by neuromarketing AI/ML algorithms to clients and businesses (Tsamados et al., Citation2021). The four concepts of privacy, bias, autonomy, and opacity breach 3-5 human rights, as outlined in , were also mentioned by 69% of the interviewees, indicating their prevalence.
Table 2. Charter of the Fundamental Rights of the EU’s violated by each ethical issue (Source: European Commission, Citation2016).
A significant finding of this study is that the GDPR hinders the benefits of AI/ML algorithms applied to digital and neuromarketing strategies. When used for marketing and neuromarketing purposes, the algorithms can profoundly impact consumers’ lives, which finds support in the literature (Magalhães, Citation2018; Kotras, Citation2020; Reuille-Dupont, Citation2023). These can sometimes be harmful, and the responsibility for this innovation should not be disregarded. Ultimately, the GDPR requires obtaining informed consent from individuals to collect and use their data. This may limit the amount and quality of data that can be used if proper consent is not obtained. In addition, companies must also provide transparency about how data is used, which may require clear explanations about the use of AI algorithms. summarizes all the interviewee’s responses in the US and Spain and highlights their perception of the GDPR’s advantages and downsides.
Some interviewees in Spain (33%) felt that the GDPR needed to be revised to defend human rights. They see it as an advantage in safeguarding individuals’ personal information since it establishes limits and restrictions on how it can be used, thus preventing its misuse or unauthorized use:
The GDPR could use some updates to make things better. They should work on ensuring people have a clear understanding of what’s going on with their data, like giving people a chance to make an informed choice about how it’s used. It’s all about giving individuals more power to take control of their personal information, which is a big deal when it comes to protecting human rights. (Interviewee ES2)
I think it [the GDPR] needs revisions to provide additional protection for vulnerable populations, including children and individuals with diminished capacity. It should have more strict regulations on the collection of data from children, minors, I mean, and measures to prevent exploitation or discrimination against vulnerable groups. (Interviewee ES3)
The GDPR is about making businesses get clear and explicit consumer consent before they can mess with their data. But here’s the thing: some peeps might not be too keen on sharing their info so that the consent rates can take a hit, and the data might end up incomplete. As a result, businesses need help getting inside the heads of consumers, which puts a damper on how they can fine-tune their marketing moves. (Interviewee ES03)
The problem with GDPR is that it enforces explicit data consent, impacting consent rates and data completeness and hindering our businesses from understanding and optimizing consumer marketing strategies. (Interviewee ES05)
Regarding regulations, the challenge is to look for an alternative, such as a technology, that allows us to access that data in an alternative way. (Interviewee ES4)
The GDPR makes it necessary for businesses to get clear and explicit consent from consumers before they can process their data. However, this condition could result in reduced consent rates or incomplete data since some consumers might hesitate to share their information. (Interviewee ES1)
The GDPR already affects us here [in the US]. It’s got some serious rules when it comes to how companies handle data. And let me tell you, those rules can really put a damper on businesses trying to gather all that vital data about consumers. They end up with this limitation that makes it pretty darn hard to get a good grip on what makes people tick. You know, understanding their likes, habits, and needs? Yeah, it’s a real tough nut to crack if we were to have something similar here. (Interviewee US4)
Implementing and maintaining compliance with stringent data protection regulations such as the GDPR can be expensive. We would need to invest in new technologies, hire data protection people, probably have to conduct regular audits, and make sure to provide staff training. These extra costs can reduce our profits and impact the company’s financial performance. (Interviewee US1)
Applying ML to neuromarketing can lead to the loss of nuance and bias in the results due to the inherent limitations of algorithms and the failure to detect uncollected aspects of human behavior or preferences. (Interviewee US4)
These algorithms can easily fail to capture uncollected aspects of consumer behavior, leading to incomplete or inaccurate assessments of preferences and decision-making. We easily see many of these examples in Tik-Tok and YouTube. (Interviewee US6)
Machine learning applied to neuromarketing can oversimplify complex human behaviors, causing a loss of nuance in the insights gained. (Interviewee ES2)
Algorithmic limitations in ML can introduce biases into neuromarketing findings, skewing the interpretation of consumer responses. (Interviewee ES1)
5.3.2. ML algorithms add value to businesses and consumers
Most US and Spain interviewees (10 out of 13) highlighted the importance of cost reduction in marketing, arguing that ML algorithms added value to businesses and consumers. This emphasis stems from the decreased reliance on human intervention, leading to a reduction in labor costs. Marketing automation, content creation, and similar strategies already play a significant role in tasks such as targeted advertisements, personalized email campaigns, content creation, and curation, all performed by machines with minimal human interaction (Levy, Citation2018; Haleem et al., Citation2022).
Six interviewees in the US believed that marketers face significant challenges in reducing the cost per acquisition while maintaining or increasing their businesses’ return on investment. One of them cited an example from Amazon:
Amazon uses an innovative application, and automated targeting, that utilizes consumer data and preferences to send samples of new products directly to consumers, enabling consumers to receive relevant product samples based on personal preferences and past purchases. (Interviewee US3)
If something like the GDPR in Europe were to be implemented here in the US, I am concerned that the costs associated with segmentation may rise significantly, and we won’t be able to leverage these algorithms and automated targeting strategies. (Interviewee US4)
I think any type of regulation so stringent like the GDPR in the US will impact profitability and neuromarketing implementations, especially considering the fact that expenses increase proportionally with the quantity of individual data to be evaluated and the number of regions that data need to be analyzed. Interviewee US6)
These neuromarketing algorithms not only makes it possible to offer products and services customized to each consumer but also to increase the efficiency and productivity of our company. (Interviewee ES03).
Algorithms allow us to analyze huge amounts of data and extract important data to support our digital marketing strategies and decision-making. (Interviewee ES05)
We rely on these algorithms to analyze our consumer’s behavior on our websites, blogs, and social media. (Interviewee US4).
My group has gained valuable insights regarding the preferences of our consumers and their product selection process. (Interviewee US6).
… the widespread adoption of AI may create an excessive dependence on the technology. (Interviewee ES4)
… it could generate vulnerabilities in the interpretation of data. (Interviewee ES5)
… the more protection there is for the consumer, the more difficult it will be for us as researchers to access consumers’ data. (Interviewee ES6)
What technology allows us is to analyze large amounts of data in less time and with less effort, so greatly refining the results we obtain in an investigation. (Interviewee ES2)
…the matter is to find a balance, therefore, obviously, the machine helps us and technology helps us to make sectors more efficient, but there must always be a human being auditing the process. (Interviewee ES4)
I worry about the increased use of consumer profiling. (Interviewee US6)
I am not sure about the ethical concerns, but they are important to be considered, especially regarding the extent to which consumer data should be utilized. (Interviewee US7)
As researchers, with our cognitive capacity, we provide context to the results of the investigations that derive from a machine from the application of an artificial intelligence algorithm or a mathematical result or machine learning… (Interviewee ES4)
Another argument expressed by 85% of interviewees in the US and Spain was regarding the critical benefit of neuromarketing and ML algorithms on market expansion, achieved through real-time automation, enabling scalability and optimal decision-making, thereby increasing a business’s competitiveness. The argument was that to develop effective marketing and advertising strategies, it is essential to meet consumers’ expectations and comprehend the factors influencing their purchasing decisions. These interviewees argued that the ever-changing nature of consumer interests and consumption behaviors necessitates using sophisticated tools and approaches that leverage advanced technologies. Neuromarketing can be perceived as one such approach by measuring consumers’ physiological and neural signals and studying their cognitive and affective responses to marketing stimuli, as deep insights can be gained into consumers’ motivations, preferences, and decision-making processes (Lee & Yang, Citation2021; Falk & Weber, Citation2022; Lee & Chamberlain, Citation2022; Park & Yoon, Citation2022; Tirandazi et al., Citation2022).
5.3.3. The issue of privacy
Collecting and analyzing consumer information enables algorithms to make better assumptions about individual preferences, leading to the provision of more personalized services by advertisers (Zeng et al., Citation2021). Neuromarketing techniques that employ AI/ML algorithms often rely on collecting and analyzing personal data, including sensitive information about individuals’ preferences, emotions, and behavior (Clark, Citation2020). Interviewees in the US (57%) and Spain (100%) expressed concerns:
The utilization of such data raises significant privacy concerns. (Interviewee ES3)
I think that using consumer data may result in the infringement of individuals’ right to control their personal information. (Interviewee US5)
Data is an essential component for the existence and functionality of AI/ML systems. These algorithms rely on input data to make predictions and require sufficient data (big data) to perform effectively. (Interviewee US3)
Most of the interviewees in the US (86%) highly value the personalized services they receive in e-commerce. However, they all expressed concerns regarding their data privacy and AI-driven personalization and profiling, which the literature supports (Zeng et al., Citation2021; Häglund & Björklund, Citation2022). Marketers aiming to enhance their services and maintain competitiveness heavily rely on AI-driven strategies, ML algorithms, and data analytics, which can intrude on privacy (Kotras, Citation2020). While data collection can enhance personalized recommendations, it also raises concerns about exposing private information, such as an individual’s sexual, religious, or political preferences. Researchers have demonstrated how predictive analytics, using Facebook likes as a single piece of information, can construct client profiles with a 60% accuracy, predicting gender, family status, sexual and political orientation, and addictions (Solove, Citation2023).
In Spain, interviewees emphasized the existence of codes of conduct and written agreements to ensure ethical business and protect consumer privacy. However, in the business field, they indicated that they know the ethics to be followed but do not usually review the documents:
…the neuroscience and neuromarketing code of ethics, I don’t know it, I don’t know what it’s called. But in the work environments, I am calm because we have market research codes that in general are quite modern and quite ethical… (Interviewee ES6)
…I don’t know these codes you are talking about, but I would say that the ethical approach is kind of present and internalized in our lives; it is something we have internalized. I have been dedicated to Market Research Business Intelligence for 40 years, and it is something that every time we do research is part of our DNA as researchers, to take care of people, to take care of brands, to take care of clients. Taking care of the collaborators. But if you tell me what specific code or so, I don’t know… (Interviewee ES5).
…Because the fact that you have an ethics committee, for example, does not mean that your research is ethical, that research has obtained the approval of the Ethics Committee does not mean that the research itself is ethical, because simply to obtain that approval sometimes it is enough to know how to complete an application, and it does not mean that the research itself will be ethical… (Interviewee ES4)
There is a need to protect the physical and mental integrity of the participants and informed consent. (Interviewee ES3)
5.3.4. The issue of bias
AI/ML algorithms used in neuromarketing can inherit biases in the data on which they are trained. Biases can manifest in various ways, such as demographic, cultural, or stimuli selection (Greenwald et al., Citation2022; Tay et al., Citation2022). As pointed out by interviewees from the US and Spain:
They [bias] can come up due to insufficient time, knowledge, or technical problems. (Interviewee US4)
I worry about biased algorithms because they can lead to unfair segmentation and targeting. (Interviewee ES1)
Bias in these algorithms can perpetuate discriminatory practices and reinforce societal biases. (Interviewee ES4)
5.3.5. The issue of autonomy
Most of the US and Spaniard interviewees believed neuromarketing techniques that leverage AI/ML algorithms have the potential to influence individuals’ decision-making processes and behaviors by exploiting their subconscious responses, as exemplified below:
Neuromarketing techniques that uses algorithms can surely subtly manipulate subconscious responses of consumers, subtly swaying their decision-making processes. (Interviewee US3)
I am certain that by tapping into deep subconscious cues, algorithms in neuromarketing can nudge consumer behaviors without their conscious awareness. (Interviewee ES6)
5.3.6. The issue of opacity
The complex nature of AI/ML algorithms used in neuromarketing poses challenges regarding transparency and interpretability. Most interviewees expressed concerns regarding algorithms operating in ‘black boxes’, meaning their decision-making processes and underlying logic may be complex to comprehend or explain. Such a lack of transparency could lead to a lack of accountability and prevent individuals from understanding how their data is used, and their decisions are influenced (Saheb et al., Citation2022; Blasco-Arcas et al., Citation2022).
6. Discussion, conclusions, recommendations, limitations, and further research
This study focused on concerns related to neuromarketing algorithms, consumer privacy, and ethical considerations. It adopted the theory of rule utilitarianism as a framework for ethical decision-making, which focuses on preserving society’s human rights while delivering them the highest value (Forrest, Citation2021; Karakash, 2021; Kumar & Choudhury, Citation2022; Dwivedi et al., Citation2023). Some of the main ethical challenges of algorithmic opacity, privacy invasion, bias, and nudging in digital and neuromarketing were identified, and their effect on human rights was observed. The findings of this study demonstrate how the European GDPR, and potentially the American CCPA, can be used as a tool for ethical AI/ML algorithms in digital and neuromarketing applications. Aside from constraining AI/ML algorithms, the data protection regulation imposes some limitations on digital marketing, advertisers, and the general public. The benefits of the AI/ML algorithms were also analyzed, and as a result, a set of recommendations are offered to address consumer privacy threats, human rights, and ethical concerns. These recommendations were broken down by separating responsibilities among stakeholder groups, which allows for a better possibility of tracing ethical concerns back to their source. They aim to preserve the benefits of implementing AI/ML algorithms in digital and neuromarketing applications while safeguarding consumers’ privacy and human rights and preventing ethical concerns from emerging.
6.1. Discussion
The novel contribution of this research lies in its systematic investigation of neuroethics, and privacy concerns related to the application of AI/ML algorithms in neuromarketing, with a comparative analysis across the U.S. and Spanish contexts. Unlike previous publications, which may have focused separately on neuromarketing technologies, AI ethics, or consumer privacy, this research integrates these domains to offer a holistic view of the ethical implications within international frameworks, particularly contrasting the impacts of different data protection regulations. The study stands out by blending systematic literature reviews, surveys, and interviews with experts to propose policy recommendations, thereby filling a gap in the literature on the interdisciplinary evaluation of neuroethics in AI-driven marketing. While it builds upon existing studies’ methodologies, it differentiates itself by connecting the technical aspects of neuromarketing with the ethical discourse, bridging academic and practical perspectives in a novel manner.
The bibliometric analysis conducted in this study revealed that the junction of two dimensions characterizes neuromarketing’s AI/ML algorithms applications: 1. the fast development of neurotechnologies, AI/ML algorithms, and more advanced ML algorithms such as ANN, SVM, and LDA for analyzing consumer behavior; and 2. neuromarketing ethical concerns, such as consumer’s manipulation in segmentation, preferences, and decision-making, consumer data privacy protection, and trust. However, the field is currently characterized by a scarcity of studies in these two areas. Thus, in the quest to build and strengthen the neuromarketing practice and the use of neurotechnologies, the research community, academics, and practitioners are encouraged to make increasing efforts to combine these two complementary strands of research.
The study also revealed that the production and dissemination of scientific contributions in the field are heavily dominated by select ethnical population samples, geographical regions, and outlets, which could substantially influence future research in neuromarketing. However, this analysis also reveals the scope for conducting future studies in the contexts of including population samples with greater diversity (not primarily whites), more in line with diversity, equity, and inclusion (DEI) principles, in emerging and frontier economies (as opposed to only advanced economies) and focusing on the ‘polemic’ topics such as developing AI/ML algorithms for neuromarketing applications with deontological principles in mind, exploring the role of culture as the determinant of organizational behavior, and suggesting the ways to overcome the critical challenges in the field of neuroethics.
Because ethical concerns endanger human rights, there is a need to protect consumers from unintended or intended ethical exploits by securing the ethical use and operation of AI/ML algorithms. The introduction of the GDPR in 2018 set a high standard of privacy protection for consumers within EU member states. The data privacy landscape in the US has changed considerably in recent years, and data protection rules are now aligned increasingly with a European approach, although some significant differences remain. The closest set of consumer protection regulations most comparable to the GDPR is the California Consumer Privacy Act (CCPA), applying only to consumers who are California residents. CCPA became effective in January 2020, but the impending California Privacy Rights Act (CPRA) amends the privacy legislation to expand opt-out rights and introduce other changes that bring it in even closer alignment with GDPR. CPRA became effective in January 2023, with Virginia and Colorado being the only two other US states signed a comprehensive data privacy law. The GDPR provides guidance and suggestions on protecting consumer data and prohibits certain practices such as gender and racial discrimination and gathering dissident data. Although EU law is the most robust regulator, it has some drawbacks, one of which is its late occurrence, which means it does not consider the most recent breakthroughs, such as big data and artificial intelligence. During the interviews in the US and Spain, neuro and digital marketing specialists emphasized the limitations created by GDPR on advertising, like stifling creativity and a high cost of compliance; none of them were aware of CPRA.
The trend of providing heightened protection for sensitive data is gaining popularity in consumer privacy protection worldwide (Gstrein & Beaulieu, Citation2022; Quach et al., Citation2022). To address the severity of situations, many privacy laws establish special categories known as ‘sensitive data’ that receive additional safeguards (Solove, Citation2023; Van Bekkum & Borgesius, Citation2023). These laws differentiate between regular personal and sensitive data by offering two levels of protection. Instead of solely considering the nature of personal data, consumer privacy protection should focus on its use, potential harm, and associated risks. This perspective extends beyond sensitive data provisions and should guide the overall framework of consumer privacy protection (Kaufmann et al., Citation2022).
Consumer privacy protection is triggered by activities involving personal data, typically defined as data related to an identified or identifiable person. Once privacy protection is triggered, it necessitates a set of protections encompassing individual rights and organizational duties. Recognizing that not all privacy situations are equal, many privacy laws acknowledge special categories of personal data called ‘sensitive data’ that require heightened protection (Solove, Citation2023; Van Bekkum & Borgesius, Citation2023). This approach allows for tailored protection based on different data types’ severity, risk, and harm (Trakman et al., Citation2019; Finck & Pallas, Citation2020). All privacy laws define their scope of personal data, as they cannot encompass all data. The most common definition of ‘personal data’ is from the GDPR, which defines it as ‘any information relating to an identified or identifiable natural person’. The proposed American Data Privacy and Protection Act (ADPPA) proposes similar definitions of identifiability, establishing when data is linked to an individual (Kaufmann et al., Citation2022). For instance, an IP address can be connected to individuals and is considered personal data under privacy laws that include identifiability in their definitions. Similarly, several privacy laws in the US define personal information as data identifying a person. Even if data cannot be directly linked to a specific individual, it can still be considered personal data if combined with other data to identify a person.
The literature regards sensitive data as a crucial aspect of modern data protection (Ghelani et al., Citation2022; Quach et al., Citation2022). Numerous privacy laws worldwide provide heightened protection for sensitive data. However, the US has historically not recognized sensitive data in its privacy laws (Peloquin et al., Citation2020; Hill, Citation2022). Many countries’ privacy laws define specific types or categories of data as ‘special categories of data’ or ‘sensitive data’, warranting more excellent protection. These laws often establish two levels of security, one for regular personal data and another for sensitive data. In contrast, some laws do not adopt this balanced approach.
6.2. Managerial implications
The practical implications of this research underscore the necessity for marketing practitioners to implement AI-driven neuromarketing techniques responsibly, ensuring consumer privacy and consent are at the forefront of strategic considerations. Companies should establish transparent data use policies and engage in continuous dialogue with stakeholders about the ethical deployment of neuromarketing tools. Practical guidelines should be formulated for practitioners to navigate the ethical complexities of consumer data analysis and application, fostering an environment where consumer trust is as central to corporate strategy as data-driven insights. Additionally, marketing teams must stay informed about technological advancements and regulatory changes, adapting their strategies to maintain ethical integrity in a dynamic digital landscape. Developing continuous education programs and ethical compliance audits could prove beneficial in aligning neuromarketing practices with the evolving expectations of consumers and regulatory bodies.
The managerial implications also emphasize the need for a strategic and ethical approach when incorporating AI/ML algorithms into neuromarketing practices. Managers must create thorough policies in advance to ensure that consumer data is used in a way that respects privacy and builds trust. This involves creating straightforward methods for collecting data transparently, obtaining informed consent, and providing easily understandable options to opt-out, all in accordance with laws and increasing consumer demands for responsible data management.
6.2.1. Training
Training and development are crucial; therefore, managers should focus on educational programs that improve the staff’s knowledge of neuroethics, data privacy laws, and the social implications of their work. The education needs to reach all areas of the organization, not just the marketing and neuromarketing department, to create a culture of ethical awareness. An effective training and development program for ethical AI/ML utilization in neuromarketing should encompass a comprehensive educational strategy. It may include the provision of ethics and compliance training that addresses general ethical principles, data privacy laws, and compliance with regulations such as the GDPR and CCPA. An introduction to neuroethics should be incorporated, establishing a foundation for understanding the ethical considerations specific to neurotechnological applications in marketing.
The program should further incorporate technical literacy in AI and ML, ensuring that marketing personnel comprehend the mechanics of these technologies, data processing methodologies, and the significance of data neutrality. Interactive workshops are recommended to apply theoretical knowledge to practical situations, enhancing identifying and resolving ethical dilemmas.
Developing soft skills is crucial, emphasizing critical thinking, empathetic engagement, and a consumer-focused approach to decision-making. Continual professional enrichment through participation in relevant industry events is advocated to maintain currency with evolving ethical discussions in AI and marketing. Facilitating cross-functional collaboration through workshops can foster a multidisciplinary perspective in ethical deliberation. Additionally, leadership training in ethical conduct and change management should be a priority, cultivating the ability to steer organizational culture toward ethical AI/ML applications.
Lastly, formal certification programs in data privacy and ethical AI utilization are recommended to underscore the commitment to expertise in these critical areas and enhance the ethical acumen of the workforce involved in neuromarketing initiatives.
6.2.2. Promotion of ethical standards
Companies are also urged to take the lead by participating in industry discussions to promote ethical standards and help shape future regulations. Managers should not avoid these conversations but instead welcome them as a chance to make an impact and establish ethical standards that have a wide-reaching impact on the industry. This could look like reviewing existing marketing strategies for their ethical impact, updating KPIs to incorporate ethical concerns, and establishing oversight committees to monitor the responsible development and execution of marketing campaigns.
To promote ethical standards in neuromarketing, especially when incorporating AI/ML algorithms, a comprehensive framework that includes internal policies and collaboration with the industry is recommended, which may include:
Creating an extensive ethical code: Companies must establish and implement a thorough set of ethics guidelines that tackle the particular issues raised by neuromarketing and AI/ML technologies. This code should incorporate guidelines for protecting data privacy, obtaining consumer consent, promoting transparency, and ensuring fairness in algorithmic decision-making.
Ethics training programs should be held regularly for all staff members to ensure that ethical codes, data protection laws, and real-life situations are prioritized in marketing decisions.
Conduct ethical impact assessments before releasing innovative marketing campaigns or algorithms to predict and address possible ethical risks.
Developing clear and accessible privacy statements, consent forms, and communication channels is needed to inform consumers about data usage and rights and promote trust and transparency in consumer communication.
Form cross-disciplinary ethics committees comprising individuals from different departments, such as legal, technical, and consumer advocacy groups, to monitor the ethical execution of marketing tactics.
Conduct ethical audits consistently and promote open reporting of marketing practices to internal stakeholders and the public.
Collaborate with other organizations, regulatory bodies, and ethical think tanks to raise industry standards and shape ethical marketing practices through partnerships and dialogues.
Create mechanisms for collecting consumer feedback on privacy and ethics, utilizing this information to improve marketing strategies on an ongoing basis.
Implement incentives for teams and individuals who uphold and promote ethical practices in the company.
Although neuromarketing offers excellent potential for consumer interaction and business development, as highlighted in this study, it also presents distinct obstacles. Managers need to confront these challenges directly and understand that the future success of AI/ML algorithms in marketing relies on a dedication to ethical practices that respect the dignity and rights of individual consumers. This proactive approach is focused on reducing risks and serves as a competitive edge in a business environment that places greater importance on corporate social responsibility.
6.3. Conclusions
This study has integrated a mix of methodological approaches, including a structured literature review, bibliometric analysis, and empirical data collection through surveys and interviews. It also delves into the implications of differing regulatory environments, such as GDPR in the EU and the comparative lack of federal regulation in the US, on neuromarketing’s application and ethical considerations. Many discussions on using neuromarketing AI/ML algorithms, expressed by interviewees in this study and available in the literature, are set around special categories of personal data classified as ‘sensitive data’ that receive heightened protections. Consumer privacy protection from neuromarketing algorithms must focus on use, harm, and risk rather than on the nature of personal data. Sensitive data allows for treating these situations with more protection, singling out specific categories of personal data for extra protection. Sensitive data is critical to the GDPR and comprehensive privacy laws worldwide. Algorithms perform significantly better than humans in making inferences about sensitive data.
Most regulations with heightened protections for sensitive data define it in specific categories. The GDPR, for example, identifies special categories of personal data that include racial or ethnic origin, political opinions, religious or philosophical beliefs, trade union memberships, health, sex life, genetic data, and biometric data. Similarly, the state consumer privacy laws in California, Connecticut, Colorado, Virginia, and Utah (CCPA & CPRA) recognize racial or ethnic origin, religious beliefs, mental or physical health diagnosis, sexual orientation, genetic or biometric data, and specific identification numbers as sensitive data (Stacey, 2022; Schmit, Citation2023; Solove, Citation2023).
6.4. Recommendations
The following are recommendations for developing, using, and managing AI/ML applications in neuromarketing. They intend to rebalance the neuromarketing and neuroethics strategies and promote future studies that can integrate the two strands of research. These propositions offer the opportunity to advance the discipline’s empirical assessment and provide neuromarketing and neuroethics insights to the practitioner community embracing AI/ML.
To protect consumers from unintended or intended consumer harm, such as invasion of privacy, surveillance, bias, and discrimination, governance organizations, and standard committees must intercede, as they are primarily responsible for regulating and enforcing standards or rules (Waldman, Citation2019; Hacker, Citation2022). ISO and IEEE are the two most common standards within the EU (Gaborović et al., Citation2022). Classifying the targeted individuals (consumers) or the broader public as stakeholders is critical, as they are the most affected party by the AI/ML algorithms’ unethical and often immoral decision-making. Hence, developing and holding stakeholders accountable is imperative.
6.4.1. Developing and Holding stakeholders accountable
Several marketing professionals and scholars involved with using AI/ML algorithms for marketing and neuromarketing applications for segmentation and targeting were identified and interviewed for this study. When an AI/ML algorithm is developed, one or a group of system developers are created. These individuals may include AI engineers, prominent data engineers, computer and information research scientists, data analysts, data engineers, data scientists, research scientists/applied research scientists, ML engineers, IT professionals, and hardware technicians. However, they may also be organizational units such as universities or businesses developing advanced technology. These stakeholders usually build the AI/ML algorithm’s functional side, which is then integrated into businesses or used in marketing and neuromarketing applications and other areas and operations departments.
There is also another group of stakeholders, technologically advanced companies, uniting the developers and users of the AI/ML algorithms. Some of the leading companies include Appier, GumGum, IBM, Heuritech, NetBase, Quantcast, Salesforce, Dstillery, Affectiva, Cognitiv, Apple, Amazon, and Google, which have often developers and users working within the same company. However, as ML algorithms necessitate a considerable amount of individual data, some companies may use the services of big data providers if they aim to generate precise targeting results or want to rely on certified providers.
The final group of stakeholders should be composed of ethics organizations or ethics committees. These experts should be ready to advise marketing organizations on what is ethical and elucidate the uncertainty around the social implications of AI/ML algorithms technology, thus reducing the moral gap created by their use (Winfield et al., Citation2019; Thomas et al., Citation2022; Albahri et al., Citation2023; Dwivedi et al., Citation2023).
6.4.2. Developing guidelines for ethical AI/ML algorithms-driven neuromarketing
The absence of explainability in AI/ML-based black box systems/models significantly hinders the widespread adoption of AI/ML in neuromarketing despite its numerous advantages in various real-world applications. Although there are several well-known methods and approaches in Explainable Artificial Intelligence (XAI) that aim to provide explanations of decisions that are easily understandable to humans, each approach has its strengths and weaknesses. There are still numerous challenges that need to be addressed.
6.4.3. Holding developers of neuromarketing applications ethically responsible
Developing AI/ML algorithms is the first step toward automated neuromarketing. At this level, creating an explainable and transparent set of algorithms is vital, reducing their chances of acting as a black box in later phases (Shin & Park, Citation2019; Islam et al., Citation2021). Developers must be able to grasp the algorithm’s inner workings, finding ways to predict consumer targeting, segmentation, preferences, and decision-making using supervised ML algorithms (e.g. Decision tree, Extra tree, Adaptive boost), Extreme gradient boost (XGBoost), Light gradient boosting method (LGBM), and Laplacian Kernel Ridge Regression (LKRR). Alternatively, SHapley Additive exPlainations (SHAP), a novel black-box interpretation approach, can elucidate the predictions (Ekanayake et al., Citation2022).
Blattner et al. (Citation2021) and Ekanayake et al. (Citation2022) highlight an issue concerning the characterization of optimal oversight for algorithms. In this scenario, an agent designs a complex prediction function while the principal has limited access to information about the prediction function. Imposing restrictions on agents, limiting them to transparent prediction functions, proves inefficient when the bias resulting from misalignment between the principal’s and agent’s preferences is slight compared to the uncertainty surrounding the actual state of the world (Blattner et al., Citation2021).
Neuromarketing algorithmic audits have the potential to enhance consumer welfare; however, the effectiveness of these audits relies heavily on the design of the tools used. Tools that primarily aim to minimize overall information loss, a common focus in many post-hoc explainer tools, tend to be inefficient as they mainly explain the average behavior of the prediction function, overlooking sources of misprediction that are crucial for consumer welfare. Instead, targeted tools that address the root causes of incentive misalignment, such as excess false positives or racial disparities, can provide optimal solutions (Blattner et al., Citation2021). Knowing how these algorithms work and understanding the dataset reduces the opacity of algorithms and makes users more trusting of the final output (Cheng et al., Citation2019; Lee, Citation2020; Ali et al., Citation2023).
Although algorithmic transparency is good, information transparency is unethical (Möhlmann, Citation2021). According to research (Kapitonova et al., Citation2022; Koritnik & Merc, Citation2022; Semantha et al., Citation2023), an approach known as privacy-by-design has proven effective. Developers must build privacy into algorithms as a default to decrease infringement cases and increase informational transparency (Galli, Citation2022; Reuter et al., Citation2022; Matulionyte, Citation2023). Regarding e-commerce platforms, privacy by design means controlling the parties who access data and excluding quasi-identifiers like gender and postcode from the algorithm. Other methods for reducing neuromarketing algorithms’ ethical risks include working in diverse teams and establishing an honor code among software R&D engineers and departments. Education in AI ethics may help reduce the transfer of human biases onto algorithms and develop an ethical AI system (Fabi & Hagendorff, Citation2022; Wirtz et al., Citation2023). Researchers conclude that imbalanced data primarily generate biased predictions but that engineer demographics also impact (Cowgill et al., Citation2020; Harjule et al., Citation2023; Jones, Citation2023). As a result, having gender and racial diversity in development teams may contribute to an ethical AI system (Goncalves et al., Citation2022; Srivastava & Bag, Citation2023).
6.4.4. Holding deployers of AI/ML algorithms ethically responsible
Deployers, or users, of AI/ML algorithms are ultimately the ones using these algorithms for segmentation and targeting, as well as predicting consumer preferences and decision-making. In the following section, a few policies for digital and neuromarketers are recommended. These policies also apply to business analysts, advertisers, and algorithm users. Explainability must be extended from the development stage to the platform clients. For instance, a movie-streaming platform such as Netflix should be able to explain why different series are being recommended to their consumers (i.e. genre, actors, and plots may be similar to ones from previously watched series), which, in turn, leads to more personalized service while justifying the recommendations. As for personal data, the law must still be obeyed; thus, data anonymization and working with relevant and recent data are required (EC GDPR, 2016), as required by the GDPR and the EU Charter of Fundamental Human Rights, where people must be informed about the collected data and allowed to object to it.
Most interviewees argued that restricting sensitive consumer data, such as gender, ethnicity, and religion, may be more beneficial than merely anonymizing it. Digital and neuromarketers who were interviewed shared that they could target the relevant consumer categories without using sensitive data, such as creating a behavior-based consumer profile. Several researchers (Scanagatta, Citation2021; Ge et al., Citation2022; Nam, Citation2022) depict a model based on clicks, basket insertions, and interest fields that do not rely on sensitive consumer data but show effectiveness.
Assuming digital and neuromarketers were unsure whether the personal data was being collected in an ethically safe way, they could build data within the company and use only first-party tracking or analytical cookies. Blocking these may result in a poor user experience and disrupt the website’s operation (Koop et al., Citation2020; Fouquet et al., Citation2023; Hanoglu, Citation2023). Blocking third-party cookies, on the other hand, decreases the likelihood of hijacking but seems to have no substantial influence on e-commerce. Google Chrome, for instance, has not been working with third-party cookies since 2018 but still tops the web browser market list (Dao & Fukuda, Citation2021). Thus, though not entirely safe, first-party cookies are preferable.
Another recommendation is to conduct regular inside-organization meetings with other departments and ethicists. Consider large multinational companies with a heavy digital presence and a varied multicultural workforce, such as Amazon, Google, Netflix, and Salesforce. It is difficult for these businesses to ensure that all their staff adheres to ethical ideals (Baker-Brunnbauer, Citation2021; Tarafdar et al., Citation2020; Lanz et al., Citation2024). As a result, a code of conduct, supported by at least quarterly meetings, may limit using unethical AI/ML. Further, it is crucial to convey the current social challenges concerning society. Due to technological breakthroughs and media influence, people may value their well-being and privacy more than ever. Knowing that most consumers regard the invasion of privacy as violating their human rights is critical for selecting the best marketing strategy. Hence, another policy recommendation should be to create a checklist with attributes that consumers may consider unethical. A starting point should be a checklist proposed by the European Commission (Citation2019), which can be adapted to different industries. Other methods include analyzing client behavior on blogs, considering complaints, and polling consumers or getting feedback for specific campaigns.
Additionally, it is crucial to raise awareness of current social challenges within society. Technological advancements and media influence have made individuals more aware of privacy threats. Recognizing that consumers perceive privacy invasion as violating their human rights is essential for devising effective marketing strategies. Therefore, creating a checklist of attributes that consumers may consider unethical is recommended. The European Commission’s (2019) proposed checklist can serve as a starting point, which can be adjusted to suit different industries. Other methods include analyzing consumer behavior on blogs, addressing complaints, and conducting polls or surveys to gather feedback on specific campaigns.
6.4.5. Policy implications
The results of this research carry important policy implications, underscoring the necessity for thorough laws that cover the ethical application of AI/ML in neuromarketing. Policymakers must think about creating global guidelines safeguarding consumer privacy, consent, and autonomy while permitting the advantageous utilization of neuromarketing advancements. Policy frameworks must be flexible to stay current with the fast evolution of AI technologies and their use in neuromarketing. It is essential to cooperate among regulatory agencies, industry professionals, consumer champions, and academic scholars to uphold the interests of all parties and create fair and efficient regulations. Furthermore, these guidelines should create an atmosphere that promotes consumer confidence and corporate transparency, ultimately guaranteeing that neuromarketing techniques benefit consumer well-being and market equity.
6.4.5.1. Recommendations for big data providers
Several digital and neuromarketing firms rely on big data providers to run their businesses. Hence, although not entirely part of the actual AI/ML algorithm development and use, big data providers should also be considered a stakeholder group due to the value they deliver to marketing firms. Ensuring privacy-preserving data collection before transferring the data to the digital and neuromarketers could reduce unethical work. For instance, IBM, a big data-providing company, has already implemented these privacy-preserving data analysis techniques, known as differential privacy (Goel et al., Citation2021; George & George, Citation2022). The corporation relies mainly on k-anonymity-based algorithms to ensure data sanitization (El Ouazzani & El Bakkali, Citation2020; Gadde et al., Citation2023). Once used by marketing and advertising firms, the anonymized data cannot be linked back to the individual and is thus not intrusive to privacy. Additionally, bias may be decreased as the data contains no sensitive consumer information, such as gender, race, or nationality.
6.4.6. Policy implications for governmental institutions
To ensure the protection of human rights in algorithmic decision-making, governmental institutions need to establish a robust framework consisting of norms and standards for the development and application of AI across various domains. Such a framework serves as a strong foundation for promoting responsible practices. Furthermore, regulatory bodies should actively address issues such as discrimination to prevent their recurrence in the future. One practical approach to promoting ethical and standardized AI/ML algorithms is through certification, which can help reduce information asymmetries (Cihon et al., Citation2021; Lucaj et al., Citation2023). For instance, IBM has obtained ISO certificates for information security in cloud services (ISO27017), privacy information management systems, and information security management systems. These certifications guarantee the security of IBM’s services to its partners, consumers, clients, and the general public across 140 countries. Standardization and governance organizations should ensure that all e-commerce platforms and data providers adhere to certification standards. In cases of uncertainties, evaluating platforms’ compliance can be done using the GDPR checklist, which provides a comprehensive framework for assessing compliance with data protection regulations.
6.4.7. Implications for consumers and broader public
Engaging in e-commerce and utilizing internet services often involves making trade-offs. The general public must be aware of these services’ ethical boundaries. For instance, while some individuals may not perceive gender or age as discriminatory, educating a broader audience about how consent for sharing information on social media platforms such as Facebook and Instagram can contribute to consumer profiling is crucial.
To protect user privacy, the GDPR mandates that websites have privacy policies and allow visitors to opt in or out. However, studies have shown that many consumers reflexively click ‘I have read the terms and conditions’ or ‘I agree’ without thoroughly reviewing the privacy policy (Karl & Tao, Citation2023; Neal et al., Citation2023). Individuals must recognize the importance of reading privacy rules before disclosing personal information.
On the other hand, specific services, such as meal or grocery delivery, often require access to the consumer’s location. In these cases, clients may face a trade-off between maintaining their privacy and receiving a higher level of service. Individuals must decide what they value more: their privacy or the quality of service they receive from being shown the nearest restaurants. Ultimately, individuals must be conscious of the ethical considerations of using e-commerce, especially when enhanced with augmented reality, webcams, and interactive real-time web services, make informed decisions about sharing personal information, and weigh the trade-offs between privacy and service quality.
6.5. Limitations
Despite the best efforts to seek all available scientific text on the topic of AI/ML algorithms applied to neuromarketing and its neuroethics implications, due to the novelty of the field and the resultant lack of epistemological differences, the data set used for the bibliometric analysis may only partially represent some of the related studies published in the field in the past five years. Moreover, since stringent scientific standards within the research in neuromarketing and neuroethics are yet to emerge, utilizing popular concepts and author keywords as an eliminatory criterion of the research process remains another limitation that future studies must address. Given the limited number of identified works and implementation of select inclusion and exclusion criteria based on a preliminary understanding of the field, the findings represent early attempts to understand the scope and the evolving nature of the field. Hence, to continue to provide an up-to-date picture of the field, neuromarketing researchers are encouraged to repeat this study at regular intervals to reveal the changing trends in the field. Future researchers must also collect data from multiple databases and continue to conduct backward and forward snowballing to develop an exhaustive dataset to facilitate comparing current and future field studies.
The findings of the semi-structured interviews cannot be generalized, as the population sample for the US and Spain were very small. The interviewees’ statements and opinions shed some light on the issues, supporting many findings in the literature. However, it is difficult to predict how applications of AI/ML for neuromarketing will evolve in the upcoming years and what trends will emerge in its industry. Although the literature examined for this study is primarily from 2019 onwards, it may need to be updated due to the ever-changing nature of AI/ML and its subsets. Furthermore, the primary analyzed legislative regulation, the GDPR, is primarily enforced in the EU region. At the same time, the American CCPA, unknown to all interviewees in the US and mainly unfamiliar to most consumers, covers only California and two other states, Virginia and Colorado, which have opted in. Both seem subject to changes.
Another area for improvement of this study is its inability to demonstrate the impact of applying the given guidelines with performance indicators. Finally, the stated ethical difficulties and associated obstacles may become irrelevant over time due to emerging and more responsible AI/ML algorithms’ development approaches, such as XAI, Decision tree, Extra tree, Adaptive boost, XGBoost, LGBM, LKRR, and the novel black-box interpretation approach, SHAP, capable of elucidating predictions (Ekanayake et al., Citation2022). These tools attempt to remove unethical features and protect consumers’ privacy at the early development stage of AI/ML algorithms.
6.6. Further research
There is a significant reason for concerning the prospect of consumer neuromarketing. With the development of neurotechnologies and advanced AI/ML algorithm strategies, there will be a substantial evolvement in neuromarketing that adds value to consumers and brands but also challenges neuroethics principles, consumer rights, and data privacy. Identifying the most suitable neuromarketing technology will require further research, as it relies on several distinctive factors. Neurotechnology selection will remain a vital component of process and product innovation strategies. Brands, mainly digital, will demand technology selection choices to enable the launching of a brand to keep up with the ever-evolving digital markets; therefore, further research on digital brands will be able to improve their managerial goals.
In the rapidly evolving field of neuromarketing, safeguarding consumer autonomy and trust is paramount. Future research should prioritize the development of strategies that bolster consumer control over personal data and enhance transparency in using neuromarketing techniques. Investigating the interplay between consumer autonomy, informed consent, and trust in brand relationships can provide deeper insights into ethical marketing practices. Such research is crucial for developing robust policies protecting consumers from covert data collection and manipulation and fostering an ethical marketing environment where consumer trust is valued and integral to business success. Empirical studies focusing on the efficacy of consumer education programs and the impact of transparent data usage on consumer trust will be instrumental in guiding policy and industry practice, ensuring that neuromarketing advances do not come at the expense of consumer rights and confidence (Degtiar & Šimulynas, Citation2022).
The framework adopted in this investigation might not be suitable in some countries, or even the US or Spain, due to the degree of exposure, the difference in the culture of neuromarketing technology use, and social and economic viability. Further research is needed to draw on and shape the expanded framework of these factors. The findings stemming from the analysis were based on academicians’ and practitioners’ perspectives. This study collected data from different samples in the US and Spain, for instance, organizations that could have been interested in exploiting neuromarketing and neuroethics research, especially as it was connected to adopting AI/ML algorithms for consumer neuromarketing strategies.
Since a substantial portion of this study was qualitative, the research design, rigor in data collection, management of the large amount of raw data involved, analysis, and credibility of the findings may require further validation. This approach may have led to unanticipated bias in data analysis, mainly since datasets from two countries, the US and Spain, were analyzed. Therefore, this study provides a biased understanding of the options and insights/information about the factors/issues shaping the challenges and limitations of neuromarketing AI/ML algorithm implementations. Hence, further research based on case studies and mixed methods should be undertaken to validate this study’s outcomes. Other research instruments and tools, such as neuroimaging and physiological tools, alongside traditional methods in other studies, will be used to test, confirm, and validate the outcomes of this study using a larger sample (population).
Future research should prioritize exploring emerging ethical challenges and developing robust frameworks to govern the application of neuromarketing strategies as AI technologies advance. It is imperative to extend the discourse beyond the current regulatory environments, such as the GDPR, to establish a cohesive global standard that accommodates the rapid technological evolution and its implications for consumer privacy and autonomy. Further, studies should investigate the nuanced impacts of neuromarketing on consumer trust, advocating for practices that bolster transparent and consumer-centric marketing approaches. This would aid in aligning the technological progression in neuromarketing with the foundational principles of consumer rights and ethical marketing, fostering an environment of trust and mutual benefit between businesses and consumers. Additionally, interdisciplinary research is called for to integrate insights from technology developers, policymakers, and marketing professionals, ensuring that the ethical guidelines devised are technologically informed and pragmatically applicable within the diverse global market landscape.
Considering the global impact of neuromarketing and AI, addressing ethical practices within an international context is essential. Future research should also expand the investigation of neuromarketing ethics to encompass a broader array of cultural and legal frameworks, acknowledging the diversity in consumer values and regulatory landscapes. A global perspective on neuromarketing ethics calls for harmonizing standards while respecting regional differences, promoting cross-cultural dialogues, and encouraging multinational collaborations. This approach is crucial in developing ethical guidelines sensitive to global market dynamics and the varying degrees of consumer protection laws. Such research would contribute to a more inclusive understanding of international consumer rights and ethical marketing practices, supporting creating policies that protect consumers and facilitate fair and competitive markets worldwide.
Considering fast technological advancements, there is a growing need to consistently review and modernize ethical guidelines in neuromarketing to align with innovation. Future research should examine how AI, machine learning, and data analytics progress impact consumer privacy and decision-making in neuromarketing. Innovative technologies like augmented reality and biometric data analysis bring about new ethical questions that must be studied and regulated in advance. Scholars should focus on anticipating and preventing ethical violations before they spread widely. In developing a proactive ethical framework for neuromarketing applications, combining knowledge from computer science, ethics, and consumer behavior is crucial to ensure consumer interests are protected and trust is maintained amidst technological advancements.
Twitter address
Marcus Goncalves: @mv_goncalves
Luis Manuel Cerdá: @LUISCERDASUAREZ-3557059
LinkedIn address
Marcus Goncalves: https://www.linkedin.com/in/marcusgoncalves/
Yiwei Hu: https://www.linkedin.com/in/trista-hu-277870165/
Irene Aliagas: https://www.linkedin.com/in/irenealiagas/
Luis Manuel Cerdá: https://www.linkedin.com/in/luis-manuel-cerdá-suárez
Disclosure statement
No potential conflict of interest was reported by the author(s).
Additional information
Notes on contributors
Marcus Goncalves
Marcus Goncalves, the lead author, is an Associate Professor and Coordinator of the Global Marketing Management program at Boston University Metropolitan College, USA. He holds a dual Ph.D. in International Business from the Catholic University of Portugal/University of Saint Joseph in Macau, China SAR, and an EdD from Boston University Wheelock College, USA. His work in the field has concentrated on the intersection of neuromarketing, AI/ML algorithms, and ethics, mainly focusing on consumer privacy and the responsible application of emerging technologies in marketing strategies. As exemplified in this paper, his research delves into how AI-driven neuromarketing strategies can affect consumer behavior while raising concerns over privacy and ethical considerations. In particular, this study contributes to a broader understanding of the need for ethical frameworks in marketing practices, aligning with global discussions on data protection, such as the GDPR and the ethical deployment of AI. Goncalves’s work is a part of the expansive dialogue on the balance between leveraging technological advances in marketing and safeguarding consumer rights and dignity in the digital age.
Yiwei Hu
Yiwei Hu holds a Master of Science in Applied Business Analytics from Boston University Metropolitan College. She specializes in big data cleansing and analytics, optimizing processes and systems, and creating insightful dashboards. Her proficiency in tools like Python, R, and Tableau was instrumental in this study’s data analysis of AI/ML algorithms in neuromarketing.
Irene Aliagas
Irene Aliagas is an assistant professor and researcher. She holds a PhD in Psychology from the Universidad Complutense de Madrid, Spain. She is a professor of work psychology, consumer psychology, and marketing. Her lines of interest focus on the study of consumer behavior, marketing, neuromarketing, and business. Specifically, she has expanded these interests to the present article, highlighting the need for ethical guidelines to guide the practices carried out concerning AI and neuromarketing. These algorithms significantly impact how neuroscientific data is collected, analyzed, and used to understand consumer behavior. However, this technological advancement raises critical ethical issues, such as data privacy, consumer manipulation, and fairness in decision-making. Therefore, she aims to contribute to developing guidelines and practices that ensure responsible and ethical use of these technologies, benefiting both businesses and consumers.
Luis Manuel Cerdá
Luis Manuel Cerdá Suárez is a researcher in economics and business. He holds a Ph.D. in Finance and Commercial Research from the Universidad Autónoma de Madrid and has a background in Economics and Business Administration from several universities in Spain. He works as a professor at the Universidad Internacional de La Rioja (UNIR). He has also been associated with other institutions, such as the Universidad Carlos III de Madrid and the Universidad de Valladolid. His research interests lie in marketing, business, management, communication, and market research, as evidenced by several publications in the field of marketing, with a focus on digital marketing, neuromarketing, and app environments. His expertise is also reflected in research and academic contributions in marketing, business, management, communication, and market research.
References
- Adams, W. C. (2015). Conducting semi-structured interviews. In Handbook of practical program evaluation (4th ed., pp. 1–48). Wiley Blackwell.
- Adeola, O., Evans, O., Ndubuisi Edeh, J., & Adisa, I. (2022). The future of marketing: Artificial intelligence, virtual reality, and neuromarketing. In Marketing communications and brand development in emerging economies Volume I: Contemporary and future perspectives (pp. 253–280). Springer.
- Adnan, K., & Akbar, R. (2019). An analytical study of information extraction from unstructured and multidimensional big data. Journal of Big Data, 6(1), 38. https://doi.org/10.1186/s40537-019-0254-8
- Adomaitis, L., Grinbaum, A., & Lenzi, D. (2022). TechEthos D2. 2: Identification and specification of potential ethical issues and impacts and analysis of ethical issues of digital extended reality, neurotechnologies, and climate engineering [Doctoral dissertation]. CEA Paris Saclay.
- Affordable MRI. (n.d). Who invented the MRI? Affordable. https://affordablemri.com/who-invented-the-mri/.
- Agrawani, S. (2022). The role of artificial intelligence (AI) in personalized marketing in fashion and apparel industry [Doctoral dissertation]. School of Petroleum Management.
- Agyeman, M. O., Guerrero, A. F., & Vien, Q. T. (2022). A review of classification techniques for arrhythmia patterns using convolutional neural networks and Internet of Things (IoT) devices. IEEE Access.
- Alamri, W. A. (2019). Effectiveness of qualitative research methods: Interviews and diaries. International Journal of English and Cultural Studies, 2(1), 65–70. https://doi.org/10.11114/ijecs.v2i1.4302
- Alarcon, M., & Ha, J. (2020). Assessment of psychological advertising along consumer rights and the rule on section 5 of the federal trade commission, part 1 of 2: Unfairness doctrine. Journal of Applied Business Research (JABR), 36(4), 153–170. https://doi.org/10.19030/jabr.v36i4.10351
- Albahri, A. S., Duhaim, A. M., Fadhel, M. A., Alnoor, A., Baqer, N. S., Alzubaidi, L., Albahri, O. S., Alamoodi, A. H., Bai, J., Salhi, A., Santamaría, J., Ouyang, C., Gupta, A., Gu, Y., & Deveci, M. (2023). A systematic review of trustworthy and explainable artificial intelligence in healthcare: Assessment of quality, bias risk, and data fusion. Information Fusion, 96, 156–191. https://doi.org/10.1016/j.inffus.2023.03.008
- Ali, S., Abuhmed, T., El-Sappagh, S., Muhammad, K., Alonso-Moral, J. M., Confalonieri, R., Guidotti, R., Del Ser, J., Díaz-Rodríguez, N., & Herrera, F. (2023). Explainable Artificial Intelligence (XAI): What we know and what is left to attain Trustworthy Artificial Intelligence. Information Fusion, 99, 101805. https://doi.org/10.1016/j.inffus.2023.101805
- Ali, A., Soomro, T. A., Memon, F., Khan, M. Y. A., Kumar, P., Keerio, M. U., & Chowdhry, B. S. (2022). EEG signals based choice classification for neuromarketing applications. A fusion of artificial intelligence and internet of things for emerging cyber systems (pp. 371–394).
- Allan, L. (2015). Meta-ethics: An introduction. Retrieved May 2, 2022, from www.RationalRealm.com.
- Alsharif, A. H., Salleh, N. Z. M., Abdullah, M., Khraiwish, A., & Ashaari, A. (2023a). Neuromarketing tools used in the marketing mix: A systematic literature and future research agenda. SAGE Open, 13(1), 215824402311565. https://doi.org/10.1177/21582440231156563
- Alsharif, A. H., Salleh, N. Z. M., Al-Zahrani, S. A., & Khraiwish, A. (2022). Consumer behaviour to be considered in advertising: A systematic analysis and future agenda. Behavioral Sciences, 12(12), 472. https://doi.org/10.3390/bs12120472
- Alsharif, A. H., Salleh, N. Z. M., Hashem E, A. R., Khraiwish, A., Putit, L., & Arif, L. S. M. (2023b). Exploring factors influencing neuromarketing implementation in Malaysian universities: barriers and enablers. Sustainability, 15(5), 4603. https://doi.org/10.3390/su15054603
- Andersen, M. M. (2022). Consequentialism. In Oxford research encyclopedia of politics.
- Anshari, M., Syafrudin, M., Fitriyani, N. L., & Razzaq, A. (2022). Ethical Responsibility and Sustainability (ERS) development in a Metaverse Business Model. Sustainability, 14(23), 15805. https://doi.org/10.3390/su142315805
- Aria, M., &Cuccurullo, C. (2017). Bibliometrix : An R-tool for comprehensive science mapping analysis. Journal of Informetrics, 11(4), 959–975. https://doi.org/10.1016/j.joi.2017.08.007
- Ascher, D., Silva, W., Polowczyk, J., & Damião da Silva, E. (2018). Neurostrategy: An advance through the paradigm epistemological in strategic management? Academy of Strategic Management Journal, 7(2), 1–19.
- Assari, S., & Boyce, S. (2021, June) Race, socioeconomic status, and cerebellum cortex fractional anisotropy in pre-adolescents. Adolescents (Basel, Switzerland), 1(2), 70–94. https://doi.org/10.3390/adolescents1020007
- Atlas, L. Y. (2021). A social affective neuroscience lens on placebo analgesia. Trends in Cognitive Sciences, 25(11), 992–1005. https://doi.org/10.1016/j.tics.2021.07.016
- Attié, E., Le Bars, S., & Quenel, I. (2021). Towards ethical neuromarketing 2.0 based on artificial intelligence. In Handbook of research on applied data science and artificial intelligence in business and industry (pp. 619–638). IGI Global.
- Baik, J. S. (2020). Data privacy against innovation or against discrimination?: The case of the California Consumer Privacy Act (CCPA). Telematics and Informatics, 52, 101431. https://doi.org/10.1016/j.tele.2020.101431
- Baker-Brunnbauer, J. (2021). Management perspective of ethics in artificial intelligence. AI and Ethics, 1(2), 173–181. https://doi.org/10.1007/s43681-020-00022-3
- Bakir, V. (2020). Psychological operations in digital political campaigns: Assessing Cambridge Analytica’s psychographic profiling and targeting. Frontiers in Communication, 5, 67. https://doi.org/10.3389/fcomm.2020.00067
- Bang, Y., Lee, N., Yu, T., Khalatbari, L., Xu, Y., Su, D., … Fung, P. (2022). AiSocrates: Towards answering ethical quandary questions. arXiv preprint arXiv:2205.05989.
- Bedoya, S. (2022). Adaptation of graphic design for inclusivity of diverse demographic audiences.
- Belascu, A. (2020, February 19). Neuromarketing ethics: How far is too far? CXL Digital Marketing: https://cxl.com/blog/neuromarketing-ethics/
- Benbya, H., Pachidi, S., & Jarvenpaa, S. (2021). Special issue editorial: Artificial intelligence in organizations: Implications for information systems research. Journal of the Association for Information Systems, 22(2), 281–303. https://doi.org/10.17705/1jais.00662
- Berente, N., Gu, B., Recker, J., & Santhanam, R. (2021). Managing artificial intelligence. MIS Quarterly, 45(3), 1433–1450.
- Bhalla, A., Nikhila, M. S., & Singh, P. (2020). Simulation of self-driving car using deep learning. In Proceedings of the 3rd International Conference on Intelligent Sustainable Systems, ICISS 2020 (pp. 519—525).
- Bibri, S. E. (2022). The social shaping of the metaverse as an alternative to the imaginaries of data-driven smart cities: a study in science, technology, and society. Smart Cities, 5(3), 832–874. https://doi.org/10.3390/smartcities5030043
- Blattner, L., Nelson, S., & Spiess, J. (2021). Unpacking the black box: Regulating algorithmic decisions. arXiv preprint arXiv:2110.03443.
- Blasco-Arcas, L., Lee, H.-H M., Kastanakis, M. N., Alcañiz, M., & Reyes-Menendez, A. (2022). The role of consumer data in marketing: A research agenda. Journal of Business Research, 146, 436–452. https://doi.org/10.1016/j.jbusres.2022.03.054
- Bočková, K., Škrabánková, J., & Hanák, M. (2021). Theory and practice of neuromarketing: Analyzing human behavior in relation to markets. Emerging Science Journal, 5(1), 44–56. https://doi.org/10.28991/esj-2021-01256
- Bowen, S. A. (2020). Communication ethics as a foundational construct in applied communication theory, research, and practice. In The handbook of applied communication Research (pp. 595–612).
- BrandWatch. (2019, October 24). 10 Essential market research method. https://www.brandwatch.com/blog/market-research-methods/
- Brenninkmeijer, J., Schneider, T., & Woolgar, S. (2020). Witness and silence in neuromarketing: Managing the gap between science and its application. Science, Technology, and Human Values, 45(1), 62–86. https://doi.org/10.1177/0162243919829222
- Briesemeister, B., & Selmer, W. K. (2020). Neuromarketing in der Praxis. Den Emotionen auf der.
- Buhalis, D., & Sinarta, Y. (2021). Big Data And Artificial Intelligence For Sustainable And Resilient Smart Cities. Journal of Travel Research, 35(1), 369–393.
- Camilleri, M. A. (2023). Artificial intelligence governance: Ethical considerations and implications for social responsibility. Expert Systems, e13406 https://doi.org/10.1111/exsy.13406
- Carrington, M. J., Neville, B. A., & Whitwell, G. J. (2010). Why ethical consumers don’t walk their talk: Towards a framework for understanding the gap between the ethical purchase intentions and actual buying behaviour of ethically minded consumers. Journal of Business Ethics, 97(1), 139–158. https://doi.org/10.1007/s10551-010-0501-6
- Casado-Aranda, L. A., & Sanchez-Fernandez, J. (2022). Advances in neuroscience and marketing: Analyzing tool possibilities and research opportunities. Spanish Journal of Marketing-ESIC, 26(1), 3–22.
- Casiraghi, S. (2023). Anything new under the sun? Insights from a history of institutionalized AI ethics. Ethics and Information Technology, 25(2), 28. https://doi.org/10.1007/s10676-023-09702-0
- Chang, Y. T., & Fan, N. H. (2023). A novel approach to market segmentation selection using artificial intelligence techniques. The Journal of Supercomputing, 79(2), 1235–1262. https://doi.org/10.1007/s11227-022-04666-2
- Cheng, L., Ma, Q., Qiu, W., & Pei, G. (2022). Decomposing the neural substrates of the supraliminal and subliminal buffering effects of money on negative emotions. Current Psychology, 42(27), 23216–23229. https://doi.org/10.1007/s12144-022-03300-x
- Cheng, H. F., Wang, R., Zhang, Z., O’Connell, F., Gray, T., Harper, F. M., & Zhu, H. (2019, May). Explaining decision-making algorithms through UI: Strategies to help non-expert stakeholders. In Proceedings of the 2019 Chi Conference on Human Factors in Computing Systems (pp. 1–12).
- Cihon, P., Schuett, J., & Baum, S. D. (2021). Corporate governance of artificial intelligence in the public interest. Information, 12(7), 275. https://doi.org/10.3390/info12070275
- Clark, K. R. (2020). A field with a view: Ethical considerations for the fields of consumer neuroscience and neuromarketing. In Developments in neuroethics and bioethics (Vol. 3, pp. 23–61). Academic Press.
- Clithero, J. A., & Rangel, A. (2013). Informatic parcellation of the network involved in the computation of subjective value. Social Cognitive and Affective Neuroscience, 9(9), 1289–1302. p https://doi.org/10.1093/scan/nst106
- Confessore, N. (2018, April 4). Cambridge Analytica and Facebook: The scandal and the fallout so far. https://www.nytimes.com/2018/04/04/us/politics/cambridge-analytica-scandal-fallout.html
- Coper Joseph, M. (2014). Edinburgh research explorer becoming a qualitative researcher becoming a qualitative researcher: A narrative account of conducting my first qualitative study involving in-depth. Life History Interviews, 3(1), 10–20. http://onlineresearchjournals.org/JSS/pdf/2014/jan/Molefe.pdf.
- Copp, D. (Ed.). (2005). The Oxford handbook of ethical theory. Oxford University Press.
- Cowgill, B., Dell’Acqua, F., Deng, S., Hsu, D., Verma, N., & Chaintreau, A. (2020). Biased programmers? Or biased data? A field experiment in operationalizing AI ethics [Paper presentation]. Proceedings of. the 21st ACM Conference on Economics and Computation (pp. 679–681). https://doi.org/10.1145/3391403.3399545
- Crepax, T., & Mühlberg, J. T. (2022). Upgrading the protection of children from manipulative and addictive strategies in online games: Legal and technical solutions beyond privacy regulation. arXiv preprint arXiv:2207.09928.
- Cristofaro, M., Butler, F., Neck, C., Parayitam, S., & Tangpong, C. (2022). Guest editorial: Behavioral strategy:(re-) affirming foundations. Management Research Review, 45(9), 1101–1109. https://doi.org/10.1108/MRR-09-2022-877
- Dąbrowska, J., Almpanopoulou, A., Brem, A., Chesbrough, H., Cucino, V., Di Minin, A., Giones, F., Hakala, H., Marullo, C., Mention, A., Mortara, L., Nørskov, S., Nylund, P. A., Oddo, C. M., Radziwon, A., & Ritala, P. (2022). Digital transformation, for better or worse: A critical multi‐level research agenda. R&D Management, 52(5), 930–954. https://doi.org/10.1111/radm.12531
- Dao, H., & Fukuda, K. (2021, December). Alternative to third-party cookies: investigating persistent PII leakage-based web tracking. In Proceedings of the 17th International Conference on Emerging Networking EXperiments and Technologies (pp. 223–229).
- De Gregorio, G. (2018). From constitutional freedoms to the power of the platforms: Protecting fundamental rights online in the algorithmic society. European Journal of Legal Studies, 11, 65.
- de Oliveira, J. H. C., & Giraldi, J. D. M. E. (2017). What is neuromarketing? A proposal for a broader and more accurate definition. Global Business and Management Research, 9(2), 19.
- de Ridder, D., Kroese, F., & van Gestel, L. (2022). Nudgeability: Mapping conditions of susceptibility to nudge influence. Perspectives on Psychological Science: a Journal of the Association for Psychological Science, 17(2), 346–359. https://doi.org/10.1177/1745691621995183
- Degtiar, S., & Šimulynas, A. (2022). Subliminal and overt social media marketing strategies on the hotel business platform. Res Humanitariae, 30, 48–67. https://doi.org/10.15181/rh.v30i0.2452
- Dwivedi, Y. K., Hughes, L., Wang, Y., Alalwan, A. A., Ahn, S. J., Balakrishnan, J., Barta, S., Belk, R., Buhalis, D., Dutot, V., Felix, R., Filieri, R., Flavián, C., Gustafsson, A., Hinsch, C., Hollensen, S., Jain, V., Kim, J., Krishen, A. S., … Wirtz, J. (2023). Metaverse marketing: How the metaverse will shape the future of consumer research and practice. Psychology & Marketing, 40(4), 750–776. https://doi.org/10.1002/mar.21767
- Dwivedi, Y. K., Kshetri, N., Hughes, L., Slade, E. L., Jeyaraj, A., Kar, A. K., Baabdullah, A. M., Koohang, A., Raghavan, V., Ahuja, M., Albanna, H., Albashrawi, M. A., Al-Busaidi, A. S., Balakrishnan, J., Barlette, Y., Basu, S., Bose, I., Brooks, L., Buhalis, D., … Wright, R. (2023). “So what if ChatGPT wrote it?” Multidisciplinary perspectives on opportunities, challenges and implications of generative conversational AI for research, practice and policy. International Journal of Information Management, 71, 102642. https://doi.org/10.1016/j.ijinfomgt.2023.102642
- Ebrahimian, S., Kalra, M. K., Agarwal, S., Bizzo, B. C., Elkholy, M., Wald, C., Allen, B., & Dreyer, K. J. (2022). FDA-regulated AI algorithms: Trends, strengths, and gaps of validation studies. Academic Radiology, 29(4), 559–566. https://doi.org/10.1016/j.acra.2021.09.002
- Ekanayake, I. U., Meddage, D. P. P., & Rathnayake, U. (2022). A novel approach to explain the black-box nature of machine learning in compressive strength predictions of concrete using Shapley additive explanations (SHAP). Case Studies in Construction Materials, 16, e01059. https://doi.org/10.1016/j.cscm.2022.e01059
- El Ouazzani, Z., & El Bakkali, H. (2020). A classification of non-cryptographic anonymization techniques ensuring privacy in big data. International Journal of Communication Networks and Information Security, 12(1), 142–152.
- European Commission. (2016). General data protection regulation (GDPR). Retrieved May 1, 2023, from https://gdpr.eu/
- European Commission. (2019). Digital single market online platforms: New European rules to improve fairness of online platforms trading practices #DSM importance of the online platform economy. https://publications.europa.eu/en/publication-detail/-/publication/04c75b09-4b2b-11e7-aea8-01aa75ed71a1/language-en
- Fabi, S., & Hagendorff, T. (2022). Why we need biased AI–How including cognitive and ethical machine biases can enhance AI systems. arXiv preprint arXiv:2203.09911. https://doi.org/10.1080/0952813X.2023.2178517
- Falk, E. B., & Weber, J. T. (2022). What neuroscience can contribute to marketing? Journal of Marketing Research, 59(1), 1–10.
- Farah, M. J. (2017). The neuroscience of socioeconomic status: Correlates, causes, and consequences. Neuron, 96(1), 56–71. https://doi.org/10.1016/j.neuron.2017.08.034
- Ferraro, R., Bettman, J. R., & Chartrand, T. L. (2009). The power of strangers: The effect of incidental consumer brand encounters on brand choice. Journal of Consumer Research, 35(5), 729–741. https://doi.org/10.1086/592944
- Finck, M., & Pallas, F. (2020). They who must not be identified—distinguishing personal from non-personal data under the GDPR. International Data Privacy Law, 10(1), 11–36. https://doi.org/10.1093/idpl/ipz026
- Fisher, C. E., Chin, L., & Klitzman, R. (2010). Defining neuromarketing: Practices and professional challenges. Harvard Review of Psychiatry, 18(4), 230–237. https://doi.org/10.3109/10673229.2010.496623
- Fitzsimons, G. J., Hutchinson, J. W., Williams, P., Alba, J. W., Chartrand, T. L., Huber, J., Kardes, F. R., Menon, G., Raghubir, P., Russo, J. E., Shiv, B., & Tavassoli, N. T. (2002). Non-conscious influences on consumer choice. Marketing Letters, 13(3), 269–279. 267–277. https://doi.org/10.1023/A:1020313710388
- Forbes, M., Hwang, J. D., Shwartz, V., Sap, M., & Choi, Y. (2020). Social Chemistry 101: Learning to reason about social and moral norms. arXiv preprint arXiv:2011.00620.
- Forrest, K. B. (2021). When machines can be judge, jury, and executioner: Justice in the age of artificial intelligence.
- Fouquet, R., Laperdrix, P., & Rouvoy, R. (2023). Breaking bad: Quantifying the addiction of web elements to JavaScript. ACM Transactions on Internet Technology, 23(1), 1–28. https://doi.org/10.1145/3579846
- Frederick, D. P. (2022). Recent trends in neuro marketing–An exploratory study.
- Gaborović, A., Karić, K., Blagojević, M., & Plašić, J. (2022). Comparative analysis of ISO/IEC and IEEE standards in the field of Internet of Things [Paper presentation]. Proceedings TIE 2022 9th International Scientific Conference Technics and Informatics in Education. University of Kragujevac, Faculty of Technical Sciences, Čačak. https://doi.org/10.46793/TIE22.243G
- Gadde, S., Amutharaj, J., & Usha, S. (2023). Cloud multimedia data security by optimization-assisted cryptographic technique. International Journal of Image and Graphics, 24(01), pp. 2450010:1–2450010:21. https://doi.org/10.1142/S0219467824500104
- Gal, U., Jensen, T. B., & Stein, M. K. (2020). Breaking the vicious cycle of algorithmic management: A virtue ethics approach to people analytics. Information and Organization, 30(2), 100301. https://doi.org/10.1016/j.infoandorg.2020.100301
- Galli, F. (2022). Algorithmic manipulation. In Algorithmic marketing and EU law on unfair commercial practices (pp. 209–259). Springer International Publishing.
- Galvin, R. (2015). How many interviews are enough? Do qualitative interviews in building energy consumption research produce reliable knowledge? Journal of Building Engineering, pp1, 2–12. https://doi.org/10.1016/j.jobe.2014.12.001
- Garofalo, C., & Gallucci, F. (2021). From marketing to neuromarketing: Ethical considerations. Applied Marketing Analytics, 7(1), 65–72.
- Ge, Y., Liu, S., Fu, Z., Tan, J., Li, Z., Xu, S., … Zhang, Y. (2022). A survey on trustworthy recommender systems. arXiv preprint arXiv:2207.12515.
- Gehl, R. W. (2016). The politics of punctualization and depunctualization in the digital advertising alliance. The Communication Review, 19(1), 35–54. https://doi.org/10.1080/10714421.2016.1128187
- George, A. S., & George, A. H. (2022). Data sharing made easy by technology trends: New data sharing and privacy preserving technologies that bring in a new era of data monetization. Partners Universal International Research Journal, 1(3), 13–19.
- Gesang, B. (2021). Utilitarianism and heuristics. The Journal of Value Inquiry, 55(4), 705–723. https://doi.org/10.1007/s10790-020-09763-0
- Ghelani, D., Hua, T. K., & Koduru, S. K. R. (2022). Cyber security threats, vulnerabilities, and security solutions models in banking. Authorea Preprints.
- Gill, S. L. (2020). Qualitative sampling methods. Journal of Human Lactation, 36(4), 579–581. https://doi.org/10.1177/0890334420949218
- GMarket Data Forecast. (2022). Global neuromarketing market segmentation by technology (functional resonance imaging (fMRI), electroencephalography (EEG), eye tracking, positron emission tomography (PET), and magneto encephalography (MEG)), end-user (retail, healthcare, food and beverage, and consumer electronics), solutions (consumer experience, people engagement, and merchandise development), and region. Retrieved May 2, 2022, from https://www.marketdataforecast.com/market-reports/neuromarketing-market.
- Goel, P., Patel, R., Garg, D., & Ganatra, A. (2021, May). A review on big data: privacy and security challenges. In 2021, 3rd International Conference on Signal Processing and Communication (ICPSC) (pp. 705–709.). IEEE.
- Goncalves, M., Luther, V., & Hayes, S. (2022). Neuromarketing’s socioeconomic status, racial discrimination and lack of transparency. Journal of International Business and Management, 5(12), 1–18. https://doi.org/10.37227/ARICON-JIBM-2022-11-6734
- Goncalves, M. V., Marques, J. A. L., Silva, B. R. S., Luther, V., & Hayes, S. (2022). Neuromarketing and global branding reaction analysis based on real-time monitoring of multiple Consumer’s biosignals and emotions. Available at SSRN 4071297.
- Goncalves, M., Marques, J. A. L., Silva, B., Luther, V., & Hayes, S. H. (2023). Neuromarketing, and global branding reaction analysis based on real-time monitoring of multiple consumer’s biosignals and emotions. Journal of International Business and Management, 6(5), 1–32. https://doi.org/10.37227/JIBM-2023-04-5912
- Greely, H. T. (2021). Human brain surrogates research: The onrushing ethical dilemma. The American Journal of Bioethics: AJOB, 21(1), 34–45. https://doi.org/10.1080/15265161.2020.1845853
- Greenwald, A. G., Dasgupta, N., Dovidio, J. F., Kang, J., Moss-Racusin, C. A., & Teachman, B. A. (2022). Implicit-bias remedies: Treating discriminatory bias as a public-health problem. Psychological Science in the Public Interest: a Journal of the American Psychological Society, 23(1), 7–40. https://doi.org/10.1177/15291006211070781
- Gstrein, O. J., & Beaulieu, A. (2022). How to protect privacy in a datafied society? A presentation of multiple legal and conceptual approaches. Philosophy & Technology, 35(1), 3. https://doi.org/10.1007/s13347-022-00497-4
- Gurgu, E., Gurgu, I.-A., & Tonis, R. B. M. (2020). Neuromarketing for a better understanding of consumer needs and emotions. Independent Journal of Management & Production, 11(1), 208. Doi https://doi.org/10.14807/ijmp.v11i1.993
- Hacker, P. (2022). The European AI liability directives–Critique of a half-hearted approach and lessons for the future. arXiv preprint arXiv:2211.13960.
- Häglund, E., &Björklund, J. (2022). Ai-driven contextual advertising: A technology report and implication analysis. Cornell University Arxrv.
- Haleem, A., Javaid, M., Qadri, M. A., Singh, R. P., & Suman, R. (2022). Artificial intelligence (AI) applications for marketing: A literature-based study. International Journal of Intelligent Networks, 3, 119–132. https://doi.org/10.1016/j.ijin.2022.08.005
- Halkiopoulos, C., Antonopoulou, H., Gkintoni, E., & Aroutzidis, A. (2022). Neuromarketing as an indicator of cognitive consumer behavior in decision-making process of tourism destination—An overview [Paper presentation]. Transcending Borders in Tourism through Innovation and Cultural Heritage: 8th International Conference, April)., IACuDiT, Hydra, Greece, 2021 (pp. 679–697). Cham: Springer International Publishing.
- Hämäläinen, N. (2016). Descriptive ethics: What does moral philosophy know about morality?. Descriptive ethics: What does moral philosophy know about morality?. Palgrave Macmillan. https://doi.org/10.1057/978-1-137-58617-9
- Hamler, T. C., Nguyen, A. W., Keith, V., Qin, W., & Wang, F. (2022). How skin tone influences relationships between discrimination, psychological distress, and self-rated mental health among older African Americans. The Journals of Gerontology. Series B, Psychological Sciences and Social Sciences, 77(11), 2026–2037. https://doi.org/10.1093/geronb/gbac115
- Hanoglu, I. S. (2023). A cookieless future: Solving the UX problems caused by the privacy protections method.
- Harjule, P., Rahman, A., Agarwal, B., & Tiwari, V. (Eds.). (2023). Computational statistical methodologies and modeling for artificial intelligence. CRC Press.
- Harmsel, T. B. H. (2021). To plant a seed is to believe in tomorrow. An experimental study on the impact of subliminal-and supraliminal priming on attitude, generativity, purchase intention and product choice with regard to green behaviour (Master’s thesis, University of Twente).
- Harrell, E. (2019, January 23). Neuromarketing: What you need to know. HBR.org: https://hbr.org/2019/01/neuromarketing-what-you-need-to-know
- Harsanyi, J. C. (1977). Rule utilitarianism and decision theory. Erkenntnis, 11(1), 25–53. https://doi.org/10.1007/BF00169843
- Hawkins, M. (2022). Metaverse live shopping analytics: Retail data measurement tools, computer vision and deep learning algorithms, and decision intelligence and modeling. Journal of Self-Governance and Management Economics, 10(2), 22–36.
- Hayes, J. L., Brinson, N. H., Bott, G. J., & Moeller, C. M. (2021). The influence of consumer–brand relationship on the personalized advertising privacy calculus in social media. Journal of Interactive Marketing, 55(1), 16–30. https://doi.org/10.1016/j.intmar.2021.01.001
- He, L., Freudenreich, T., Yu, W., Pelowski, M., & Liu, T. (2021). Methodological structure for future consumer neuroscience research. Psychology & Marketing, 38(8), 1161–1181. https://doi.org/10.1002/mar.21478
- Hensel, D., Wolter, L., & Znanewitz, J. (2016). A guideline for ethical aspects in conducting neuromarketing studies. In A. R. Thomas, N. A. Pop, A. M. Iorga, & C. Ducu (Eds.), Ethics and neuromarketing - implications for market research and business practice (pp. 65–87). Switzerland: Springer. https://doi.org/10.1007/978-3-319-45609-6_4
- Hensel, D., Iorga, A., Wolter, L., & Znanewitz, J. (2017a). Conducting neuromarketing studies ethically-practitioner perspectives. Cogent Psychology, 4(1), 1320858. https://doi.org/10.1080/23311908.2017.1320858
- Hensel, D., Wolter, L. C., & Znanewitz, J. (2017b). A guideline for ethical aspects in conducting neuromarketing studies. In Ethics and neuromarketing (pp. 65–87). Springer.
- Hill, K. (2022). The secretive company that might end privacy as we know it. In Ethics of data and analytics (pp. 170–177). Auerbach Publications.
- Howard, P. N. (2020). Lie machines: How to save democracy from troll armies, deceitful robots, junk news operations, and political operatives. Yale University Press.
- Huang, T., & Rust, R. T. (2022). Transforming marketing through data analytics: Insights and research opportunities. Journal of Marketing, 86(2), 17–20.
- Hula, R. (2022a). Strategy of ethical approach in neuromarketing in terms of marketing research. Ekonomické Rozhľady – Economic Review, 51(2), 105–128. https://doi.org/10.53465/ER.2644-7185.2022.2.105-128
- Hula, R. (2022b). Neuromarketing as a tool for data acquisition in consumer behaviour. Central and Eastern Europe in the Changing Business Environment.
- Ienca, M., Fins, J. J., Jox, R. J., Jotterand, F., Voeneky, S., Andorno, R., Ball, T., Castelluccia, C., Chavarriaga, R., Chneiweiss, H., Ferretti, A., Friedrich, O., Hurst, S., Merkel, G., Molnár-Gábor, F., Rickli, J.-M., Scheibner, J., Vayena, E., Yuste, R., & Kellmeyer, P. (2022). Towards a governance framework for brain data. Neuroethics, 15(2), 20. https://doi.org/10.1007/s12152-022-09498-8
- Islam, M. A., & Aldaihani, F. M. F. (2022). Justification for adopting qualitative research method, research approaches, sampling strategy, sample size, interview method, saturation, and data analysis. Journal of International Business and Management, 5(1), 01–11.
- Islam, S. R., Eberle, W., Ghafoor, S. K., & Ahmed, M. (2021). Explainable artificial intelligence approaches: A survey. arXiv preprint arXiv:2101.09429.
- Ivanova, Y. (2021). The Role of the EU Fundamental Right to data protection in an Algorithmic and big data world. In Data protection and privacy, Volume 13: Data protection and artificial intelligence (pp. 13–145). Bloomsbury Publishing.
- Jaiswal, A., Arun, C. J., & Varma, A. (2022). Rebooting employees: Upskilling for artificial intelligence in multinational corporations. The International Journal of Human Resource Management, 33(6), 1179–1208. https://doi.org/10.1080/09585192.2021.1891114
- Jehn, K. A., & Jonsen, K. (2010). A multimethod approach to the study of sensitive organizational issues. Journal of Mixed Methods Research, 4(4), 313–341. https://doi.org/10.1177/1558689810380920
- Jiang, P., Dieckmann, E., Han, J., & Childs, P. R. (2021b). A bibliometric review of sustainable product design. Energies, 14(21), 6867. https://doi.org/10.3390/en14216867
- Jiang, L., Hwang, J. D., Bhagavatula, C., Bras, R. L., Forbes, M., Borchardt, J., … Choi, Y. (2021a). Delphi: Towards machine ethics and norms. arXiv preprint arXiv:2110.07574.
- Jin, G. Z. (2018). Artificial intelligence and consumer privacy. In A. Agrawal, J. Gans, A. Goldfarb (Eds), The economics of artificial intelligence: An agenda (pp. 439–462).
- Jöhnk, J., Weißert, M., & Wyrtki, K. (2021). Ready or not, AI comes—An interview study of organizational AI readiness factors. Business & Information Systems Engineering, 63(1), 5–20. https://doi.org/10.1007/s12599-020-00676-7
- Jones, K. (2023). AI governance and human rights.
- Kabanda, G. (2023). 14 Performance of machine learning and big data analytics paradigms in cyber security. AI, machine learning and deep learning: A security perspective.
- Kaheh, S., Ramirez, M., Wong, J., & George, K. (2021). Neuromarketing using EEG Signals and Eye-tracking. In 2021 IEEE International Conference on Electronics, Computing and Communication Technologies (CONNECT) (pp. 1–4). IEEE.
- Kalaganis, F. P., Georgiadis, K., Oikonomou, V. P., Laskaris, N. A., Nikolopoulos, S., & Kompatsiaris, I. (2021). Unlocking the subconscious consumer bias: a survey on the past, present, and future of hybrid EEG schemes in neuromarketing. Frontiers in Neuroergonomics, 2, 672982. https://doi.org/10.3389/fnrgo.2021.672982
- Kalkova, N., Yarosh, O. B., Mitina, E. A., & Velgosh, N. Z. (2023). Neuromarketing research on consumers’ Visual perception of cryptomarking a product package. ABAC Journal, 43(1), 69–84.
- Kannan, P. K., & Li, H. (2022). Digital marketing and analytics: The power of big data. Journal of Advertising Research, 62(1), 13–17.
- Kapitonova, M., Kellmeyer, P., Vogt, S., & Ball, T. (2022). A framework for preserving privacy and cybersecurity in brain-computer interfacing applications. arXiv preprint arXiv:2209.09653.
- Karl, K., & Tao, Y. (2023). Correcting overconfidence in online privacy: Experimenting with an educational game. Information, Communication & Society, 26(5), 990–1007. https://doi.org/10.1080/1369118X.2023.2166360
- Kaufmann, J., Hilgert, F., & Wohlthat, R. (2022). The proposed American data privacy and protection act in comparison with GDPR: Does the current US bill of the ADPPA converge towards the “gold standard” concepts under the EU GDPR–or not? Computer Law Review International, 23(5), 146–152. https://doi.org/10.9785/cri-2022-230505
- Kaul, V. (2022). Awareness and perception of the neuromarketing [Doctoral dissertation].
- Kaur, V. (2023). Knowledge-based dynamic capabilities: A scientometric analysis of marriage between knowledge management and dynamic capabilities. Journal of Knowledge Management, 27(4), 919–952. https://doi.org/10.1108/JKM-02-2022-0112
- Kaur, R., Singh, R., Gehlot, A., Priyadarshi, N., & Twala, B. (2022). Marketing strategies 4.0: Recent trends and technologies in marketing. Sustainability, 14(24), 16356. https://doi.org/10.3390/su142416356
- Keller, K. L. (2000). The brand report card. Harvard Business Review, 78, 147–157.
- Khurana, V., Gahalawat, M., Kumar, P., Roy, P. P., Dogra, D. P., Scheme, E., & Soleymani, M. (2021). A survey on neuromarketing using EEG signals. IEEE Transactions on Cognitive and Developmental Systems, 13(4), 732–749. https://doi.org/10.1109/TCDS.2021.3065200
- Kietzmann, J., Paschen, J., & Treen, E. (2018). Artificial intelligence in advertising: How marketers can leverage artificial intelligence along the consumer journey. In. Journal of Advertising Research, 58(3), 263–267. https://doi.org/10.2501/JAR-2018-035
- Kislov, A., Shestakova, A., Ushakov, V., Martinez-Saito, M., Beliaeva, V., Savelo, O., Vasilchuk, A., & Klucharev, V. (2023). The prediction of market-level food choices by the neural valuation signal. PloS One, 18(6), e0286648. https://doi.org/10.1371/journal.pone.0286648
- Kliestik, T., Zvarikova, K., & Lăzăroiu, G. (2022). Data-driven machine learning and neural network algorithms in the retailing environment: Consumer engagement, experience, and purchase behaviors. Economics, Management, and Financial Markets, 17(1), 57–69.
- Koop, M., Tews, E., & Katzenbeisser, S. (2020). In-depth evaluation of redirect tracking and link usage. Proceedings on Privacy Enhancing Technologies, 2020(4), 394–413. https://doi.org/10.2478/popets-2020-0079
- Koritnik, B., & Merc, P. (2022). New digital public health tools: Privacy by design in contact tracing mobile apps for COVID-19. International Journal of Public Sector Performance Management, 9(4), 399–410. https://doi.org/10.1504/IJPSPM.2022.123707
- Kotras, B. (2020). Mass personalization: Predictive marketing algorithms and the reshaping of consumer knowledge. Big Data & Society, 7, 2053951720951581. https://doi.org/10.1177/2053951720951581
- Krieger, N. (2021). Ecosocial theory, embodied truths, and the people’s health. Oxford University Press.
- Kritikos, M. (2018). What if algorithms could abide by ethical principles? Retrieved April 12, 2022, from http://www.europarl.europa.eu/thinktank.
- Kumar, S., & Choudhury, S. (2022). Normative ethics, human rights, and artificial intelligence. AI and Ethics, 1–10.
- Kyaw, K. S., Tepsongkroh, P., Thongkamkaew, C., & Sasha, F. (2023). Business intelligent framework using sentiment analysis for smart digital marketing in the E-commerce era. Asia Social Issues, 16(3), e252965-e252965. https://doi.org/10.48048/asi.2023.252965
- Laczniak, G. R., & Murphy, P. E. (2019). The role of normative marketing ethics. Journal of Business Research, 95, 401–407. https://doi.org/10.1016/j.jbusres.2018.07.036
- Lanz, L., Briker, R., & Gerpott, F. H. (2024). Employees adhere more to unethical instructions from human than AI supervisors: Complementing experimental evidence with machine learning. Journal of Business Ethics, 189(3), 625–646.
- Lee, N., & Chamberlain, L. (2022). The rise and rise of neuromarketing: A bibliometric analysis. Journal of Business Research, 145, 391–403.
- Leese, M., Lidén, K., & Nikolova, B. (2019). Putting critique to work: Ethics in EU security research. Security Dialogue, 50(1), 59–76. https://doi.org/10.1177/0967010618809554
- Lee, I., & Shin, Y. J. (2020). Machine learning for enterprises: Applications, algorithm selection, and challenges. Business Horizons, 63(2), 157–170. https://doi.org/10.1016/j.bushor.2019.10.005
- Lee, S., & Yang, C. (2021). Artificial intelligence and machine learning in tourism and hospitality: Applications and implications. Journal of Travel Research, 72(4), 132–148.
- Lee, O. (2020). Artificial intelligence and data protection how the GDPR regulates AI. https://www.lexico.com/definition/artificial_intelligence.
- Levrini, G. R., & Jeffman dos Santos, M. (2021). The influence of price on purchase intentions: Comparative study between cognitive, sensory, and neurophysiological experiments. Behavioral Sciences, 11(2), 16. https://doi.org/10.3390/bs11020016
- Levy, J. (2018). 7 ways AI is impacting marketing. https://www.entrepreneur.com/article/308471.
- Liljevall, A., & Lillskog, E. (2023). Mind the gap between your intelligence and choice architecture.
- Liu, H., & Chen, C. (2019). Data processing strategies in wind energy forecasting models and applications: A comprehensive review. Applied Energy, 249, 392–408. https://doi.org/10.1016/j.apenergy.2019.04.188
- Liu, M. T., Liu, Y., & Mo, Z. (2020). Moral norm is the key: An extension of the theory of planned behaviour (TPB) on Chinese consumers’ green purchase intention. Asia Pacific Journal of Marketing and Logistics, 32(8), 1823–1841. https://doi.org/10.1108/APJML-05-2019-0285
- Loi, M., Ferrario, A., & Viganò, E. (2021). Transparency as design publicity: Explaining and justifying inscrutable algorithms. Ethics and Information Technology, 23(3), 253–263. https://doi.org/10.1007/s10676-020-09564-w
- Lõuk, K. (2023). Check for relevance and challenges of ethics committees Kristi Lõuk () University of Tartu. Ülikooli 18, 50090 Tartu, Estonia Kristi. louk@ ut. ee. Ethics and Responsible Research and Innovation in Practice: The ETHNA System Project, 13875, 125.
- Lucaj, L., van der Smagt, P., & Benbouzid, D. (2023, June). AI regulation is (not) all you need [Paper presentation]. Proceedings of the 2023 ACM Conference on Fairness, Accountability, and Transparency (pp. 1267–1279). https://doi.org/10.1145/3593013.3594079
- Luna-Nevarez, C. (2021). Neuromarketing, ethics, and regulation: An exploratory analysis of consumer opinions and sentiment on blogs and social media. Journal of Consumer Policy, 44(4), 559–583. https://doi.org/10.1007/s10603-021-09496-y
- Ma, L., & Sun, B. (2020). Machine learning and AI in marketing – Connecting computing power to human insights. International Journal of Research in Marketing, 37(3), 481–504. https://doi.org/10.1016/j.ijresmar.2020.04.005
- Magalhães, J. C. (2018). Do algorithms shape character? Considering algorithmic ethical subjectivation. Social Media + Society, 4(2), 205630511876830. https://doi.org/10.1177/2056305118768301
- Mandolfo, M., & Lamberti, L. (2021). Past, present, and future of impulse buying research methods: A systematic literature review. Frontiers in Psychology, 12, 687404. https://doi.org/10.3389/fpsyg.2021.687404
- Martínez-López, F. J., Li, Y., Liu, H., & Feng, C. (2020). Do safe buy buttons and integrated path-to-purchase on social platforms improve users’ shopping-related responses? Electronic Commerce Research and Applications, 39, 100913. https://doi.org/10.1016/j.elerap.2019.100913
- Marzi, G., Caputo, A., Garces, E., & Dabic, M. (2020). A three-decade mixed-method bibliometric investigation of the IEEE transactions on engineering management. IEEE Transactions on Engineering Management, 67(1), 4–17. https://doi.org/10.1109/TEM.2018.2870648
- Mason, J. A. (2023). Clinical ethics consultation and liturgical practices of participation: A theology of technique for practical ethics [Doctoral dissertation]. Saint Louis University.
- Masten, C. L., Telzer, E. H., & Eisenberger, N. I. (2011). An FMRI investigation of attributing negative social treatment to racial discrimination. Journal of Cognitive Neuroscience, 23(5), 1042–1051. https://doi.org/10.1162/jocn.2010.21520
- Matulionyte, R. (2023). Increasing transparency around facial recognition technologies in law enforcement: Towards a model framework. Available at SSRN, 4408997.
- Mau, G., Schweizer, M., & Oriet, C. (Eds.). (2023). Multisensory in stationary retail: Principles and practice of consumer-centered store design. Springer Nature.
- May, J. (2023). Neuroethics: Agency in the age of brain science. Oxford University Press.
- McKinsey. (2021). Artificial intelligence (AI) adoption worldwide in 2020, by industry and function. https://www.statista.com/statistics/1112982/ai-adoption-worldwide-industry-function/.
- McQuoid-Mason, D. J. (2022). Is there a legal and ethical duty on public sector doctors whose complaints to hospital administrators have been ignored to inform the public about harm to child patients due to intentional maladministration, negligence or indifference at the local and pr. South African Medical Journal, 112(8), 513–515.
- Merz, E. C., Wiltshire, C. A., & Noble, K. G. (2019). Socioeconomic inequality and the developing brain: Spotlight on language and executive function. Child Development Perspectives, 13(1), 15–20. https://doi.org/10.1111/cdep.12305
- Möhlmann, M. (2021). Algorithmic nudges don’t have to be unethical. Harvard Business Review, 22, 1–7.
- Moral-Muñoz, J. A., Herrera-Viedma, E., Santisteban-Espejo, A., & Cobo, M. J. (2020). Software tools for conducting bibliometric analysis in science: An up-to-date review. Profesional de la Informacion, 29(1), 513–515.
- Mordor Intelligence. (2021). Global neuromarketing market - Growth, trends, Covid-19 impact, and forecasts (2022–2027). Retrieved May 12, 2022, from https://www.mordorintelligence.com/industry-reports/neuromarketing-marketplace.
- Morrison, D. (2021). AI and machine learning in digital marketing: Transforming the consumer experience. https://www.digitalmarketing.org/blog/ai-and-machine-learning-in-digital-marketing.
- Mouammine, Y., & Azdimousa, H. (2019). Using Neuromarketing and AI to collect and analyse consumer’s emotion: Literature review and perspectives. https://www.researchgate.net/publication/341369618_Using_Neuromarketing_and_AI_to_collect_and_analyse_consumer%27s_emotion_Literature_review_and_perspectives.
- Mule, J. L. (2021). The effects of native advertising disclosure and advertising recognition on perceptions of news story and news website credibility: A consumer neuroscience approach [Master’s thesis]. Faculty of Commerce.
- Mulgund, P., Mulgund, B. P., Sharman, R., & Singh, R. (2021). The implications of the California Consumer Privacy Act (CCPA) on healthcare organizations: Lessons learned from early compliance experiences. Health Policy and Technology, 10(3), 100543. https://doi.org/10.1016/j.hlpt.2021.100543
- Mumtaz, W., Rasheed, S., & Irfan, A. (2021). Review of challenges associated with the EEG artifact removal methods. Biomedical Signal Processing and Control, 68, 102741. https://doi.org/10.1016/j.bspc.2021.102741
- Nam, K. (2022). Conversion paths of online consumers: A sequential pattern mining approach. Expert Systems with Applications, 202, 117253. https://doi.org/10.1016/j.eswa.2022.117253
- Nanda, S., Khattar, K., & Nanda, S. (2019, February). Internet of Things based remote wearable health solutions: Prospects and area of research. In Proceedings of International Conference on Sustainable Computing in Science, Technology and Management (SUSCOM). Amity University Rajasthan, Jaipur-India.
- Närväinen, L. (2023). The ethical problems of neuromarketing: Focus on the Finnish market.
- Neal, D., Gaber, S., Joddrell, P., Brorsson, A., Dijkstra, K., & Dröes, R. M. (2023). Read and accepted? Scoping the cognitive accessibility of privacy policies of health apps and websites in three European countries. Digital Health, 9, 20552076231152162. https://doi.org/10.1177/20552076231152162
- Neuwirth, R. J. (2022). The EU artificial intelligence act: regulating subliminal AI systems. Taylor & Francis.
- Nguyen, T. A. (2021). Fundamentals of neuromarketing: An interdisciplinary field that changes how marketers understand consumers.
- Nguyen, M. H., Le, T. T., & Vuong, Q. H. (2023). Ecomindsponge: A novel perspective on human psychology and behavior in the ecosystem. Urban Science, 7(1), 31. https://doi.org/10.3390/urbansci7010031
- Nishant, R., Schneckenberg, D., & Ravishankar, M. N. (2023). The formal rationality of artificial intelligence-based algorithms and the problem of bias. Journal of Information Technology, 39(1), 19–40. https://doi.org/10.1177/02683962231176842
- Oh, H., & Synn, J. (2022). Consumer neuroscience in marketing: Current insights and future directions. Journal of Interactive Marketing, 69, 144–156.
- Ongsulee, P. (2017). 2017 15th International Conference on ICT and Knowledge Engineering (ICT and KE). IEEE.
- Ozmen Garibay, O., Winslow, B., Andolina, S., Antona, M., Bodenschatz, A., Coursaris, C., Falco, G., Fiore, S. M., Garibay, I., Grieman, K., Havens, J. C., Jirotka, M., Kacorri, H., Karwowski, W., Kider, J., Konstan, J., Koon, S., Lopez-Gonzalez, M., Maifeld-Carucci, I., … Xu, W. (2023). Six human-centered artificial intelligence grand challenges. International Journal of Human–Computer Interaction, 39(3), 391–437. https://doi.org/10.1080/10447318.2022.2153320
- Park, S. Q., & Yoon, C. (2022). The future of neuromarketing: Insights from advances in neuroscience and AI. Journal of Advertising, 51(1), 55–69.
- Parrish, B., Heptonstall, P., Gross, R., & Sovacool, B. K. (2020). A systematic review of motivations, enablers and barriers for consumer engagement with residential demand response. Energy Policy, 138, 111221. https://doi.org/10.1016/j.enpol.2019.111221
- Peloquin, D., DiMaio, M., Bierer, B., & Barnes, M. (2020). Disruptive and avoidable: GDPR challenges to secondary research uses of data. European Journal of Human Genetics: EJHG, 28(6), 697–705. https://doi.org/10.1038/s41431-020-0596-x
- Penrod, J. (2022). Ethics and biopower in neuromarketing: A framework for an ethical approach to marketing. Springer Nature.
- Penrod, J. (2022a). A new look at ethics: Veridical sovereignty and its implications. In Ethics and biopower in neuromarketing: A framework for an ethical approach to marketing (pp. 99–155). Springer International Publishing.
- Penrod, J. (2022b). Consumer neuroscience, neuromarketing, and foucault. In Ethics and biopower in neuromarketing: A framework for an ethical approach to marketing (pp. 1–26). Springer International Publishing.
- Petrillo, A., De Felice, F., Achim, M. V., & Mirza, N. (Eds.). (2022). Digital transformation: Towards new frontiers and business opportunities. BoD–Books on Demand.
- Pluta-Olearnik, M., & Szulga, P. (2022). The importance of emotions in consumer purchase decisions—A neuromarketing approach. Marketing of Scientific and Research Organizations, 44(2), 87–104. https://doi.org/10.2478/minib-2022-0010
- Portmore, D. W. (Ed.). (2020). The Oxford handbook of consequentialism. Oxford University Press.
- Poulsen, A. H. N., & Christensen, A. M. S. (2023). The invention and re-invention of meta-ethics. The Journal of Value Inquiry, 1–18. https://doi.org/10.1007/s10790-023-09935-8
- Quach, S., Thaichon, P., Martin, K. D., Weaven, S., & Palmatier, R. W. (2022). Digital technologies: Tensions in privacy and data. Journal of the Academy of Marketing Science, 50(6), 1299–1323. https://doi.org/10.1007/s11747-022-00845-y
- Rachels, J., & Rachels, S. (2012). The elements of moral philosophy 7e. McGraw Hill.
- Radua, J. (2021). PRISMA 2020-An updated checklist for systematic reviews and metaanalyses. Neuroscience and Biobehavioral Reviews, 124, 324–325. https://doi.org/10.1016/j.neubiorev.2021.02.016
- Raj, R., Balakrishna, S., Shruthi, M. L. J., Kulal, D. H., & Singh, S. (2022). A survey on machine learning-based facial recognition algorithm [Paper presentation]. 2022 International Conference on Smart Technologies and Systems for Next Generation Computing (ICSTSN) (pp. 1–7). IEEE. https://doi.org/10.1109/ICSTSN53084.2022.9761288
- Rakić, V. (2021). The ultimate enhancement of morality. Springer.
- Ranganathan, B. (2022). Deontology. In Encyclopedia of religious ethics (pp. 178–186).
- Rawnaque, F. S., Rahman, K. M., Anwar, S. F., Vaidyanathan, R., Chau, T., Sarker, F., & Mamun, K. (2020). Technological advancements and opportunities in Neuromarketing: A systematic review. Brain Informatics, 7(1), 10. https://doi.org/10.1186/s40708-020-00109-x
- Reith, G. (2022). Capitalism and the commodification of pleasure (p. 1846). Routledge Handbook of Intoxicants and Intoxication.
- Remišová, A., Lašáková, A., & Kirchmayer, Z. (2019). Influence of formal ethics program components on managerial ethical behavior. Journal of Business Ethics, 160(1), 151–166. https://doi.org/10.1007/s10551-018-3832-3
- Reuille-Dupont, J. (2023). The power of algorithms and big data: A marketing perspective on consumer manipulation in business. University Honors Theses. Paper 1309. https://doi.org/10.15760/honors.1338
- Reuter, C., Iacono, L. L., & Benlian, A. (2022). A quarter century of usable security and privacy research: Transparency, tailorability, and the road ahead. Behaviour & Information Technology, 41(10), 2035–2048. https://doi.org/10.1080/0144929X.2022.2080908
- Rialti, R., Marzi, G., Ciappei, C., & Busso, D. (2019). Big data and dynamic capabilities: A bibliometric analysis and systematic literature review. Management Decision, 57(8), 2052–2068. https://doi.org/10.1108/MD-07-2018-0821
- Rogers, S. (1992). How a publicity blitz created the myth of subliminal advertising. Public Relations Quarterly, 37, 12–17.
- Rogerson, S., Miller, K. W., Winter, J. S., & Larson, D. (2017). Information systems ethics–challenges and opportunities. Journal of Information, Communication, and Ethics in Society, 17(1), 87–97.
- Rollins, O. (2021). Towards an antiracist (neuro)science. Nature Human Behaviour, 5(5), 540–541. https://www.nature.com/articles/s41562-021-01075-y. https://doi.org/10.1038/s41562-021-01075-y
- Royo-Vela, M., & Varga, Á. (2022). Unveiling neuromarketing and its research methodology. Encyclopedia, 2(2), 729–751. https://doi.org/10.3390/encyclopedia2020051
- Saad, G. (2020). The marketing of evolutionary psychology. Journal of Business Research, 120, 485–491. https://doi.org/10.1016/j.jbusres.2019.03.048
- Saarikivi, K., Chan, T. M. V., Huotilainen, M., Tervaniemi, M., & Putkinen, V. (2023). Enhanced neural mechanisms of set shifting in musically trained adolescents and young adults: converging fMRI, EEG, and behavioral evidence. Cerebral Cortex, 33(11), 7237–7249. https://doi.org/10.1093/cercor/bhad034
- Saheb, T., Dehghani, M., & Saheb, T. (2022). Artificial intelligence for sustainable energy: A contextual topic modeling and content analysis. Sustainable Computing: Informatics and Systems, 35, 100699.
- Sætra, H. S. (2020). A shallow defense of a technocracy of artificial intelligence: Examining the political harms of algorithmic governance in the domain of government. Technology in Society, 62, 101283. https://doi.org/10.1016/j.techsoc.2020.101283
- Sample, M., Sattler, S., Blain-Moraes, S., Rodríguez-Arias, D., & Racine, E. (2020). Do publics share experts’ concerns about brain–computer interfaces? A trinational survey on the ethics of neural technology. Science Technology and Human Values, 45(6), 1242–1270. https://doi.org/10.1177/0162243919879220
- Sapra, L., Bhatt, R., & Thakur, G. (2022). An analysis for the prediction of human behaviour & observation level on social media using machine learning approaches. Mathematical Statistician and Engineering Applications, 71(4), 2606–2620.
- Savage, C. W. (2019). Managing the ambient trust commons: The economics of online consumer information privacy. Stan. Tech. L. Rev., 22, 95.
- Scanagatta, C. (2021). Exploring the consumer’s brain: a neuromarketing approach.
- Scheyer, P. (2019). Corrupting effectiveness: Utilitarianism and moral impartiality toward future persons in pragmatic evaluation of altruistic interventions.
- Schibuk, H. A. (2022). Adulting ‘when’ the struggle is real’: Does executive function predict career adaptability in emerging adults [Doctoral dissertation]. Fordham University.
- Schmit, C. (2023). Ongoing and emerging issues in privacy and security in a post COVID-19 era: An environmental scan. National Committee on Vital and Health Statistics.
- Schwarz, E. (2022). Memory index: When are stimuli stored and how?. In Neuro-advertising: Brain-friendly advertising for more success in your market (pp. 109–142). Springer Fachmedien Wiesbaden.
- Schweidel, D. A., Reisenbichler, M., Reutterer, T., & Zhang, K. (2023). Leveraging AI for content generation: A consumer equity perspective. In Artificial intelligence in marketing (pp. 125–145). Emerald Publishing Limited.
- Seif-Farshad, M., Kheire, Y., & Madayen, S. M. A. (2021). Inference of Mulla Sadra’s ethical views in the four realms of meta-ethics. International Journal of Multicultural and Multireligious Understanding, 8(9), 109–119. https://doi.org/10.18415/ijmmu.v8i9.2895
- Semantha, F. H., Azam, S., Shanmugam, B., & Yeo, K. C. (2023). PbDinEHR: A novel privacy by design developed framework using distributed data storage and sharing for secure and scalable electronic health records management. Journal of Sensor and Actuator Networks, 12(2), 36. https://doi.org/10.3390/jsan12020036
- Sengur, F. K., & Goncalves, M. (2023). Neuromanagement and neuromarketing: Neuroscience in Organisations, chapter 10. In The Routledge companion to the future of management research. https://doi.org/10.4324/9781003225508-12
- Seth, S., & Mahato, M. K. (2023). Decoding neurotic stimulations via application of neuromarketing & bio-imaging techniques. Journal of Coastal Life Medicine, 11(1), 437–446.
- Sheth, J. (2021). New areas of research in marketing strategy, consumer behavior, and marketing analytics: the future is bright. Journal of Marketing Theory and Practice, 29(1), 3–12. https://doi.org/10.1080/10696679.2020.1860679
- Shin, D., Lim, J. S., Ahmad, N., & Ibahrine, M. (2022). Understanding user sensemaking in fairness and transparency in algorithms: algorithmic sensemaking in over-the-top platform. AI & Society, 1–14. Springer. https://doi.org/10.1007/s00146-022-01525-9
- Shin, D., & Park, Y. J. (2019). Role of fairness, accountability, and transparency in algorithmic affordance. Computers in Human Behavior, 98, 277–284. https://doi.org/10.1016/j.chb.2019.04.019
- Sidorenko, E. L., Khisamova, Z. I., & Monastyrsky, U. E. (2021). The main ethical risks of using artificial intelligence in business. In Current achievements, challenges and digital chances of knowledge-based economy (pp. 423–429). Springer.
- Şik, E., & Soba, M. (2021). Eyes do not lie. Investigating the effectiveness of outdoor advertising instruments: an applied neuromarketing research with eye-tracking technique. Acta Bioethica, 27(2), 235–246.
- Solove, D. J. (2023 , January 11). Data is what data does: Regulating Use, Harm, and risk instead of sensitive data. Harm, and risk instead of sensitive data.
- Southern, M. G. (2021). Google to face $5B lawsuit over tracking users in incognito mode. Retrieved December 1, 2022, from https://www.searchenginejournal.com/google-to-face-5b-lawsuit-over-tracking-users-in-incognito-mode/399113/#close
- Spence, C. (2020). On the ethics of neuromarketing and sensory marketing. In Organizational neuroethics (pp. 9–29). Springer.
- Srinivasa, R. (2022). Effectiveness of neuromarketing in food companies: Hijacking brains [Doctoral dissertation]. Dublin, National College of Ireland.
- Srivastava, G., & Bag, S. (2023). Modern-day marketing concepts based on face recognition and neuro-marketing: A review and future research directions. Benchmarking: An International Journal, 31(2), 410–438. https://doi.org/10.1108/BIJ-09-2022-0588
- Srivastava, M., Sharma, G. D., Srivastava, A. K., & Kumaran, S. S. (2020). What’s in the brain for us: A systematic literature review of neuroeconomics and neurofinance. Qualitative Research in Financial Markets, 12(4), 413–435. https://doi.org/10.1108/QRFM-10-2019-0127
- Stahl, B. C., Akintoye, S., Bitsch, L., Bringedal, B., Eke, D., Farisco, M., Grasenick, K., Guerrero, M., Knight, W., Leach, T., Nyholm, S., Ogoh, G., Rosemann, A., Salles, A., Trattnig, J., & Ulnicane, I. (2021). From responsible research and innovation to responsibility by design. Journal of Responsible Innovation, 8(2), 175–198. 2 https://doi.org/10.1080/23299460.2021.1955613
- Stanton, S. J., Sinnott-Armstrong, W., & Huettel, S. A. (2017). Neuromarketing: Ethical implications of its use and potential misuse. Journal of Business Ethics, 144(4), 799–811. https://doi.org/10.1007/s10551-016-3059-0
- Strieder, N. (2022). Neuromarketing: Human behaviour & decision making in consumer based neuroscientific research.
- Suhler, C. L., & Churchland, P. S. (2009). Control: Conscious and otherwise. Trends in Cognitive Sciences, 13(8), 341–347. https://doi.org/10.1016/j.tics.2009.04.010
- Suphasomboon, T., & Vassanadumrongdee, S. (2022). Toward sustainable consumption of green cosmetics and personal care products: The role of perceived value and ethical concern. Sustainable Production and Consumption, 33, 230–243. https://doi.org/10.1016/j.spc.2022.07.004
- Susser, D., &Cabrera, L. Y. (2023). Brain data in context: Are new rights the way to mental and brain privacy?. AJOB Neuroscience, 1–12. https://doi.org/10.1080/21507740.2023.2188275 37017379
- Susser, D. (2019). Invisible influence: Artificial intelligence and the ethics of adaptive choice architectures [Paper presentation]. AIES 2019 - Proceedings of the 2019 AAAI/ACM Conference on AI, Ethics, and Society (pp. 403–408). https://doi.org/10.1145/3306618.3314286
- Taghikhah, F., Voinov, A., Shukla, N., & Filatova, T. (2021). Shifts in consumer behavior towards organic products: Theory-driven data analytics. Journal of Retailing and Consumer Services, 61, 102516. https://doi.org/10.1016/j.jretconser.2021.102516
- Tarafdar, M., Teodorescu, M., Tanriverdi, H., Robert, L., & Morse, L. (2020). Seeking ethical use of AI algorithms: Challenges and mitigations.
- Tay, L., Woo, S. E., Hickman, L., Booth, B. M., & D’Mello, S. (2022). A conceptual framework for investigating and mitigating machine-learning measurement bias (MLMB) in psychological assessment. Advances in Methods and Practices in Psychological Science, 5(1), 25152459211061337. https://doi.org/10.1177/25152459211061337
- Thomas, D. M., Kleinberg, S., Brown, A. W., Crow, M., Bastian, N. D., Reisweber, N., Lasater, R., Kendall, T., Shafto, P., Blaine, R., Smith, S., Ruiz, D., Morrell, C., & Clark, N. (2022). Machine learning modeling practices to support the principles of AI and ethics in nutrition research. Nutrition & Diabetes, 12(1), 48. https://doi.org/10.1038/s41387-022-00226-y
- Thomas, A. R., Pop, A. N., Iorga, A. M., & Ducu, C. (2017). Ethics and neuromarketing: Implications for market research and business practice. Springer. https://doi.org/10.1007/978-3-319-45609-6
- Tippins, N. T., Oswald, F. L., & McPhail, S. M. (2021). Scientific, legal, and ethical concerns about AI-based personnel selection tools: A call to action. Personnel Assessment and Decisions, 7(2), 1. https://doi.org/10.25035/pad.2021.02.001
- Tirandazi, P., Bamakan, S. M. H., & Toghroljerdi, A. (2022). A review of studies on Internet of Everything as an enabler of neuromarketing methods and techniques. The Journal of Supercomputing, 79(7), 7835–7876. https://doi.org/10.1007/s11227-022-04988-1
- Tiutiu, M., & Dabija, D. C. (2023). Improving consumer experience using artificial intelligence in online retail. Proceedings of the International Conference on Business Excellence, 17(1), 1139–1147. (https://doi.org/10.2478/picbe-2023-0102
- Tolmeijer, S.,Kneer, M.,Sarasua, C.,Christen, M., &Bernstein, A. (2021). Implementations in machine ethics. ACM Computing Surveys, 53(6), 1–38. https://doi.org/10.1145/3419633
- Torbert, P. M. (2021). “Because it is wrong”: An essay on the immorality and illegality of the online service contracts of Google and Facebook. Journal of Law, Technology, & the Internet, 12(1), 2.
- Trakman, L., Walters, R., & Zeller, B. (2019). Is privacy and personal data set to become the new intellectual property? IIC - International Review of Intellectual Property and Competition Law, 50(8), 937–970. https://doi.org/10.1007/s40319-019-00859-0
- Trettel, A., Cherubino, P., Cartocci, G., Rossi, D., Modica, E., Maglione, A. G., … Babiloni, F. (2017). Transparency and reliability in neuromarketing research. In Ethics and neuromarketing (pp. 101–111). Springer.
- Tsamados, A., Aggarwal, N., Cowls, J., Morley, J., Roberts, H., Taddeo, M., & Floridi, L. (2021). The ethics of algorithms: key problems and solutions. In Ethics, Governance, and Policies in Artificial Intelligence (pp. 97–123).
- Ulman, Y. I., Cakar, T., & Yildiz, G. (2015). Ethical issues in neuromarketing: “I consume, therefore I am!”. Science and Engineering Ethics, 21(5), 1271–1284. https://doi.org/10.1007/s11948-014-9581-5
- Ulnicane, I. (2022). Artificial intelligence in the European Union: Policy, ethics, and regulation. In The Routledge handbook of european integrations. Taylor and Francis.
- UN News Global Perspective Human Stories. (2020, December 30). Bias, racism, and lies: Facing up to the unwanted consequences of AI. UN News: https://news.un.org/en/story/2020/12/1080192.
- Van Bekkum, M., & Borgesius, F. Z. (2023). Using sensitive data to prevent discrimination by artificial intelligence: Does the GDPR need a new exception? Computer Law & Security Review, 48, 105770. https://doi.org/10.1016/j.clsr.2022.105770
- Van Den Meerssche, D. (2022). Virtual borders: International law and the elusive inequalities of algorithmic association. European Journal of International Law, 33(1), 171–204. https://doi.org/10.1093/ejil/chac007
- Velkova, I. (2023). Unstructured social media data processing with artificial intelligence. Industry 4.0, 8(2), 65–67.
- Venkatraman, V., Dimoka, A., Pavlou, P. A., Vo, K., Hampton, W., Bollinger, B., Hershfield, H. E., Ishihara, M., & Winer, R. S. (2015). Predicting advertising success beyond traditional measures: New insights from neurophysiological methods and market response modeling. Journal of Marketing Research, 52(4), 436–452. https://doi.org/10.1509/jmr.13.0593
- Verhulst, N., De Keyser, A., Gustafsson, A., Shams, P., & Van Vaerenbergh, Y. (2019). Neuroscience in service research: An overview and discussion of its possibilities. Journal of Service Management, 30(5), 621–649. https://doi.org/10.1108/JOSM-05-2019-0135
- Vogel, C., Zwolinsky, S., Griffiths, C., Hobbs, M., Henderson, E., & Wilkins, E. (2019). A Delphi study to build consensus on the definition and use of big data in obesity research. International Journal of Obesity (2005), 43(12), 2573–2586. https://doi.org/10.1038/s41366-018-0313-9
- Voigt, PV., & Dem Bussche, A. (2017). The EU general data protection regulation (GDPR). A practical guide (1st ed.). Springer International Publishing (Vol. 10, pp. 10–5555).
- Waldman, A. E. (2019). Privacy law’s false promise. Washington University Law Review, 97, 773.
- Wang, H. (2022). Transparency as manipulation? Uncovering the disciplinary power of algorithmic transparency. Philosophy & Technology, 35(3), 69. https://doi.org/10.1007/s13347-022-00564-w
- Wexler, A., & Thibault, R. (2019). Mind-reading or misleading? Assessing direct-to-consumer electroencephalography (EEG) devices marketed for wellness and their ethical and regulatory implications. Journal of Cognitive Enhancement, 3(1), 131–137. https://doi.org/10.1007/s41465-018-0091-2
- Wicinski, S. (2022). A historical and practical analysis for neuromarketing in business practices today.
- Wilden, R., Hohberger, J., Devinney, T. M., & Lumineau, F. (2019). 60 Years of March and Simon’s organizations: An empirical examination of its impact and influence on subsequent research. Journal of Management Studies, 56(8), 1570–1604. https://doi.org/10.1111/joms.12531
- Winfield, A. F., Michael, K., Pitt, J., & Evers, V. (2019). Machine ethics: The design and governance of ethical AI and autonomous systems [scanning the issue]. Proceedings of the IEEE, 107(3), 509–517. https://doi.org/10.1109/JPROC.2019.2900622
- Wirtz, J., Kunz, W. H., Hartley, N., & Tarbit, J. (2023). Corporate digital responsibility in service firms and their ecosystems. Journal of Service Research, 26(2), 173–190. https://doi.org/10.1177/10946705221130467
- Xiao, Y., & Watson, M. (2019). Guidance on conducting a systematic literature review. Journal of Planning Education and Research, 39(1), 93–112. https://doi.org/10.1177/0739456X17723971
- Yarosh, O. B., Kalkova, N. N., & Reutov, V. E. (2021). Consumer emotions when making an online purchase decision: Results of neuromarketing experiments. Upravlenec, 12(4), 42–58.
- Yin, R. K. (2014). Case study research: Design and methods (5th ed.). Sage.
- Zeng, Z., Zhang, M., Chen, T., & Hong, Z. (2021). A new selection operator for differential evolution algorithm. Knowledge-Based Systems, 226, 107150. https://doi.org/10.1016/j.knosys.2021.107150
- Zhu, S., Qi, J., Hu, J., & Hao, S. (2022). A new approach for product evaluation based on integration of EEG and eye-tracking. Advanced Engineering Informatics, 52, 101601. https://doi.org/10.1016/j.aei.2022.101601
- Zhu, C., & Lehot, L. (2022). US: Artificial intelligence comparative guide, Foley and Lardner LLP, Mondaq. Retrieved May 16, 2022, from https://www.mondaq.com/unitedstates/technology/1059776/artificial-intelligence-comparative-guide?
- Zulaikha, N., Amjad, M., Hussain, F., Tahir, A., & Ali, A. (2020). Advanced data analysis techniques for e-marketing: An empirical study. In Proceedings of the International Conference on E-Commerce, E-Business and E-Government (pp. 69–77). Springer.
- Zulaikha, S., Mohamed, H., Kurniawati, M., Rusgianto, S., & Rusmita, S. A. (2020). Consumer predictive analytics using artificial intelligence. The Singapore Economic Review, 66(3), 1–12. https://doi.org/10.1142/S0217590820480021