Abstract
Despite the growing popularity and commercial potential of live streaming, research on live streaming trade is still in its early stages. Moreover, previous studies have produced inconsistent results regarding the influence of digital celebrity perceptions on consumer shopping intentions. Therefore, this study aims to investigate the antecedents of live-streaming shopping intentions by integrating the Perception of Digital Celebrities with the Uses and Gratifications theory. To achieve this objective, a quantitative approach was employed, and primary data were collected from 504 Indonesian consumers through the distribution of questionnaires. The collected data were analyzed using Structural Equation Modeling based on Partial Least Squares with the aid of SmartPLS 3. The findings of this study suggest that the Perception of Digital Celebrities has a significant effect on consumers’ live-streaming shopping intentions. Additionally, Social Interaction was found to have a significant effect on live-stream shopping intention, and Social Interaction was found to mediate the relationship between the Perception of Digital Celebrities and Live-Stream Shopping Intentions.
Introduction
Indonesia is one of the countries with the largest population in the world. According to data released by We Are Social, in January 2023, Indonesia’s total population reached 276.4 million people, with 49.7% being women and 50.3% being men. In terms of internet usage, Indonesia has around 212.9 million users, which is an increase of 5.2% compared to the previous year. However, there are still about 64 million people who have not been touched by the internet (Dataareportal, Citation2023). In particular, social media in Indonesia is also very popular, with 167 million users, or 60.4% of the entire population using platforms such as Facebook, Instagram, TikTok, and others. This data provides an overview of the digital behavior of Indonesian people that continues to grow and is a very interesting thing to study further (Dataareportal, Citation2023).
E-commerce has grown rapidly in Indonesia and is considered an important part of consumers’ lives in the country. The COVID-19 pandemic accelerated changes in consumer behavior in shopping, from physical to online (Galhotra & Dewan, Citation2020). These developments offer new opportunities and challenges for businesses and consumers. According to the ‘Big Data Review of the Impact of Covid-19 2020’ report published by the Central Statistic Agency (2020), online sales in Indonesia increased sharply during the pandemic. Online sales in April 2020 increased by 480% compared to January 2020 (Pink & Laoli, Citation2020). Based on a McKinsey report, Indonesia’s e-commerce industry is projected to grow eightfold from 2017 to 2022, with total e-commerce spending increasing from $8 billion to $55–65 billion (MTarget, Citation2019). This growth of e-commerce is expected to dominate market trends in the future. Some examples of leading e-commerce in Indonesia are Tokopedia, Shopee, Bukalapak, JD.id, and Lazada.
The increasing trend of e-commerce in Indonesia should be a concern for businesspeople and consumers. The growing digital market provides both opportunities and challenges for those who want to succeed in this industry. Further studies are needed to understand the dynamics of the e-commerce market and its impact on consumers and companies in Indonesia. In an increasingly advanced digital era, business competition is getting tougher and encourages business actors to find the right media to grow and compete in the digital market. In Indonesia, internet users’ interest in digital transactions and purchases is increasing, prompting e-commerce companies to implement business development strategies that focus on digital channels. Digital marketing is a medium that is often used by e-commerce players to reach consumers. Through digital marketing, consumers can view product information and details on the internet, interact with merchants anytime and anywhere, and facilitate ordering and product comparisons (M. T. Febriyantoro & Arisandi, Citation2018; Kotller, Citation2009).
Although the growth of e-commerce with digital marketing has brought many advantages, there are some obstacles that need to be considered. One of them is the lack of presence of human and social elements in the online shopping experience, which makes the lack of customer engagement compared to shopping in offline markets (Clement Addo et al., Citation2021; Hu & Chaudhry, Citation2020). Over the past few years, online businesses have more often described their products through interactive text and images. Unfortunately, this way it is difficult for customers to touch, test, or try the goods directly and get information in real-time before making a purchase (Hu & Chaudhry, Citation2020; Steinhoff et al., Citation2019) Such a shopping experience can increase purchasing risk and create uncertainty in the minds of customers, thus hindering purchasing activities (Constantinides, Citation2004; Kozlenkova et al., Citation2017).
Solving these barriers can be achieved by integrating human and social elements into the online shopping experience. One way to overcome this is to bring interaction between customers and sellers in real-time via chat or video call. This can provide a more personalized shopping experience and allow customers to ask questions and get information in real time (Hu & Chaudhry, Citation2020). In addition, virtual and augmented reality technologies can also help customers to see and try products more clearly and interactively, thereby increasing customer confidence in making purchasing decisions (Steinhoff et al., Citation2019). In an increasingly advanced digital era, e-commerce companies must continue to innovate to create more engaging and engaging online shopping experiences for customers.
In an increasingly advanced digital era, online businesses are constantly looking for new ways to promote their products. One of the new forms of e-commerce is live streaming, which utilizes live broadcasting as a channel to achieve marketing goals. Live streaming or also called live e-commerce has opened new avenues for businesses and consumers to remove barriers to distance and time and increase social presence in e-commerce. This opens up new opportunities for businesses to increase sales and increase consumer engagement in the buying process (Qian, Citation2021).
According to several studies, live streaming provides a more personalized, visual, and interactive shopping experience for consumers. In live streaming, consumers can view products live through live video broadcasts guided by live-stream hosts. Consumers can also interact with the host and provide comments or questions while making purchases directly through e-commerce platforms. Live streaming allows consumers to get closer to a product, seeing and hearing streamers describe what the quality, appearance, or way of wearing a product is like (Farman, Citation2019; Hu & Chaudhry, Citation2020). However, even though live streaming is becoming increasingly popular, there is still little research being done to reveal what factors influence consumers’ intention to live-stream shopping on e-commerce platforms. Therefore, research on the factors that influence consumers’ intention to do live-streaming shopping on e-commerce platforms is important to do (Clement Addo et al., Citation2021; Zafar et al., Citation2021).
Live stream in e-commerce has become an increasingly popular trend in recent times because it provides benefits in overcoming distance and time constraints and increasing social presence in e-commerce (Clement Addo et al., Citation2021). In live streaming, streamers can display products as well as themselves by doing various interesting activities such as talking, eating, and others, so streamers function as sources of information and contributors who display products in real-time (Kang et al., Citation2021). Streamers show product information, how products are developed and used, provide information that clarifies customer confusion, and demonstrate hands-on activities that motivate customers to buy (Lu et al., Citation2018).
Customers as co-creators can interact with streamers and fellow customers through chatboxes in real-time, and these interactions can affect broadcasts and streamers (Kang et al., Citation2021; Sjöblom et al., Citation2017). In addition, customers can send virtual gifts as support to streamers, so streamers become increasingly motivated and encouraged to provide better product information and presentation (Yu et al., Citation2018). Live streaming activities create interactions between streamers and customers that can reduce customer concerns and increase customer purchase intentions (Zhang et al., Citation2020a; Citation2020b).
In the live streaming trade, interesting streamers become the main content promoting commercial information in live streaming (X. Xu et al., Citation2020). As the popularity of live-streaming platforms increases, the commercial value of streamers with a large fan base continues to increase (Hill et al., Citation2020; Park & Lin, Citation2020). Streamers in live streaming can be store owners, digital celebrities, and professional streamers. When a streamer reaches a certain level of popularity, they are referred to as digital celebrities (C. C. Chen & Lin, Citation2018). According to research (R. Li, Citation2018). Digital celebrities should have some characteristics such as a large number of followers, a high level of interactivity in their profile, and promising business value. A digital celebrity as a streamer has its own streaming style, professionalism, personality, or appeal (X. Xu et al., Citation2020; Zhao et al., Citation2018). When shaping certain values and attitudes, digital celebrities act as individuals or groups that are liked by consumers and can become key opinion leaders as well as exert a great influence on social media (R. Li, Citation2018; Luo et al., Citation2021; Park & Lin, Citation2020; Schiffman & Cohn, Citation2009).
Although several studies have been conducted to examine the influence of digital celebrities on online shopping intentions, most studies have been conducted in the context of traditional mass media or other forms of social media such as blogs or social networking services (Djafarova & Rushworth, Citation2017; Jiménez-Castillo & Sánchez-Fernández, Citation2019; Lim et al., Citation2020; Xin Jean et al., Citation2017). Little research has been done on the phenomenon of live streaming or the new types of celebrities that appear in it (Park & Lin, Citation2020). In addition, the findings of an earlier study from (Park & Lin, Citation2020). showed that the attractiveness of digital celebrities did not have a significant effect on shopping intent in the context of live streaming, which is different from previous findings by Z. Wang et al. (Citation2017), Chan et al., Citation2021). Which stated that the attractiveness of digital celebrities affects shopping intentions on live streaming. Therefore, this research is expected to add to the understanding of the influence of digital celebrity perceptions on shopping intentions in the context of live streaming.
Literature review
E-commerce
According to Khan (Citation2016) electronic commerce or e-commerce is a trading activity that involves buying and selling goods and services over the internet. In this context, the internet serves as a source of information for price comparisons or the latest products before a customer makes a purchase online or in a physical store. E-commerce is changing the way companies do business, connect with customers and suppliers, and think about marketing and logistics (Clayton & Criscuolo, Citation2002; Khan, Citation2016). Išoraitė and Miniotienė (Citation2018) states that e-commerce includes many activities, including the sale of goods and services, electronic transmission of digital information, direct sales to consumers, and electronic marketing. E-commerce allows buyers and sellers to do business on the same platform without affecting personal requirements and relationships between participants quickly (Bairagi, Citation2011; Harris & Spence, Citation2002).
Digital celebrities
Digital celebrity is a new phenomenon that has emerged thanks to the development of digital and social media (R. Li, Citation2018). They are individuals who gained fame and popularity through strong interactive abilities in communicating through online media such as Instagram, YouTube, TikTok, and others (Luo et al., Citation2021; Sun et al., Citation2019). According to R. Li (Citation2018) digital celebrities should have characteristics such as the number of followers, a high level of interactivity in their profile, and promising business value. Digital celebrities are also known as individuals with effective and competent social skills in a particular field (Katz et al., Citation2017; Ma, Citation2021). They are recognized as experts in social media platforms (Khamis et al., Citation2017).
Researchers have used various labels to describe new types of celebrities emerging through social media, such as social media influencers (Freberg et al., Citation2011; Glucksman, Citation2017). Influential bloggers (Uzunoğlu & Misci Kip, Citation2014), Celebgrams, and famous Instagram bloggers (Maghfiroh & Hapsari, Citation2015; Marwick, Citation2015a), or YouTube stars (Marwick, Citation2015b). However, they all have one thing in common, which is the influence gained through the use of digital, which makes them called digital celebrities (CBN Data, Citation2016; R. Li, Citation2018). When a streamer reaches a certain level of popularity, he can have the same effect as other celebrities and is often referred to as a digital celebrity (C. C. Chen & Lin, Citation2018).
Social interaction
Social interaction is a fundamental human need and is a very important part of human life (Ma, Citation2021; Yin et al., Citation2019). According to the theory of social interaction first introduced by Blau (Citation1964). The interaction between individuals is based on an analysis of personal costs and benefits, in which individuals seek to establish social relationships in order to obtain more benefits (Ghahtarani et al., Citation2020). Social interaction is also an important factor that facilitates the continued use of social media (Cheung et al., Citation2015; J. C. Wang & Chiang, Citation2009) and triggers purchase intentions in e-commerce, especially in live-streaming shopping (Ma, Citation2021; Zhou et al., Citation2016).
Interaction between users in live streaming has also proven to be a significant factor in influencing consumer intentions. A study by Hou et al. (Citation2020) showed that social interactivity can influence an individual’s intention to watch live streaming. In addition, Hsu et al. (Citation2020) found that hospitality can predict consumer loyalty to live-streaming channels. With social interactions, live-streaming platforms can facilitate the flow of information and resources, thereby increasing public knowledge about the products and services offered by sellers (Ghahtarani et al., Citation2020).
Live stream shopping intention
E-commerce live streaming is a new form of e-commerce that uses live broadcasting as a channel to achieve marketing goals (Qian, Citation2021). It involves live streaming platforms, technology, and live streaming infrastructure to provide a virtual network environment that enables real-time interaction, entertainment, social activities, and business with unhindered available leads (X. Xu et al., Citation2020). Live streaming has opened up new avenues for businesses and consumers to remove barriers to distance and time (such as face-to-face or real-time human interaction) and increase social presence in e-commerce Clement Addo et al. (Citation2021) This allows consumers to get closer to a product, see and hear streamers tell what the quality, appearance, or how to use a product is like (Farman, Citation2019; Hu & Chaudhry, Citation2020). Therefore, live streaming creates visual, authentic, and interactive experiences in online shopping (Hu & Chaudhry, Citation2020).
According to Blackwell et al. (Citation2001), shopping intention is the consumer’s plan to choose the place of purchase of a product. Shopping intent can be classified as one of the components of the cognitive behavior of consumers about how a person intends to buy products and services in a certain period of time (Kwek et al., Citation2010). Dodds et al. (Citation1991) define shopping intent as a consumer’s willingness to purchase a product or service. This research focuses on consumer spending intentions in e-commerce live streaming. Therefore, ‘live-stream shopping intentions’ in this study are defined as consumers’ desire and willingness to buy products or services through live streaming.
The effect of perception of digital celebrities on live-stream shopping intentions
In this context, perceptions of digital celebrities can be used as heuristic clues (Ma, Citation2021; Todorov et al., Citation2012). A digital celebrity as a streamer in live streaming has distinctive characteristics such as streaming style, professionalism, personality, or attractiveness that varies, and can influence others and act as an opinion leader (Lakhan et al., Citation2021; X. Xu et al., Citation2020; Zhao et al., Citation2018). According to Gräve (Citation2017) perceptions of digital celebrities can influence the effectiveness of endorsers which will ultimately impact customers’ attitudes towards brands and shopping intentions. The attractiveness and influence of digital celebrities is also important in attracting consumers to use the live streaming trading environment (Cai et al., Citation2018; X. Xu et al., Citation2020). Research by De Veirman et al. (Citation2017) shows that influencers with large follower counts tend to be more popular and likable. The number of followers and the perception of popularity also increase the perception of influencer opinion leadership (De Veirman et al., Citation2017; Ladhari et al., Citation2020; Ma, Citation2021). Therefore, in the context of live-stream shopping, perceptions of digital celebrities can influence consumers’ spending intentions. The hypotheses proposed are:
H1: Perception of Digital Celebrities has a significant influence on Live-Stream Shopping Intentions.
The effect of perception of digital celebrities on social interaction
Digital celebrities often have effective social skills and high interactivity on social media (Katz et al., Citation2017; R. Li, Citation2018). They are also considered to have high familiarity and are able to attract more follower interactions than traditional endorsers (Gräve, Citation2017; C. W. Chen et al., Citation2016; S. Chen et al., Citation2016). According to Hu et al. (Citation2017) the intimacy of social interaction between digital celebrities and viewers can be formed because digital celebrities often respond to viewers’ comments in a timely manner and pay attention to the viewer’s viewing experience and personal feelings.
In live streaming, viewers can interact with preferred digital celebrities as well as with other viewers and enjoy fun social communication (Zhao et al., Citation2018; X. Xu et al., Citation2020). Competent digital celebrities can maintain and enhance the atmosphere in the live room by responding to viewers’ questions, suggestions, and responses in real-time, as well as providing clues based on the viewer’s individual concerns, such as making jokes or singing X. Xu et al. (Citation2020) In addition, digital celebrities can also share feelings about their own lives and work with viewers, so viewers can feel they have a virtual connection and perception with the host (Hu et al., Citation2017). Direct cues and feedback can also provide social satisfaction to viewers in live streaming (Tseng et al., Citation2019) The hypotheses proposed are:
H2: Perception of Digital Celebrities has a significant influence on Social Interaction
The effect of social interaction on live-stream shopping intentions
Studies on media richness show that simultaneous visual interactions increase interactions between digital celebrities and viewers (Liao et al., Citation2020). According to Hou et al. (Citation2020) social interactivity in a live-streaming environment can affect an individual’s intention to watch. In addition, Hsu et al. (Citation2020) found that digital celebrity friendliness can predict consumer loyalty to live-streaming channels.
In the context of e-commerce, good interaction between streamers and consumers can reduce consumer concerns and increase their purchase intentions (Zhang et al., Citation2020a; Citation2020b). In an enthusiastic and engaging shopping environment, consumer emotions can be extensively triggered, which can encourage impulsive consumption (X. Xu et al., Citation2020; Ma (Citation2021) in her research states that consumers who expect higher interaction with digital celebrities and other viewers tend to be more engaged and shop on live streams. The hypotheses proposed are:
H3: Social Interaction has a significant impact on Live-Stream Shopping Intentions.
Social interaction mediates the relationship between the perception of digital celebrities and live-stream shopping intentions
Interactions between individuals can be an important channel in the flow of information and resources, which can increase people’s knowledge of products and services as well as influence the sharing behavior of knowledge or user information (Ghahtarani et al., Citation2020). In the context of live streaming, digital celebrities can provide explanations on how to use the product and provide clear information to solve customer confusion directly Lu et al. (Citation2018) In addition, digital celebrities tend to attract more follower interactions than traditional endorsers and have a high familiarity with their followers (Gräve, Citation2017; C. W. Chen et al., Citation2016; S. Chen et al., Citation2016). In e-commerce, good interaction between streamers and consumers can reduce customer concerns and increase customer purchase intentions () (Zhang et al., Citation2020a; Citation2020b) Therefore, the hypotheses proposed are:
H4: Social Interaction mediates the relationship between the Perception of Digital Celebrities and Live-Stream Shopping Intentions.
Method
This research employed a quantitative approach, which is based on positivist philosophy and uses instruments to collect data for examining a specific population or sample, with statistical analysis of data (Sugiyono, Citation2015). The research population comprised people in Jakarta and surrounding areas who have watched live-streaming sales. Non-probability sampling was utilized as the sampling method, specifically purposive sampling, as the research had specific criteria to investigate. The researcher established certain criteria that the sample must meet, such as having watched live-streaming sales. Primary data was collected through online questionnaires distributed via social media platforms such as Instagram and WhatsApp. The study focused on live-stream sales on various e-commerce platforms, including Instagram, Facebook, TikTok, Shopee, Lazada, and others. Celebrities that were included had certain characteristics, such as a certain number of followers, high interactivity levels on their profiles, and promising business value. Determining an adequate sample size for an unknown population presents inevitable challenges for researchers. With no precise information on the actual population size, it is arduous to ascertain the minimum percentage or proportion of subjects that necessitate sampling. However, general guidelines established by seminal academics such as Hair et al. (Citation2017) provide invaluable direction.
Despite the vagueness of total population size, the prescribed threshold that factor analysis necessitates a minimum sample of 50 and a generally accepted level of 100 samples remains applicable. This delineates the baseline standard for statistical validity of all research findings. Additionally, conserving the conventional 10:1 subject-to-variable ratio continues prudent practice; with 5 variables slated for analysis, the requisite sample size is 50 subjects. Naturally, larger samples engender enhanced results, but pragmatic constraints on resources must temper aspirations. Within this study, there are 12 indicators multiplied by 10 as recommended by the 10:1 subject-to-variable ratio rule, resulting in a minimum sample size of 120 subjects. However, 504 respondents were surveyed, surpassing the minimum 100-subject threshold for unknown populations. Thus, the chosen sample size is considered adequately robust, and the resultant findings may be generalized to the broader population with statistical confidence.
Descriptive statistical analysis was used to provide respondent characteristics presented in tabular form. Inferential analysis and hypothesis testing were performed using a Partial Least Squares Structural Equation Modeling (PLS-SEM) method, which involved two evaluation stages: the outer model and the inner model. The outer model was used to determine the relationship between latent variables and their indicators, including testing for convergent and discriminant validity and reliability. The inner model was used to predict the causality relationships between latent variables and involved testing R-square and bootstrapping. Smartpls 3.2.9 software was used for data processing.
Result and discussion
In a sample of 504 respondents, there were 285 women (56.6%) and 219 men (43.4%). Of the 285 women, 201 (70.5%) have accessed live-streaming sales. Similarly, of the 219 men, 154 (70.3%) have accessed live-streaming sales. This shows that many of both women and men have accessed live streaming sales, with very little difference between the percentages. This suggests that accessing live streaming sales has become common for both genders. Some factors that make live streaming appealing regardless of gender.
In the total sample of 504 respondents, the majority that accessed live streaming sales were in the 18–35 age brackets. Specifically, 274 out of the 504 respondents were aged 18–35 years old (54.4% of the sample). This indicates that more than half of the respondents who have watched live stream sales are millennials and early Gen Z. The high percentage of young adults accessing these live streams aligns with their greater comfort with technology, social media engagement, and interest in the products promoted like fashion/beauty deals. The interactive nature of live streams is also more appealing and familiar for those in this age group compared to traditional advertisements. Factors like pricing ease of mobile access, and influencer marketing also likely contribute to the popularity of live commerce amongst 18–35-year-old consumers.
Outer model
This research employed SmartPLS as the data analysis technique, which consists of three evaluation stages to assess the outer model, including Convergent Validity, Discriminant Validity, and Composite Reliability. The first stage, Convergent Validity, is used to test whether the observed variables in the measurement model converge to their respective latent variables. The second stage, Discriminant Validity, is used to examine the extent to which each latent variable is distinct from other latent variables in the model. Finally, Composite Reliability is used to evaluate the reliability of the latent variables in the model. These stages are important in ensuring the validity and reliability of the research model and ensuring that the data analysis process is accurate and trustworthy ().
Convergent validity
Ghozali (Citation2013) stated that to test the validity of a survey questionnaire, a validity check was conducted. A survey can be considered valid if the analysis reveals meaningful results, which is indicated by a loading factor above 0.6. To test the outer model, SmartPLS data analysis techniques require three criteria to be met: Convergent Validity, Discriminant Validity, and Composite Reliability. Convergent validity was assessed by evaluating the correlation between the score of the construct and the score of its components calculated by the PLS Software. A reflexive measure is considered high if it is correlated with the construct it measures by more than 0.70. (Chin, Citation1998) suggested that loading values of 0.5 to 0.6 are sufficient for developing a measurement scale in the early stages of research. For this study, a loading factor of 0.60 was chosen as the threshold ().
Table 2. Convergent validity test.
Based on the results of the SmartPLS analysis, it was found that the loading factor values of some items in PODS5, PODS6 were below 0.6. This indicates that these items needed to be removed and retested to increase their validity. The reason for removing these items is that their low loading factor values indicate that they were not effective in measuring the intended variables and did not have a strong correlation with them.
After retesting, the highest loading factor value was found in the perception of digital celebrities (PODS) variable for PODS2 with a loading factor value of 0.773. This suggests that the items in PODS2 were strongly correlated with the intended variable and can be relied upon for measuring it. Similarly, for the social interaction (SI) variable, the highest loading factor value was found in SI2 with a loading factor value of 0.888. This suggests that the items in SI2 were strongly correlated with the intended variable and can be relied upon for measuring it. Finally, for the live-stream shopping intention (LSSI) variable, the highest loading factor value was found in LSSI2 with a loading factor value of 0.914. This suggests that the items in LSSI2 were strongly correlated with the intended variable and can be relied upon for measuring it. The validity of all variables was confirmed based on the Average Variance Extract (AVE) values, which were above 0.5. This indicates that the variables were able to explain more than 50% of their respective constructs, suggesting that they were valid indicators of the constructs they were intended to measure.
Based on the two convergence validity tests, loading factor and AVE, all indicators and variables met the convergence validity criteria. The loading factor values for each indicator were above the recommended threshold of 0.6, indicating that each indicator had a strong and significant relationship with its respective construct. Meanwhile, the AVE values for each variable were above the recommended threshold of 0.5, indicating that each variable was able to explain more than 50% of the variance in its respective construct. This suggests that the variables were valid indicators of the constructs they were intended to measure.
Discriminant validity
Discriminant validity is the extent to which two supposedly distinct constructs have significant differences in a statistical sense. The Fornell-Larcker criterion is a popular method of assessing the discriminant validity of constructs in measurement models. The Fornell-Larcker criterion suggests that the square root of the average extracted variance (AVE) for each construct must be greater than the correlation between that construct and other constructs in the model. In other words, the shared variance between two constructs must be smaller than the variance described by each of the constructs themselves (Hair et al., Citation2017) ().
Table 3. Fornell-Larcker Test.
Based on the Fornell-Larcker results showed that each variable has a higher loading coefficient or correlation with the corresponding construct than with the construct or other variable. Thus, it can be concluded that each variable can distinguish itself from other variables in the study. All variables in this study have met the discriminant validity criteria based on the results of the Fornell-Larcker test, so this study can be considered to have good validity and reliable.
Reliability
Composite reliability, on the other hand, is a measure of reliability for constructs consisting of several measurement items or indicators. Composite reliability measures how consistent these items are in measuring the same construct. The higher the composite reliability value, the more reliable the construct measurement will be. Composite reliability can be calculated using factor analysis techniques or SEM.
Based on the results of composite reliability measurements that have been carried out, information was obtained that the perception of digital celebrities construct has a reliability value of 0.799, social interaction has a reliability value of 0.871, and live-stream shopping intention has a reliability value of 0.908. This shows that the items in each construct are consistent in measuring the same variables, and these measurements are reliable. From these results, it can be concluded that the study used reliable and valid measurement instruments in measuring the constructs studied. Thus, the results of such research are reliable and can be interpreted accurately.
Inner model (structural)
Upon completion of the analysis of the outer model and confirmation of its fulfillment of the research criteria, the subsequent step involves testing the structural model. The structural model provides insight into the predictive relationship between latent variables within the research model. An R-square test is an effective measure to evaluate the level of goodness of the structural model. This measure assesses the degree of influence that independent latent variables have on the dependent latent variable. An R-square value of 0.75 or higher is indicative of a strong model, while a value of 0.50 indicates a moderate model, and a value of 0.25 or lower indicates a weak model (Ghozali & Latan, Citation2015) ().
Based on the results of the R-Square test, it was found that the value of R-Square in the social interaction variable was 0.312. It can be interpreted that the perception of digital celebrities’ variable has an influence of 31.2% on social interaction, while the rest is influenced by other variables that were not studied in this study. Meanwhile, in the live stream shopping intention variable, the R-Square value was found to be 0.397. This means that the perception of digital celebrities and social interaction variables had an influence of 39.7% on live stream shopping intention, while the influence of the rest was influenced by other variables that were not studied in this study ().
Table 4. Bootstrapping test.
Based on the SmartPLS bootstrapping measurement, it was found that there is a positive influence between perception of digital celebrities and live stream shopping intention, with a path coefficient value of 0.147 and a P-value of 0.000. This result indicates that the higher the level of perception of digital celebrities, the greater the tendency to engage in live stream shopping. Previous research findings (Chetioui et al., Citation2020, Jiménez-Castillo & Sánchez-Fernández, Citation2019; Zhaoxing et al., Citation2018) have shown that digital celebrities have a positive influence on consumer shopping intention. These findings are consistent with the results of this study, which demonstrate a positive influence of perception of digital celebrities on live stream shopping intention. The interest in online shopping continues to increase, especially during the COVID-19 pandemic which has limited physical access to stores and malls. Live stream shopping has become an increasingly popular alternative for Indonesian consumers to shop online. Therefore, understanding the factors that influence live stream shopping intention in Indonesia is important. Perception of digital celebrities can be leveraged to increase interest and intention to shop via live streams among Indonesian consumers.
Furthermore, a significant influence was found between perception of digital celebrities and social interaction, with a path coefficient value of 0.558 and a P-value of 0.000. This indicates that the higher the level of perception of digital celebrities, the greater the likelihood of social interaction. The perception of digital celebrities significantly influences social interaction. This result is consistent with previous studies on the motivation of live-stream users C. C. Chen and Lin (Citation2018) as well as studies by Hu et al. (Citation2017), Ma (Citation2021). When the perception of digital celebrities is high, it encourages viewers to interact during live streams, thereby increasing the perceived social satisfaction during the live streaming experience. According to C. C. Chen and Lin (Citation2018) when the perception of digital celebrities is high, viewers are more likely to interact with them during live streams. The more famous the digital celebrity, the more positive the attitude of the viewers toward them. This enhances the viewers’ perception of the digital celebrity’s suitability and encourages them to interact during the live stream. In relation to consumers in Indonesia, live stream shopping viewers tend to be more interested in and trusting of recommendations given by more famous digital celebrities. The popularity and public acceptance of a digital celebrity builds credibility in the eyes of Indonesian consumers. If Indonesian consumers feel a digital celebrity is among important and trustworthy people, they are more likely to be drawn to watch their shopping live stream and even purchase the recommended products.
Meanwhile, a positive influence was found between social interaction and live stream shopping intention, with a path coefficient value of 0.536 and a P-value of 0.000. This result indicates that the higher the level of social interaction, the higher the tendency to engage in live-stream shopping. Social interaction significantly influences Live-Stream Shopping Intentions. This result is not surprising as live stream shopping is essentially characterized by high levels of interaction and engagement (X. Xu et al., Citation2020). This finding is consistent with the results of Hou et al. (Citation2020) on the continuous intention to watch and consume during live streaming. Similarly, (Ma, Citation2021; C. Wang et al., Citation2017; Zhou et al., Citation2016) have stated that social interactivity is a significant indicator of purchase intention. In terms of e-commerce, good interaction between digital celebrities and consumers can reduce customer concerns and increase their purchasing intentions (Zhang et al., Citation2020a; Citation2020b). By facilitating interaction with digital celebrities, live stream commerce creates a socially friendly shopping context, making viewers believe that digital celebrities, products, and other information provided are more reliable and less risky (Hu et al., Citation2017).
Additionally, it was found that social interaction mediates the relationship between the perception of digital celebrities and live stream shopping intention, with a path coefficient value of 0.299 and a P-value of 0.001. This means that social interaction plays a mediating role between the perception of digital celebrities and live stream shopping intention. These findings provide an important contribution to expanding the understanding of the factors that influence live stream shopping intention in the context of consumer behavior. Further research can be conducted to deepen the understanding of these factors and develop a more comprehensive conceptual model.
Conclusion
Based on the results of the analysis, it was found that perception of digital celebrities has a positive effect on both live-stream shopping intention and social interaction. Additionally, social interaction also has a positive effect on live stream shopping intention. Furthermore, social interaction was found to mediate the relationship between perception of digital celebrities and live stream shopping intention.
The influence of digital celebrities on consumer shopping intentions indicates that they are increasingly seen as credible and relatable endorsers that resonate with modern audiences. As social media platforms continue to reshape the celebrity landscape, digital celebrities and influencers that accumulate immense following wield substantial persuasive impact. Their association with specific products or brands heightens awareness, shapes positive brand image, and induces greater willingness amongst followers to purchase the promoted items.
Moreover, the revealed role of social interaction as a mediator reinforces live streaming not just as a convenient alternative distribution channel but a portal for consumers to immerse within participative communities. The ability to interact in real-time accentuates the feeling of inclusivity and shared experience, consequently enforcing purchasing decisions. As COVID-19 alters consumption habits with temporary store closures and movement restrictions, consumers deprived of social connections increasingly embrace live streaming’s distinctive convergence of entertainment, engagement, and transaction.
Hence, leveraging digital celebrities within interactive live streams uniquely caters to contemporary consumer preferences for more personalized and participative shopping. Their marketability, coupled with the viral features of live streaming will further propagate this emerging retail channel even amidst post-pandemic market recovery and stabilize its position within the broader e-commerce landscape. Retailers must continue enhancing live stream capabilities and optimizing celebrity partnerships to stimulate both social interaction and commercial outcomes simultaneously.
Disclosure statement
No potential conflict of interest was reported by the author(s).
Table 1. Variables and indicators.
Additional information
Notes on contributors
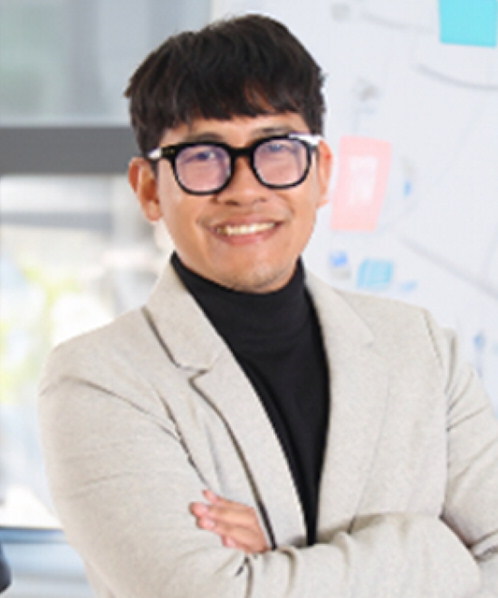
Mohamad Trio Febriyantoro
Mohamad Trio Febriyantoro is a lecturer in the Management Study Program at Universitas Pembangunan Jaya Tangerang. He earned his PhD in Technology Management from Universiti Tun Husein Onn Malaysia (UTHM). His research interests include marketing management, entrepreneurship, innovation, and business sustainability.
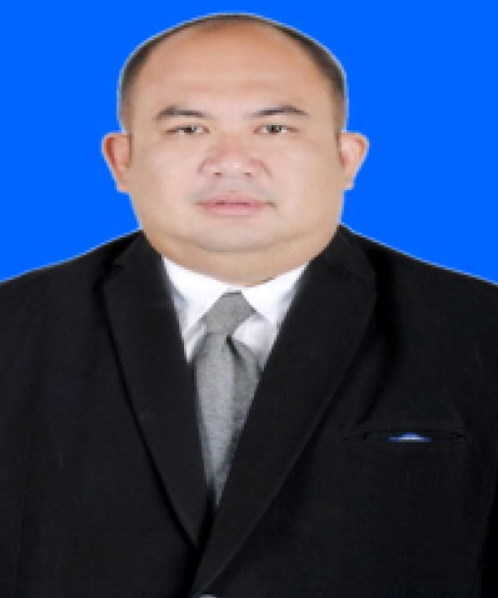
Tirta Mulyadi
Tirta Mulyadi is a lecturer in the Management Culinary at Batam tourism Polytechnic, Study Master Program at NHI Bandung tourism polytechnic. His research interests include marketing management, Tourism entrepreneurship, innovation, and business sustainability.

Eka Hendrayani
Eka Hendrayani is a lecture in the accountancy Study Program at Haji Agus Salim Institute of Technology and Business Bukittinggi. Her research interests include marketing management, entrepreneurship, innovation, and business sustainability.

Rifadli D. Kadir
Kraugusteeliana Kraugusteeliana is a lecturer in the Faculty of Computer Science at Universitas Pembangunan Nasional ‘Veteran’ Jakarta. She earned her Master in University Budi Luhur. Her research interests include Audit Information system, Egoverment, Etourism, and IT Risk Management.)
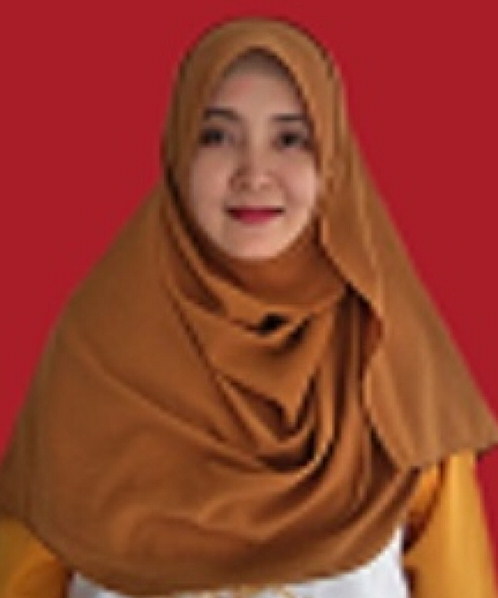
Kraugusteeliana Kraugusteeliana
Hersa Farida Qoriani is a lecturer in the Faculty of Economics and Business at Universitas Islam Negeri Kiai Haji Achmad Siddiq Jember. She earned her Doctor in Syariah Economics from UIN Sunan Ampel Surabaya. Her research interests include Islamic Economic, and Islamic Financial Management.

Hersa Farida Qoriani
Achmad Fageh is a lecturer in the Department of Shariah Economic Master’s Program at Universitas Islam Negeri Sunan Ampel. He earned his Doctor in Islamic Studies from UIN Sunan Ampel Surabaya. His research interests include Islamic Economic, Islamic Law, Jurisprudence, Digital Economic and Maqasid Syariah.

Achmad Fageh
Rifadli D. Kadir is a lecturer in the Department of Islamic Economics, Faculty Islamic Economics and Business, IAIN Sultan Amai Gorontalo, Indonesia. He is a Doctoral student in Economics at Brawijaya University. His research interests include Economics, Islamic Finance, Islamic Banking and Islamic Economics.
References
- Agency, C. S. (2020). Big Data Review of the Impact of Covid-19.
- Bairagi, A. K. …, (2011). Utilization of E-commerce can change the auction culture of Bangladesh specially in public sector. International Journal of Computer Science and, 02(01).
- Blackwell, R. D., Miniard, P. W., & Engel, J. F. (2001). Consumer behavior (9th ed.) South-Western Thomas Learning.
- Blau, P. (1964). Power and exchange in social life. International Journal of Business and Social Science.
- Cai, J., Wohn, D. Y., Mittal, A., & Sureshbabu, D. (2018 Utilitarian and hedonic motivations for live streaming shopping [Paper presentation]. TVX 2018 - Proceedings of the 2018 ACM International Conference on Interactive Experiences for TV and Online Video,.
- CBN Data. (2016). Wanghong economy Report of 2016.
- Chan, L. Q., Kong, Y. M., Ong, Z. Y., Toh, J. X., Von, Y. H., Lee, V. H., Loh, X. M., & Tan, G. W. H. (2021). Driving factors towards live-stream shopping in Malaysia. Lecture Notes in Networks and Systems, 299.
- Chen, S., Glass, D. H., & McCartney, M. (2016). Characteristics of successful opinion leaders in a bounded confidence model. Physica A: Statistical Mechanics and Its Applications, 449, 426–436.
- Chen, C. C., & Lin, Y. C. (2018). What drives live-stream usage intention? The perspectives of flow, entertainment, social interaction, and endorsement. Telematics and Informatics, 35(1), 293–303.
- Chen, C. W., Yu, P. H., & Li, Y. J. (2016). Understanding group-buying websites continuous use behavior: A use and gratifications theory perspective. Journal of Economics and Management, 12(2), 177–204.
- Chetioui, Y., Benlafqih, H., & Lebdaoui, H. (2020). How fashion influencers contribute to consumers’ purchase intention. Journal of Fashion Marketing and Management, 24(3).
- Cheung, C. M. K., Liu, I. L. B., & Lee, M. K. O. (2015). How online social interactions influence customer information contribution behavior in online social shopping communities: A social learning theory perspective. Journal of the Association for Information Science and Technology, 66(12), 2511–2521.
- Chin, W. W. (1998). The partial least squares approach to structural equation modelling. In Marcoulides G. A. (Ed.). Modern methods for business research (Vol. 295(2), pp. 295–336 .
- Clayton, T., & Criscuolo, C. (2002). Electronic commerce and business change. Economic Trends, 583, 62–69.
- Clement Addo, P., Fang, J., Asare, A. O., & Kulbo, N. B. (2021). Customer engagement and purchase intention in live-streaming digital marketing platforms. Service Industries Journal, 41(11–12), 767–786.
- Constantinides, E. (2004). Influencing the online consumer’s behavior: The Web experience. Internet Research, 14(2), 111–126. https://doi.org/10.1108/10662240410530835
- Dataareportal. (2023). Digital 2023: Indonesia. https://datareportal.com/reports/digital-2023-indonesia
- De Veirman, M., Cauberghe, V., & Hudders, L. (2017). Marketing through Instagram influencers: The impact of number of followers and product divergence on brand attitude. International Journal of Advertising, 36(5), 798–828.
- Djafarova, E., & Rushworth, C. (2017). Exploring the credibility of online celebrities’ Instagram profiles in influencing the purchase decisions of young female users. Computers in Human Behavior, 68, 1–7.
- Dodds, W. B., Monroe, K. B., & Grewal, D. (1991). Effects of price, brand, and store information on buyers’ product evaluations. Journal of Marketing Research, 28(3), 307.
- Farman, D. (2019). January 16 Why Live Video Shopping is the Future of Ecommerce.
- Febriyantoro, M. T., & Arisandi, D. (2018). Utilization of digital marketing for micro, small and medium enterprises in the era of the ASEAN economic community. JMD: Jurnal Riset Manajemen & Bisnis Dewantara, 1(2), 61–76.
- Freberg, K., Graham, K., McGaughey, K., & Freberg, L. A. (2011). Who are the social media influencers? A study of public perceptions of personality. Public Relations Review, 37(1), 90–92.
- Galhotra, B., & Dewan, A. (2020). Impact of COVID-19 on digital platforms and change in E-commerce shopping trends. Proceedings of the 4th International Conference on IoT in Social, Mobile, Analytics and Cloud, ISMAC 2020.
- Ghahtarani, A., Sheikhmohammady, M., & Rostami, M. (2020). The impact of social capital and social interaction on customers’ purchase intention, considering knowledge sharing in social commerce context. Journal of Innovation & Knowledge, 5(3), 191–199.
- Ghozali, I. (2013). Aplikasi Analisis Multivariate dengan Program IBM SPSS 21 Update PLS Regresi. Badan Penerbit Universitas Diponegoro.
- Ghozali, I., & Latan, H. (2015). Concepts, techniques, applications using Smart PLS 3.0 for empirical research. BP Undip. Semarang.
- Glucksman, M. (2017). The rise of social media influencer marketing on lifestyle branding: A case study of Lucie fink. Elon Journal of Undergraduate Research in Communications, 8(2).
- Gräve, J. F. (2017). Exploring the perception of influencers vs. Traditional celebrities: Are social media stars a new type of endorser? [Paper presentation]. ACM International Conference Proceeding Series, Part F129683.,
- Hair, J. F., Hult, G. T. M., Ringle, C. M., & Sarstedt, M. (2017). A primer on partial least squares structural equation modeling (PLS-SEM). Sage Publications.
- Harris, L., & Spence, L. J. (2002). The ethics of ebanking. Journal of Electronic Commerce Research, 3(2).
- Hill, S. R., Troshani, I., & Chandrasekar, D. (2020). Signalling effects of Vlogger popularity on online consumers. Journal of Computer Information Systems, 60(1), 76–84.
- Hou, F., Guan, Z., Li, B., & Chong, A. Y. L. (2020). Factors influencing people’s continuous watching intention and consumption intention in live streaming: Evidence from China. Internet Research, 30(1), 141–163.
- Hsu, C. L., Lin, J. C. C., & Miao, Y. F. (2020). Why are people loyal to live stream channels? The perspectives of uses and gratifications and media richness theories. Cyberpsychology, Behavior, and Social Networking, 23(5), 351–356.
- Hu, M., & Chaudhry, S. S. (2020). Enhancing consumer engagement in e-commerce live streaming via relational bonds. Internet Research, 30(3), 1019–1041.
- Hu, M., Zhang, M., & Wang, Y. (2017). Why do audiences choose to keep watching on live video streaming platforms? An explanation of dual identification framework. Computers in Human Behavior, 75, 594–606.
- Išoraitė, M., & Miniotienė, N. (2018). Electronic commerce theory and practice. IJBE (Integrated Journal of Business and Economics), 2(2), 73.
- Jiménez-Castillo, D., & Sánchez-Fernández, R. (2019). The role of digital influencers in brand recommendation: Examining their impact on engagement, expected value and purchase intention. International Journal of Information Management, 49, 366–376.
- Kang, K., Lu, J., Guo, L., & Li, W. (2021). The dynamic effect of interactivity on customer engagement behavior through tie strength: Evidence from live streaming commerce platforms. International Journal of Information Management, 56, 102251.
- Katz, E., Lazarsfeld, P. F., & Roper, E. (2017). Personal influence: The part played by people in the flow of mass communications. In Personal influence: The part played by people in the flow of mass communications.
- Khamis, S., Ang, L., & Welling, R. (2017). Self-branding, ‘micro-celebrity’ and the rise of social media influencers. Celebrity Studies, 8(2), 191–208.
- Khan, A. G. (2016). Electronic commerce: A study on benefits and challenges in an emerging economy. Type: Double Blind Peer Reviewed International Research. Journal Publisher : Global Journals Inc. 16.
- Kotller, K. (2009). Marketing management. Jilid 1. Edisi 13 Jakarta:Erlangga. In Jakarta.
- Kozlenkova, I. V., Palmatier, R. W., Fang, E., Xiao, B., & Huang, M. (2017). Online relationship formation. Journal of Marketing, 81(3), 21–40.
- Kwek, C. L., Tan, H. P., & Lau, T. C. (2010). Investigating the shopping orientations on online purchase intention in the e-commerce environment: A Malaysian study. Journal of Internet Banking and Commerce, 15(2).
- Ladhari, R., Massa, E., & Skandrani, H. (2020). YouTube vloggers’ popularity and influence: The roles of homophily, emotional attachment, and expertise. Journal of Retailing and Consumer Services, 54, 102027.
- Lakhan, G. R., Ullah, M., Channa, A., Abbas, M., & Khan, M. A. (2021). Factors effecting consumer purchase intention : Live streaming commerce. Psychology and Education, 58.
- Li, R. (2018 The secret of internet celebrities: A qualitative study of online opinion leaders on Weibo [Paper presentation]. Proceedings of the Annual Hawaii International Conference on System Sciences, 2018-January.
- Liao, G. Y., Huang, T. L., Cheng, T. C. E., & Teng, C. I. (2020). Impacts of media richness on network features and community commitment in online games. Industrial Management & Data Systems, 120(7), 1361–1381.
- Lim, Y. S., Ng, T. H., & Hng, Y. S. (2020). Internet celebrities: How they effect the purchase intention of generation Y in cyberspace? Journal of Information System and Technology Management, 5(19), 51–59.
- Lu, Z., Xia, H., Heo, S., & Wigdor, D. (2018). You watch, you give, and you engage: A study of live streaming practices in China [Paper presentation]. Conference on Human Factors in Computing Systems - Proceedings, 2018-April,.
- Luo, H., Cheng, S., & Zhou, W. (2021). The factors influencing sales in online celebrities’ live streaming [Paper presentation].2021 IEEE International Conference on Information Communication and Software Engineering, ICICSE 2021.
- Ma, Y. (2021). Telematics and Informatics To shop or not : Understanding Chinese consumers ‘ live-stream shopping intentions from the perspectives of uses and gratifications, perceived network size, perceptions of digital celebrities, and shopping orientations. Telematics and Informatics, 59, 101562. https://doi.org/10.1016/j.tele.2021.101562
- Maghfiroh, L., & Hapsari, N. F. (2015). A celebrification of Celebgram on Instagram: A Case Study of @Shireeenz. Universitas Airlangga.
- Marwick, A. E. (2015a). Instafame: Luxury selfies in the attention economy. Public Culture, 27(1), 137–160.
- Marwick, A. E. (2015b). You may know me from YouTube: (Micro-)Celebrity in social media. In A companion to celebrity.
- MTarget. (2019). The Development of E-Commerce in Indonesia.
- Park, H. J., & Lin, L. M. (2020). The effects of match-ups on the consumer attitudes toward internet celebrities and their live streaming contents in the context of product endorsement. Journal of Retailing and Consumer Services, 52, 101934.
- Pink, B., & Laoli, N. (2020). BPS noted that online sales jumped sharply during the corona pandemic.
- Qian Z. (2021). Report of commerical model innovation and investment opportunities analysis on china webcast industry (2021-2026)
- Schiffman, L. G., & Cohn, D. Y. (2009). Are they playing by the same rules? A consumer gifting classification of marital dyads. Journal of Business Research, 62(11), 1054–1062.
- Sjöblom, M., Törhönen, M., Hamari, J., & Macey, J. (2017). Content structure is king: An empirical study on gratifications, game genres and content type on Twitch. Computers in Human Behavior, 73, 161–171.
- Steinhoff, L., Arli, D., Weaven, S., & Kozlenkova, I. V. (2019). Online relationship marketing. Journal of the Academy of Marketing Science, 47(3), 369–393.
- Sugiyono. (2015). Metode Penelitian Kombinasi (Mix Methods). Alfabeta.
- Sun, Y., Shao, X., Li, X., Guo, Y., & Nie, K. (2019). How live streaming influences purchase intentions in social commerce: An IT affordance perspective. Electronic Commerce Research and Applications, 37(August), 100886.
- Todorov, A., Chaiken, S., & Henderson, M. D. (2012). The heuristic-systematic model of social information processing. In The Persuasion Handbook: Developments in theory and practice.
- Tseng, F. C., Cheng, T. C. E., Yu, P. L., Huang, T. L., & Teng, C. I. (2019). Media richness, social presence and loyalty to mobile instant messaging. Industrial Management & Data Systems, 119(6), 1357–1373.
- Uzunoğlu, E., & Misci Kip, S. (2014). Brand communication through digital influencers: Leveraging blogger engagement. International Journal of Information Management, 34(5), 592–602.
- Wang, J. C., & Chiang, M. J. (2009). Social interaction and continuance intention in online auctions: A social capital perspective. Decision Support Systems, 47(4), 466–476.
- Wang, C., Zhou, Z., Jin, X. L., Fang, Y., & Lee, M. K. O. (2017). The influence of affective cues on positive emotion in predicting instant information sharing on microblogs: Gender as a moderator. Information Processing & Management, 53(3), 721–734.
- Xin Jean, L., Radzol, A. A. R. b M., Jun-Hwa Cheah, (., Mun Wai, W., Lim, X., … Radzol, A. A. R. b M, J. C.-A. J. of, & 2017, U. (2017). The impact of social media influencers on purchase intention and the mediation effect of customer attitude. Asian Journal of Business Research, 7(2).
- Xu, X., Wu, J. H., & Li, Q. (2020). What drives consumer shopping behavior in live streaming commerce? Journal of Electronic Commerce Research, 21(3).
- Yin, X., Wang, H., Xia, Q., & Gu, Q. (2019). How social interaction affects purchase intention in social commerce: A cultural perspective. Sustainability, 11(8), 2423.),
- Yu, E., Jung, C., Kim, H., & Jung, J. (2018). Impact of viewer engagement on gift-giving in live video streaming. Telematics and Informatics, 35(5), 1450–1460.
- Zafar, A. U., Qiu, J., Li, Y., Wang, J., & Shahzad, M. (2021). The impact of social media celebrities’ posts and contextual interactions on impulse buying in social commerce. Computers in Human Behavior, 115, 106178.
- Zhang, M., Qin, F., Wang, G. A., & Luo, C. (2020a). The impact of live video streaming on online purchase intention. Service Industries Journal, 40(9–10), 656–681.
- Zhang, M., Sun, L., Qin, F., & Wang, G. A. (2020b). E-service quality on live streaming platforms: swift guanxi perspective. Journal of Services Marketing, 35(3), 312–324.
- Zhao, Q., Chen, C. D., Cheng, H. W., & Wang, J. L. (2018). Determinants of live streamers’ continuance broadcasting intentions on Twitch: A self-determination theory perspective. Telematics and Informatics, 35(2), 406–420.
- Zhaoxing, W., Lee, S.-J., & Lee, K.-R. (2018). Factors influencing product purchase intention in Taobao live streaming shopping. Journal of Digital Contents Society, 19(4).
- Zhou, Z., Su, C., Zhou, N., & Zhang, N. (2016). Becoming friends in online brand communities: Evidence from China. Journal of Computer-Mediated Communication, 21(1), 69–86.