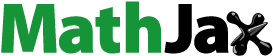
Abstract
The NEST or potential Emerging and growth-leading economies (EAGLEs) have been playing an increasing role in global growth, but the problems of their long-term growth attract the least attention, specifically the possibility of endogenous growth. The study was conducted to test for the endogenous growth possibility of the 15 NEST economies of the first wave. Based on macro data over 17 years, using the Bayesian non-linear regression method through the Metropolis–Hastings algorithm and Gibbs sampling, the author specified individual Constant elasticity of substitution (CES) functions for the researched economies. The Bayesian non-linear regressions are recognized as appropriate for empirical analysis of growth processes. The research revealed that out of the 15 studied NEST economies, the nine that have probably generated endogenous growth have acquired, on average, the higher magnitude of output growth, capital growth, FDI inflows, HDI, as well as expenditure on R&D. This is because these countries have implemented reasonable growth policies, such as encouraging investments from domestic and foreign companies, accumulating human capital, developing national R&D activities, extensively applying high technologies. Specifically, our findings are consistent with the predictions of Romer’s endogenous growth theory.
PUBLIC INTEREST STATEMENT
Though, along with EAGLEs, NESTs are considered a driving force of global growth, the problems of their long-term growth, specifically endogenous growth, have drawn little attention. This paper may be one of the first works, where NESTs’ endogenous growth is evaluated through the specification of individual CES production functions. The CES models of nine of 15 NESTs report the possibility of endogenous growth. These findings are consistent with the Romer growth theory. The study provides some important growth policy implications.
1. Introduction
Nowadays, undoubtedly, global economic growth is determined by the macroeconomic dynamics of the emerging world, where a particularly important position belongs to the EAGLE and NEST countries, the potential EAGLEs. These two groups are expected to increase their contribution to global economic growth by up to 74% by 2025 (BBVA Research, Citation2016). In other words, as the engine of global growth, the emerging economies will determine the growth prospects of the world economy in the next decade. Therefore, there is a great necessity to examine the endogenous growth possibility of the EAGLEs and NESTs. Endogenous growth is a way to achieve sustainable economic growth. Nevertheless, to verify whether an economy has exhibited endogenous growth, we should correctly specify an appropriate production function. Note, however, that most previous empirical and theoretical studies in growth theory, from Robert Solow to his followers, such as Kenneth Arrow or Paul Romer, have used Cobb–Douglas specifications for analysis. The fundamental drawback of this functional form is that the factor substitution elasticity should always equal one. This constraint masks the role of capital and labor interaction for economic growth process. In contrast, a considerable deal of empirical investigations explored the estimates of the elasticity of substitution between capital and labor different from one (see, for example, Nerlove, Citation1967; E. Asher, Citation1972; Blair & Kraft, Citation1974; Berndt, Citation1976; McFadden, Citation1978; Hamermesh, Citation1993; Duffy & Papageorgiou, Citation2000; Karabarbounis & Neiman, Citation2014, Thach, Citation2020c, and so on). Theoretically and empirically the elasticity of factor substitution has been proven to impact on economic growth (see, Pitchford, Citation1960; Sato, Citation1963; La Grandville, Citation1989; Barro & Sala-i-martin, Citation1995; Galor, Citation1995; Pereira, Citation2003; Palivos & Karagiannis, Citation2004, and so on). The CES production function with the elasticity of factor substitution different from unity built by Arrow et al. (Citation1961) has shown an increasing advantage over the Cobb–Douglas in studying economic growth, in particular, in testing the possibility of endogenous growth of an economy. Furthermore, the majority of the empirical studies on growth have applied the outdated frequentist approach, currently provoking widespread criticism due to inaccuracy found in many cases of statistical inference and prediction (Briggs and Hung T. Nguyen, Citation2019; Kreinovich et al., Citation2019; Nguyen, Trung, et al., Citation2019b).
Stemming from the above reasons, the study aims to test for the endogenous growth possibility of the 15 NEST emerging countries of the so-called first wave or the NESTs of 2011 through using a Bayesian non-linear regression method. With the empirical results obtained, the research will provide some useful implications for growth policies for the studied countries.
The structure of the study is as follows: Section 1 presents a conceptual framework and related empirical studies. The chosen method, model, and data are described in Section 3. Section 4 reports Bayesian simulation results and presents discussions. Conclusions and policy implications are provided in Section 5.
2. Theoretical background and related studies
2.1. Romer’s endogenous growth theory
The modern growth theory began with the first wave represented by the Solow-Swan model, the Ramsey model, and the Diamond overlapping–generation model over 20 years (1950-1960). Though the Ramsey model is considered the starting point, the most significant contribution to this generation, even the modern growth theory, perhaps, belongs to the Solow-Swan model. The two latter models did not advance us further than the former, or in other words, their logic in the end still comes down to the paradigm of the Solow-Swan model. In other words, all economies’ growth paths go under the law of positive and diminishing marginal productivities. The essence of the Solow-Swan model is simple. Because of fixed workforce growth, long-term economic growth depends on one only factor, that is, capital. Nonetheless, due to the law of positive and diminishing marginal productivities, at some point in time, accumulated capital will be entirely spent on the depreciation of machines necessary for all existing workforce. At that time, the growth of per capita income will stop. Therefore, all economies will converge to their steady state sooner or later. A modification of the Solow-Swan model is to bring exogenous technical change into the model. But this assumption does not help. For savings, population and technology seemingly “floats in from the outside,” the Solow-Swan model is only a kind of depicting facts, but not a true growth model describing the process of decision-making by economic agents. In exogenous growth theories, long-term economic growth is achieved only through exogenous effects. Secondly, the Solow–Swan model’s predictions about convergence seemed consistent with growth tendencies in the world in the 1960s. In the 1980s, they, however, began to raise considerable doubts: rich countries grew more rapidly than poor ones. Technical change and income growth have accelerated together in the former. Lastly, Solow’s (Citation1956, Citation1957) and Swan’s (Citation1956) exogenous growth model could not explain observations related to the law of diminishing returns to capital and labor. As a matter of fact, in developed economies, some industries experienced no decrease in returns to inputs as capital accumulation reached a certain level. Why? Endogenous growth theories born between the 1980s and 1990s provided reasonable answers. Thus, after two decades of a lull (1970-1980), the second wave of the modern growth theory came into sight with a powerfully influential model—the Romer model (Romer, Citation1986, Citation1987). However, we cannot analyze this first model separately from his later model, the Schumpeterian growth model (Romer, Citation1990). Let us briefly describe the Romer model first.
The essential idea of the Romer model lies in that new knowledge comprised discoveries and know-how and created by investing firms is widely disseminated to other firms. The formers, in turn, also benefit from the latter’s investments. As a result of knowledge spillover effects, returns to capital cease to be diminishing at the economy-wide level. In the 1980s, relying on the main ideas of his dissertation, which came from Arrow’s “learning-by-doing” hypothesis (Arrow, Citation1962), Paul Romer, a winner of the Nobel Prize in Economic Sciences (shared with William Nordhaus) in 2018, decided to build a new growth theory, where the conditions for endogenous growth are formulated without assuming exogenous technical progress. Romer was interested in how to make technical progress, the critical source of growth, endogenous. Since technology does not just “fall from heaven,” like everything else in the economy, it results from the actions of the agents optimizing their decisions. The “learning-by-doing” approach of Kenneth Arrow (Citation1962), another recipient of the Nobel Prize, states that the creation of new knowledge, i.e., new methods of more efficient production, skills to use them in job-practice, is a byproduct of mechanization (measured by capital-labor ratio). Seeking to maximize long-term profit, competing firms decide to invest. Investments in physical capital will surely give them great production opportunities in perspective, as shown in the models of the 1960s. Still, they are guided by the law of positive and diminishing marginal productivities. Equipped by a new machine, the production environment progressively evolves. Striving to operate the new equipment, workers learn the latest technologies, discover new forms of production organization, and search for new ideas to improve upon it. To sum up, when a firm raises its level of mechanization, its stock of knowledge rises through “learning-by-doing.” Therefore, grows by
percent each 1% increase in
:
where refers to a firm’s knowledge stock,
refers to its level of mechanization,
denotes the learning-by-doing elasticity of
with respect to
,
is a parameter,
.
The endogenous growth theory, devised by Romer, is well known as a combination of Arrow’s “learning-by-doing” hypothesis with the “knowledge spillover” effect. Accordingly, new knowledge generated by a firm through its own “learning-by-doing” will instantaneously and costlessly spill over all other firms. But, at the same time, this firm obtains the new knowledge created through the latter’s “learning-by-doing.” Thus, at any time all the firms have the same level of knowledge, which is equal to the economy-wide knowledge,
From expression (2), we obtain: . That means that all firms have the same capital-labor ratio equivalent to the economy-wide (aggregate) capital-labor ratio. Mainly, EquationEquation (2)
(2)
(2) also shows that the knowledge level of the economy is no longer exogenously achieved as it depends on the capital-labor rate determined by the firms and becomes an endogenous variable.
Romer’s endogenous growth theory is presented through an individual Cobb–Douglas production function:
EquationEquation (3)(3)
(3) satisfies the conditions of a neoclassical function with constant returns to scale (CRTS) and positive and ever-decreasing MPL (Marginal productivity of labor) and MPK (Marginal productivity of capital). By summating all the individual production functions, we obtain an aggregate function:
From , it follows that:
where stands for a depreciation rate,
denotes the rate of population growth,
represents ratio of savings to income, and
where Therefore, from EquationEquation (5)
(5)
(5) :
It follows that:
The capital-labor ratio increases at a constant rate only in case
, i.e., only if the elasticity of learning-by-doing” is unitary
From that, the aggregate production function takes the following:
As well-known from the growth theory, EquationEquation (9)(9)
(9) is a type of “AK model,” in which the outcome is proportional to the stock of capital for
supposed as a constant of proportionality.
Based on EquationEquations (6)(6)
(6) and (Equation8
(8)
(8) ), assuming
we have:
. Note that
is not yet a necessary and sufficient condition for endogenous growth. In case
an economy generates endogenous growth as long as
. Then, our model acquires a balanced growth steady state. From the conditions satisfied above, we can prove that:
Similarly to per capita variables, level variables grow at the same rate in the steady state:
where and
are the growth rate of per capital and level variables, respectively, in the steady state of an economy.
Notably, an AK-type economy is always in steady state; it does not tend to move towards the long-run equilibrium, or in other words, the long-run equilibrium and the short-run equilibrium coincide. From EquationEquation (9)(9)
(9) ,
. Because
and
at all
an economy of AK-type is permanently in a steady state. We would also highlight that the steady state must not be the same. A higher savings rate or stronger international integration shifts an economy from a steady state to a new one, and this effect should be permanent as compared to the Solow-Swan model. Variations in the pace of population growth or depreciation of fixed assets have an opposite impact on economic growth.
The Romer’s “learning by doing” model does not avoid some drawbacks. First, this model faces the problem of so-called observational equivalence, i.e., the same data set are consistent with more than one theory. Solow (Citation1997, p. 16) stated correctly that “all but impossible to make a convincing empirical case. Observations consistent with constant returns to capital would inevitably also be consistent with a fair range of increasing and decreasing returns to capital”. Second, the Romer model has a so restricted endogenous growth condition, that is, precisely If
then the economy confronts the tendency of diminishing returns to inputs, and the model does not differ from the Solow-Swan model without technical progress. If
, then the economy has explosive growth. However, different from the Solow-Swan model, capturing the economic agents’ decision-making behavior, the Romer model is capable of providing practical implications for growth policies.
If Romer’s above described “learning by doing, knowledge spillover” approach associates mainly to know-how and minor technological improvements made by the workers doing routine tasks, then his Schumpeterian model focusses on inventions created by firms specializing in producing technical progress on purpose. In the first case, firms produce new knowledge unconsciously in the investment process and spill over other firms gratis. But within the latter, new knowledge is intentionally initiated.
The Schumpeterian growth model is assumed to have three sectors in an economy: the final-good sector, the intermediate capital-good sector, and the R&D sector. The final-good sector uses human capital , unskilled labor
and various capital goods
to provide final-good output
for the economy. The technology of this sector is described as:
where is the income share of human capital (skilled labor), unskilled labor, respectively.
The intermediate capital-good sector produces intermediate capital goods utilizing the saved part of the output produced by the final-good sector together with knowledge generated by the research sector. Different from the final-good sector, which behaves as competitive, firms in the intermediate capital-good sector act as monopolists because they hold patents on the knowledge they invented or bought from the research sector. We have the amount of capital used in year :
or
where is units of
to produce one unit of any intermediate capital-good.
Substituting (14) into (12) yields:
The research sector utilizing human capital and the knowledge stock
produces knowledge for the intermediate capital-good sector. The growth rate
of
is:
where is a parameter.
As it is known:
where is rate of interest and constant.
With an assumption that is fixed, thus:
Therefore
Along the steady state balanced growth path of the economy:
From the above, Romer’s Schumpeterian model concludes that to generate unceasing growth, an economy is to expand its stock of knowledge continually. For this purpose, relevant are mainly two following conditions: (1) to increase human capital relative to raw labor (unskilled labor), and (2) to raise human capital engaged in research relative to the total human capital,
In real life, Romer’s two growth models reflect two different but corresponding processes. One is R&D activities, which creates new knowledge capable of reconstructing existing industries and giving rise to new ones. The other, in the meantime, brings small improvements into existing processes and products as the result of “learning by doing.” When an invention coming up from R&D activities is implemented, its full possibilities to raise will not be exhausted instantly. This is because it takes some time for the labor force to achieve the full command of this new invention. Here, “learning by doing” takes its turn at increasing
. So, “learning by doing” effect induces
to grow fast at the beginning and then slowly when the full possibilities of the new invention are reached.
2.2. Endogenous growth conditions in the CES
In the Romer models, where the basic condition for an economy to grow endogenously is that the elasticity of “learning-by-doing” should be equal to one . However, for endogenous growth, this is just a necessary condition, an implication of endogenous growth possibility, whereas we need a sufficient condition, that should be:
. The use of the Cobb–Douglas with the unitary elasticity of substitution leads to an extreme restriction, which is exactly
An enormous amount of empirical work revealed that in reality, the actual elasticity of substitution is not equal to one (see below). These findings paved the way for the formation of a more appropriate production function. Arrow et al. (Citation1961) eventually formulated the CES. Compared to the Cobb-Douglas, the CES has less restrictive propositions about the capital-labor interaction. One of its essential advantages is that the substitution elasticity differs from one, which can provide necessary implications for growth processes. Also, the CES specifications are appropriate for an empirical analysis with a sample period. Calculating the substitution elasticity, we can test for the endogenous growth possibility of an economy. According to (Miller, Citation2008), using CES variants can yield inconsistent estimates of the substitution elasticity. But, Klump and Preissler (Citation2000) proved that the inconsistency of the CES is related only to a functional form Barro and Sala-i-Martin formulated.
Therefore, we choose the standard (ACMS—Arrow, Chenery, Minhas, and Solow) CES specification for analysis, which is the following:
where =
is substitution parameter,
is distribution parameter;
is efficiency parameter, which is treated as neutral in the sense of Hicks, elasticity of substitution
Once the conditions
are satisfied, EquationEquation (21)
(21)
(21) is a neoclassical function.
By dividing both sides of EquationEquation (21)(21)
(21) by
, we obtain an expression for output per capita:
From EquationEquation (22)(22)
(22) , the marginal and average products are as follows:
and
As it is known:
That is to say:
With the condition:
We substitute (26) into (27) and divide by , the growth rate of
is thus:
In the CES, the marginal and average products depend on the substitution parameter , so will economic growth rate. We easily prove that in the case of a high degree of substitution, i.e.,
we obtain the limits of the marginal and average products as:
The marginal and average products thus do not approach zero as grows over time. Pereira (Citation2003) views the elasticity of substitution as an index of the pace at which diminishing marginal returns set in when one input (capital) grows with respect to the other (labor). In case the elasticity of substitution has a substantial value, substituting one input for the other or increasing output by raising the amount of one input is easy because diminishing marginal returns set in at a very slow pace or not set at all, or in other words, the greater the substitution elasticity, the smaller the drag due to diminishing marginal returns. In this context, it can be concluded that the economy generates endogenous growth as long as
or
We can easily prove that with the substitution elasticity lower than one, the marginal and average products go to zero as the capital-labor ratio approaches infinity. Hence, the model does not satisfy endogenous growth conditions.
Similarly, Palivos and Karagiannis (Citation2004), examining the relationship between the marginal product of capital, the substitution elasticity and unbounded endogenous growth, theoretically proved that a bounded away from zero marginal product of capital implies an elasticity of substitution between capital and labor that is asymptotically higher than one, and if the elasticity of substitution which is in the limit more than one (joined with the appropriate restriction on the rate of time preference or the savings rate holding, the model will exhibit potentially perpetual endogenous growth. The conclusion concerning the CES is generalized to the Variable Elasticity of Substitution (VES).
2.3. Empirical studies
2.3.1. Empirics on romer models
Regarding the empirics of Romer’s “learning-by-doing” approach and Schumpeterian growth theory, we can find broad support at the firm as well as industry levels. Specifically, the “learning-by-doing” effect appeared in the airplane construction (H. Asher, Citation1956; Wright, Citation1936), the shipbuilding industry (Rapping, Citation1965; Searle, Citation1946), and others. They found experience accumulated by workers to affect labor productivity in the researched industries. Besides, the “learning-by-doing” effect was investigated from the perspective of the connection between physical investment and the number of patents. Analyzing various unrelated industries, Schmookler (Citation1966) revealed that investment is followed by patents with a lag of one period.
In modern empirical literature, Valdes (Citation1999) utilized the U.S. data to verify the endogenous growth conditions and
. How well do these predictions fit the data? For the U.S. economy, the capital stock is roughly two-and-one-half times the yearly flow of output, i.e.
or
From EquationEquation (9)
(9)
(9) ,
The average annual rate of capital depreciation is supposed to be around 0.04. Historical database reports the 1.5 percent annual growth rate of that U.S. population and their savings ratio of 0.187. From these figures, we quickly see that
Notably, moreover, there are a lot of analyses performed to empirically test not only the Romer endogenous growth theory but also his modified versions or perhaps ideas beyond them. For example, relying on the Summer-Heston data set, Binder and Pesaran (Citation1999) using a cross-country dataset reveal the effects of labor growth, saving ratio, and the variance of capital-output on the capital-output ratio in light of an AK model. Based on the theoretical implications of an AK model, with the utilization of panel-data, verifying the long-run growth-investment linkage, De Long and Summers (Citation1991, Citation1992, Citation1994) prove the existence of fixed returns to scale in reproducible factors. In like manner, these relationships were also tested in several empirical studies (see, e.g., Bond et al., Citation2004; Kim, Citation2003; Li, Citation2002; Romero-Ávila, Citation2009, Citation2013; Temple, Citation1998). Empirical research on the impact of education on economic growth, as implied in endogenous growth literature, was carried out through a variety of regression specifications by many authors, such as Barro (Citation1991), Barro and Lee (Citation1994), Bils and Klenow (Citation2000), and Krueger and Lindhal (Citation2001), or Jalil and Idrees (Citation2013). Based on macro data of 98 countries for the period between 1960-1985, Barro (Citation1991) discovers the growth rate of real per capita GDP to be positively correlated to initial human capital. Bils and Klenow (Citation2000) find a positive link between schooling and growth on a cross-country data set. In a study of Krueger and Lindhal (Citation2001), microeconometric evidence shows that education is a key determinant of individual income, while education attainment impacts on income growth on cross-country data. Of 67 predictor variables Sala-i-Martin et al. (2004) reveal 18 to affect significantly on long–term growth and primary school enrollment, investment, and initial real GDP per capita level are proven to be the strongest evidence. Cohen and Soto (Citation2007) utilizing a panel data for the 1960-2000 period empirically find that years of schooling influence economic growth. Barro and Lee (Citation1994), Jalil and Idrees (Citation2013) confirm the effect of human capital on economic growth. Last but not Jones (Citation1995a, Citation1995b), Gong et al. (Citation2004), Romero-Ávila (Citation2009, Citation2013) applied time series regressions to analyze the impact of research and development expenditures and investment on economic growth. Jones (Citation1995a, Citation1995b), Gong et al. (Citation2004) empirically discover the impact of R&D expenditures and physical investment on economic growth, whereas in an AK-type Romero-Ávila (Citation2009, Citation2013) finds a long-term connection between investment and growth. Recently, endogenous growth for an emerging economy is analyzed in Thach (Citation2020a, Citation2020b, Citation2020c). Within the Bayesian framework, empirical results demonstrate that the model outcomes depend on prior specification. The use of various priors results in the different estimates of elasticity of substitution.
2.3.2. The elasticity of factor substitution
There is a good deal of evidence found against the application of the Cobb–Douglas in growth theory. As well known, growth economists such as Solow (Citation1956, Citation1957) and his followers used Cobb-Douglas specifications with the assumption that technical change is neutral and the variations of the substitution elasticity are completely disregarded as it is considered always equal to one. On the contrary, the elasticity of substitution alters as revealed by a vast amount of empirical investigations. Conducting a specific survey, Nerlove (Citation1967) found that the application of different sample periods or econometric methods may lead to various estimates of the substitution elasticity. Berndt (Citation1976) provided a similar conclusion in comparing estimated values of the elasticity of substitution based on two estimation techniques, six alternative specifications, and five various measures of the rental price of capital. Testing for the constancy of the substitution elasticity for the steam–electric generating industry, McFadden (Citation1978) estimated an elasticity close to 0.75. According to an empirical finding (Hamermesh, Citation1993), the elasticity of substitution changes in a range of 0.49–6.86 for the UK and of 0.32–1.16 for the US. Relying on data set of 59 countries throughout 1975-2012, Karabarbounis and Neiman (Citation2014) found the elasticity of substitution more than one at the sector-, state- and country-levels in the vast majority of cases.
Many empirical findings show that the elasticity of substitution increases with economic development. A review of empirical studies on the U.S. processing industry over 200 years demonstrates that the value of the substitution elasticity tends to vary. E. Asher (Citation1972), Uselding (Citation1972) found the elasticity of substitution of nearly zero in the 19th century. Its values fluctuated around one in the middle of the 20th century (Zarembka, Citation1970). In the meantime, according to Blair and Kraft (Citation1974, Citation1996, the substitution elasticity became larger than one at the end of the 20th century. Applying a Variable Elasticity of Substitution function to an analysis of 12 OECD economies for 1965–1986, Genç and Bairam (Citation1998) found the average elasticity of substitution higher than one. Estimating the elasticity of substitution using a CES specification on a cross-section of 82 economies by Duffy and Papageorgiou (Citation2000) resulted in a value smaller than one for developing countries and greater than one for advanced countries. From this finding, the authors went to the conclusion that the elasticity values are associated with a country’s development stages. In general, the above-mentioned analyses showed that the elasticity of substitution remains relatively stable through a sample period, but grows with economic development. Notably, a variety of empirical results comes from the difference in data and estimation methods. In the studies of Thach (Citation2020a, Citation2020b, Citation2020c), prior choice greatly influences the posterior distributions in the use of Bayesian methods.
2.3.3. Effect of elasticity of factor substitution on economic growth
Many economic branches, including growth literature, have long recognized the importance of the substitution elasticity. Several researchers of early growth theory attempted to evince its role in growth processes. The relationship between the elasticity of substitution and economic growth was first analyzed systematically by La Grandville (Citation1989), who theoretically proved that per capita income growth is an increasing function of the substitution elasticity at all stages of development. Ferguson (Citation1965) documented that in the case of an elasticity of substitution different from one, the economic growth rate depends on the elasticity of substitution and the rate of savings ratio growth. The use of the Slutsky equation by La Grandville (Citation1989) produced similar evidence on the positive relationship between the elasticity of substitution and economic growth: a greater elasticity of substitution allows for achieving a higher production level. Performing comparative analysis of the US and South Korean economies, Yuhn (Citation1991) revealed that the substitution elasticity was higher for South Korea, which supports the fact that this economy acquired a higher growth rate since the 1960s. Based on data set between 1961 and 1991, Cronin et al. (Citation1997) found that the elasticity of substitution between telecommunication and capital is 13.01. As production is an increasing function of the substitution elasticity, its variations affect growth.
Similarly to Solow (Citation1957), Sato (Citation1963), and Pitchford (Citation1960) affirmed that a variety of substitution elasticities would result in multiple growth paths, among which there will be some unbalanced. The likelihood of poverty traps depends on elasticity values, as presented in an overlapping generations model by Azariadis (Citation1993). Barro and Sala-i-martin (Citation1995) argued that high elasticity of substitution could create endogenous, steady-state growth under certain circumstances. In the case of the substitution elasticity of more than one, endogenous growth possibility with a unique steady-state can be achieved. While CES specifications in (Pitchford, Citation1960), (Azariadis, Citation1993), and (Galor, Citation1995) probably cause poverty traps for income per capita and multiple steady states if a substitution elasticity is less than one. Later, Klump and La Grandville (Citation2000) ensured that a greater elasticity of substitution generates higher long-run growth paces and more likely endogenous growth. The more an elasticity of substitution, the larger per capita income in steady state. Utilizing a simple CES model, Klump and Preissler (Citation2000) also found that a larger substitution elasticity induces a higher steady state, and continual growth is more probable. For the CES specifications, the elasticity of substitution affects production tendency in almost all cases, except when labor and capital rise at the same pace (see Kamien & Schwartz, Citation1968). Generally, we can see the similarity of the results in the above empirical investigations.
To the author’s knowledge, the majority of studies on economic growth in Vietnam access frequentist statistics or the accounting method in specifying Cobb–Douglas functions. However, no research was performed on the Vietnamese economy’s endogenous growth (see, e.g., Huynh, Citation2019; Khuc & Tran, Citation2016; Nguyen, Citation2013; Pham & Phuong, Citation2016; Tu & Phuc, Citation2012).
Pay attention to the fact that because of the strong restrictiveness of assumptions in the Cobb-Douglas, the CES specifications have been applying increasingly in growth theory. In the current work, therefore, a standard CES function is specified to estimate the elasticity of substitution based on the macro data of the 15 NEST countries.
3. Methodology and data
3.1. Method and model specification
Growth economists make use of a variety of indirect and direct methods to estimate the elasticity of substitution. In a direct way, we estimate the elasticity of substitution via a specified production function. In the meanwhile, an indirect method helps to explore the relationship between the substitution elasticity and factor shares to obtain the needed estimates. Sharing Mizon’s (Citation1977) view, we declare a preference for the direct methods since they can provide estimates for plenty of production functions on a common methodological foundation.
In the overwhelming majority of earlier studies on economic growth, the estimation of the substitution elasticity is performed within the frequentist approach applying CES, VES, or Cobb-Douglas specifications. Since the 1990s, Bayesian analysis has been used extensively in a wide variety of humanity and social sciences due to its significant advantages (e.g., Anh et al., Citation2018; Nguyen & Thach, Citation2018; Kreinovich et al., Citation2019; Nguyen & Thach, Citation2019; Briggs and Hung, Citation2019; Nguyen et al., Citation2019a, Citation2019b; Sriboonchitta et al., Citation2019; Svítek et al., Citation2019; Thach, Citation2020a, Citation2020b). Firstly, undoubtedly, universality is the most significant methodological advantage of the Bayesian approach to frequentist statistics because Bayesian inference relies on a simple rule of probability, the Bayes rule. In contrast, in the frequentist approach, various estimation methods are designed for specific statistical problems and models, so the methods constructed for a class of models cannot support another. Secondly, frequentist inference does not provide an intuitive probabilistic interpretation in comparison with Bayesian inference. A 95% confidence interval is often interpreted as follows: Let us repeat the same experiment millions of times and compute a confidence interval for each experiment, 95% of the obtained intervals will encompass the true value of the parameter. A Bayesian credible interval is interpreted as the interval to which a parameter belongs with a certain probability. Thirdly, precision in Bayesian inference is not limited by sample size.
In Bayesian analysis, we can use the rule of conditional probability:
to derive Bayes’s theorem:
with as random vectors.
A data vector is assumed as a sample derived from a probability model, utilizing a likelihood function; we express this model thus:
where is unknown parameter vector
stands for a probability density function of
given
.
We can infer some properties of given data
We begin our analysis by specifying a posterior model combining prior knowledge with available data to describe the probability of all model parameters. Two components, a likelihood function incorporating information about the parameters conveyed by data and prior knowledge of the parameters, are integrated to set up a posterior model by Bayes’ law:
Given and
as random variables, by Bayes’s theorem, we calculate the posterior distribution of
given
:
where as marginal distribution of
is the following:
where is a likelihood function of X given
,
stands for a prior for
,
denotes prior predictive distribution.
Our research strategy in this paper is designed as follows:
Step 1: To generate the elasticity of substitution for each of the 15 NEST economies through specifying its individual CES function. Concerning parameter of interest, , we perform a sensitivity analysis to select appropriate priors.
Step 2: With the elasticity of substitution estimated for each economy in the NEST group, we will check for this economy’s endogenous growth possibility.
Applying the direct method, we estimate the elasticity of substitution through the specification of a CES function. In growth literature (see, e.g., Davidian & Giltinan, Citation1995; Pinheiro & Bates, Citation2000), growth processes are modeled using nonlinear regressions. Thus, the CES can be estimated through the utilization of the Bayesian nonlinear regression.
The selection of appropriate priors is crucial in Bayesian analysis. Frequentists sharply criticize Bayesians for their subjectivity when different researchers choose various priors for the same model. Our experience with fitting Bayesian regression models suggests that priors can be specified based on prior information: belief, theory, expert knowledge, or experimental evidence. To avoid subjectivity, objective Bayesians tend to apply vague or weakly informative priors. Although, as stated by Walley et al. (Citation1996), Fisher (Citation1996), and Irony and Singpurwalla (Citation1997), all priors should provide certain information and in the case of sparse data, even priors that tend to be flat might produce an unintentionally great extent of influence on posterior inferences. Yang and Berger (Citation1998) also advocate objectivity in prior choice. Sharing their view, we set weakly informative priors on all the parameters with reference to expert knowledge and empirical evidence. Based on the neoclassical production theory and previous investigations (Arrow et al., Citation1961; Lagomarsino, Citation2017; Thach, Citation2020c), we put Igamma(0.001, 0.001) prior on the variance parameter (), normal N(0,100) prior on parameter
, uniform(0,1) prior on parameter
. Previous studies suggest gamma(1,1) prior on parameter
, but empirically it can range from −1 to 1, so we decided to try more with the uniform(−1,1) prior. Furthermore, a sensitivity analysis performed through a Bayes factor test will indicate which prior to pick. The Hicks-neutral technology terms
is supposed to grow exponentially according to
Taking logs of both sides of EquationEquation (21)
(21)
(21) yields our Bayesian non-linear regression model as follows:
Likelihood function:
Prior distributions:
where denotes natural log of output
and
denote capital stock and labor (workforce), respectively,
is substitution parameter used to obtain the elasticity of substitution,
,
,
is a random error.
3.2. Data description
The estimation of the elasticity of substitution requires data on real GDP, capital stock, and labor. We utilized time-series data of the 15 NEST countries of the first wave, candidates for the EAGLE (Emerging and Growth–Leading Economies) membership (Argentina, Bangladesh, Chile, Colombia, Egypt, Malaysia, Nigeria, Pakistan, Peru, the Philippines, Poland, South Africa, Thailand, Ukraine, and Vietnam) obtained from a variety of sources and covering the 17 years from 2000 to 2016. Data on GDP and capital stock at constant 2011 prices were collected from the Penn World Tables introduced by the Federal Reserve Bank of St. Louis (Citation2019). Data on labor are gathered from the data source of the World Bank (Citation2019). Time frequency indicates the year. GDP and stock of capital are measured in billions of dollars and labor in millions of employees.
4. Research results
4.1. A Glimpse at NEST Economies’ growth
At present, there is no commonly recognized concept of an emerging economy. The IMF combines developing and emerging economies into one group, while the World Bank classifies countries by per capita income. There is a variety of concepts established to refer to emerging economies. But the common criteria for evaluating an economy as emerging are a steady increase in GDP, a steady increase in GDP per capita, an increase in foreign trade exceeding that of international trade, the presence of foreign capital invested over the long term, global companies based in several countries, a diversified economy, promising economic prospects, political stability, optimism about the future (Ithurbide & Bellaiche, Citation2019). Among lots of concepts, nonetheless, the EAGLE and NEST concept is worth the closest attention due to their transparency (clear selection criteria), dynamism (fast enough growth) and flexibility (short horizon of 10 years, flexible number of countries according to their economic performances) (BBVA Research, Citation2012). The EAGLE concept was established at the end of the 2010s by BBVA Research to point out the emerging economies, whose share in global growth between 2011-2021 is expected to be higher than the G6 average level (G7 without the U.S.). In this concept, EAGLEs undergo a continuous follow–up, and the membership of them can alter according to their predicted economic performances. As a part of the EAGLE concept, the NEST is the second group of economies, whose forecasted GDP growth in the next decade will be less than the G6 average but more than Italy’s (G6 minimum), the economy expected to contribute least among the G7 members to the world GDP. The membership of the NESTs is also subject to annual reexamination and can alter. To become EAGLEs, the NESTs should achieve an expected GDP threshold.
According to the data shown in , the average rate of economic growth throughout 2000–2016 varies from the lowest of 1.5% for Ukraine to the highest of 6.5% for Vietnam. The growth rate expected for the 15 NEST economies between 2011–2021 is presented in . Based on the data of the Federal Reserve Bank of St. Louis (Citation2019), the economic growth rate for 2011-2016 equals 0.75% (Argentina), 6.6% (Bangladesh), 2.2% (Chile), 3.2% (Colombia), 3.6% (Egypt), 5.2% (Malaysia), 2.7% (Nigeria), 5.0% (Pakistan), 3.6% (Peru), 6.6% (the Philippines), 3.3% (Poland), 1.5% (South Africa), 2.8% (Thailand), −2.4% (Ukraine), and 6.2% (Vietnam). As presented in the 2016 report (BBVA Research, Citation2016), seven of the 15 NEST countries became EAGLEs; those are Nigeria, Philippines, Pakistan, Egypt, Bangladesh, Malaysia, and Vietnam. Having achieved the poorest performance, Ukraine was stripped of the NEST membership. Now, a question is here: Will the researched NEST economies be able to grow far faster until 2021 and further 2025 to obtain the growth goal projected by BBVA Research? This goal is now more difficult to be gained in the context of the continuing U.S.-China trade war, and the Covid-19 outbreak first detected in Wuhan (China) in December 2019 and spreading to almost all the countries, which will certainly harm global economic growth in 2020 and the following years.
Table 1. Some main performances of studied 15 NEST economies
4.2. Bayesian simulation results
4.2.1. Results of sensitivity analysis
Based on the estimation results of the substitution parameter for two proposed prior distributions:
and
, through checking for sensitivity to the candidate priors, we chose the
prior to the CES functions of Egypt, Nigeria, Pakistan, the Philippines, and Ukraine and the
prior to those of Argentina, Bangladesh, Chile, Colombia, Malaysia, Peru, Poland, South Africa, Thailand, and Vietnam. From these results, we calculated the substitution elasticity for the researched economies ().
Table 2. The elasticity of substitution of 15 studied NEST economies
4.2.2. Robustness checks
Before performing Bayesian inference, we need to check for model robustness. For this, we inspect whether Markov chain Carlo Monte (MCMC) chains converged to the stationary distribution. Two conventional methods of convergence diagnostics, formal and graphical, are practiced. In formal diagnostics, we can use two criteria: acceptance rate and sampling efficiency. Acceptance rate is understood as the ratio of accepted proposals to the total proposals. Efficiency implies mixing attributes of chain sampling. Both these rates have effects on chain convergence. According to the estimation results, the specified models obtain a sufficiently high acceptance rate in a range from 0.5 to 0.7, which is acceptable according to Roberts and Rosenthal (Citation2001). The model summaries report that average efficiencies range from 0.1 to 0.3, which are higher than the sufficient level of 0.08 ().
Table 3. Model summaries
Also, we use cusum plots as a visual diagnostics for chain convergence. Cusum lines point to the sign of convergence if they, jagged and not smooth, start from the horizontal axis and intersect this axis in the end. Cusum plots of all the parameters of 15 selected models look convincing ().
4.3. Discussion
To test for the endogenous growth possibility for the 15 NEST countries of the first generation, the study derived the elasticity of substitution by specifying the individual CES function for each economy. In fitting the Bayesian non-linear regression model, the study chose suitable priors based on the neoclassical production function theory, which help to specify the CES in several previous analyses. The primary attention was focused on the substitution parameter because it determines the substitution elasticity value. A sensitivity analysis helped to select suitable priors on this parameter. As a result, five economies have the elasticity of substitution smaller than one, and four of them, (except for Egypt) experienced low capital growth in the sample period. The remaining economies of the sample with the elasticity of substitution greater than one faster accumulated capital. Specifically, Vietnam obtained the highest elasticity of 3.3 mainly thanks to the highest rate of capital accumulation. On the contrary, Ukraine had the lowest growth of capital with the most moderate elasticity of 0.5. These findings are consistent with the predictions of the endogenous growth theory: the elasticity of factor substitution is determined by the capital–labor ratio, which in turn significantly depends on the growth rate of capital with respect to labor.
According to the endogenous growth conditions conceptually formulated by Romer and proven later within the framework of CES functions by Pereira (Citation2003) and Palivos and Karagiannis (Citation2004), the elasticity of factor substitution greater than one is both necessary and sufficient for an economy to create endogenous growth. Therefore, nine out of the 15 NEST economies in our sample obtained this possibility; those are Argentina, Bangladesh, Colombia, Malaysia, Peru, Poland, South Africa, Thailand, and Vietnam.
Based on Romer’s endogenous growth theory, we can provide some essential explanations.
First, capital formation contributes largely to endogenous growth. The NEST countries could actively enhance investment in the research period. As a consequence, the stock of capital has steadily grown over the years in comparison with labor. Specifically, the average growth pace of capital relative to that of labor reached 2.2 times in the nine endogenously growing economies in comparison with 1.6 times for the six remaining ones. The endogenously growing economies also achieved a higher rate of output growth than the rest, 4.4% against 3.9% (calculated on the data in ).
Second, through education, human capital is both the motor and the beneficiary of growing middle classes. The endogenously growing economies were able to accumulate fast human capital, which is expressed by an increase in the number of people having secondary and higher studies and has led to a rise in the share of wealthier segments. Notably, on average, HDI of these nations is higher that of the remaining ones, namely 0.65 against 0.58 in 2000 and 0.75 against 0.67 in 2019 (United Nations, Citation2019). In general, emerging economies are predicted to raise the number of people with secondary and higher education and hence their share in global middle classes from 58% in 2015 to 75% in 2025 (BBVA Research, Citation2016).
Third, generally, research and development expenditure tends to grow in emerging economies, which has led to a rapid rate of technological progress. On average, expenditure on R&D (% of GDP) amounted to 0.5% in the endogenously growing economies as compared to 0.3% in the rest (World Bank, Citation2019). Emerging economies, in particular, developing Asian ones, have converged rather quickly to the most developed countries in the application of the latest IT and high technologies.
Fourth, we witness stable FDI inflows to developing and emerging economies. During 2013–2018, the share of developing countries in world FDI inflows remained almost unchanged, approximately in a range of 2.1–2.3%. Nevertheless, FDI inflows to developing countries in Asia and Oceania sharply increased in 2017-2018 and accounted for 73% of those inflows (UNCTAD, Citation2019). Specifically, the share of the NEST economies in world FDI inflows is almost stable, though FDI inflows rose in most of the endogenously growing economies (World Bank, Citation2019).
5. Conclusions
This study was conducted to test for the possibility of endogenous growth in the 15 NEST economies ranked in the 2012 report of BBVA Research. Utilizing the Bayesian non-linear regression method through the Metropolis–Hastings algorithm and Gibbs sampling, based on the time-series data of the researched countries, the study calculated the elasticity of substitution between capital and labor through specifying the individual CES production functions for the economies of interest. A check for MCMC convergence indicated that Bayesian inference is robust. The application of weakly informative priors in previous studies is appreciated in this work. A sensitivity analysis using the Bayes factor helped to pick more appropriate priors. The empirical results show that out of the 15 NEST countries, nine (Argentina, Bangladesh, Colombia, Malaysia, Peru, Poland, South Africa, Thailand, and Vietnam) have substitution elasticity greater than one, while the rest smaller than one. In other words, a higher growth rate of capital contributes largely to greater substitution elasticity. Except for Egypt, in five “stagnant” NEST economies, capital accumulated at a rather low pace. From these findings, the author concludes that the nine just mentioned countries can potentially generate endogenous growth. Political and social stability, high investment in physical and human capital, extensive application of IT and high technologies have significantly contributed to the NEST countries’ socio-economic and technological improvements. However, in the author’s opinion, to achieve sustainable growth in the long-run, the NEST economies need to take some essential measures:
First, for the researched NEST countries, there is a great necessity to continuously foster progress in educational attainment, through which to increase access to at least secondary education for the overwhelming majority of the population. The continuous growth of income will significantly contribute to the expansion of human capital and middle classes. Raising the quality of teachers and modernizing school programs are really necessary. Governments must be responsible for education quality accreditation. For this, it is substantial to increase public expenditure on education and fundamental research, and encourage the development of private educational services.
Second, more public and private investments in R&D activities, physical and human capital, should be strongly stimulated among the NESTs. At the same time, an emphasis on policies of attracting FDI towards improving the quality of investment projects such as improving economic infrastructure, increasing quality of workforce, applying preferences for foreign firms is necessary. Technology transfers should be enhanced actively. Specifically, for the “stagnant” NEST subgroup, to raise the savings ratio and to encourage productive investments should be their central growth policies.
Third, Internet access and the application of IT and high technologies to production should be pervasively enhanced. Governments must quickly create an environment for IT application and development, such as for widespread use of personal accounts, salary payment via accounts, commercial transactions via accounts, etc. Moreover, governments need to push up the quality of governance to minimize macroeconomic instability and motivate private businesses. By creating a highly competitive environment, IT is an important tool to improve productivity and efficiency of production, sales and services. In a highly competitive environment, organizations, businesses and individuals consider the application of IT an indispensable solution to improve their competitiveness.
Additional information
Funding
Notes on contributors
Nguyen Ngoc Thach
Nguyen Ngoc Thach is a Doctor of Science (Economics). His area of research includes macroeconomics, economic growth, institutions, and Bayesian statistics. He has published many research papers in international conference proceedings and refereed journals related to these expert fields.
References
- Anh, L. H., Kreinovich, V., & Thach, N. N. (eds). (2018). Econometrics for Financial Applications. Springer. https://doi.org/10.1007/978-3-319-73150-6
- Arrow, K. J. (1962). The Economic Implications of Learning by Doing. Review of Economic Studies, 29(June), 155–22. https://doi.org/10.2307/2295952
- Arrow, K. J., Chenery, H. B., Minhas, B. S., & Solow, R. M. (1961). Capital Labour Substitution and Economic Efficiency. Review of Econ. And Statistics, 63(3), 225–250. https://doi.org/10.2307/1927286
- Asher, E. (1972). Industrial efficiency and biased technological change in American and British manufacturing: The case of textiles in the nineteenth century. Journal of Economic History, 32(2), 431–442. https://doi.org/10.1017/S0022050700067188
- Asher, H. (1956). Cost-Quantity Relationships in the Airframe Industry, R-291, Santa-Monica: The Rand Corporation.
- Azariadis, C. (1993). Intertemporal Macroeconomics. Blackwell Publishers.
- Barro, R. J. (1991). Economic growth in a cross-section of countries. Quarterly Journal of Economics, 106(2), 407–443. https://doi.org/10.2307/2937943
- Barro, R. J., & Lee, J.-W. (1994). Sources of economic growth. Carnegie-Rochester Conference Series on Public Policy, 40(1), 1-46.
- Barro, R. J., & Sala-i-martin, X. (1995). Economic Growth. McGraw Hill.
- BBVA Research (2012). Annual Report 2012 https://www.bbvaresearch.com/en/publicaciones/eagles-economic-outlook-annual-report-2012/
- BBVA Research (2016). https://www.bbvaresearch.com/en/publicaciones/eagles-economic-outlook-annual-report-2016/
- Berndt, E. (1976). Reconciling alternative estimates of the elasticity of substitution. Review of Economics and Statistics, 58(1), 59–68. https://doi.org/10.2307/1936009
- Bils, M., & Klenow, P. (2000). Does schooling cause growth? American Economic Review, 90(5), 1160–1183. https://doi.org/10.1257/aer.90.5.1160
- Binder, M., & Pesaran, M. H. (1999). Stochastic growth models and their econometric implications. Journal of Economic Growth, 4(2), 139–183. https://doi.org/10.1023/A:1009802421114
- Blair, R., & Kraft, J. (1974). Estimation of elasticity of substitution in American manufacturing industry from pooled cross-section and time-series observations. Review of Economics and Statistics, 56(3), 343–347. https://doi.org/10.2307/1923972
- Bond, S., Lebeblicioglu, A., & Schiantarelly, F. (2004). Capital accumulation and growth: a new look at the evidence, IZA Discussion Paper 1174, Bonn.
- Briggs, W. & Hung T. Nguyen. (2019). Clarifying ASA's View on P-Values in Hypothesis Testing. Asian Journal of Economics and Banking,3(2), 1–16.
- Cohen, D., & Soto, M. (2007). Growth and human capital: Good data, good results. Journal of Economic Growth, 12(1), 51–76. https://doi.org/10.1007/s10887-007-9011-5
- Cronin, F. J., Colleran, E., & Gold, M. (1997). TELECOMMUNICATIONS, FACTOR SUBSTITUTION AND ECONOMIC GROWTH. Contemporary Economic Policy, 15(3), 21–31. https://doi.org/10.1111/j.1465-7287.1997.tb00474.x
- Davidian, M., & Giltinan, D. M. (1995). Nonlinear models for repeated measurement data. Chapman & Hall/CRC.
- De Long, B. J., & Summers, L. H. (1991). Equipment investment and economic growth. Quarterly Journal of Economics, 106(2), 445–502. https://doi.org/10.2307/2937944
- De Long, B. J., & Summers, L. H. (1992). Equipment investment and economic growth: How strong is the nexus. Brookings Papers on Economic Activity, 2(2), 157–199. https://doi.org/10.2307/2534583
- De Long, B. J., & Summers, L. H. (1994). Equipment investment and economic growth: Reply. Quarterly Journal of Economics, 109(3), 803–807. https://doi.org/10.2307/2118423
- Duffy, J., & Papageorgiou, C. (2000). A cross-country empirical investigation of the aggregate production function specification. Journal of Economic Growth, 5(1), 87–120. https://doi.org/10.1023/A:1009830421147
- Federal Reserve Bank of St. Louis. (2019). Penn World Table. Available online: https://fred.stlouisfed.org.
- Ferguson, C. (1965). The elasticity of substitution and the savings ratio in the neoclassical theory of growth. Quarterly Journal of Economics, 79(3), 465–471. https://doi.org/10.2307/1882709
- Fisher, L. D. (1996). Comments on Bayesian and frequentist analysis and interpretation of clinical trials. Control Clinical Trials, 17(5), 423–434. https://doi.org/10.1016/S0197-2456(96)00043-8
- Galor, O. (1995). Convergence? Inference from theoretical models. Economic Journal, 106(437), 1056–1069. https://doi.org/10.2307/2235378
- Genç, M., & Bairam, E. (1998). The box-cox transformation as a VES production function. chapter 4. In E.I. Bairam: Production and cost functions: Specification, measurement, and applications (pp. 54–61). Ashgate Press.
- Gong, G., A.,, & Semmler, W. (2004). Endogenous growth: Estimating the Romer model for the US and Germany. Oxford Bulletin of Economics and Statistics, 66(2), 147–164. https://doi.org/10.1046/j.0305-9049.2003.00082.x
- Hamermesh, D. (1993). Labor Demand. Princeton University Press.
- Hsing, Y. (1996). An empirical estimation of regional production function for the U.S. manufacturing. Annals of Regional Science, 30(4), 351–358. https://doi.org/10.1007/BF01581948
- Huynh, T. N. (2019). Factors affecting technical efficiency in Vietnamese small and medium enterprises. Journal of Asian Business and Economics Studies, 30(7), 43-65. accessed 11 January 2020 Available online http://jabes.ueh.edu.vn/Home/SearchArticle?article_Id=8fbfecc6-ffc8-4ab8-84b7-5ca9b1ab8c50
- Irony, T. Z., & Singpurwalla, N. D. (1997). Noninformative priors do not exist: A discussion with Jose M. Bernardo. Journal of Statistical Inference and Planning, 65(1), 159–189. https://doi.org/10.1016/S0378-3758(97)00074-8
- Ithurbide, P., & Bellaiche, M. (2019). How to differentiate emerging countries? New approaches for classification and typology. Amundi Research team. 3–60. https://research-center.amundi.com/ezjscore/call/ezjscamundibuzz::sfForwardFront::paramsList=service=ProxyGedApi&routeId=_dl_NWVmN2Q5MjViMjJmOTRmYTU3NGRiMTcxNGM2ZjA5OWU
- Jalil, A., & Idrees, M. (2013). Modeling the impact of education on the economic growth: Evidence from aggregated and disaggregated time series data of Pakistan. Economic Modelling, 31(1), 383–388. https://doi.org/10.1016/j.econmod.2012.11.035
- Jones, C. I. (1995a). Time series tests of endogenous growth models. Quarterly Journal of Economics, 110(2), 495–525. https://doi.org/10.2307/2118448
- Jones, C. I. (1995b). R&D-based economic models of economic growth. Journal of Political Economy, 103(4), 759–784. https://doi.org/10.1086/262002
- Kamien, M. I., & Schwartz, N. L. (1968). Optimal “induced” technical change. Econometrica, 36(1), 1–17. https://doi.org/10.2307/1909600
- Karabarbounis, L., & Neiman, B. (2014). The global decline of the labor share. The Quarterly Journal of Economics, 129(1), 61–103. https://doi.org/10.1093/qje/qjt032
- Khuc, V. Q., & Tran, Q. B. (2016). Identifying the determinants of forestry growth during the 2001–2014 period. Journal of Agriculture and Rural Development, 12, 2–9.
- Kim, J. U. (2003). Economic growth and returns to scale for reproducible factors. Applied Economics Letters, 10(14), 925–928. https://doi.org/10.1080/1350485032000159031
- Klump, R., & La Grandville, O. (2000). Economic growth and the elasticity of substitution: Two theorems and some suggestions. American Economic Review, 90(1), 282–291. https://doi.org/10.1257/aer.90.1.282
- Klump, R., & Preissler, H. (2000). CES production functions and economic growth. Scandinavian Journal of Economics, 102(1), 41–56. https://doi.org/10.1111/1467-9442.00183
- Kreinovich, V., Thach, N. N., Trung, N. D., & Thanh, D. V. 2019. Beyond Traditional Probabilistic Methods in Economics Springer. https://doi.org/10.1007/978-3-030-04200-4.
- Krueger, A., & Lindhal, M. (2001). Education for growth: Why and for whom? Journal of Economic Literature, 39(4), 1101–1136. https://doi.org/10.1257/jel.39.4.1101
- La Grandville, O. (1989). In quest of the Slutsky Diamond. American Economic Review, 79(3), 468–481.
- Lagomarsino, E. (2017). A study of the approximation and estimation of CES production functions. Ph.D. thesis, Heriot-Watt University.
- Li, D. (2002). Is the AK model still alive? The long-run relation between growth and investment re-examined. Canadian Journal of Economics, 35(1), 92–114. https://doi.org/10.1111/1540-5982.00122
- McFadden, D. (1978). Estimation techniques for the elasticity of substitution and other production parameters. Production Economics: A Dual Approach to Theory and Applications, 2, M. Fuss & D. McFadden (eds). North Holland. Contributions to Economic Analysis, 4(1), 73–123.
- Miller, E. (2008). An Assessment of CES and Cobb-Douglas Production Functions. Congressional Budget Office. Working Paper, https://www.cbo.gov/sites/default/files/cbofiles/ftpdocs/94xx/doc9497/2008-05.pdf
- Mizon, G. E. (1977). Inferential procedures in nonlinear models: An application in a UK industrial cross-section study of factor substitution and returns to scale. Econometrica, 45(5), 1221–1242. https://doi.org/10.2307/1914069
- Nerlove, M. (1967). Recent Empirical Studies of the CES and related production functions. NBER Chapters, In: The Theory and Empirical Analysis of Production, 55–136.
- Nguyen, H. T., & Thach, N. N. (2019). A closer look at the modeling of economics data. In V. Kreinovich, N. Thach, N. Trung, & D. Van Thanh (Eds.), Beyond traditional probabilistic methods in economics. ECONVN 2019. Studies in Computational Intelligence (Vol. 809, pp. 100–112). Springer. https://doi.org/10.1007/978-3-030-04200-4_7
- Nguyen, H. T., Sriboonchitta, S., & Thach, N. N. (2019a). On quantum probability calculus for modeling economic decisions. In V. Kreinovich & S. Sriboonchitta (Eds.), Structural changes and their econometric modeling. TES 2019. Studies in Computational Intelligence (Vol. 808, pp. 18–34). Springer. https://doi.org/10.1007/978-3-030-04263-9_2
- Nguyen, H. T., Trung, N. D., & Thach, N. N. (2019b). Beyond traditional probabilistic methods in econometrics. In V. Kreinovich, N. Thach, N. Trung, & D. Van Thanh (Eds.), Beyond traditional probabilistic methods in economics. ECONVN 2019. Studies in Computational Intelligence (Vol. 809, pp. 3–21). Springer. https://doi.org/10.1007/978-3-030-04200-4_1
- Nguyen, H. T., & Thach, N. N. (2018). A panorama of applied mathematical problems in economics. Thai Journal of Mathematics, Special Issue: Annual Meeting in Mathematics, 1–20.
- Nguyen, Q. H. (2013). Sources of province Hung Yen‘s economic growth. Journal of Economic Development, 275, 28–39.
- Palivos, T., & Karagiannis, G. (2004). The elasticity of substitution in convex models of endogenous growth. Unpublished Manuscript.
- Pereira, C. M. (2003). Empirical essays on the elasticity of substitution, technical change, and economic growth. Ph.D. dissertation, North Carolina State University.
- Pham, L. T., & Phuong, T. L. (2016). Technical efficiency of Vietnamese manufacturing enterprises. Journal of Economics and Development, 229, 43–51.
- Pinheiro, J. C., & Bates, D. M. (2000). Mixed-Effects Models in S and S-PLUS. Springer.
- Pitchford, J. D. (1960). growth and the elasticity of factor substitution. Economic Record, 36(76), 491–503. https://doi.org/10.1111/j.1475-4932.1960.tb00537.x
- Rapping, L. (1965, February). Learning and World War II Production Functions. Review of Economics and Statistics, 47(1), 81–86. https://doi.org/10.2307/1924126
- Roberts, G. O., & Rosenthal, J. S. (2001). Optimal scaling for various Metropolis-Hastings algorithms. Statistical Science, 16(4), 351–367. https://doi.org/10.1214/ss/1015346320
- Romer, P. M. (1986, October). Increasing returns and long-run growth. Journal of Political Economy, 94(5), 1002–1037. https://doi.org/10.1086/261420
- Romer, P. M. (1987). Crazy explanations for the productivity slowdown. In NBER Macroeconomics Annual. S. Fischer (eds). MIT Press.
- Romer, P. M. Endogenous Technical Change. (1990). Journal of Economic Perspective, 8(1), 3–22. Winter. https://doi.org/10.1257/jep.8.1.3
- Romero-Ávila, D. (2009). Productive physical investment and growth: Testing the validity of the AK model from a panel perspective. Applied Economics, 41(23), 3027–3043. https://doi.org/10.1080/00036840701335611
- Romero-Ávila, D. (2013). Is physical investment the key to China’s growth miracle? Economic Inquiry, 51(4), 1948–1971. https://doi.org/10.1111/ecin.12005
- Sato, K. (1963). Growth and the elasticity of factor substitution: A comment– How plausible is imbalanced growth. Economic Record, 39(87), 355–361.
- Schmookler, J. (1966). Invention and Economic Growth. Harvard University Press.
- Searle, A. D. (1946). Productivity changes in selected wartime shipbuilding programs. Monthly Labor Review
- Solow, R. M. A contribution to the theory of economic growth. (1956). Quarterly Journal of Economics, 70(1), 65–94. February. https://doi.org/10.2307/1884513
- Solow, R. M. (1957). Technical Change and the aggregate production function. The Review of Economics and Statistics, 39(3), 312–320. https://doi.org/10.2307/1926047
- Solow, R. M. (1997). Learning from “Learning-by-Doing”. Lessons for Economic Growth. Stanford University Press.
- Sriboonchitta, S., Nguyen, H. T., Kosheleva, O., Kreinovich, V., & Nguyen, T. N. (2019). Quantum approach explains the need for expert knowledge: On the example of econometrics. In V. Kreinovich & S. Sriboonchitta (Eds.), Structural changes and their econometric modeling. TES 2019. Studies in Computational Intelligence (Vol. 808, pp. 191–199). Springer. https://doi.org/10.1007/978-3-030-04263-9_15
- Svítek, M., Kosheleva, O., Kreinovich, V., & Nguyen, T. N. (2019). Why Quantum (Wave Probability) Models Are a Good Description of Many Non-quantum Complex Systems, and How to Go Beyond Quantum Models. In V. Kreinovich, N. Thach, N. Trung, & D. Van Thanh (Eds.), Beyond traditional probabilistic methods in economics. ECONVN 2019. Studies in Computational Intelligence (Vol. 809, pp. 168–175). Springer. https://doi.org/10.1007/978-3-030-04200-4_13
- Swan, T. W. (1956, November). Economic growth and capital accumulation. The Economic Record, 32(2), 334–361. https://doi.org/10.1111/j.1475-4932.1956.tb00434.x
- Temple, J. (1998). Equipment investment and the Solow model. Oxford Economic Papers, 50(1), 39–62. https://doi.org/10.1093/oxfordjournals.oep.a028635
- Thach, N. N. (2020a). Endogenous economic growth: The Arrow-Romer theory and a test on vietnamese economy. WSEAS Transactions on Business and Economics, 17, 374–386. https://doi.org/10.37394/23207.2020.17.37
- Thach, N. N. (2020b). The variable elasticity of substitution function and endogenous growth; an empirical evidence from vietnam. International Journal of Economics and Business Administration, VIII(1), 263–277. https://doi.org/10.35808/ijeba/424
- Thach, N. N. (2020c). How to explain when the ES is lower than one? A Bayesian nonlinear mixed-effects approach. J. Risk Financial Manag, 13(2), 21. https://doi.org/10.3390/jrfm13020021
- Tu, T. G., & Phuc, T. N. (2012). Using the Cobb-Douglas to analyze the impact of inputs on coffee productivity in province DakLak. Journal of Economics and Development, 8, 90–93.
- UNCTAD. (2019). Handbook of Statistics. Accessed 4 April 2020). file:///D:/data/Unctad%20handbook2019.pdf
- United Nations (2019). Human development data. http://hdr.undp.org/en/data# (Accessed 4 April 2020).
- Uselding, P. (1972). Factor Substitution and labor productivity growth in american manufacturing, 1839–1899. The Journal of Economic History, 32(3), 670–681. https://doi.org/10.1017/S0022050700077172
- Valdes, B. (1999). Economic growth: theory, empirics and policy. Edward Elgar.
- Walley, P., Gurrin, L. C., & Burton, P. R. (1996). Analysis of clinical data using imprecise prior probabilities. The Statistician, 45(4), 457–486. https://doi.org/10.2307/2988546
- World Bank. (2019). World Bank National Accounts Data and OECD National Accounts Data Files. available online: https://data.worldbank.org/indicator/NY.GDP.MKTP.KD.ZG?locations=VN (Accessed on 10 January 2020).
- Wright, T. P. (1936). Factors affecting the cost of airplanes. Journal of the Aeronautical Sciences, 3(4), 18–122. https://doi.org/10.2514/8.155
- Yang, R. Y., & Berger, J. O. (1998). [ Unpublished manuscript]. A catalog of noninformative priors. http://www.stats.org.uk//priors/noninformative/YangBerger1998.pdf (Accessed on 18 April 2020)
- Yuhn, K.-H. (1991). Economic growth, technical change biases, and the elasticity of substitution: A test of the la Grandville hypothesis. The Review of Economics and Statistics, 73(2), 340–346. https://doi.org/10.2307/2109526
- Zarembka, P. (1970). On the empirical relevance of the CES production function. Review of Economics and Statistics, 52(1), 47–53. https://doi.org/10.2307/1927596