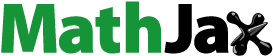
Abstract
The study aims to a comprehensive measure of listed insurance companies’ technical efficiency in GCC countries and benchmarking, peer count summary, and measuring productivity changes in the first stage. In the second stage, the technical efficiency, pure technical efficiency, and scale efficiency scores have been regressed with internal (company variables) and external environmental variables. This paper first examines financial freedom and reinsurance activities on 60 insurers’ efficiency from all GCC countries during 2016–2019. The results suggest a positive impact of economic freedom, solvency on technical efficiency components, and the negative effect of reinsurance ceding, market concentration on GCC insurers’ technical efficiency. In productivity changes, UAE companies are doing well as compared to other countries’ insurers. Overall, GCC insurers’ efficiency is improving with pure technical efficiency having a CAGR of 2.13% and scale efficiency a CAGR of 2.96%. Based on the peer count summary, only the UAE and Saudi Arabia’s companies can be benchmarked against another insurance company. Also, this research is one of the most comprehensive ones in the GCC region; this is the first study on an extensive data set to the best of our knowledge, including conventional insurers operating across GCC countries. This study is based on 60 insurers listed on the stock exchanges of six GCC countries. This will also be the first empirical evidence on the GCC insurers, which measures the impact of company-specific variables and unique environmental variables separately on the two components of technical efficiency using Tobit regression. The positive association of competition and financial freedom with technical efficiency may encourage regulators and policymakers to ensure less government intervention and give more support and freedom for a better competitive insurance industry.
PUBLIC INTEREST STATEMENT
Recent work assessed the efficiency of the Decision-Making Units (DMUs), which are performing similar functions, and finds out the Technical Efficiency (TE), pure technical efficiency (PTE), and Scale efficiency (SE) levels for the Insurance Industry. Evidence as to the best possible strategies to identify inefficient units and provide them suitable remedies with the help of benchmarking and super efficiency techniques.
The companies’ performance for the period under review has been assessed and compared through the use of the Malmquist Productivity Index (MPI) and Tobit Regression. This study adds the various factors that affect the performance and efficiency of the insurance sectors.
1. Introduction
The Gulf Cooperation Council (GCC) countries are a homogeneous group of six countries rich in oil and gas resources and have similarities in language and culture. The GCC countries’ economy is dependent on its oil and gas sector, and all the countries are striving hard to diversify their economy with the help of a well-developed financial sector. The stock market in GCC concentrated in petrochemicals, property/construction, and financial sector companies. Banks mainly dominate GCC’s financial industry; the stock market for equity and debt securities is not healthy and developed. One of the prime sources of funds is commercial banks. The insurance companies are the next critical component of the financial sector in GCC. These insurance companies play a vital role in a country’s economic development by doing risk transfer. They minimize the risk exposure on corporates, allowing banks to provide capital at a lower cost. In this way, insurance helps in better allocation of capital and asset protection. All the GCC countries have long-term strategies to diversify the economy; governments invest and invite FDI into projects. This drive to diversify the economy will help expand the economy, which will increase the number of insurable assets.
GCC has extreme fragmentation and intense competition in its insurance sector. Around 200 big and small insurers are operating in the GCC market; they are different in product offerings, foreign collaboration, and stock market listing. The premium collection lies with a few big insurers; 90 listed insurance companies in GCC control 80.9% of the total insurance premium. The other 114 smaller companies compete for 19.1% of the GCC market (Alpen capital, 2019). Enhancement in financial institutions’ efficiency may lead to higher profits, better pricing, and service quality satisfaction in consumers (Allen & Berger, Citation1992). Efficiency is a critical factor for improving insurers’ profitability. Still, insurers with low-efficiency scores need to get more profit enhancement with slight improvement in efficiency (Eling & Jia, Citation2019). Therefore, these insurers’ improved efficiency will be critical to their success and survival in the market. The presence of foreign players and stiff competition has augmented GCC financial institutions’ relevance and insurers’ effectiveness. The efficient and productive insurance sector can help strengthen the capital market and economic diversification (Rao et al., Citation2010). The efficiency measurement of insurance companies plays a vital role in the insurance industry (Brockett et al., 1996). The insurance companies’ efficiency analysis is of paramount importance to insurers and policymakers as the insurance sector is the fundamental constituent of the financial system supporting its country’s infrastructure.
1.1. Overview of the insurance industry in GCC
UAE and Saudi Arabia are the two leading insurance markets, with 44.3% and 33.6% market share in the region’s gross written premium. The insurance penetration (insurance premium/GDP) and insurance density (per capita insurance premium) are relatively low in GCC countries. According to 2019 data, the UAE and Bahrain are the top two performers with 2.9% and 1.8% insurance penetration, but even these best GCC figures are much below the global insurance penetration of 5.9% (Swiss Re, 2019). Kuwait has the lowest insurance penetration rate, with about 1%. The GCC countries practice the same religion and belief system; all the nations have well-established social welfare system supported by the government. The market share of life insurance products is meager; the non-life insurance premium constitutes 87% of the total insurance premiums written in 2018. Motor and health insurances are the leading non-life segments contributing approximately 50% of the region’s total non-life premiums. The UAE ranks highest in life and non-life insurance penetration in the GCC region with 0.7% and 2.3%, respectively, in 2018.
Another common characteristic of GCC insurers is that most of them are engaged in a substantial reinsurance amount. In 2018, 42.4% of the total premium received was ceded to reinsurers compared to the global average of 5% (Alpen capital, 2019). This extremely low retention ratio in GCC insurers may be due to a lack of expertise and geographic diversification. There are a limited number of insurers with a pan-GCC presence. GCC’s insurance market is mainly driven by health insurance and motor insurance, which are mandatory in GCC. In GCC, the insurance penetration is much below the global average, which provides a growth potential to the insurance sector (Alpen capital, 2019); findings of this study highlight the growth potential and future opportunities in GCC’s insurance sector.
1.2. Rationale of the study
The significance of efficiency for the insurance sector has been studied by authors like Cummins et al. (Citation1999); Barros et al. (Citation2010); Biener and Eling (Citation2011); Barros and Wanke (Citation2014); Cummins and Xie (Citation2010); Kao and Hwang (Citation2008); Luhnen (Citation2009) using a nonparametric technique, Data Envelopment Analysis (DEA). But a few authors have also used a parametric approach, Stochastic Frontier Analysis (SFA) (Cummins and Weiss, Citation2000; Gardner & Grace, Citation1993a; Ennsfellner et al., Citation2004; Fecher et al., Citation1993; Fenn et al., Citation2008), to analyze the efficiency of insurance companies. Several authors like Barros & Wanke (Citation2014); Ariff and Can (Citation2008); Cummins & Xie (Citation2008); and Lai et al. (Citation2011) applied two stages to estimate relative efficiency scores, used DEA or SFA in the first stage to determine the comparable efficiency scores followed by multivariate analysis as the second stage with a set of contextual internal (company-specific) and external variables related to environment and economy. There are very few studies based on GCC insurers in the existing literature, like (Almulhim & McMillan, Citation2019; Benyoussef et al., Citation2019; Naushad et al., Citation2020) based on Saudi Arabian takaful insurers. Rao et al. (Citation2010) measured the managerial efficiency of insurers in the UAE. Al‐Amri et al. (Citation2012) studied the technical efficiency and productivity changes for GCC countries’ 39 insurers during 2005–2007. All these studies are focused on either Saudi Arabia or the UAE insurance market. The one covering the entire GCC market is not representative as the sample size was just 39 insurers. Another point is that Islamic insurance is at the center stage of all these studies. So, there is a need to study the entire GCC market covering the conventional insurers. Therefore, in this article, we attempt to explore the impact of unique company-specific and environmental variables on GCC insurers’ efficiency with a larger and more representative sample.
1.3. Organization of the study
The remaining part of the paper is structured as follows. The second section is devoted to a literature review concerning the insurer’s efficiency and hypothesis development. The third section presents the methodology, data, and variables. The fourth section details the empirical findings. The fifth section deals with discussions and conclusion, and, finally, the sixth section highlights the implications and limitations of the study.
2. Literature review and hypothesis development
There is an extent of literature on the efficiency of financial institutions; the banking sector’s efficiency has been the most researched area. Next to banking efficiency, efficiency and productivity measurement of insurers have also gained the researchers’ attention. It has been observed that most of the studies are focused on the developed market of insurance in the USA and Europe (Gharaei et al., Citation2019; Weiss, Citation1991; Donni and Fecher, 1997; Cummins and Weiss, Citation2000; Cummins and Rubio-Misas, Citation2006; Fenn et al., Citation2008; Luhnen, Citation2009; Kasman & Turgutlu, Citation2009; Vencappa et al., Citation2003; Biener et al., Citation2016; Cummins et al., Citation2017; Eling & Jia, Citation2018). Few studies are from emerging markets like Asia (Boonyasai et al., Citation2002; Tone and Sahoo, Citation2005; Hao & Chou, Citation2005; Wanke & Barros, Citation2016). Very few studies are from underdeveloped markets like Africa and the GCC region (like Barros & Wanke, Citation2014; Barros et al., Citation2014; Al‐Amri et al., Citation2012; Al-Amri, Citation2015; A. L. Alhassan et al., Citation2015).
The efficiency-based studies on insurance companies can be categorized into; efficiency measurement to observe the impact of consolidation or mergers in the industry (Gharaei, Hoseini Shekarabi et al., Citation2020; Cummins et al., Citation1999; Noulas, Citation2001, Citation2004; Bikker, Citation2016) efficiency of insurers in a specific country and cross-country analysis of efficiency, estimation of efficiency determinants (Barros et al., Citation2014, Citation2010; Biener et al., Citation2016; Luhnen, Citation2009; Wanke & Barros, Citation2016) efficiency and market structure relationship (Alhassan & Biekpe, Citation2016; Bikker & Van Leuvensteijn, Citation2008; Choi & Weiss, Citation2005; Cummins et al., Citation2004; Fenn et al., Citation2008; Hoseini Shekarabi et al., Citation2019; A. L. Alhassan et al., Citation2015). This paper is focused on measuring the unique environmental variables and firm-specific variables on the GCC insurers’ technical efficiency. The upcoming section discusses the hypothesized effect of firm-specific variables and external environmental variables on the insurers’ technical efficiency scores.
Naushad et al. (Citation2020) computed the managerial efficiency of 30 insurance companies listed on the Saudi stock exchange from 2015 to 2018. They have applied BCC and CCR models of DEA and revealed that a good number of insurance companies operating in KSA were found to be efficient on managerial efficiency scale. Ilyas and Rajasekaran (Citation2019) examined the Indian general insurance sector in terms of efficiency, productivity and returns to scale economies. They had employed DEA bootstrapped regression to estimated bias-corrected efficiency scores and also applied truncated bootstrapped regression to identify the effect of firm-level characteristics on the efficiency of insurers. Their results revealed that the public insurers were more cost efficient than the private insurers, size and reinsurance have a statistically significant negative relationship with efficiency.
Eling and Jia (Citation2019) disused about the efficiency and profitability in the global insurance industry. They had examined the relationship with a global dataset of over 5000 insurance companies. They have found the significant positive correlation between the efficiency measures and profitability measures. Also, they identified the nonlinear relationship between efficiency and profitability and the marginal impact of efficiency on profitability decrease as the insurer’s efficiency was close to the best practice.
Lee et al. (Citation2019) wanted to identify the factors that influence the efficiency level (cost efficiency and technical efficiency) of the Takaful industry and to examine the effects of Takaful insurance firm’s specific factors that influence the efficiency of Takaful insurance in Malaysia. Their results indicated that Takaful operators in general have allocative inefficiency but family Takaful was more cost efficient than general Takaful.
Benyoussef et al. (Citation2019) measured the relative efficiency of insurance companies in Saudi Arabia in the year 2014. Taking into account two main approaches of this technique and considering a sample of 23 insurance companies, the results revealed that insurance companies did not operate efficiently. Also, Takaful insurance was relatively more efficient than cooperative insurance companies.
Almulhim and McMillan (Citation2019) examined the performance of Saudi Arabia’s insurance market using a two-stage data envelopment analysis to assess the efficiency of the two production stages and accordingly, define the leader stage. Further, it offered key implications for decision makers, regulators, and managers associated with the insurance industry in Saudi Arabia and other emerging insurance markets.
Karbhari et al. (Citation2018) examined the relationship between corporate governance attributes and efficiencies of the global Takaful Insurance operators. They found that CEO/chair duality, board size, organization age, regulatory jurisdiction and firm size have a positive relationship with technical efficiency. Also, they reported that non-executive directors, shariáh board, product diversification and institutional ownership improve the scale efficiency of the Takaful industry in the Middle East North Africa (MENA) and the Southest East Asian (ASEAN) region.
Nourani et al. (Citation2018) measured technical efficiency of insurance companies using dynamic network DEA for the Malaysian insurance companies. They had employed a dynamic network data envelopment analysis for performance evaluation of insurer and ownership structure types, from the year 2007–2014. They have suggested that the high usage of input quantities and lack of total investment as key reasons for log efficiency, particularly among the local insures.
Grmanová and Pukala (Citation2018) discussed the efficiency of insurance companies in the Czech Republic and Poland markets. They had used descriptive statistics, DEA model and Tobit regression model. Also, found that 10 insurance companies were efficient on the common Czech-Polish insurance market. Efficiency scores of Czech insurance companies were greater than the efficiency scores of Polish insurance companies.
Reyna and Fuentes (Citation2018) Examined the cost-efficiency analysis of the insurance industry in Mexico. They wanted to do a comprehensive analysis of cost variations in an intertemporal manner, by breaking them down into the economic sources that produce them, including productivity. Their results demonstrated that some companies achieved cost reductions from technological progress or improvements in efficiency.
2.1. Firm-specific variables
2.1.1. Firm size
The size of the firm or size of the insurance company has been mainly represented by the “log of the total assets” and has been one of the critical control variables in the efficiency scores or the profitability regression (Ansah‐Adu et al., Citation2012; Biener & Eling, Citation2012; Biener et al., Citation2016; Gardner & Grace, Citation1993a; Hao & Chou, Citation2005; Huang & Eling, Citation2013; Kasman & Turgutlu, Citation2009). A more prominent firm may always benefit from economies of scale (Berger et al., Citation1993), which may help achieve a lower per-unit production cost. The optimum usage of assets by more significant firms may lead to an increase in efficiency and an increase in market share. However, challenges in managing large-scale operations may lead to wastages and increased cost (Fama & Jensen, Citation1983); in this scenario, scale diseconomies may reduce efficiency (Halkos & Tzeremes, Citation2007). Another view on a positive size–efficiency relationship is that trust is a predominant factor and usually goes with big firms as the small firms are more susceptible to insolvency (Shujie et al., Citation2007).
Many authors have established a positive effect of firm size on firm efficiency (see Ansah‐Adu et al., Citation2012; Biener & Eling, Citation2012; Biener et al., Citation2016; Hao & Chou, Citation2005; Lundvall & Battese, Citation2000), but still, some of them have a contrary view. Gardner and Grace (Citation1993a) believe that when total assets increase, inefficiency rises; similarly, Diacon, Starkey, O’Brien et al. (Citation2002a) believe that a lower scale efficiency can be a feature of small and large firms. There is no conclusive evidence on the relationship between size and the insurer’s profitability.
H1: Size has a significant relationship with technical efficiency
2.1.2. Solvency
The solvency measure is always an important financial indicator that can directly influence the cost of capital, risk, and profitability. Stockholder’s Equity to Assets (SEA) ratio has been frequently used as an indicator of solvency in the second stage of DEA analysis, truncated regression analysis (Biener & Eling, Citation2012; Huang & Eling, Citation2013; Luhnen, Citation2009). Leverage has always been considered a double-edged sword, so theoretically, it can have a positive and negative coefficient in efficiency regression. Insurers with a high insolvency risk are less likely to attract risk-averse policyholders, resulting in a negative leverage–efficiency relationship (Berger et al., Citation2000). The findings on leverage-efficiency connection indicate a positive impact of SEA ratio on the technical and cost-efficiency scores of the insurers (see Kasman & Turgutlu, Citation2009; Huang & Eling, Citation2013; Biener & Eling, Citation2012; Grmanová & Pukala, Citation2018) as a rise in SEA ratio is a reflection of less risk (Fries & Taci, Citation2005). The two studies have concluded the significant negative influence of capital structure on cost efficiency; Barros et al. (Citation2010) in Greece and Ansah‐Adu et al. (Citation2012) in Ghana. Similarly, Cummins et al. (Citation2010) established a significant negative effect of solvency on technical efficiency (TE), pure technical efficiency (PTE), and scale efficiency (SE) of insurers in the USA. Following Biener and Eling (Citation2012) and Huang and Eling (Citation2013), we expect a positive impact of solvency on efficiency.
H2: Solvency has a significant positive relationship with technical efficiency
2.1.3. Reinsurance activities
All the insurers worldwide are opting for reinsurance activities; the percentage of business reinsured depends typically upon the insurer’s risk-taking capacity and the risk involved in the new business. Reinsurance transactions provide a buffer and enhancement in the ceding company (Choi & Weiss, Citation2005) and reduce the risk of insolvency for small insurers (Kader et al., Citation2010). The percentage of the total written premium ceded to the reinsurers will affect the ceding company’s likelihood and income. The reinsurance–efficiency relationship depends upon the trade-off between the risk diversification in the policy pool and the previous income to reinsurers. The sign of the reinsurance coefficient can be positive or negative.
Cummins et al. (Citation2010) used the “percentage of premiums reinsured” as one of the US insurance industry’s control variables. They regressed the TE, PTE, and SE for life-health insurers and property-liability insurers separately. In both cases, reinsurance was inversely related to all three types of efficiency scores. This negative relationship may be due to the sharing of profit with the reinsurers. However, in the study done by M. M. Jaloudi (Citation2019), it was concluded that reinsurance is insignificant to insurers’ efficiency. We expect a negative relationship for reinsurance like Cummins et al. (Citation2010) and (Ilyas & Rajasekaran, Citation2019)
H3: Reinsurance activities has a significant negative relationship with technical efficiency
2.1.4. Age of the company
The company’s age is the number of years of its establishment; some authors have also considered age as one of its determinants of efficiency and performance. In their study on Taiwanese banks, (Chiu and Chen (Citation2009) argued that the number of established years would directly correlate with firm efficiency due to customers’ higher confidence in older banks. A similar argument in the insurance sector companies has also been proposed (Arrow, Citation1962; Jovanovic, Citation1982). The trust of the insurance companies is one of the critical factors behind policy purchases, and that is developed by building a long-term relationship with customers (Alhassan & Biekpe, Citation2016). In the literature, the age–efficiency relationship has been confirmed to be positive and negative (Lundvall & Battese, Citation2000). Biener et al. (Citation2016) regressed the technical, revenue, cost efficiency of life, property & causality (p/c), and reinsurance sectors for Swiss insurance companies. They found mixed results for the age–efficiency relationship in the companies. There was a significant positive relationship between p/c insurance and a negative correlation between reinsurance and life insurance companies. Recent studies by (Alhassan & Biekpe, Citation2016) have confirmed a negative connection, while Barros et al. (Citation2014) have established a positive age–efficiency relationship.
H4: The age of the company has a significant relationship with technical efficiency
2.2. External environmental variables
2.2.1. Insurance development indicators
Environmental variables are out of the manager’s control, but they play a crucial role in business operations and firm performance. In the insurance sector, insurance density and insurance penetration are the two well-known development indicators that exist. Penetration rate and insurance density can be considered environmental variables for an insurance company (Shieh et al., Citation2020; (Copeland & Cabanda, Citation2018). An increase in insurance penetration may increase moral hazard and resource scarcity. Studies like (Biener & Eling, Citation2012; Cummins et al., Citation2017; Shieh et al., Citation2020) have shown insurance density and penetration as the significant efficiency determinants of insurers. Following the results of Biener and Eling (Citation2012), we are expecting a positive relationship for insurance density and unfavorable for insurance penetration.
H5 a: Insurance density has a significant positive relationship with technical efficiency
H5 b: Insurance penetration has a significant negative relationship with technical efficiency
2.2.2. Market concentration
The changes in insurer’s efficiency are reflected in the level of market competition, and “increased competition would force insurance firms to drive up their efficiency” (Cummins et al., Citation2017). In a competitive market, efficient firms have the upper hand in business performance, which helps achieve a more significant market share (Cummins et al., Citation2017); therefore, efficiency is also considered an indirect measure of competition. The higher market concentration or market power means lesser competition in the market. Concentration ratios have been commonly used in the banking and insurance industry to measure competition and market power (Berger and Hannan, Citation1989; Bajtelsmit and Bouzouita, Citation1998; Bikker & Van Leuvensteijn, Citation2008; Fenn et al., Citation2008; A. L. Alhassan et al., Citation2015). Herfindahl–Hirschman Index (HHI) is considered a superior measure for market concentration (Stigler, Citation1964). The HHI as a concentration measure has been accepted by (Choi & Weiss, Citation2005; Hay and Liu, Citation1997; Kasman & Turgutlu, Citation2009; A. L. Alhassan et al., Citation2015; Bikker, Citation2016; Biener et al., Citation2016).
The quiet-life (QL) hypothesis (Hicks, Citation1935) defines the relationship between market concentration and firm efficiency. The QL hypothesis suggests that management of the firms with higher market power enjoys the quiet life or, in other words, they work in a more relaxed environment. This comfortable situation may sometimes allow the existence of inefficient managers in the system or, in other cases, the policies preferring growth rate over profit-making and employee retention with higher salaries (Berger and Hannan, 1998); all these reasons lead to the reduction of efficiency in the organization. Therefore, the QL hypothesis supports the negative relationship between market concentration and firm profitability. One of the pioneering works on the effect of market power on insurers’ efficiency was Hao and Chou (Citation2005), where authors established the negative and significant impacts of the game on Taiwanese life insurers and supported the QL hypothesis.
Similarly, in banking, Nguyen and Stewart (Citation2013) also supported the QL hypothesis by using concentration ratios based on bank loans. Alshammari et al. (Citation2019) also tested the QL hypothesis by showing a positive relationship between HHI and GCC insurers’ inefficiency scores. Thus, following the work of (Hao & Chou, Citation2005; Alshammari et al., Citation2019; Mandal & Ghosh Dastidar, Citation2014), we expect a significant negative relationship between market concentration and efficiency.
H6: Concentration has a significant negative impact on technical efficiency
2.2.3. Regulatory environment
The banking and financial industry always has regulators. The degree of freedom and liberty for financial institutions depends on these regulators’ rules and regulations worldwide. The country’s regulatory environment can affect competition in the market and mainly access to foreign players. The Heritage Financial Freedom Index (FFI) has been selected as a proxy for the regulatory environment, which captures the country’s financial freedom (see Heritage Foundation, 2020). The value of FFI ranges from 0 to 100, with 100 means negligible government interference, 50 means considerable government interference, and 20 indicates substantial government interference, and so on. The FFI index’s value captures the extent of direct and indirect government interference in banking and financial services and openness to foreign firms (Chortareas et al., Citation2013). FFI is an indicator of the extent of government regulation of financial services, banking, insurers, and capital markets (Gaganis et al., Citation2013; Huang & Eling, Citation2013). FFI has been used as a proxy of insurance regulations in studies like (Huang & Eling, Citation2013; Park et al., Citation2002; Trinh et al., Citation2015), and it has been used as a predictor of insurer’s efficiency in (Gaganis et al., Citation2013; Lin et al., Citation2016). In Gaganis et al. (Citation2013), the FFI was negatively significant on cost inefficiency. In Lin et al. (Citation2016), there was a mixed relationship with cost efficiency; before the financial crisis, there was a positive relationship, and after the problem, negative. FFI has also been used to determine insurance companies’ financial soundness in Pasiouras and Gaganis (Citation2013). Even in the banking sector study (Chortareas et al., Citation2013), the results were similar, a positive relationship between FFI and bank efficiency and bank performance. Therefore, based on previous studies (Chortareas et al., Citation2013; Gaganis et al., Citation2013; Karbhari et al., Citation2018; Lin et al., Citation2016), we expect a positive relationship for FFI.
H7: Financial freedom has a significant positive impact on technical efficiency
2.2.4. Macroeconomic environment
The macroeconomic environment of a country is always one of the key determinants for the profitability and growth of industries. The demand for bank loans and general insurance coverage should increase with the increase in economic activities. Similarly, an increase in economic activities means an increase in per capita income, growing savings, and life insurance purchases (Beck & Webb, Citation2003; Esho et al., Citation2004). Following the same justification, many previous studies have measured the effect of GDP and other macroeconomic variables on insurance firms’ profitability and efficiency (Dorofti & Jakubik, Citation2015; Ismail et al., Citation2018). Hasan et al. (Citation2018) studied the effect of macroeconomic variables, including the “GDP growth rate” on 32 public insurance companies’ performance throughout 2009–2015. In his findings, he suggested that GDP growth was insignificant on the firm performance. In one of the studies, Al‐Amri et al. (Citation2012) stated that the GDP’s rapid growth rate had enhanced GCC countries’ insurance industry growth. Therefore, following the theory and studies (Beck & Webb, Citation2003; Esho et al., Citation2004), we expect a positive GDP relationship.
H8: GDP has a significant positive impact on technical efficiency
2.3. Research gap of the study
The paper will make three main contributions to the literature. To the best of our knowledge, this will be the first study on an extensive data set, including conventional insurers operating across GCC countries. The previous efficiency-based studies in the GCC region are primarily done for Takaful insurance (Almulhim & McMillan, Citation2019; Benyoussef et al., Citation2019), and most of them covered only Saudi Arabia and UAE insurers. This study is based on 60 insurers listed on the stock exchanges of six GCC countries. Second, this will be the first empirical evidence on the GCC insurers, which measures the impact of company-specific variables and unique environmental variables separately on the two components of technical efficiency using Tobit regression. The effect of the reinsurance variable has not been studied so far in GCC. The GCC market shows more dependence on reinsurance (42.4% of the total premium received was ceded to reinsurers compared to the global average of 5% in 2018) than the rest of the world. This fact has motivated us to investigate the effect of reinsurance activities on GCC insurers’ efficiency scores. Thirdly, studying the impact of market regulations on GCC insurers’ efficiency scores also seems justified as, except for the UAE and Qatar, the rest of the GCC countries have lesser economic freedom, especially Saudi Arabia. There is a clear gap in the existing literature; none of the previous studies have captured the effect of regulations and reinsurance on GCC insurers’ efficiency. Lastly, this study is also testing a quite-life hypothesis on technical efficiency scores. It is very comprehensive in terms of different techniques used like benchmarking, peer-count, and productivity index analysis to get the most recent and more in-depth insights about the GCC region’s insurers. Thus, the current paper will enrich the literature with its findings on the underdeveloped insurance market, having a lot of growth potential.
3. Research methodology and approach
The present analysis considered the Insurance companies operating in six Gulf countries, namely Saudi Arabia, UAE, Qatar, Kuwait, Bahrain, and Oman, listed on nationalized Stock Exchange. The sector comprises 60 companies emphasizing insurance activities. We have included 26 companies listed in Saudi Stock Exchange “Tadawul,” 17 companies listed in Abu Dhabi Securities Exchange from the UAE, 3 companies from the Qatar Stock Exchange, 4 companies from the Kuwait Stock Exchange, 3 companies from the Bahrain Stock Exchange, and 7 companies from Muscat Securities Market, Oman. The reinsurance companies, takaful insurance companies, and the companies with missing data were excluded from the sample.
In GCC countries, before 2016, most of the health expenditure was borne by the GCC governments. Against the backdrop of low oil prices, all the GCC states changed the law. A mandatory health insurance regime was implemented in 2016, where everyone, including expatriates, must get private health insurance from his employer. Health insurance is one of the significant premium contributors in the insurance business of GCC. To keep uniformity in the data, a time before 2016 is not considered in the sample data. Thus, the data used in the study is from 2016 to 2019.
In the current study, the DEA technique has been used to measure GCC insurers’ technical efficiency. DEA is the best technique used for measuring performance for service or manufacturing sectors in recent years. Initially, DEA was developed by Farrell (1957)Footnote1 and was extended by Charnes et al. (1978)Footnote2 and Banker et al. (1984),Footnote3 the CCR (Charnes, Cooper, and Rhodes) model, and the BCC (Banker, Charnes, and Cooper) model.
4. Approach
4.1. Data Development Analysis (DEA)
The input-oriented BCC model can be stated as:
Min
Subject to:
is unrestricted in sign, and
,
,
where is slack in the ith input of the target unit,
is slack in the rth output of the target firm,
are non-negative dual variables,
is the simultaneous adjustment applied to all outputs of the target unit, which leads to a radial movement towards the envelopment surface. The BCC model is the dual of the CCR model along with an added convexity constraint of
4.2. First stage analysis
The input-oriented CCR (constant returns to scale) and the BCC models (variable returns to scale) have been used initially to get overall technical scores (OTE) and pure technical efficiencies (PTE) of GCC insurers from 2016 to 2019. Later, scale efficiency (SE) scores were calculated by the TE scores with PTE scores so that companies can even identify their scale or size, whether it must be increased, decreased, or remains constant. After getting the relative efficiency scores, the benchmarking technique was applied, which provides the insurers with a clear picture of what they need to do to function efficiently and utilize their resources judiciously and efficiently. A peer count summary has also been used in this study to set efficient firms as references for inefficient firms. The summary of peers presents the value and the connection of the organizations that are to be adopted. Therefore, the percentage variation indicates the peer weights to embrace the reference organization’s trend to becoming efficient. The peer count shows the number of times an efficient organization acts as a reference for inefficient organizations. In previous studies, inputs such as capital and gross premiums represent the resources used to produce the insurance’s output (Allen & Berger, Citation1992; Reyna & Fuentes, Citation2018). Also, claims and investment income have been used as an output by some other authors like Duan et al. (Citation2018), Diacon, Starkey, O’Brien et al. (Citation2002a), A.s. (Citation1999), Gardner and Grace (Citation1993a), and Fukuyama (Citation1997). The following input variables, namely, total operating expenses, debt and owner’s equity, and full technical provisions and Outputs variables like net earned premiums and investments income have also been used in efficiency measurement with DEA by the following authors in their research (Gharaei et al., Citation2019; Yang, Citation2006; A. L. Alhassan et al., Citation2015; M. M. Jaloudi, Citation2019; Hannah & Yeung, Citation1998a).
In this study, input orientation has been used to control the cost elements, that is, labor, materials, and other service sectors’ expenses. Also, organizations are more concerned about minimizing the input costs to achieve the standard outputs. Concerning the existing literature and estimating the companies’ technical efficiency scores, the two output variables, namely investment income and net premium earned, and three input variables, general and administrative expenses, gross claim paid, and total assets, have been considered. As per the rule of thumb exhibits in the DEA, this dataset validates the direction two times of several inputs and outputs.
The results of descriptive statistics of the input and output variables are shown in .
Table Ia. Descriptive statistics of the variables (in millions USD)
4.3. Second stage analysis
Lastly, as a stage two analysis, the TE, PTE, and SE scores are regressed using the Tobit regression method with selected company-specific and environmental variables. displays the independent variables’ list with a description of variables and their expected and actual relationship with efficiency scores followed by the following author in their research (Gharaei, Karimi et al., Citation2020; (Nourani et al., Citation2018).
Table Ib. List of independent variables in the Tobit regression
5. Analysis and findings
5.1. Overall and pure technical efficiency
described the input-oriented OTE and PTE scores for all 60 insurers from 2016 to 2019. Based on CCR input-oriented model for the year 2016, only six insurers, namely A2, A6, A12, A22, A27, and A43, were found efficient with a score of 1 out of 60 units; it is astonishing and exciting to know that only 10 percent units were found efficient. In 2017, seven insurers, namely A2, A11, A12, A13, A25, A27, and A43, were found efficient with a score of 1. In 2018, seven insurers, namely A5, A11, A12, A13, A19, A43, and A47, were found efficient with the score of 1, and in 2019, ten insurers, namely A5, A6, A11, A12, A14, A25, A29, A33, A41, and A43 were found efficient with the score of 1, it shows the highest number of efficient insurers from last 4 years. However, only two insurers, namely A12 and A43, have been found efficient consistently during the sample period.
Table 2. Summary of efficiency scores from input-oriented model
Based on BCC input-oriented model for 2016, 18 insurers were found efficient with a score of 1 out of 60 units; 30% of insurers were found efficient. In 2017, 20 insurers were found efficient, with a score of 1. In 2018, 22 insurers were found efficient with a score of 1, and in 2019, 24 insurers were found efficient with a score of 1; it shows the highest number of efficient insurers from the last four years. Under the BCC model, more insurers have been found efficient because of their unique properties and assumption (variable return to scale). However, 10 insurers out of 60, namely A6, A12, A25, A34, A35, A41, A43, A29, A52, and A56, have been found efficient consistently during the sample period from 2016 to 2019.
5.2. Scale efficiency
The SE scores are displayed in , computed by TE scores’ division by the PTE scores. According to the reported results, 9 out of 60 companies are scale efficient in the year 2016. Seven companies were scaling efficiently in 2017, and seven companies were range efficient in 2018. Twelve companies maintained their scale efficiency in 2019, which is the most efficient year based on their scale efficiency score. However, only two companies, namely A12 and A43, were found to be scale efficient, consistently for the 4 years starting from 2016 to 2019.
Table 3. Scale efficiency scores and returns to scale
The companies operating for more profits to scale, decreasing returns to scale, demonstrate that companies should reduce their scale and consistent performance to scale describes that there is no change is required to operate their business. Based on , from 2016 to 2019, we can conclude that the following companies need to increase their size in the form of revenue, income, or market share to become efficient as compared to other companies, namely A1, A3, A4, A8, A9, A10, A15, A16, A17, A18, A20, and A21.
The companies A24, A27, A30, A33, A34, A35, A37, A40, A41, A48, A49, A50, A54, A55, A56, A57, A58, A59, and A60 have been found decreasing return to scale from 2016 to 2019. A12 and A43 have produced constant returns to scale, so they do not need to enlarge their operation or size. With the current flow of revenue, they can achieve the efficiency level.
Further, the average PTE and SE scores were calculated for each year during the sample period, 2016–2019. They compounded annual growth rate (CAGR) calculation on PTE’s average score, and SE shows an increasing trend for efficiencies. The CAGR for PTE from 2016 to 2019 is 2.13%, and the CAGR for SE is 2.96%, an overall increasing trend for all the GCC insurers.
5.3. Benchmarking
After running DEA, 51 inefficient firms need to be benchmarked to know the most appropriate input to be used by them. Only nine relatively efficient firms, namely A5, A6, A11, A12, A14, A25, A29, A33, A41, A43, do not need to minimize their inputs. They are efficiently using these inputs against revenue and investment.
From the technical efficiency scores, the following companies need to minimize their inputs from 1% to 10%: A1, A2, A3, A13, A20, A36, and A47; between 0% and 20%: A7, A10, A19, A26, A35, A48, and A49; between 0% and 30%: A4, A9, A17, A21, A22, A23, A28, A45, A50, A53, and A59; between 0% and 40%: A8, A16, A18, A31, A32, A34, A38, A56, and A57; between 0% and 50%: A15, A27, A30. However, we can also identify that companies recorded the most inefficient and need more than 50% to minimize their inputs to become efficient, namely A24, A37, A39, A40, A42, A44, A46, A51, A54, A55, A58, A60, infers that the company is producing 50% less than the efficient production level.
For all the inefficient insurers, the actual general and administrative (G&A) expenses are more than the projected G&A expenses. Based on the comparison, we can say that Kuwait and Saudi Arabia insurers are doing better in managing G&A expenses as the gap between actual and projected costs is more diminutive. In Kuwait, only one (A50) out of four insurers are suggested to cut down their G&A expenses by 50% or more to become efficient. Among Saudi Arabian insurers, only six (A7, A19, A21, A23, A24, and A26) out of 26 insurers are suggested to cut down their G&A expenses by 50% or more efficiently. The insurers in Qatar are the worst in managing G&A expenses as 3 out of 3 companies are suggested to cut down their G&A costs by 70% or more to become efficient, followed by Omani insurers as five (A54, A55, A56, A58 & A60) out of seven insurers are suggested to cut down their G&A expenses by 50% or more to become efficient. In the UAE, nine (A27, A30, A35, A36, A37, A38, A39, A40 and A42) out of 17 insurers are suggested to cut down their G&A expenses by 50% or more to become efficient. A similar observation is visible in “total assets”; Kuwait and Saudi Arabian insurers are relatively better in asset management than the UAE, Oman, Bahrain, and Qatar.
5.4. Peer count summary
We further analyzed the peer group activities to identify the best-performing insurance companies among all 60 GCC insurers for 2019. Efficiency scores are relative scores; a company can be termed a benchmark company for its group companies if it appears in the peer group (Mostafa, Citation2019). demonstrates the insurers, score, peer count, and its summary among the peer group.
Table 4. Peer count summary for the year 2019 by CCR input-oriented method
Based on the peer group activities, only nine companies are on peer count summary with a reasonably good number of 60 insurance companies for the year 2019. A6 (11 times), A11 (18 times), A12 (4 times), A14 (23 times), A25 (29 times), A29 (03 times), A33 (06 times), A41 (31 times), and A43 (37 times) are found to be highly robust insurance companies with a high peer count. They can be used as a benchmark for inefficient insurance companies. The remaining insurance companies, which are not in the “peer count summary,” are the insurers, which cannot be benchmarked against another insurance company. Either they are providing special services or operating on a small size or level of business.
Out of a total of nine companies, five are from Saudi Arabia, and four are from the UAE; Abu Dhabi national insurance company appears highest peer count (37 times), followed by Al Fujairah National Insurance (31 times) and Walaa Cooperative Insurance Co. (29 times). We have not found any insurance company from the remaining four countries which can be benchmarked against another insurance company.
5.5. Malmquist Productivity Index (MPI)
The Malmquist Productivity Index (MPI) created in the year 1953 shows the growth of the DMUs by representing the Total Factor Productivity (TFP). In TFP, both progress and regress of the efficiency and the frontier technology are assessed under multiple inputs and outputs. MPI gauges the variation in the productivity of a DMU over a period. It is calculated as a product of two aspects—Catch up and Frontier shift. The Catch up (or recovery) denotes the progress that a DMU has made during a period. If the Catch up is more significant than one, it indicates growth in relative efficiency from period 1 to 2; otherwise, it shows no change and regress in relative efficiency. The frontier shift shows the difference in the frontier technology around the DMU from one period to another. displayed the annual means of all the 60 general insurance companies for 2016, 2017, 2018, and 2019 on all five parameters. The results show that productivity was higher in the year 2019 (1.052 MPI), followed by the year 2017 (1.01 MPI), the year 2018 (0.977 MPI). However, productivity was lower in the year 2018.
Table 5. Malmquist index summary of annual means from 2016 to 2019
[Note that all Malmquist index averages are geometric means]
Further information on country-wise productivity changes is displayed in ; it seems that the mean value of technical efficiency change (1.09) for the UAE is the highest among GCC countries. Insurance companies operating in the UAE are most technically efficient compared to other GCC insurers. The mean value of technological changes (1.056) for Qatar is highest among GCC; Qatar’s insurance companies are the most technologically efficient than other GCC insurers. However, the mean value of pure technical change for Saudi Arabia (1.04) is the highest, the mean weight of scale efficiency (1.08), and the Malmquist index (1.06) are the highest for the UAE as compared to other GCC.
Table 6. Country-wise Malmquist index summary 2016–2019
On analyzing at the company level, it is observed that 29 out of 60 firms have not shown any improvement in their MPI score during the period 2016–2019. A27 has registered the highest decline in the MPI score (0.685), followed by the A22 (0.713) and A60 (0.735). On the other hand, A54 (1.735) and A33 (1.577) are the most productive DMUs during the past 4 years, 2016–2019. UAE insurance firm A33 has registered the highest technical efficiency change, pure technical change, and scale efficiency change during 2016–2019. However, UAE insurance firm A27 reported a decline in all the categories. Among Saudi firms, A4 and A5 have registered productivity improvement during 2016–2019.
5.6. Tobit regression
Probit is used when the dependent variable is binary (true/false one/zero). Tobit is used when the dependent variable is continuous but bounded/cut off at one end. Tobit is for continuous y variables but censored. Obit relies on the underlying y being normally distributed and standard errors being homoscedastic. Also, the Tobit model evolved out of the Probit model, and the limited and quantal response methods share many properties and characteristics; they are sufficiently different to make separate treatment more convenient
To examine the hypothesis that insurance company efficiency, the Tobit regression has been used to identify factors affecting insurance companies’ efficiency in GCC. The DEA model was selected from balance sheets. Still, the Tobit model variables are independent of this establishing the separation between efficiency drivers and balance sheet variables that characterize
In the second stage of the analysis for testing the hypothesis regarding the influence of company-specific variables and environmental variables on the GCC insurers’ technical efficiency, we have selected Tobit regression (like Diacon, Starkey, O’Brien et al., Citation2002a). The Tobit regression has been chosen because the efficiency scores are truncated scores between 0 and 1. With the help of the Eview-11 edition, the results of Tobit Regression have been reported in this study. The equations mentioned below represent the six Tobit models used in the study. EquationEquation (1)(1)
(1) represents the Tobit model used for measuring the effect of company-specific variables on the three efficiency scores: overall technical efficiency (OTE), Pure Technical Efficiency (PTE), and Scale Efficiency (SE) for the period 2016 to 2019. EquationEquation (2)
(2)
(2) represents the second model used to measure environmental variables’ effect on GCC insurers’ said efficiency scores.
The equation is given below:
In the second stage (), we have estimated a Tobit regression with the three efficiency scores regressed in terms of characteristics of the insurance companies, i.e., company age, company size, solvency, and reinsurance ceded. Contrary to (Lee et al., Citation2019) on firm size, in this study, the pure technical efficiency scores are positively related to company size, while scale efficiency scores are negatively related. The negative relationship of “company size” with scale efficiency indicates that more prominent companies have higher scale inefficiency, consistent with Biener et al. (Citation2016), and Diacon, Starkey, O’Brien et al. (Citation2002a). The solvency ratio (stockholder equity to assets) is significantly positive for both SE and PTE scores, which means higher solvency leads to favorable selection and efficiency, results support the previous findings (Biener & Eling, Citation2012; Huang & Eling, Citation2013; Kasman & Turgutlu, Citation2009) but contradict with Cummins et al. (Citation2010). The variable “reinsurance ceded” is negatively related to SE and OTE (like Ilyas & Rajasekaran, Citation2019) but positively related to PTE; this partially supports the results of Cummins et al. (Citation2010). The negative relationship of reinsurance reflects the loss of profit to GCC insurers as they share a significant percentage of earnings with their reinsurers. The company’s age does not impact the company’s productivity. Whether it is old or new, it is immaterial for efficiency; this contradicts the findings of Ilyas and Rajasekaran (Citation2019) on “age of the company” significantly positive with technical efficiency.
Table 7. Tobit regression results with company-specific variables
, GDP PPP, HHI, Insurance density, insurance penetration, and financial freedom index have been used as independent variables against the OTE, PTE, and SE scores of GCC insurance companies. All the selected environmental variables are having a significant relationship with efficiency scores. Insurance penetration is significant and negative for all types of efficiency scores and has the highest impact on efficiency, whereas insurance density is positively related to efficiency scores showing consistency (Biener & Eling, Citation2012; Cummins et al., Citation2017). In this study, FFI has been used as a proxy of insurance regulations (like Huang & Eling, Citation2013; Park et al., Citation2002; Trinh et al., Citation2015); FFI is positively significant for OTE and PTE while negative for SE score. The results on FFI justify the importance of financial freedom for insurers in GCC countries, and they support the previous studies (like Chortareas et al., Citation2013; Gaganis et al., Citation2013; Lin et al., Citation2016). A high concentration ratio indicates less competition; therefore, the relationship between HHI and efficiency will be the opposite of competition and efficiency. Based on the results, we can say that higher concentration or market power for the insurers causes inactive management or increasing inefficiency. The HHI coefficient supports the quite-life hypothesis and previous studies (like Alshammari et al., Citation2019; Hao & Chou, Citation2005). GDP is also positively significant for OTE and PTE scores while insignificant for SE scores.
Table 8. Tobit regression results with environmental variables
6. Managerial implications
The study reveals the importance of solvency for insurers as the increase in solvency positively affects efficiency. The insurer’s reputation is the foundation on which customers’ trust and confidence can be built. Solvency is one of the critical financial variables for building reputation and goodwill in customers. It is suggested that management should keep a check on their solvency ratio to attract genuinely and risk-averse policyholders. Another prominent finding is about “reinsurance ceded,” which shows a negative relationship with efficiency scores. GCC insurers heavily use the reinsurance route to minimize and diversify their risk; due to this, they share a big chunk of premium with the reinsurers. Therefore, it is recommended that GCC insurers invest in developing their risk management capabilities or revise their underwriting criteria to reduce reinsurance dependence. In environmental variables, insurance density, FFI, and competition are positively related to the efficiency scores. Therefore, the government in GCC countries should increase the insurance activities to their fullest; there should be an increased awareness about the insurance products and their benefits. This will boost the premium income and healthy competition in the sector and improve insurers’ better profitability and efficiency. Governments in GCC should also consider giving more financial freedom and less government intervention in financial institutions’ decision-making. The benchmarking results have revealed that Kuwait and Saudi Arabia insurers are doing better managing their general & administrative (G&A) expenses as the gap between actual and projected costs is less compared to other countries. The managers of Qatar and Oman’s insurance companies should be vigilant in handling G&A expenses as most insurers are suggested to cut down their costs by more than 50%. Management of these insurance companies should invest in new technology to improve efficiency to reduce G & A costs.
7. Discussion and conclusion
The study aims at the comprehensive measure of technical efficiency of listed GCC insurers along with benchmarking, peer count summary, and productivity changes in the first stage. The second stage is regressing the (CCR) or TE and (BCC) or PTE scores and SE scores with company-specific and environmental variables. If we compare the TE and PTE scores of GCC insurers, it is observed that their PTE scores are much better than TE scores. Since PTE is an index to capture managerial performance (Kumar & Gulati, Citation2008), we can say that administrative inefficiency is not the primary reason behind GCC insurers’ inefficiency. Scale inefficiency is typical among GCC insurers; they do not use the optimum size of resources, either too big or small. Another critical observation about GCC insurers is that their PTE (CAGR 2.13%) and SE (CAGR 2.96%) show an increasing trend over the sample period, which is a positive sign for this emerging insurance market. In the scale efficiency table, it is evident that all the Omani companies have scale inefficiency of the DRS type. This means that insurance companies are oversized; similarly, three out of four listed insurance companies of Kuwait are also too big, so there scale inefficiency.
On the contrary, 12 out of 26 Saudi insurers have IRS scale inefficiency, which suggests that these Saudi insurers should increase their size in revenue, income, or market share. The two insurance companies, “Bupa cooperative insurance (A12)” and “Abu Dhabi national insurance (A43),” are scale-efficient throughout the sample period. Based on the peer group activities, only four companies are on peer count summary with a reasonably good number for 2018. A11, A16, A10, and A6 are highly robust insurance companies with a high peer count. They can be used as a benchmark for all inefficient GCC insurance companies. Among the firm-specific variables, “age of the firm” has proved insignificant for the firm efficiency. Company size is negatively significant for scale efficiency, indicating that larger insurance firms have higher scale inefficiency, consistent with Biener et al. (Citation2016) and Diacon, Starkey, O’Brien et al. (Citation2002a). The other key variable: reinsurance ceded is negative and significant, an indication of loss of profit to GCC insurers. The GCC insurers need to focus on developing their risk management skills to lessen their dependency on reinsurance and avoid revenue sharing with reinsurers. The financial freedom in GCC needs to be enhanced as this will help increase the efficiency of the insurers, improve economic activities is also positively related to the efficiency of the insurers. The insurance density is positively associated with efficiency scores showing consistency (Biener & Eling, Citation2012; Cummins et al., Citation2017). The study supports the “Quiet Life” hypothesis, higher competition leading to higher GCC insurance companies’ efficiency.
8. Limitations
Although this study is one of the most comprehensive ones in the GCC region, having a few limitations like a more significant period could have given better results. The study is focused only on listed companies and has excluded non-listed companies. Future research in this area is possible using a different metric for market competition and other forms of efficiency like cost and revenue efficiency. Researchers can also apply a similar analysis, separately on life insurance, health insurance, and general insurance business in GCC. That may give a better understanding of insurance efficiency and its determinants.
Additional information
Funding
Notes on contributors

Rohit Bansal
Dr. Rohit Bansal holds a doctoral degree in Corporate Finance from the Indian Institute of Technology, Roorkee. He possesses a rich professional experience of over 10 years in Finance, Accounting, and Econometrics. He has published many articles & research papers in various international and refereed journals of repute Elsevier, Taylor & Francis, Springer, Sage, Emerald, and IGI Global, most of them listed in ABDC and Scopus. His research area includes corporate finance, stock markets, and financial markets. He also serves as a reviewer for select journals.
Notes
1. Farrell, M.J. (1957), “The measurement of productive efficiency”, Journal of the Royal, Statistical Society, Series A (General), Vol. 120 No. 3, pp. 253–290.
2. Charnes, A., Cooper, W.W. and Rhodes, E. (1978), “Measuring the efficiency of decision making units”, European Journal of Operational Research, Vol. 2 No. 6, pp. 429–444.
3. Banker, R.D., Charnes, A. and Cooper, W.W. (1984), “Some models for estimating technical and scale inefficiencies in data envelopment analysis”, Management Science, Vol. 30 No. 9, pp. 1078–1092.
References
- A.s., V. (1999). Canadian life and health insurance productivity evaluation using data envelopment analysis. University of Toronto.
- Al‐Amri, K., Gattoufi, S., & Al‐Muharrami, S. (2012). Analyzing the technical efficiency of insurance companies in GCC. The Journal of Risk Finance, 13(4), 362–380. https://doi.org/10.1108/15265941211254471
- Alhassan, A. L., Addisson, G. K., & Asamoah, M. E. (2015). Market structure, efficiency and profitability of insurance companies in Ghana. International Journal of Emerging Markets, 10(4), 648–669. https://doi.org/10.1108/IJoEM-06-2014-0173
- Alhassan, A. L., & Biekpe, N. (2016). Competition and efficiency in the non-life insurance market in South Africa. Journal of Economic Studies, 43(6), 882–909. https://doi.org/10.1108/JES-07-2015-0128
- Allen Berger and Timothy Hannan. (1989). The Price-Concentration Relationship in Banking, The Review of Economics and Statistics,71(2), 291–99.
- Allen, N., & Berger, D. B. H. (1992). ‘Measurement and efficiency issues in commercial banking’. In Z. Griliches (Ed..), Output measurement in the service sectors (pp. 245–25). University of Chicago Press. http://www.nber.org/chapters/c7237%0D
- Almulhim, T., & McMillan, D. (2019). Analysis of Takaful vs. Conventional insurance firms’ efficiency: Two-stage DEA of Saudi Arabia’s insurance market. Cogent Business & Management, 6(1), 1633807. https://doi.org/10.1080/23311975.2019.1633807
- Alshammari, A. A., Alhabshi, S. M. B. S. J., & Saiti, B. (2019). The impact of competition on cost efficiency of insurance and takaful sectors: Evidence from GCC markets based on the Stochastic Frontier Analysis. Research in International Business and Finance, 47, 410–427. https://doi.org/10.1016/j.ribaf.2018.09.003
- Ansah‐Adu, K., Andoh, C., & Abor, J. (2012). Evaluating the cost efficiency of insurance companies in Ghana. The Journal of Risk Finance. The Journal of Risk Finance, 13(January), 61-76.
- Ariff, M. and Can, L. (2008). Cost and Profit Efficiency of Chinese Banks: A non-parametric Analysis.China Economic Review 19(2), 260–273.
- Arrow, K. J. (1962). The economic implications of learning by doing. Review of Economic Studies, 29(3), 155–173. https://doi.org/10.2307/2295952
- Barrors, C., Wanke, P. Banking efficiency in Brazil. Journal of International Financial Markets. Institutions & Money,28, 54–65.
- Barros, C. P., Dumbo, S., & Wanke, P. (2014). Efficiency determinants and capacity issues in Angolan insurance companies. South African Journal of Economics, 82(3), 455–467. https://doi.org/10.1111/saje.12056
- Barros, C. P., Nektarios, M., & Assaf, A. (2010). Efficiency in the Greek insurance industry. European Journal of Operational Research, 205(2), 431–436. https://doi.org/10.1016/j.ejor.2010.01.011
- Barros, C. P., & Wanke, P. (2014). Insurance companies in Mozambique: A two-stage DEA and neural networks on efficiency and capacity slacks. Applied Economics, 46(29), 3591–3600.
- Beck, T., & Webb, I. (2003). Economic, demographic, and institutional determinants of life insurance consumption across countries. The World Bank Economic Review, 17(1), 51–88. https://doi.org/10.1093/wber/lhg011
- Benyoussef, S., Hemrit, W., & Papavassiliou, V. (2019). Measuring the relative efficiency of insurance companies in Saudi Arabia: The case study of Takaful vs cooperative industries. Cogent Economics & Finance, 7(1), 1590818. https://doi.org/10.1080/23322039.2019.1590818
- Berger, A. N., Hunter, W. C., & Timme, S. G. (1993). The efficiency of financial institutions: A review and preview of research past, present and future. Journal of Banking & Finance, 17(2–3), 221–249. https://doi.org/10.1016/0378-4266(93)90030-H
- Berger, A.N., Cummins, J.D., Weiss, M.A. and Zi, H. (2000) ‘Conglomeration versus strategic focus: Evidence from the insurance industry, Journal of Financial Intermediation, 9(4), 323–362.
- Biener, C. and Eling, M. (2011) ‘The performance of microinsurance programs: A data envelopment analysis, Journal of Risk and Insurance, 78(1), 83–115.
- Biener, C., & Eling, M. (2012). Organization and efficiency in the international insurance industry: A cross-frontier analysis. European Journal of Operational Research, 221(2), 454–468. https://doi.org/10.1016/j.ejor.2012.03.037
- Biener, C., Eling, M., & Wirfs, J. H. (2016). The determinants of efficiency and productivity in the Swiss insurance industry. European Journal of Operational Research, 248(2), 703–714. https://doi.org/10.1016/j.ejor.2015.07.055
- Bikker, J. A. (2016). Performance of the life insurance industry under pressure: Efficiency, competition, and consolidation. Risk Management and Insurance Review, 19(1), 73–104. https://doi.org/10.1111/rmir.12059
- Bikker, J. A., & Van Leuvensteijn, M. (2008). Competition and efficiency in the Dutch life insurance industry. Applied Economics, 40(16), 2063–2084. https://doi.org/10.1080/00036840600949298
- Boonyasai, T., Grace, M.F. and Skipper Jr., H.D. (2002). The effect of liberalization and deregulation on life insurer efficiency, working paper no.02–2,Center for Risk Management and Insurance Research, Georgia State University, Atlanta.
- Chiu, Y. H., & Chen, Y. C. (2009). The analysis of Taiwanese bank efficiency: Incorporating both external environment risk and internal risk. Economic Modelling, 13(1), 456–463. https://doi.org/10.1016/j.econmod.2008.09.003
- Choi, B. P., & Weiss, M. A. (2005). An Empirical Investigation of Market Structure, Efficiency, and Performance in Property-Liability Insurance. Journal of Risk and Insurance, 72(4), 635–673. https://doi.org/10.1111/j.1539-6975.2005.00142.x
- Chortareas, G. E., Girardone, C., & Ventouri, A. (2013). Financial freedom and bank efficiency: Evidence from the European Union. Journal of Banking & Finance, 37(4), 1223–1231. https://doi.org/10.1016/j.jbankfin.2012.11.015
- Copeland, M. K., & Cabanda, E. (2018). ‘Efficiency Analysis of the U.S. Publicly Held Insurance Industry’. International Journal of Information Systems in the Service Sector, 10(1), 1–15. https://doi.org/10.4018/IJISSS.2018010101.
- Cummins, J. D., Rubio-Misas, M., & Vencappa, D. (2017). Competition, efficiency and soundness in European life insurance markets. Journal of Financial Stability, 28, 66–78. https://doi.org/10.1016/j.jfs.2016.11.007
- Cummins, J. D., Rubio-Misas, M., & Zi, H. (2004). The effect of organizational structure on efficiency: Evidence from the Spanish insurance industry. Journal of Banking & Finance, 28(12), 3113–3150. https://doi.org/10.1016/j.jbankfin.2004.05.004
- Cummins, J. D., Tennyson, S., & Weiss, M. A. (1999). Consolidation and efficiency in the US life insurance industry. Journal of Banking & Finance, 23(2–4), 325–357. https://doi.org/10.1016/S0378-4266(98)00089-2
- Cummins, J. D., Weiss, M. A. (2000). Analyzing Firm Performance in the Insurance Industry Using Frontier Efficiency and Productivity Methods. In: Dionne G. (eds) Handbook of Insurance. Huebner International Series on Risk, Insurance, and Economic Security, vol 22. Springer, Dordrecht. https://doi.org/10.1007/978-94-010-0642-2_24
- Cummins, J. D., Weiss, M. A., Xie, X., & Zi, H. (2010). Economies of scope in financial services: A DEA efficiency analysis of the US insurance industry. Journal of Banking & Finance, 34(7), 1525–1539. https://doi.org/10.1016/j.jbankfin.2010.02.025
- Cummins, J. D and X, Xie. 2008. Mergers & Acquisitions in the US PropertyLiability Insurance Industry: Productivity and Efficiency Effects, Journal of Banking & Finance, 32, 30–55.
- Cummins, J.D. and Rubio-Misas, M. (2006). Deregulation, consolidation, and efficiency: Evidence from the Spanish insurance industry. Journal of Money, Credit, and Banking, 38(2), 323–355.
- Diacon, S. R., Starkey, K., & O’Brien, C. (2002a). Size and efficiency in European long-term insurance companies: An international comparison. Geneva Papers on Risk and Insurance - Issues and Practice, 27(3), 444–466.
- Dorofti, C., & Jakubik, P. (2015). Insurance sector profitability and the macroeconomic environment.
- Duan, C., Deng, C., Gharaei, A., Wu, J., & Wang, B. (2018). ‘Selective maintenance scheduling under stochastic maintenance quality with multiple maintenance actions’. International Journal of Production Research, 56(23), 7160–7178. https://doi.org/10.1080/00207543.2018.1436789.
- Eling, M., & Jia, R. (2018). Business failure, efficiency, and volatility: Evidence from the European insurance industry. International Review of Financial Analysis, 59(1), 58–76. https://doi.org/10.1016/j.irfa.2018.07.007
- Eling, M., & Jia, R. (2019). ‘Efficiency and profitability in the global insurance industry’. Pacific-Basin Finance Journal, 57(1), 101190. https://doi.org/10.1016/j.pacfin.2019.101190.
- Ennsfellner, Karl C., Lewis, D., & Anderson, Randy I. (2004). Production Efficiency in the Austrian Insurance Industry: A Bayesian Examination, Journal of Risk and Insurance,71(1), 135–159.
- Esho, N., Kirievsky, A., Ward, D., & Zurbruegg, R. (2004). Law and the Determinants of Property-Casualty Insurance. Journal of Risk and Insurance, 71(2), 265–283. https://doi.org/10.1111/j.0022–4367.2004.00089.x
- Fama, E. F., & Jensen, M. C. (1983). Agency problems and residual claims. The Journal of Law & Economics, 26(2), 327–349. https://doi.org/10.1086/467038
- Fecher, F., Kessler, D., Perelman, S., Pestieau, P., 1993. Productive performance of the French insurance industry. Journal of Productivity Analysis, 4, 77–93.
- Fenn, F., Vencappa, D., Diacon, S., Klumpes, P., & O’Brien, C. (2008). Market structure and the efficiency of European insurance companies: A stochastic frontier analysis. Journal of Banking and Finance, 32(1), 86–100. https://doi.org/10.1016/j.jbankfin.2007.09.005
- Fries, S., & Taci, A. (2005). Cost efficiency of banks in transition: Evidence from 289 banks in 15 post-communist countries. Journal of Banking & Finance, 29(1), 55–81. https://doi.org/10.1016/j.jbankfin.2004.06.016
- Fukuyama, H. (1997). ‘Investigating productive efficiency and productivity changes of Japanese life insurance companies’. Pacific-Basin Finance Journal, 5(4), 481–509. https://doi.org/10.1016/S0927-538X(97)00016-4.
- Gaganis, C., Hasan, I., & Pasiouras, F. (2013). Efficiency and stock returns: Evidence from the insurance industry. Journal of Productivity Analysis, 40(3), 429–442. https://doi.org/10.1007/s11123-013-0347-x
- Gardner, L. A., & Grace, M. F. (1993a). X-efficiency in the US life insurance industry. Journal of Banking & Finance, 17(2–3), 497–510. https://doi.org/10.1016/0378-4266(93)90048-I
- Gardner, L. A., & Grace, M. F. (1993b). ‘X-Efficiency in the US life insurance industry’. Journal of Banking & Finance, 17(2–3), 497–510. https://doi.org/10.1016/0378-4266(93)90048-I.
- Gharaei, A., Hoseini Shekarabi, S. A., & Karimi, M. (2020). ‘Modelling And optimal lot-sizing of the replenishments in constrained, multi-product and bi-objective EPQ models with defective products: Generalised Cross Decomposition’. International Journal of Systems Science: Operations & Logistics, 7(3), 262–274. https://doi.org/10.1080/23302674.2019.1574364.
- Gharaei, A., Karimi, M., & Hoseini Shekarabi, S. A. (2019). ‘An integrated multi-product, multi-buyer supply chain under penalty, green, and quality control polices and a vendor managed inventory with consignment stock agreement: The outer approximation with equality relaxation and augmented penalty algorithm’. Applied Mathematical Modelling, 69, 223–254. https://doi.org/10.1016/j.apm.2018.11.035.
- Gharaei, A., Karimi, M., & Hoseini Shekarabi, S. A. (2020). ‘Joint economic lot-sizing in multi-product multi-level integrated supply chains: Generalized benders decomposition’. International Journal of Systems Science: Operations & Logistics, 7(4), 309–325. https://doi.org/10.1080/23302674.2019.1585595.
- Grmanová, E., & Pukala, R. (2018). ‘Efficiency of insurance companies in the Czech Republic and Poland’. Oeconomia Copernicana, 9(1), 71–85. https://doi.org/10.24136/oc.2018.004.
- Halkos, G. E., & Tzeremes, N. G. (2007). Productivity efficiency and firm size: An empirical analysis of foreign owned companies. International Business Review, 16(6), 713–731. https://doi.org/10.1016/j.ibusrev.2007.06.002
- Hannah, E., & Yeung, V. (1998a, October). Report of the task force on the future of the Canadian financial services sector. Financial Regulation Report, 1998.
- Hannah, E., & Yeung, V. (1998b) Report of the task force on the future of the Canadian financial services sector, Financial Regulation Report, October.
- Hao, J. C., & Chou, L. Y. (2005). The estimation of efficiency for life insurance industry: The case in Taiwan. Journal of Asian Economics, 16(5), 847–860. https://doi.org/10.1016/j.asieco.2005.08.004
- Hasan, M. B., Islam, S. N., & Wahid, A. N. (2018). The effect of macroeconomic variables on the performance of non-life insurance companies in Bangladesh. Indian Economic Review, 53(12), 369–383. https://doi.org/10.1007/s41775-019-00037-6
- Hay, Donald A. & Liu, Guy S. (1997). THE EFFICIENCY OF FIRMS: WHAT DIFFERENCE DOES COMPETITION MAKE? The economic journal, 107(442) May 1997, 597–617
- Hicks, J. (1935). Annual Survey of Economic Theory: The Theory of Monopoly, Econometrica, 3(1), 1–20
- Hirbod, A & Mostafa, P & A. Badamchizadeh, 2019. Sound Deposit Insurance Pricing Using a Machine Learning Approach, „ Risks, MDPI, Open Access Journal, 7(2), 1–18
- Hoseini Shekarabi, S. A., Gharaei, A., & Karimi, M. (2019). ‘Modelling and optimal lot-sizing of integrated multi-level multi-wholesaler supply chains under the shortage and limited warehouse space: Generalised outer approximation’. International Journal of Systems Science: Operations & Logistics, 6(3), 237–257. https://doi.org/10.1080/23302674.2018.1435835.
- Huang, W., & Eling, M. (2013). An efficiency comparison of the non-life insurance industry in the BRIC countries. European Journal of Operational Research, 226(3), 577–591. https://doi.org/10.1016/j.ejor.2012.11.008
- Ilyas, A. M., & Rajasekaran, S. (2019). ‘An empirical investigation of efficiency and productivity in the Indian non-life insurance market’. Benchmarking: An International Journal, 26(7), 2343–2371. https://doi.org/10.1108/BIJ-01-2019-0039.
- Ismail, N., Ishak, I., Manaf, N. A., & Husin, M. M. (2018). Macroeconomic Factors Affecting Performance of Insurance Companies in Malaysia. Academy of Accounting and Financial Studies Journal, 22, 1–5. https://search.proquest.com/openview/1c1c6f1359e051bb384216bcaea9819e/1?pq-origsite=gscholar&cbl=29414
- Jaloudi, M. M. (2019). The efficiency of Jordan insurance companies and its determinants using DEA, slacks, and logit models. Journal of Asian Business and Economic Studies, 26(1), 153–166. https://doi.org/10.1108/JABES-10-2018-0072
- Jovanovic, B. (1982). Selection and the Evolution of Industry. Econometrica: Journal of the Econometric Society, 50(3), 649–670. https://doi.org/10.2307/1912606
- K, Al-Amri. 2015. Takaful insurance efficiency in the GCC countries. Humanomics: The International Journal of Systems and Ethics, Emerald Group Publishing,31(3), 344–353..
- Kader, H. A., Adams, M., & Hardwick, P. (2010). The Cost Efficiency of Takaful Insurance Companies. The Geneva Papers on Risk and Insurance - Issues and Practice, 35(1), 161–181. https://doi.org/10.1057/gpp.2009.33
- Kao, C., & Hwang, S. N. (2008). Efficiency decomposition in two-stage data envelopment analysis: An application to non-life insurance companies in Taiwan. European Journal of Operational Research, 185(1), 418–429. https://doi.org/10.1016/j.ejor.2006.11.041
- Karbhari, Y., Muye, I., Hassan, A. F. S., & Elnahass, M. (2018). ‘Governance mechanisms and efficiency: Evidence from an alternative insurance (Takaful) market’. Journal of International Financial Markets, Institutions and Money, 56, 71–92. https://doi.org/10.1016/j.intfin.2018.02.017.
- Kasman, A., & Turgutlu, E. (2008). Competitive conditions in the Turkish non-life insurance industry. Review of Middle East Economics and Finance, 4(1), 81–96. https://doi.org/10.2202/1475-3693.1071
- Kasman, A., & Turgutlu, E. (2009). Cost efficiency and scale economies in the Turkish insurance industry. Applied Economics, 41(24), 3151–3159. https://doi.org/10.1080/00036840701367663
- Kumar, S., & Gulati, R. (2008). An examination of technical, pure technical, and scale efficiencies in Indian public sector banks using data envelopment analysis. Eurasian Journal of Business and Economics, 1(2), 33–69.
- Lai, G. C., and J. P. Lee. 2011. Organizational Structure, Corporate Governance and Risk Taking in the U.S. Property/Casualty Insurance Industry, Working paper, Washington State University
- Lee, H. S., Cheng, F. F., Har, W. M., Md Nassir, A., & Ab Razak, N. H. (2019). ‘Efficiency, firm-specific and corporate governance factors of the Takaful insurance’. International Journal of Islamic and Middle Eastern Finance and Management, 12(3), 368–387. https://doi.org/10.1108/IMEFM-06-2018-0187.
- Lin, K. L., Doan, A. T., & Doong, S. C. (2016). Changes in ownership structure and bank efficiency in Asian developing countries: The role of financial freedom. International Review of Economics & Finance, 43, 19–34. https://doi.org/10.1016/j.iref.2015.10.029
- Luhnen, M. (2009). Determinants of efficiency and productivity in German property-liability insurance: Evidence for 1995–2006. The Geneva Papers on Risk and Insurance - Issues and Practice, 34(3), 483–505. https://doi.org/10.1057/gpp.2009.10
- Lundvall, K., & Battese, G. E. (2000). Firm size, age and efficiency: Evidence from Kenyan manufacturing firms. The Journal of Development Studies, 36(3), 146–163. https://doi.org/10.1080/00220380008422632
- Maghyereh, A. I., & Awartani, B. (2014). The effect of market structure, regulation, and risk on banks efficiency. Journal of Economic Studies.
- Mandal, S., & Ghosh Dastidar, S. (2014). ‘A DEA-investigation of efficiency of the Indian general insurance during recession’. Journal of Advances in Management Research, 11(1), 115–136. https://doi.org/10.1108/JAMR-07-2012-0030.
- Mensi, S., & Zouari, A. (2010). Efficient structure versus market power: Theories and empirical evidence. International Journal of Economics and Finance, 2(4), 151–166. https://doi.org/10.5539/ijef.v2n4p151
- Naushad, M., Faridi, M. R., & Faisal, S. (2020). ‘Measuring the managerial efficiency of insurance companies in Saudi Arabia: A data envelopment analysis approach’. The Journal of Asian Finance, Economics and Business, 7(6), 297–304. https://doi.org/10.13106/jafeb.2020.vol7.no6.297.
- Nguyen, T. N., & Stewart, C. (2013). Concentration and efficiency in the Vietnamese banking system between 1999 and 2009. Journal of Financial Regulation and Compliance. https://doi.org/10.1108/JFRC-10-2012-0041
- Noulas, A.G., Hatzigayios, T., Lazaridis, J. and Lyroudi, K. (2001). Non-parametric production frontier approach to the study of efficiency of non-life insurance companies in Greece. Journal of Financial Management and Analysis,14(1), 19–26.
- Nourani, M., Devadason, E. S., & Chandran, V. (2018). ‘Measuring technical efficiency of insurance companies using dynamic network dea: An intermediation approach’. Technological and Economic Development of Economy, 24(5), 1909–1940. https://doi.org/10.3846/20294913.2017.1303649.
- Park, H., Borde, S. F., & Choi, Y. (2002). Determinants of insurance pervasiveness: A cross-national analysis. International Business Review, 11(1), 79–96. https://doi.org/10.1016/S0969-5931(01)00048-8
- Pasiouras, F., & Gaganis, C. (2013). Regulations and soundness of insurance firms: International evidence. Journal of Business Research, 66(5), 632–642. https://doi.org/10.1016/j.jbusres.2012.09.023
- Rao, A., Kashani, H., & Marie, A. (2010). Analysis of managerial efficiency in insurance sector in the UAE: An emerging economy. International Journal of Managerial Finance, 6(4), 329–343. https://doi.org/10.1108/17439131011074477
- Reyna, A. M., & Fuentes, H. J. (2018). ‘A cost efficiency analysis of the insurance industry in Mexico’. Journal of Productivity Analysis, 49(1), 49–64. https://doi.org/10.1007/s11123-017-0521-7.
- Shieh, H. S., Hu, J. L., & Ang, Y. Z. (2020). The efficiency of Life Insurance Companies: An Empirical Study in Mainland China and Taiwan. SAGE Open, 10(1), 2158244020902060. https://doi.org/10.1177/2158244020902060
- Shujie, Y. A. O., Zhongwei, H. A. N., & Genfu, F. E. N. G. (2007). On technical efficiency of China’s insurance industry after WTO accession. China Economic Review, 18(1), 66–86. https://doi.org/10.1016/j.chieco.2006.10.005
- Stigler, G. (1964). Public Regulation of the Securities Markets. The Journal of Business,37(2), 117–142.
- Tone, K. and Sahoo, B.K. (2005). Evaluating cost efficiency and returns to scale in the life insurance corporation of India using data envelopment analysis. Socio-Economic Planning Sciences, 39(4), 261–285.
- Trinh, T., Sgro, P., & Nguyen, X. (2015). Global financial crisis, economic freedom, and the demand for non-life insurance: An empirical investigation. Working Paper). Department of Economics, Faculty of Business and Law, Deakin University. https://www.rse.anu.edu.au/media/772517/Trinh-Tam.pdf
- Venceppa, D., Fenn, P. & Diacon, S. (2003). Productivity Growth in the European Insurance Industry: Evidence from Life and Non-Life Companies. International Journal of the Economics of Business,20(2).
- Vickie, L. Bajtelsmit & R, Bouzouita. 1998. Profit and Concentration in Commercial Automobile Insurance Losses. Journal of Insurance Issues. Western Risk and Insurance Association,21(2), 172–182.
- Wanke, P., & Barros, C. P. (2016). Efficiency drivers in Brazilian insurance: A two-stage DEA meta frontier-data mining approach. Economic Modelling, 53, 8–22. https://doi.org/10.1016/j.econmod.2015.11.005
- Weiss, M.A. (1991). Efficiency in the property-liability insurance industry. Journal of Risk and Insurance,58(3), 452–479.
- Yang, Z. (2006). ‘A two-stage DEA model to evaluate the overall performance of Canadian life and health insurance companies’. Mathematical and Computer Modelling, 43(7–8), 910–919. https://doi.org/10.1016/j.mcm.2005.12.011.
- Zijiang., Y. (2006). A two-stage dea model to evaluate the overall performance of Canadian life and health insurance companies.”. Mathematical and Computer Modelling, 43(7–8), 910–919. https://doi.org/10.1016/j.mcm.2005.12.011.