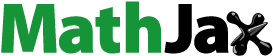
Abstract
In this study, we analyze the effect of knowledge spillover on productivity in the Indonesian manufacturing industry from 2010 to 2014 using inter-sectoral linkages and inter-regional linkages. For the first time in the literature, we apply an input-output table and geographic distance between regions as the weight matrix in spatial econometric estimation to measure the productivity spillover. We find that: (1) productivity spillover from transactions of intermediate goods in vertical linkage (customer-supplier) is dominated by inter-industry downstream and intra-industry upstream; (2) the adoption of foreign technology by domestic firms through imported materials is more vital than foreign direct investment; (3) productivity spillover created from capital-intensive industries is higher than that from labor-intensive industries; (4) in productivity spillover flows through inter-regional spillover and intra-regional spillover, the latter creates higher productivity spillover than the former. This implies that the shorter the geographic distance, the narrower the technology gap; (5) investments in human capital and physical capital are a prerequisite for absorbing technology and thus essential absorptive capacity factors for firms/industries/regions as they narrow down the technology gap between developing and advanced firms/industries/regions.
PUBLIC INTEREST STATEMENT
Industrial product developments such as computers, smartphones, automotive, etc., are changing rapidly. Their production process is very complex, involving various suppliers with specific skills. However, this provides an opportunity for the suppliers to absorb knowledge and adopt technology from their upstream and downstream partners by becoming part of the global supply chain.
Domestic industries can benefit from being part of the global supply chain network by learning from other advanced firms/industries. However, to have the capability to effectively absorb the technology and narrow their technology gap with advanced firms, local suppliers should have certain minimum capacities (e.g., minimum set of skills). The local suppliers’ ability to catch up is key to their development. Another strategy they can employ is to form communities within the local region. In these communities, local industries can increase their efficiency by sharing resources (capital, machinery, workers, etc.).
1. INTRODUCTION
In this study, we analyze the effect of knowledge spillover on productivity in the Indonesian manufacturing sector from 2010–2014. This is an important topic because the rising complexity of industrial goods promotes linkages between industries. In turn, these linkages are expected to improve productivity through knowledge adoption and technology diffusion, known as knowledge spillover—a form of positive and uncompensated externality. However, the knowledge spillovers discussed in this study are little related to R&D spillover activity. Instead, they are closely related to the knowledge spillovers resulting from industrial linkages based on vertical activities (customer-supplier) and agglomeration (specialization and concentration). Indeed, our focus is on how domestic/local industries can optimally absorb the knowledge (both tacit and codified), adapt the technology, and incorporate them into their production methods or processes.
Regarding spillover resulting from the linkage between sectors (inter-sectoral spillover), Arrow (Citation1962) proposed a theory that: “technological knowledge possessed by one industry can spread to other industries and increase the latter’s productivity through inter-industry trading of intermediate goods.” Subsequent scholars have adopted this theory. For example, Romer, (1986); Cohen and Levinthal (Citation1990); Grossman and Helpman (Citation1991), who studied the transmission channels of knowledge spillover and their impact on industrial productivity. Another strand of the literature pioneered by Marshall (Citation1920), Arrow (Citation1962), Jacobs (Citation1969), Romer (Citation1990), Krugman (Citation1991), and Porter (Citation1996) explains why industries within regions find internalization knowledge spillover useful in the concept of agglomeration and underline the importance of resource-based regions. An example of the Marshall Arrow Romer (MAR) spillover is the concentration of semiconductor suppliers and similar technologies in Silicon Valley, called intra-regional spillover (Audretsch & Feldman, Citation1996). This spillover serves as the global center for the high technology industry and innovation. Meanwhile, unlike MAR and Porter, Jacobs argues that the majority of significant knowledge transfers occur outside the resource-based region (inter-regional spillover).
This study is consistent with Sena (Citation2004), who explains the channels of transmission through which technological knowledge can spillover: that is (1) inter-industry and intra-industry linkages; (2) inter-sectoral based vertical linkages or customer-supplier relationships (forward linkage/downstream and backward linkage/upstream); (3) agglomeration-based geographical proximity.
Based on these channels, we investigate the impact of knowledge spillover on productivity in the Indonesian manufacturing industry through inter-sectoral linkages and inter-regional linkages. We focus on how domestic/local industries can optimally absorb technology. However, to absorb the technology successfully, Cohen and Levinthal (Citation1990) suggest that firms/industries/regions should have a minimum or sufficient absorption capacity, which refers to a technological capability to absorb external knowledge. This means that the recipients of these spillovers (inter-sectoral and inter-regional spillovers) are limited to only a few firms/industries/regions.
The empirical strategy of spillovers used in this study is consistent with that of Wolff and Nadiri (Citation1993), Bartelsman et al. (Citation1994), Abreu et al. (Citation2004), Caragliu et al. (Citation2012), Peng and Hong (Citation2013), and Stiller (Citation2018). In the inter-sectoral approach, we use the input-output linkage (backward and forward) based on the I-O table and apply inter-sectoral Durbin panel estimation to analyze the productivity spillover in linked sectors (inter-sectoral linkage). In the inter-regional linkage, we create an inverse distance matrix based on the geographic distance between regions (centroid point to point) and apply spatial Durbin panel estimation to analyze the productivity spillover between neighboring regions.
Based on the foregoing issues, we propose three hypotheses: (1) if knowledge spillover flows based on inter-industry and intra-industry linkages in a vertical linkage (downstream and upstream) between suppliers and customers, which of the two linkages is more dominant in the case of downstream or upstream or both linkage(s)? (2) if industrial-agglomeration-based geographic distance creates a productivity spillover, is this inter-regional spillover? What factors affect the externalities from agglomeration in inter-regional and intra-regional spillover(s)? (3) if there is a positive relationship between knowledge spillovers and absorptive capacity, what is the nature of this relationship? What factors contribute positively to its absorptive capacity?
Suffice it to say, we clarify the transmission of knowledge spillover in the Indonesian manufacturing industry by using productivity spillover through inter-sectoral spillover (which originates from the externality of the input-output/vertical linkage) and inter-regional spillover (which originates from agglomeration based on geographical proximity). For the first time in the literature, we apply an input-output table and geographic distance between regions as the weight matrix in spatial econometric estimation to measure productivity spillover. We find that productivity spillover in vertical linkages (customer-supplier) is dominated by inter-industry downstream and intra-industry upstream. Productivity spillover also flows through inter-regional spillover (between) and intra-regional spillover (within). The latter creates stronger productivity spillover than the former, which implies that geographic distance proximity narrows down the technology gap. Another important finding is that investments in human capital and physical capital are a prerequisite for absorbing technology and thus important absorptive capacity factors for firms/industries/regions as they also narrow the technology gap between developing and advanced firms/industries/regions. The rest of the paper is outlined as follows: Section 2-Theoretical Foundation and Literature Review. Section 3-Overview of the Indonesian Manufacturing Industry. Section 4-Data and Methodology. Section 5-Empirical Results and Discussion. Section 6-Conclusions and Policy Implications.
2. THEORETICAL FOUNDATION AND LITERATURE REVIEW
2.1. The concept of knowledge spillover in manufacturing industry
The knowledge spillover theory is derived from a firm-level concept where one company benefits from the private information of another company (companies) for free. There are many cross-referencing benefits for firms that imitate the successes of others and those who learn from the efforts of others. Jaffe (Citation1998) argued that the concept of the public good of knowledge is closely tied to spillovers, that the benefits of the new knowledge are higher than those of its creator holding on to it. The benefits are so diffuse that they will not be reduced by each individual. At the industry level, the industry collects external knowledge through externalities from vertical linkages (supplier-customer), leading to positive or negative impacts on either customers or suppliers in the market (Wolff & Nadiri, Citation1993; Lopez and Sudekum, Citation2007). At the regional level, the major externalities are caused by geographic factors from interactions among people who work within and between regions (Abreu et al., Citation2004; Stiller, Citation2018).
The literature related to technological knowledge spillover should be considered an important determinant of productivity, as pioneered by Griliches (Citation1979). He examined the relationship between information processing activities and knowledge in his knowledge production function. He linked the output of new knowledge to two types of input: investment in discovering new knowledge (e.g., spending on R&D) and flow of ideas from the existing stock of knowledge. He also mentioned that external input for creating innovation is also essential to consider being the source of externalities. This argument implies that the closer one is to the source of externalities, the easier it is to integrate and diffuse innovations from others. Recently, industrial spillover literature has been concerned with how the technology of a firm and its productivity level rely on the same industry and related industries. Scholars have found that knowledge spillovers flow through intra-industry and inter-industry spillovers (Bernstein & Nadiri, Citation1989; Los & Verspagen, Citation2000). In the spatial spillover literature, spillovers that flow within and between regions are estimated via geographic borders (Abreu et al., Citation2004; Orlando, Citation2004; Stiller, Citation2018).
A more general approach allows technology spillovers to flow across regions and industries. It is assumed that the benefit a firm can obtain from the technological efforts of other firms is inversely proportional to their distance from the externality-producing firm (Piga & Medda, Citation2007). They presumed that the spillover strength of the firm/industry/region is
where S is the spillover effect, subscript j is the origin of the spillover (e.g., a firm, an industry, or a region), subscript i is the firm/industry/region receiving the spillover, R is a measure of technological capital, and Wij is an inter-industry weight matrix and spatial weight matrix representing the distance (economic distance and geographic distance) between i and j.
The construction of an inter-industry weight matrix was pioneered by Brown and Conrad (Citation1967), followed by other scholars (Bartelsman et al., Citation1994; Peng & Hong, Citation2013; Terleckyj, Citation1974; Wolff & Nadiri, Citation1993), who constructed the inter-industry weight matrix based on input-output tables. These authors made their weights equal to the input/output coefficient, calculated by dividing the cell values by the corresponding column/row sum. These methods are used to distinguish between the potential spillover from downstream (demand-driven spillovers) and the potential spillover from upstream (supply-driven spillovers) using the inter-industry matrix. This matrix indicates that the more industry i buys or sells goods to industry j, the more it can benefit from technological spillover originating from industry j, and vice versa.
Another approach is to construct a spatial weight matrix. Abreu et al. (Citation2004) and Stiller (Citation2018) constructed an inverse distance matrix between regions using coordinates in latitude and longitude (centroid point to point). This matrix indicates that the closer the distance between regions, the greater the spillover of knowledge. They also argue that geographical distance leads to a narrow technology gap.
2.2. Absorptive capacity
Many studies have focused on understanding the key characteristics of firms, regions, and countries that make it easier to understand and decode information coming from outside in an economically efficient manner since the publication of Cohen and Levinthal (Citation1990) on the firm’s absorptive capacity. The concept of absorptive capacity, which originated in the context of a firm’s theory, can now be applied to more complex institutions, such as regions and countries. The notion that a proper knowledge base is required to comprehend more and better information.
2.3. Inter-sectoral linkage
A firm or industry must have a certain level of absorptive capacity to recognize, access, adopt, and benefit from these external sources of knowledge, whether through market-internalized transactions or externalities. Absorptive capacity is defined as the capability to acquire new information (Cohen & Levinthal, Citation1990). It has been suggested that the ability to catch up is influenced by the level of difference between the leader and the follower in foreign direct investment/FDI (Fagerberg, Citation1995; Findlay, Citation1978). He argued that the technology or efficiency gap is the distinction between two firms (or industries). Moreover, Sjoholm (1999) argued that FDI benefits local/domestic industries, but the effect differs between industries. He found that sectors with a high degree of competition will have FDI spillovers. Moreover, he concluded that the larger the technology gap between domestic and foreign companies, the larger the spillover.
However, large technological gaps may pose a barrier to technology transfer (Cohen & Levinthal, Citation1990). These arguments are supported by Girma et al. (Citation2005). They argued that companies with a productivity gap of 10% or less than the market frontier level are more likely to benefit from FDI spillovers. Therefore, in this case, the concept of absorptive capacity is important to narrow the technology gap between developing and advanced firms.
Foreign firm characteristics may be a key factor in the extent to which externalities from foreign companies exist. Scholars find evidence of productivity spillovers through the backward linkage between multinational companies (MNCs) and domestic suppliers (Clare, Citation1996) and partially foreign-investment customers in Lithuania (Javorcik, Citation2004). Moreover, she finds no evidence of intra-sector spillover or spillover from foreign-invested input suppliers and domestic input. She explains that, compared to fully foreign-invested companies, foreign owners are more likely to be better linked to the local economy through local sourcing.
Kokko and Blomstrom (Citation1996) also argued that firm characteristics reduce performance gaps between foreign companies and local/domestic firms. This argument is supported by Marin and Bell (Citation2006), Giroud (Citation2012), and Elkomy et al. (Citation2020). They found that local/domestic firms’ investment in capital-embodied technology and skill training will make it easy for them to absorb technology from foreign companies. Moreover, they argued that, for policies to attract FDI to generate technology externalities, foreign-invested firms’ knowledge-creation activities and technological capabilities are more important than just technology transfer externalities.
2.4. Inter-regional linkage
The inter-regional linkage mostly focuses on capital investment’s role in enhancing regions’ growth capabilities. A region can recognize and apply the appropriate external knowledge. However, some evidence shows that regions that produce codified knowledge cannot fully absorb, decode, and exploit it to its full potential. Therefore, this raises some questions. What caused this discrepancy? What drives this outcome? Why are the factors alone insufficient to explain regional efficiency growth? We try to answer these questions by applying spatial knowledge spillovers and regions’ absorptive capacities. Caragliu et al. (Citation2012) linked productivity with technical knowledge accumulation to answer. He argued that productivity is better proxied by total factor productivity (TFP) to measure aggregate technology. The TFP’s rate of change over time depends on human capital (Moretti, Citation2004) and physical capital (Baffes & Shah, Citation1998). These authors refer to the literature on direct and indirect effects as the foundation for the Outward Knowledge Spillovers (OKS) measure. This study constructs this OKS using spatial econometric estimation (direct and indirect) to observe the relationship between absorptive capacity and TFP through the explanatory variables (human capital and physical capital).
2.5. Trade growth approach
According to endogenous growth theory, country growth rates are linked to international trade links and embodied and disembodied knowledge spillovers (Grossman & Helpman, Citation1991). In this case, a country’s development relies heavily on its knowledge capital, as determined by national innovation and international technology diffusion rates. The degree of international openness can affect domestic innovation, the quantity of transferred technology, and the rate at which more advanced countries’ technologies are adopted (Redding & Proudman, Citation1998). Scholars find the productivity growth of exporting firms when examining the role of exports at the firm level (Aw & Hwang, Citation1995). They find spillover effects on exporting firms. These externalities are referred to as productivity spillovers by exports. Another branch of empirical research, initiated by Coe and Helpman (Citation1995), analyzed the knowledge capital incorporated in imported goods. Imports can result in a spillover effect when domestic firms learn about the innovative content of imported goods. Coe et al. (Citation1997) estimated a large positive effect of import-weighted foreign research and development investment (R&D).
2.6. The role of spatial spillovers
That knowledge spills over was pointed out by Marshall (Citation1920) and, more recently, by Arrow (Citation1962) and Romer (1986). Nevertheless, the geographical range of such spillovers is highly disputed. Krugman (Citation1991) and others do not question the importance of the externalities of knowledge in explaining the geographical concentration of economic activity. Indeed, they contend that such externalities are so important and powerful that geographic boundaries should not limit them. This reasoning line contends that the issue is not whether knowledge spillover but whether it should stop spilling over simply because it crosses a geographic border, such as a city limit, state border, or national boundary. Geographical proximity is essential for radical innovation (Caniëls & Romijn, Citation2003). The authors recommend stronger interactions between firms and research labs, and universities will yield more original and/or complex innovations. Greenstone et al. (Citation2010) argued that agglomeration spillovers are larger between plants that share labor pools and similar technology. The above research studies have shown the importance of spatial proximity in cluster networks to create local knowledge spillover through agglomeration, which positively affects industrial productivity.
3. THE OVERVIEW OF THE INDONESIAN MANUFACTURING INDUSTRY
Indonesia experienced industrialization by increasing manufacturing in the economy and diversifying the manufacturing industry. However, industrialization abruptly stopped during the Asian Financial Crisis of 1997–1998, and it never returned to its former levels. The manufacturing sector’s contribution to gross domestic product has been declining. Workers have moved away from agriculture towards non-tradable sectors, such as sales, restaurant work, retail work, and construction. However, the manufacturing industry in Indonesia still drives economic growth. The contribution of manufacturing to the economy’s size is approximately 20 percent of the economy’s total.
Moreover, linkage and spillover effects are stronger in manufacturing than in other sectors of the economy. Linkage effects refer to inter-sectoral purchases and sales, while spillover effects refer to knowledge flows between sectors/regions. Below are some characteristics that the manufacturing industry possesses from sectoral and spatial dimensions.
3.1. Employment
Manufacturing adds relatively little to overall employment. The aggregated service sector contributes the most. The two main sub-sectors of manufacturing that create employment (labor-intensive sectors) are textile and food.
3.2. Value added
Value-added manufacturing sectors tend to be dominated by a small number of low-tech manufacturing subsectors. The largest contribution of value-added is in the food and petroleum sectors. While larger than the employment share, the share of value-added accounted for by manufacturing is lower than in other Asian economies like Japan, the Republic of Korea, Taipei, and China based on the World Input-Output Database (WIOD) released in 2016. In the value-added case, Indonesia has the highest share in the primary sectors, reflecting the importance of Indonesia’s mining sector.
3.3. Labor productivity
According to WIOD 2016, Indonesia performs poorly in terms of labor productivity compared to other Asian economies such as Japan, Korea, Taiwan, and China. Indonesia’s labor productivity growth rate is also low. From 2000 to 2014, the growth rate of labor productivity in the manufacturing sector was negative. Based on this issue, we measure manufacturing characteristics by sub-sectors in Indonesia (). The value-added is deflated based on the 2010 base year by the Indonesian Whole Price Index.
Table 1. Characteristics of manufacturing by sub-sectors in Indonesia in the year 2014
Based on , the top five sub-sectors of the Indonesian manufacturing industry that generate high labor productivity are (1) motor vehicles (ISIC 29), (2) chemical (ISIC 20), (3) basic metal (ISIC 24), (4) other transport equipment (ISIC 30), and (5) electrical machinery (ISIC 27). Next, we review Indonesian manufacturing from the perspective of spatial/regional dimensions or industry agglomeration.
3.4. Agglomeration
Agglomeration is a key process in facilitating modern manufacturing activities. The manufacturing agglomeration process is present in Indonesia. While manufacturing activities across Indonesia often started in certain major cities such as Jakarta, Bandung, and Surabaya. The process seems to reinforce itself, as new manufacturers tend to base their operations in areas where manufacturing activities already exist. The process also appears to be associated with productivity gains and increased product variety. Market forces and associated externalities appear to be the main drivers of these forces. Manufacturing agglomeration or clustering of selected industries continues to occur today, such as garments in the Bandung area, machinery and heavy industries in the Greater Jakarta and Surabaya areas, rattan in Cirebon and Jepara, leather and footwear in Sidoarjo, and Ceramics in Plered (West Java). Firm co-location can increase productivity spillover from foreign direct investment and exports. Spillovers can occur through various transmission channels, including changing market forces (such as increased competition and demonstration), labor turnover, and supply chains. Such channels are more likely to work when foreign and domestic firms are located close to each other. Empirical evidence for Indonesian manufacturing over the period 1990–2005 suggests that a higher share of exporters in a province (export spillovers), where multinational firms have a significantly positive effect on firm-level export propensity. The characteristics of manufacturing by region (island) are listed in .
Table 2. Characteristics of manufacturing by islands in Indonesia in the year 2014
Based on , we can see a deep disparity inter-region (inter-island), with Java as the center of the Indonesian economy, whereas other islands contribute less to economic growth. Approximately 70% of the Indonesian manufacturing industry’s value-added is located on Java Island. However, although agglomeration in the manufacturing sector is crucial in Indonesia, only a few studies have been conducted on the issue. Henderson et al. (Citation1995) used data from manufacturing industries to search for production externalities in cities between 1970 and 1987. From 1990 to 2003, Kuncoro and Wahyuni (Citation2009) studied industry agglomeration on Java Island, illustrating how agglomeration contributes to increased manufacturing productivity via localization.
Moreover, Raharja and Kuncoro (Citation2012) suggest that firms in agglomeration areas are more productive than those outside. Core agglomeration in Indonesia is still attractive to new manufacturing entrants. The core region performs better in terms of technology and worker availability, infrastructure, transportation, and access to finance. They argued that agglomeration, based on survey analysis of Indonesian manufacturing firms in 2009, confirms that the share of foreign manufacturers in the core region was 3.1 percent compared with only 0.9 percent in non-core regions.Footnote1 The percentage of direct exporters in core regions was 4.1%, compared with 2.1 percent in non-core regions. Since foreign firms and exporters are generally more productive, their presence in agglomerations increases the possibility of productivity spillovers within the same industry/intra-industry in upstream or downstream industries (vertical spillovers). Therefore, in Indonesia, agglomerations tend to be characterized by a higher share of foreign investors and exporters.
3.5. The Indonesian industrial revolution 4.0 (4IR)
In 2018, the Indonesian government initiated a new road map, namely the Indonesian Industrial Revolution 4.0 (Making Indonesia 4.0), which has the top five priority sectors of the industry to be developed. There are food and beverage, chemical, textile and apparel, electronics, and automotive industries. One of the goals of the Indonesian 4IR is to achieve a double ratio between productivity and cost. Therefore, the implementation of technology 4IR enhances labor productivity.
4. DATA AND METHODOLOGY
The Indonesian Central Statistics Agency conducts annual surveys on Indonesian medium-large industrial manufacturing enterprises. Our data are mostly obtained from these surveys for the years 2010 to 2014. There is a 5-digit level numeric code for the manufacturing sub-sector classification (ISIC). Before estimating, we merge the data into a panel model (five years), and a data cleaning procedure is carried out to eliminate zero values for each period. We deflate value-added and capital based on 2010 with the Indonesia Whole Price Index.
This study’s methodology is derived from Romer’s (Citation1990) endogenous growth theory, which extends the Cobb-Douglas production function with human capital input. This theory explains that productivity growth is determined by technological knowledge that depends on productivity parameters, namely the share of human capital allocated to research and access to existing ideas. This study assumes that technological knowledge, which is embodied in intermediate input, flows through intra-industry and inter-industry linkages (Bernstein & Nadiri, Citation1989; Los & Verspagen, Citation2000) and can be divided into upstream and downstream. Concerning inter-regional linkages, technological knowledge is embodied in geographically closed regions that develop similar industry structures and increase technological proximity (Abreu et al., Citation2004; Stiller, Citation2018).
We used an inter-industry weight matrix from the input-output (I-O) table on the inter-sectoral model to test the intensity of the knowledge spillover transmission mechanism. This model captures the innovative activity performed by a firm or industry that may affect both customers’ technology (forward spillover/upstream spillover) and suppliers (backward spillover/downstream spillover). In the inter-regional model, we capture knowledge spillovers between regions as spatial dependence using a spatial weight matrix that contains the inverse of the geographic centroid distance between the regions.
4.1. Productivity measurement
We use two productivity indicators: labor productivity and TFP. The formula used are listed in .
Table 3. Indicators of Productivity
Labor productivity is defined as the ratio between output and input(s). It indicates how efficiently labor is used in production (Schreyer, Citation2001). TFP is the ratio of output and combined capital and labor input. These two tools (TFP and labor productivity) are important for productivity decomposition. In this study, TFP is computed using the Levinsohn Petrin (LevPet) formula, as shown in . The change in TFP indicates whether productivity is driven by investment in traditional capital (rise in capital intensity) or by investment in intangible capital (spillover, innovation, organizational change, R&D). Next, Schreyer & Pilat, (Citation2001) summarized the productivity measures as shown in .
Table 4. Productivity measures
4.2. Weight matrix measurement
4.2.1. Inter-industry matrix (Wij)
An inter-industry matrix is a technical coefficient matrix with element ij, which shows the value of products from industry i used as intermediate materials in industry j (forward linkage) and the value of products from industry j used as input materials in industry i (backward linkage).
4.2.1.1. Backward linkage
The jith element divided by the sum of the ith column of the technical coefficient matrix gives the weight applied to the activity of industry j as a supplier to industry i:
4.2.1.2. Forward linkage
The ijth element of the matrix, divided by the sum of the ith row of the technical coefficient matrix, gives the weight applied to the activity of industry j that purchases products from industry i.
4.2.2. Geographic distance matrix (Wsm)
Wsm is defined as
Measures R x R, where R is the number of district/regencies in Indonesia
Spatial data in the form of coordinates for each district (x, y) is taken from Indonesia Spatial Agency
The formula distance between regions based on centroid point to point.
4.3. Inter-sectoral model
The purpose of this model is to provide an answer to the first hypothesis on the existence of inter-sectoral spillover. The model is derived from the Cobb Douglas production function.
A is a positive constant, Q/L is productivity, K is physical capital, and L is the number of workers.
We modify the model above to construct a new panel model and inter-sectoral Durbin panel model to measure the effect of inter-sectoral linkages on labor productivity. These two models are used to answer the hypothesis on the existence of productivity spillovers on vertical linkages or inter-sectoral spillovers. First, the panel model is used to test the dominant effect of inter-industry linkages on productivity (both downstream and upstream). Second, the inter-sectoral Durbin panel model is used to determine the dominant effect of intra-industry linkages on productivity (both downstream and upstream).
4.3.1. Panel model
The model in Equationequation (6)(6)
(6) is a panel model at the firm level. It is used to answer the first hypothesis on the dominant effect of inter-industry linkages (both downstream and upstream) on productivity. where A is constant; Q/L is firm labor productivity; K is physical capital, and L is the number of workers; Skill is the worker’s skill obtained from the ratio of workers in the non-production floor and total labor, Age is the firm’s age, and Import is the ratio of imported material to total material. Foreign is the portion of the firm’s share capital owned by foreign investors; Dummyinter is a dummy variable that equals zero if the pairs of industries are in the same sector (or intra-industry linkage) and equals one if the pairs of industries are in different sectors (or inter-industry linkages); W is a weighting multiplier based on pairing firm i with firm j either by forward or backward linkage coefficients.
We precisely match one on one for pairing firm i with firm j using the following treatments:
a) Measure the forward and backward linkage coefficients from the input-output table.
b) Select each sector pairs based on the highest forward and backward values.
c) Select each firm pair based on the sector pairs above.
We use an inter-industry matrix derived from the I-O table in 2010 as the weight matrix.
4.3.2. Inter-sectoral Durbin panel model
The model in Equationequation (7)(7)
(7) is an inter-sectoral Durbin panel model, following Peng and Hong (Citation2013). It is used to answer the first two hypotheses on the existence of productivity spillovers in the intra-industry linkage (both downstream and upstream).
A’ is a constant, and δ1 is the inter-sector autoregressive coefficient reflecting knowledge spillover. It is expected to be positive when a sector’s productivity levels are positively related to a linear combination of other sector values. The terms θ1,θ2, θ3, θ4, and θ5 are parameters; i = 1, …, N sector; t = 1, …, T is the period; the subscript it means own sector and jt means other sector; Q’/L’ is average sectoral labor productivity; K/L’ is average sectoral physical capital per worker; Skill’ is the average sectoral ratio between non-production workers and total workers; Age’ is average sectoral age; Import is the average ratio of the material’s imported and total material used; Foreign’ is the average share of foreign capital ownership; Dummytech equals one if capital-intensive sectors and equals zero if labor-intensive sectors, and is an inter-industry matrix (314 × 314). This model states that the dependent variable in sector i is influenced by the dependent and independent variables in sector j.
is a weighted average of productivity of other sectors, it captures the inter-sectoral effects working through dependent variables, while
, and
Foreign’jt refers to the average of independent variables from other related sectors. θ1, θ2, θ3, θ4, and θ5 are the parameters associated with these variables. These coefficients estimate the inter-sectoral effect of the explanatory variables and capture two types of externality effects: first, the effect of working through labor productivity as a dependent variable, and second, the effects working through independent variables.
Therefore, if the coefficient δ1 is positive then productivity spillover flows among linked sub-sectors through forward linkages (downstream spillover effects) and backward linkages (upstream spillover effects).
4.4. Inter-regional model
The model in equation (8) is a spatial Durbin panel model, following Peng and Hong (Citation2013). It is used to answer the second hypothesis on the existence of productivity spillovers in intra-regional and inter-regional linkages.
C is a constant, and δ2 is the inter-regional autoregressive coefficient reflecting knowledge spillover. It is expected to be positive, indicating that a region’s productivity levels are positively related to a linear combination of other region values. The terms θ’1, θ’2, and θ’3 are parameters; i = 1, …, R region; t = 1, …, T is the period; the subscript st means own region and mt means other region; In this spatial Durbin panel model, all values of variables are in the region-level average that consists of 223 regions. Y/L is labor productivity, K/L is the physical capital per worker, and specialization is the location quotient (LQ) index. It quantifies how concentrated a particular industry is in a region rather than a nation. It can reveal what makes a particular region “unique” compared to the national average; Infra/Lr is the infrastructure index per total labor in a region. The index is based on accessibility to water, electricity, and roads in each district and the regency. Wsm is the spatial weight distance matrix (223 × 223). This model states that the dependent variable in region s is influenced by the dependent and independent variables in the related region m. In this model, geographical proximity is a channel for the transmission of technology spillovers between regions. If the coefficient δ2 is positive, this implies that a productivity spillover exists among neighboring regions based on geographic distance.
4.5. Moran-I test and Moran scatter plot
The Moran-I test and Moran scatter plot are used to examine inter-sectoral and inter-regional spillovers. Then, the results are compared with the results from previous methods as robustness checks.
4.5.1. Inter-sectoral spillover
The Moran-I test is used to measure inter-sectoral spillover in terms of TFP and labor productivity indicators using the following formula:
Wij refers to the inter-industry weight matrix, industry i sell/buy output to industry j, and y is the dependent variable (productivity).
4.5.2. Inter-regional spillover
The Moran-I test is used to measure the inter-regional spillover in terms of TFP and labor productivity indicators using the following formula:
Wsm refers to the spatial weight matrix, region s supply/receives information from region m, and y is the dependent variable (productivity).
4.6. Direct and indirect effect of knowledge spillover
The interconnection with other sectors or regions in the economy means that inter-sectoral linkages and inter-regional linkages provide sectors or regions with access to a greater amount of technical knowledge that they do not directly produce (Olivier et al., Citation2005; Abreu et al., Citation2004). Inter-sectoral interactions exist between suppliers and customers because of inter-sectoral linkages. This means that a particular sector has access to the knowledge produced by its input demanders, direct knowledge spillovers, and the total knowledge to which these sectors have access, indirect knowledge spillovers. In the inter-regional linkage, the total knowledge available in the domestic economy is the sum of regional knowledge and the total available knowledge for inter-regional linkages. Then, we break down the total knowledge as the sum of the direct and indirect effects in Equationequation (11)(11)
(11) below. These effects are used to answer the third hypothesis that there is a positive relationship between knowledge spillover and absorptive capacity.
Total Effect = Direct effect + Indirect effect
4.7. Intensity of R&D
The sub-sectors of manufacturing are classified by R&D intensity taken from the OECD (Citation2011) in : high-tech, medium-high-tech, medium-low-tech, and low-tech. We do not use our R&D data because it is challenging to obtain R&D data in Indonesia. Therefore, this study’s analysis uses only two categories: high-tech and low-tech because of insufficient sample firms in the other two categories: medium-low tech and medium-high tech. Therefore, medium high-tech is included in the high-tech industry group (capital-intensive industries), and medium low-tech industries are included in the low-tech industry (labor-intensive industries).
Table 5. The classification of sub-sector of manufacturing based on R&D intensity
5. EMPIRICAL RESULT
5.1. Inter-sectoral spillover
We explain the inter-sectoral model’s descriptive statistics for each variable in . We show that the average labor productivity varies between 3.182 and 17.612 with a standard deviation of 1.445 at the firm level and between 8.056 and 17.375, with a standard deviation of 1.182 at the sectoral/5-digit ISIC level.
Table 6. Descriptive statistics of inter-sectoral variables (2010–2014)
We introduce the dummy variable (Dummyinter). This variable represents the types of inter-sectoral linkages. It equals one if an industry pairs with another industry in different sectors and equals 0 if an industry pairs with another industry in the same or similar sectors. The classification used in the same/similar sectors and sectors is based on 2-digit ISIC. Next, we present the matrix of correlations between the variables in .
Table 7. Correlation matrix of inter-sectoral variables (2010–2014)
In the next section, we present the inter-sectoral spillover results using the panel models in . We test the best panel models between fixed and random effects, both upstream and downstream, in analyzing the models. The results are as follows:
Upstream spillover. We use the Hausman test to choose the best model between the fixed and random effects. The preferred model is the random effect model (chi2 = 1040.27; prob>chi2 = 0.000).
Downstream spillover. We use the Hausman test to choose the best model between the fixed and random effects. The preferred model is the random effect model (chi2 = 1031.21; prob>chi2 = 0.000).
Table 8. Estimation results of panel models (2010–2014)
Based on the random effect model (the selected model), our findings show a positive significant impact of explanatory variables on labor productivity (ln(Q/L)it) such as a) capital per labor (ln(K/L)it) in upstream (0.176***) and downstream (0.160***); b) labor skill (ln(Skill)it) in upstream (0.163***) and downstream (0.124***); c). imported materials (Importit) upstream (0.540***) and downstream (0.572***); d). foreign capital ownership (Foreignit) in upstream (0.006***) and downstream (0.007***); e) and the maturity of firms (Ageit) upstream (0.008***) and downstream (0.007***). Our findings suggest that the channel of foreign technology entry to domestic firms is stronger through imported materials than foreign ownership share (FDI).
Another important factor is the coefficient of the dummy variable (Dummyinterjt) that was used to answer the first hypothesis. The hypothesis is:
H1a. There is a more dominant effect from the inter-industry linkages between upstream and downstream.
Based on the random effect model (the selected model), by the value of the coefficient of the Dummy-Inter variable (Dummyinterjt), our findings suggest that inter-industry linkages positively affect productivity in the downstream industries (0.484***), while in the upstream industries, this effect is negative and not significant (−0.025). This implies that productivity spillover flows dominantly through inter-industry linkages in downstream than inter-industry linkage in upstream. Next, our results in below are used to examine the previous results as robustness checks using Moran-I test in equation (9).
Table 9. Moran-I test results of inter-sectoral spillover (2010–2014)
shows that the TFP indicator is better than the labor productivity indicator in explaining the existence of productivity spillover among linked sectors in vertical linkage or inter-sectoral spillover. Our finding support Schreyer (Citation2001), who mentions that TFP can explain the source of productivity that is driven not only by investment in traditional capital (rise in capital intensity) but also investment in intangible capital (spillover, innovation, organizational change, R&D). The main results from show that inter-industry linkage in downstream dominantly generates more productivity spillover than inter-industry linkage in upstream. These results are the same as previous results. Therefore, the downstream spillover effect dominantly flows from the customer to the supplier through inter-industry linkages (hypothesis H1a is proven).
Next, we present the inter-sectoral spillover results using the inter-sectoral Durbin panel model in to answer the first (two) hypothesis. The hypothesis is:
H1b. There is a more dominant effect from the intra-industry linkages between upstream and downstream.
Table 10. Estimation results of inter-sectoral Durbin panel model (2010–2014)
(in the first row) below shows productivity spillover (both upstream and downstream) in the intra-industry linkage.
In analyzing the models, similar to the previous model, we choose the best model (between fixed effect and random effect model), and we perform the test of both models using a Hausman test. The results are as follows:
Upstream spillover. We find that the preferred models are the random effect model (chi2 = 51.17; prob>chi2 = 0.000) on capital in-intensive intra-industry spillover and fixed effect model (chi2 = −33.95) on labor-intensive intra-industry spillover.
Downstream spillover. We find that the preferred models are the random effect model (chi2 = 44.91; prob>chi2 =0.000) on capital-intensive intra-industry spillover and the fixed effect model (chi2 =- 33.92) on labor-intensive intra-industry spillover.
Based on the upstream spillover (the left side), our findings show that intra-industry linkage positively affects productivity by the value of coefficient (σ1) for capital-intensive industries (0.526**) and low-tech, labor-intensive industries (0.264**). Based on the downstream spillover (the right side), our findings suggest that intra-industry linkages do not have a significant effect on productivity by the value of coefficient (σ1) for capital-intensive industries (0.346) and labor-intensive industries (0.217). This implies that productivity spillover flows dominantly through intra-industry linkages in upstream than intra-industry linkage in downstream. Therefore, the upstream spillover effect dominantly flows from supplier to customer in intra-industry linkages (hypothesis H1b is proven). Based on hypothesis H1a and H1b above, our findings support that knowledge spillovers flow through intra-industry and inter-industry spillovers (Bernstein & Nadiri, Citation1989; Los & Verspagen, Citation2000; Sena, Citation2004).
Next, Moran’s scatter plot () is used to map manufacturing sub-sectors. Sectors with the strongest productivity spillover are those in Quadrant I. According to Hirschman (Citation1958), those sectors are referred to as hub sectors. These hub sectors generate a multiplier effect on the economy. An example of this hub sector is the automotive industry (ISIC 29100). The graph based on the 5-digit ISIC in 2010 is shown in .
5.2. Inter-regional spillover
Inter-regional spillover is used to answer the second hypothesis about the existence of productivity spillovers through agglomeration based on geographic distance (inter-regional and intra-regional). First, we present the descriptive statistics in .
Table 11. Descriptive statistics of inter-regional variables (2010–2014)
The above descriptive statistics of inter-regional spillover variables in reveal that the average labor productivity varies between 1.215 and 17.100, with a standard deviation of 1.215. Next, we present the correlation matrix in . We introduce infrastructure per labor (Infra/Lr) and location-quotient (Specialization) variables specified in the inter-regional model.
Table 12. Correlation matrix of inter-regional variables (2010–2014)
Next, we present the results of inter-regional spillovers using the spatial panel Durbin model in . We choose the best model (between the fixed effect and random effect model) using the Hausman test to analyze the models. The results are as follows:
Table 13. Estimation results of spatial Durbin panel (2010–2014)
a. Inter-regional spillovers. We find that the preferred models between regions are the fixed effect model (chi2 = −29.32) for Indonesia’s all regions (including Java) and the fixed effect model (chi2 =−18.22) on Indonesia’s all regions (excluding Java).
b. Intra-regional spillovers. We find that the preferred models within each region are
Java uses fixed effect model (chi2 =0.54; prob>chi2 =0.9093)
Sumatera uses random effect model (chi2 =9.00; prob>chi2 =0.029)
Borneo uses fixed effect model (chi2 =2.92; prob>chi2 =0.403)
Bali uses random effect model (chi2 =23.25; prob>chi2 =0.000)
Celebes uses fixed effect model (chi2 =−11.74)
The results in are used to answer the second hypothesis that productivity spillovers exist among neighboring regions, based on geographic distance. This hypothesis is:
H2. There is a productivity spillover among (neighboring) regions
Based on the first row in , our findings show that productivity spillover flows significantly in intra-region (within) (Java (0.801***) and Celebes (0.715***)) and inter-region (between) (Indonesia’s all regions include Java (0.652***) and Indonesia’s all regions exclude Java (0.492**)). It means that productivity spillover within the region (intra-regional) is higher than the productivity spillover between the regions (inter-regional). This implies that knowledge spillover flows more on the specific core region (a region surrounded by other regions with similar characteristics), such as Java, which contains sub-regions (i.e., districts and regencies with Jakarta as a center and surrounded by satellite regions such as Karawang, Bekasi, Tangerang, Bogor, etc.) that cause agglomeration. These findings support Krugman (Citation1991) in his New Economic Geography (NEG) theory that the closer the distance, the greater the interaction between industries. Moreover, in a specific core region such as Java, it is easier to exchange material, joint use of machinery, labor migration, and cooperation between firms or companies than regions outside Java. Our findings support those of Stiller (Citation2018). He argued that productivity spillover exists because a shorter geographical distance narrows the technology gap. Next, our results in below are used to examine the previous results as robustness checks using Moran-I test in Equationequation (10)(10)
(10) .
Table 14. Moran-I test results of inter-regional spillover in Indonesia (2010–2014)
Table 15. Moran-I test results of intra-regional spillover in Java (2010–2014)
shows that the TFP indicator is better than the labor productivity indicator in explaining the existence of productivity spillover between the regions (inter-regional spillover). These results also support Schreyer (Citation2001), who mentions that TFP can explain the source of productivity that is driven not only by investment in traditional capital (rise in capital intensity) but also investment in intangible capital (spillover, innovation, organizational change, R&D). shows the productivity spillover within the region (intra-regional) on the Java islands.
Based on the results in the two tables above (), our findings suggest that productivity spillover within the region (intra-regional) is higher than the productivity spillover between the regions (inter-regional). These results are the same as previous results.
Next, spatial descriptive is used to map the distribution of the manufacturing’s TFP by region (districts and regencies) throughout Indonesia, as shown in and Java island, as shown in .
show that the darker the color, the higher the productivity or TFP. Most of the regions with the highest TFP are located on Java Island. Next, the Moran scatter plot is used to map each region’s position in .
show that regions with the strongest productivity spillover are located in Quadrant I, referred to as core regions (e.g., Jakarta and its satellite region). These regions own industrial districts or clusters that form industrial agglomerations. Therefore, productivity spillover exists among neighboring regions (hypothesis H2 is proven).
5.3. The relationship between knowledge spillover and absorptive capacity
We analyzed both inter-sectoral and inter-regional spillover using spatial econometric estimations in the section above. From the positive coefficients of δ1 and δ2, we know that knowledge spillover positively impacts productivity in the Indonesian manufacturing industry. However, the degree to which each firm/industry/region benefits from these spillovers depends on the absorptive capacity owned by the firms/industries/region itself (Cohen & Levinthal, Citation1990). Therefore, we analyzed the relationship between knowledge spillover and absorptive capacity to answer the third hypothesis. The hypothesis is:
H3. There is a positive relationship between knowledge spillover and absorptive capacity.
To test this hypothesis, we use the concept of technological gap and investment in human and physical capital in the internal industry/region. Both investments are located in the explanatory variables as a proxy for absorptive capacity. This study follows that of Caragliu et al. (Citation2012). They argued that the explanatory variable’s direct effect on TFP is represented the absorption capacity factors. Following their models, both investments narrow the technology gap between developing and advanced firms/industries/regions as a precondition requirement to absorb technology if their effects are significantly positive.
5.4. Direct and indirect spillover effect
Based on the inter-sectoral spillover in (on the left side) below, the TFP is computed using Levinsohn Petrin (LP) formula on the firm level. Then, the results are aggregated at the sectoral level (as an average value). Sub-sectors TFP is then regressed with the inter-sectoral Durbin panel model in Equationequation (4)(4)
(4) . In this model, Dummytech is used to proxy for the technology gap. Dummytech equals one if the sectors’ sub-samples are capital-intensive industries that reflect a lower technology gap. Dummytech equals 0 if the sectors’ sub-samples are only labor-intensive industries that reflect a higher technology gap.
Table 16. Direct and indirect spillover effect (2010–2014)
In analyzing the models, we choose the best model (between fixed effect and random effect model), and we perform the test of both models using a Hausman test. The results are as follows:
Capital-intensive industries (high absorption). We find that the preferred model is the fixed-effect model (chi2 = −0.01).
Labor-intensive industries (low absorption). We find that the preferred model is the fixed effect model (chi2 = − −14.94).
Based on the inter-regional spillover in (on the right side), the TFP at the firm level is measured using the Levinsohn–Petrin (LP) formula. Then, the results are aggregated at the regional level (as an average value). The regions’ TFP are then regressed with the spatial Durbin panel model in EquationEquation (8)(8)
(8) . Similar to previous studies, Dummytech is used to proxy the technology gap. Dummytech equals one if the regions’ sub-sample is only in the core regions that reflect a lower-gap technology. Dummytech equals 0 if the region’s sub-sample is only in the non-core regions that reflect a higher technology gap. Similar to previous studies, we choose the best model (between fixed effect and random effect model), and we performed the test of both models using a Hausman test. The results are as follows:
All the regions in Java have high absorption. We find that the preferred model is the fixed-effect model. (chi2 = 0.11).
Indonesia, all regions exclude Java (low absorption). We find that the preferred model is the fixed-effect model (chi2 = −24.13).
By definition of absorptive capacity as the capability to take in new information, it tends to close with the internal factors of firm/industry/region. Therefore, the factors are located in the coefficient values of the explanatory variables of sectors/regions. Based on the inter-sectoral spillover in (on the left side), the absorptive capacity is located on the direct effect of explanatory variables such as a) skill labor variable (ln(Skill)) by the value (0.284**) on capital-intensive but a negative value (−0.004) on labor-intensive; b) capital per labor (ln(K/L)) by the value (0.095**) on capital-intensive industries and (0.044***) on labor-intensive industries. Based on the inter-regional spillover in (on the right side), the absorptive capacity is located on the direct effect of explanatory variables such as a) physical capital per labor (ln (K/L)) by the value (0.058***) in Java, but a negative value (−0.024) outside Java; b) the location-quotient/LQ (ln (Specialization)) by the value (0.920***) in Java and (0.451***) outside Java.
Based on both results (inter-sectoral spillover and inter-regional spillover), our findings suggest that the knowledge spillover created from capital-intensive industries (high absorptive capacity) is higher than that of labor-intensive industries (low absorptive capacity). Second, knowledge spillover created from a core-region/Java (high absorptive capacity) is higher than that from the non-core regions/outside Java (low absorptive capacity). Therefore, the results imply a positive relationship between knowledge spillovers and absorptive capacity. Hence, Hypothesis H3 is supported.
5.5. Discussion and analysis
In this study, we aim to disseminate the transmission of knowledge spillover in the Indonesian manufacturing industry by using productivity spillover through inter-sectoral spillover (which originates from the externality of the input-output/vertical linkage) and inter-regional spillover (which originates from agglomeration based on geographical proximity).
5.5.1. Inter-sectoral spillover
Our finding answers the first hypothesis that in the inter-sectoral approach, spillover flows dominantly through inter-industry linkages (mainly via downstream) and intra-industry linkages (mainly via upstream) from the transaction of intermediate goods between customers and suppliers. Therefore, this study contributes to distinguishing productivity spillovers between closely related industries. Our findings are consistent with those of Lopez and Sudekum (Citation2007) who found that intra-industry linkage in Chile’s manufacturing sector occurred mainly upstream. In Indonesia, inter-industry spillover activities mainly occur downstream, while intra-industry spillovers mainly occur upstream. Our results also support the findings of Los and Verspagen (Citation2000) and Javorcik (Citation2004) who argue that industrial spillover depends on more collaborative research efforts through upstream intra-industry linkages compared to downstream inter-industry linkages.
Next, we describe the flow of intermediate goods in vertical linkage. We take the automotive industry’s supply chain network (ISIC 29100) as the hub sector to explain this inter-sectoral spillover with greater clarity. In this industry, first, the raw material (for example, iron) is processed into mixed iron and processed again into a metal alloy as an intermediate good input. The intra-industry linkage conducts these processes in the upstream industries by a few steel industries. Then, they create upstream spillover effects from a few multinationals (MNCs) of steel producers (who produce metal alloys) to local/domestic metalworking downstream industries. These local industries process this input (metal alloy) into parts or components. The upstream spillover flows through good-quality material input (metal alloy) produced by the intra-industry collaboration of a few MNCs of steel producers delivered to local/domestic metalworking industries to produce parts and components. These parts and components are then delivered to many downstream industries as inter-industry linkages, such as motor vehicle assembly, construction, accessories, and shipbuilding industries. The downstream spillover effects will flow from customers, such as the MNC of motor vehicle manufacturers (e.g., Toyota and Honda) to the local/domestic metalworking industries as suppliers of parts and automotive components. In this case, knowledge spillovers flow from technical expert coaching, renting a (modern) machine, and doing business consultation conducted by the customer so that their local/domestic suppliers can fulfill the standard requirements of products related to quality, cost, and delivery (QCD). With these two types of spillovers (downstream spillover effect and upstream spillover effect), the local/domestic suppliers can produce a range of products with high quality and competitive prices that enhance their productivity and efficiency.
Regarding the positive effect of inter-sectoral linkages on productivity, this empirical result implies that the government should create a business climate conducive to supporting linkages and partnerships between upstream, midstream, and downstream industries. The government must support the local/domestic intermediate goods supplier industries to be part of the global supply chain network to absorb and diffuse technological knowledge from their trading partners (intra-industry and inter-industry) to other local/domestic industries.
Other interesting facts also arise. First, we claim a positive impact on productivity spillover resulting from the investment of imported materials. This result supports Coe and Helpman (Citation1995) and Grossman and Helpman (Citation1991), who argued that knowledge capital is embodied in imported goods. Our findings suggest that foreign technology’s entry channel to domestic firms is stronger through imported materials than foreign direct investment (FDI). We argue that imports could result in a spillover effect when domestic firms learn imported goods’ innovative content. This finding is also supported by Coe et al. (Citation1997), who estimated a large positive effect of import-weighted foreign R&D investment. Second, we claim that foreign capital ownership positively impacts domestic/local industries’ productivity. Our findings are consistent with the findings of Fransman (1985) and Kokko and Blomstrom (Citation1996) who argue that the channels through which MNCs diffuse technology are: a) active and formal transactions (joint ventures, licensing), b) active and informal transactions (linkage), c) passive and formal transactions (goods trade), and passive transactions (trade journals, scientific exchange). Our findings are also consistent with those of Redding and Proudman (Citation1998) who argue that trade openness in international trade can affect the domestic innovation rate through technology transfer and the adoption of advanced foreign technologies. Third, we claim that firm maturity positively affects productivity. This implies that this positive productivity spillover benefits mature firms and industries. Our findings are consistent with Porter’s (1980) industrial life cycle theory. According to him, the industrial life cycle refers to the industry’s four-stage evolution based on the business characteristics commonly shown in each phase. The industry’s four phases of the life cycle are introduction, growth, maturity, and decline.
5.5.2. Inter-regional spillover
The next finding answers the second hypothesis that using the inter-regional approach, industry agglomeration based on geographic distance produces externalities that create productivity spillover among neighboring regions. Our findings suggest that productivity spillover created from within the region (intra-regional) is stronger than the productivity spillover created between regions (inter-regional). This implies the important role of geographical and technological distance in producing knowledge spillovers in Indonesia.
The results confirm previous productivity spillover relevance and dependence on geographical and technological distance. As Boschma (Citation2005) and Stiller (Citation2018) describe, the major role of geographic distance is its reinforcing effect on other proximity dimensions. Geographically close (core regions) are more likely to develop similar industry structures and increase technological proximity. In constructing the model, we classified regions between the two groups. The first group is the core region. This region has the complete infrastructure and capital (including ICT technology). In Indonesia, the core region can be proxied by Java islands (Raharja & Kuncoro, Citation2012) because it fulfills the above requirements of capital and infrastructure. The second group is a non-core region that can be proxied by other islands (excluding Java). These regions lack infrastructure and other physical capital (electricity, ICT, roads, bridges, universities, laboratories, industrial districts, etc.).
Agglomeration is important for facilitating the growth of modern manufacturing activities. The manufacturing agglomeration process is present in Indonesia. While manufacturing activities across Indonesia often started in certain major cities such as Jakarta, Bandung, and Surabaya. The process also seems to be associated with productivity gains and increased product variety (specialization) by region. The specialization of selected industries continues until now, such as garments in the Bandung area, machinery and heavy industries in the Greater Jakarta and Surabaya areas, rattan in Cirebon and Jepara, leather footwear in Sidoarjo, and Ceramics in Plered (West Java). This implies that investment in public capital, such as industrial innovation centers in each region, will generate strong and positive productivity spillover effects. The development of infrastructure also promotes sectoral factor mobility, leads to specialization, and increases production possibilities (Baffes & Shah, Citation1998). Infrastructures such as freeway toll roads and ICT are needed to build another new core region that will increase the connectivity of the region and enhance productivity spillover.
5.5.3. Absorptive capacity
The third finding answers the third hypothesis that there is a positive relationship between knowledge spillover and absorptive capacity and what factors contribute positively to absorptive capacity. First, our results suggest that investment in human capital and physical capital are important absorptive capacity factors that narrow the technology gap between developing and advanced firms/industries/regions as a prerequisite for absorbing technology and enhancing productivity (Kokko and Blomstrom, Citation1996; Giroud, Citation2012; Marin & Bell, Citation2006). Second, knowledge spillover created from capital-intensive industries (high absorptive capacity) is higher than that from labor-intensive industries (low absorptive capacity). Third, knowledge spillover created from the core region (high absorptive capacity) is higher than that from the non-core region (low absorptive capacity). Therefore, there is a positive relationship between knowledge spillovers and absorptive capacity.
6. CONCLUSION AND POLICY IMPLICATIONS
In this study, we analyze the effect of knowledge spillover on productivity in the Indonesian manufacturing industry from 2010 to 2014 using inter-sectoral and inter-regional linkages. Contrary to the existing studies, we apply, for the first time, an input-output analysis as a proxy of inter-sectoral spillover and geographic distance as a proxy for inter-regional spillover using spatial econometric estimation. We focus more on absorbing knowledge (both tacit and codified) optimally, adapting the technology, and incorporating that technological knowledge into production methods or processes to increase the local/domestic industrial productivity and efficiency. Our significant findings can be summarized as follows:
First, productivity spillover from intermediate goods transactions in vertical linkage (customer-supplier) is dominated by inter-industry downstream and intra-industry upstream. Therefore, the downstream spillover effect predominantly flows from customers to suppliers through inter-industry linkages, while the upstream spillover effect predominantly flows from suppliers to customers through intra-industry linkage. Domestic industries/local industries can absorb knowledge by becoming suppliers of parts and components to their downstream/MNC industries and becoming customers of intermediate goods produced by their similar upstream sectors. This implies that the government should support and create a tied linkage between upstream, midstream, and downstream industries to enhance productivity spillover among the linked sectors.
Second, our findings suggest that foreign technology’s entry channel to domestic firms is stronger through imported materials than foreign direct investment (FDI). The government may continue to support the local intermediate goods/supplier industries to become part of the global supply chain network and encourage foreign-owned companies to establish partnerships with domestic/local supplier industries so that domestic/local supplier industries can absorb and disseminate technological knowledge possessed (from advanced foreign/MNC companies) to other domestic and local industries.
Third, productivity spillover created from intra-regional spillover (within the region) is stronger than the productivity spillover created from inter-regional spillover (between the region), which implies that the shorter the geographic distance, the narrower the technology gap. This implies that investment in new public capital in specific regions, such as infrastructure and industrial-specific zones in the new-core region, will generate strong and positive productivity spillover effects. Development infrastructure such as ICT and freeway toll roads (especially outside Java) will increase the connections among sectors and developing new industrial-specific zones (new core regions) in critical sectors will promote the spillover of related sectors and improve systemic competitiveness.
Fourth, investment in human and physical capital (land, building, machinery, etc.) are essential absorptive capacity factors for firms/industries/regions. This implies that both investments narrow the technology gap between developing and advanced firms/industries/regions as a precondition requirement to absorb technology.
Fifth, knowledge spillover created from capital-intensive industries is higher than that of labor-intensive industries. This implies that the Indonesian government has the right path to boost sectoral labor productivity through the national program of Indonesia 4IR by implementing digital technology 4.0 in the Indonesian manufacturing sector.
Acknowlegement
The authors thank the editors, two anonymous reviewers, and Dr. Arie Damayanti for their insightful comments. We request to add Dr. Arie Damayanti in acknowledgment if she can not be the third co-author.
Additional information
Notes on contributors

Irvan Kuswardana
Irvan Kuswardana holds a B.E. in Electrical Engineering from Bandung Institute of Technology and obtained his Master’s in Magister Management from Padjadjaran University and Ph.D. students in Economics from Universitas Indonesia. He currently works as industrial analyst at the Ministry of Industry of the Republic of Indonesia. His research interests include industrial policy, automotive industry, and international trade.
Nachrowi Djalal Nachrowi
Nachrowi Djalal Nachrowi is a professor of economics at the Department of Economics, Faculty of Economics and Business, Universitas Indonesia. He is an expert on the manufacturing industry, finance, labor economics, and international trade.
Telisa Aulia Falianty
Telisa Aulia Falianty is an associate professor at the Department of Economics, Faculty of Economics and Business, Universitas Indonesia. He received his B.S. degree, Master’s and Ph.D. degrees in Economics from Universitas Indonesia. She teaches several subjects such as international trade and international finance. Her other specialty is on macroeconomic policy.
Arie Damayanti
Arie Damayanti is a lecturer in the Faculty of Economics and Business, Universitas Indonesia. She received her B.S. in Economics from Universitas Indonesia, M.Sc. from University of Essex, England, and Ph.D. degrees from Kyoto University, Japan. She is an expert on econometrics and labor economics.
Notes
1. “Core” includes DKI Jakarta and West Java, while “non-core” includes Central Java, East Java, Banten, Lampung, Bali, North Sumatera, and South Sulawesi.
References
- Abreu, M., De Groot, H. L. F., & Florax, R. J. G. M. (2004). Space and growth: A survey of empirical evidence and methods. Region Et Developpement, 21(21, 13), 13–31. https://doi.org/10.2139/ssrn.631007
- Arrow, K. J. (1962). The economic implications of learning by doing. Review of Economic Studies, 29(3), 155–173. https://doi.org/10.2307/2295952
- Audretsch, D., & Feldman, M. (1996). R&D spillovers and the geography of innovation and production. American Economic Review, 86(3), 630–640. https://www.jstor.org/stable/2118216
- Aw, B.-Y., & Hwang, A. R. (1995). Productivity and the export market: A firm-level analysis. Journal of Development Economics, 47(2), 313–332. https://doi.org/10.1016/0304-3878(94)00062-H
- Baffes, J., & Shah, A. (1998). Productivity of public spending, sectoral allocation choices, and economic growth. Economic Development and Cultural Change, 46(2), 291–303. https://doi.org/10.1086/452339
- Bartelsman, E. J., Caballero, R. J., & Lyons, R. K. (1994). Customer- and supplier-driven externalities. American Economic Review, 84 (4), 1075–1084. 1994.
- Bernstein, J., & Nadiri, M. (1989). Research and development and intra-industry spillovers: an empirical application of dynamic duality. Review of Economic Studies, 56(2), 249–267. https://doi.org/10.2307/2297460
- Boschma, R. A. (2005). Proximity and Innovation: A critical assessment. Regional Studies, 39(1), 61–74. https://doi.org/10.1080/0034340052000320887
- Brown, M., & Conrad, A. (1967). The Influence of Research and Educations on CES Production Relations. In Brown, M. (ed.), The Theory and Empirical Analysis of Production, Studies in Income and Wealth, Vol. 3, New York: Columbia University Press, 275–340..
- Caniëls, M., & Romijn, H. A. (2003). Agglomeration advantages and capability building in industrial clusters: the missing link. The Journal of Development Studies, 39(3), 129–154. https://doi.org/10.1080/00220380412331322851
- Caragliu, A., & Bo, D., Chiara, Nijkamp. (2012). c. Creative Knowledge Cities: Myths, Visions and Realities. 10.4337/9780857932846.00016
- Clare, R. (1996). Multinationals, linkage, and economic development. American Economic Review, 86(4), 852–873. http://www.jstor.org/stable/2118308
- Coe, D. T., Helpman, E., & Hoffmaister, A. W., & Coe. (1997). North-South R&D spillovers. The Economic Journal, 107(440), 134–139. https://doi.org/10.1111/1468-0297.00146
- Cohen, W. M., & Levinthal, D. A. (1990). Absorptive capacity: A new perspective on learning and innovation. Administrative Science Quarterly, 35 (1), 128–152. Special Issue: Technology, Organizations, and Innovation. https://doi.org/10.2307/2393553
- David, C & Elhanan, H. (1995), International R&D spillovers, European Economic Review, 39 (5), 859–887.
- Elkomy, S., Ingham, H., & Read, R. (2020). The impact of foreign technology and embodied R&D on productivity in internationally oriented and high-technology industries in Egypt, 2006–2009. Journal of Industry, Competition and Trade. https://doi.org/10.1007/s10842-020-00349-x
- Fagerberg, J. (1995). Convergence or divergence? the impact of technology on “Why Growth Rates Differ”. Journal in Evolutionary Economics, (3), 269–84. https://doi.org/10.1007/BF01198307
- Findlay, R. (1978). Relative backwardness, direct foreign investment, and the transfer of technology: A simple dynamic model. The Quarterly Journal of Economics, 92(1), 1–16. https://doi.org/10.2307/1885996
- Girma, S., Kneller, R., & Pisu, M., & Girma. (2005). Exports versus FDI: an empirical test. Review of World Economics, 141(2), 193–218. https://doi.org/10.1007/s10290-005-0025-9
- Giroud, A. (2012). Mind the gap: how linkages strengthen understanding of spillovers. European Journal of Development Research, 24(1), 20–25. https://doi.org/10.1057/ejdr.2011.48
- Greenstone, M., Hornbeck, R., & Moretti, E. (2010). Identifying agglomeration spillovers: evidence from winners and losers of large plant openings. Journal of Political Economy, 118(3), 536–598. https://doi.org/10.1086/653714
- Griliches, Z. (1979). Issues in assessing the contribution of R&D to productivity growth. Bell Journal of Economics, 10(1), 92–116. https://doi.org/10.2307/3003321
- Grossman, G. M., & Helpman, E. (1991). Endogenous growth: trade, knowledge spillovers, and growth. European Economic Review, 35(2–3), 517–526. https://doi.org/10.1016/0014-2921(91)90153-A
- Henderson, V., Kuncoro, A., & Turner, M. (1995). Industrial development in cities. Journal of Political Economy, 103(5), 1067–1090. https://doi.org/10.1086/262013
- Hirschman, A. O. (1958). The strategy of economic development. Yale University Press.
- Jacobs, J. (1969). The economy of Cities. Random House, 1969. https://doi.org/10.1177/107808747000500406
- Jaffe, A. B. (1998). The importance of “spillovers” in the policy mission of the advanced technology program. Journal of Technology Transfer, 23(2), 11–19. https://doi.org/10.1007/BF02509888
- Javorcik, B. S. (2004). Does foreign direct investment increase the productivity of domestic firms? in search of spillovers through backward linkages. American Economic Review, 94(3), 605–627. https://doi.org/10.1257/0002828041464605
- Kokko, A., & Blomström, M. (1996). The impact of foreign investment on Host Countries: A review of the empirical evidence. World Bank Policy Research Working Paper, 1745, 44.
- Krugman, P. (1991). Increasing returns and economic geography. Journal of Political Economy, University of Chicago Press, 99(3), 483–499. https://doi.org/10.1086/261763
- Kuncoro, M., & Wahyuni, S. (2009). FDI impacts on industrial agglomeration: the case of Java, Indonesia. Journal of Asia Business Studies, 3(2), 65–77. https://doi.org/10.1108/15587890980000412
- López, R., & Suedekum, J. (2007). Vertical industry relations, spillovers and productivity: evidence from chilean plants. Journal of Regional Science, 49. 10.1111/j.1467-9787.2009.00631.x.
- Los, B., & Verspagen, B. (2000). R&D spillovers and productivity: evidence from U.S. manufacturing microdata. Empirical Economics, 25(1), 127–148. https://doi.org/10.1007/s001810050007
- Marin, A., & Bell, M. (2006). Technology spillovers from foreign direct investment (FDI): the active role of MNC subsidiaries in Argentina in the 1990s. Journal of Development Studies, 42(4), 678–697. https://doi.org/10.1080/00220380600682298
- Marshall, A. (1920). Principles of Economics (8th ed). Macmillan and Co. 1920.
- Moretti, E. (2004). Workers’ education, spillovers, and productivity: evidence from plant-level production functions. American Economic Review, 94(3), 656–690. https://doi.org/10.1257/0002828041464623
- OECD. (2011) . ISIC Rev.3 Tehnology Intensity Definition: Classification of manufacturing industries into categories based on R&D intensities. Directorate for Science, Technology, and Industry. Economic Analysis and Statistics Division.
- Olivier, L.-N., Olarreaga, M., & Schiff, M. (2005). On ‘indirect’ trade related research and development spillovers. European Economic Review, 49(7), 1785–1798. https://doi.org/10.1016/j.euroecorev.2004.06.004
- Orlando, M. (2004). Measuring spillovers from industrial R&D: on the importance of geographic and technological proximity. The RAND Journal of Economics, 35(4), 777–786. https://doi.org/10.2307/1593773
- Peng, L., & Hong, Y. (2013). Productivity spillovers among linked sectors. China Economic Review, 25(1), 44–61. https://doi.org/10.1016/j.chieco.2013.01.002
- Piga, C., & Medda, G. (2007). Technological spillovers and productivity in Italian manufacturing. Journal of Productivity Analysis, Springer. 41(3), 419–434. https://doi.org/10.1007/s11123-013-0351-1.
- Porter, M. E. (1996). Competitive advantage, agglomeration economies, and regional policy. International Regional Science Review, 19(1c2), 85–90. https://doi.org/10.1177/016001769601900208
- Raharja, S., & Kuncoro, A. (2012). Agglomeration and Manufacturing Activities in Indonesia. World Bank Policy Note 6, World Bank Office Jakarta.
- Redding, S. J., & Proudman, J. (1998). Productivity convergence and international openness. Bank of England Working Paper No. 77.
- Romer, P. M. (1990). Endogenous technological change. Journal of Political Economy, 98(5, Part 2), S71–S102. https://doi.org/10.1086/261725
- Schreyer, P. & D. Pilat (2001), “Measuring Productivity”, OECD Economic Studies, OECD Publishing.
- Schreyer, P. (2001). Measuring Productivity: OECD Manual. Measurement of Aggregate and Industry-Level Productivity Growth.
- Sena, V. (2004). Total factor productivity and the spillover hypothesis: some new evidence. International Journal of Production Economics, 92(1), 31–42. https://doi.org/10.1016/j.ijpe.2003.10.003
- Stiller. (2018). On the spatial economics of knowledge accumulation. Ph.D Thesis, Universitat Regensburg.
- Terleckyj, N. E. (1974). Effects of R&D on the productivity growth of industries: An exploratory study. National Planning Association, The World Input-Output Database (WIOD) November 2016.
- Wolff, E. N., & Nadiri, M. I. (1993). SPILLOVER EFFECTS, LINKAGE STRUCTURE, AND RESEARCH AND DEVELOPMENT. Structural Change and Economic Dynamics, 4(2), 315–331. https://doi.org/10.1016/0954-349X(93)90022-C