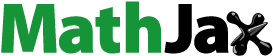
Abstract
In this article, optimal investment portfolios with minimal risk and maximum efficiency were calculated. The portfolios were designated for ten selected European stock exchanges, based on the listings of the twenty largest companies in each of those markets. All calculations were made based on company shares only, company shares and investments in gold, shares of companies and investments in crude oil as well as shares of companies and investments in gold and crude oil. The research hypothesis tested in the study is: The share of alternative investments in the investment portfolio does not depend on the stock exchange, but it differs depending on the length of the estimation window used. The study showed that for most exchanges there were statistically significant differences for the distribution of the determined weight for alternative investments. However, it was noted that the longer the estimation window, the greater the number of exchanges with no differences in the distributions of that weight. In addition, for portfolios with minimal risk, there were larger differences between the designated weights of alternative investments than for the same portfolios, which were determined based on maximizing efficiency. It was also found that the longer the estimation window, the higher the efficiency of the designated portfolios. Moreover, the investment in gold had an average weight, in four-element portfolios with a minimum risk, greater than 60%. Oil investment in the same portfolios had an average weight of 28%.
PUBLIC INTEREST STATEMENT
This paper investigates if using investments in gold and crude oil gives investors extra value in reducing risk or higher efficiency of portfolios on different stocks exchange. Author asses if in each analyzed market the share of these alternative investments is on the same level. For all calculations, Markowitz’s portfolio theory was adopted and Kruskal-Wallis tests were done. The empirical findings show that for portfolios with minimal risk, there were larger differences between the designated weights of alternative investments than for the same portfolios, which were determined based on maximizing efficiency. What is more, the share of gold and crude oil is on average almost half the value of the portfolio.
1. Introduction
The purpose of the article is to compare the share of investments in gold and oil in optimal investment portfolios characterized by minimal risk and maximum efficiency on selected European stock exchanges. Research conducted in that article answers if a share of investing in gold and crude oil is the same in different markets, and also how big these weights are. Currently, numerous scientific studies show that capital markets are closely related, hence it should demonstrate that the share of investments in gold or oil will be similar for individual national exchanges. Therefore, the study adopts the following research hypothesis: The share of alternative investments in the investment portfolio does not depend on the stock exchange, but it differs depending on the length of the estimation window used. Different gold shares depend on the length of the estimation window, the author combines with the phenomenon of high volatility that characterizes capital markets. In order to obtain a reliable answer for the research hypothesis, the study uses the quotations of the top twenty companies (in terms of capitalization) from each of the capital markets analyzed. Investments in these companies are referred to as blue-chip investments, which are characterized by a lower risk than other companies. Therefore, if the research hypothesis regarding the length of the estimation window is confirmed for this type of company, it can be strongly assumed that it is also true for other medium and smaller companies, which, in principle, are more volatile than the largest companies analyzed in this study. Investments in gold and oil were selected to supplement investment portfolios as representatives of the alternative investment market. Gold is considered a so-called safe haven, while investment in crude oil belongs to the same category as gold, an investment in commodities. As is described for example, in Šoja (Citation2019) gold is a very good choice for diversifying the portfolio. The following studies are important for investors especially in times of turmoil in financial markets, as they should provide an answer as to what should be the share of investment in gold and crude oil in an optimally constructed investment portfolio and if this share is the same in different markets.
The main contribution of this study is to determine optimal investment portfolios for ten national exchanges, for different lengths of estimation window and for one common assessment window. In the study, portfolios with minimal risk and maximum effectiveness were assessed in terms of efficiency, understood as the ratio of portfolio return to risk. The accepted measure of effectiveness, in all calculations, is the Sharpe ratio presented in Sharpe (Citation1994). In addition to determining three-, four- and five-element portfolios, the differences were assessed considering whether the choice of the estimation window had a significant impact on the results obtained and whether it was the same for all the exchanges examined, which should be indicated by a strong connection between capital markets. In addition, an assessment was made of how the inclusion of subsequent investments (gold or crude oil as well as gold and crude oil at the same time) affected the efficiency of investment portfolios. Author also compare obtained results with naive strategy.
The following part of the study is a literature review. Next, the third part describes the data used in the study and presents the research methodology. The fourth part presents the research results, which are summarized in the last part of this article.
2. Literature review
Numerous scientific studies have been written about the choice of gold and crude oil as the source of diversification in investment portfolios. In most of them, researchers assessed whether these investments constitute so-called safe haven investments in times of turmoil in capital markets.
Baur and McDermott (Citation2010), found that gold acts as a safe haven in selected European capital markets and in the US capital market, but the same characteristics were not confirmed for countries such as Australia, Canada, Japan, and for large emerging capital markets such as Brazil, Russia, China and India. In the study, the research period was thirty years from 1979 to 2009. The authors used the daily rate of return for analysis. Importantly, the study emphasized that investment in gold has the property of reducing losses during a period of turmoil in capital markets.
The same author in second paper Baur and Lucey (Citation2010), also analyzed two European markets (capital markets in the UK and Germany) and the capital market in the USA. It was also emphasized here that investment in gold is a hedge investment for capital markets. In addition, the authors concluded that in times of turmoil in the financial markets, investment in gold acts as a safe haven (within 15 days after the market has fallen sharply) but only for the stock market and not for the bond market. The research period adopted in the study was 1995–2005.
The same two European capital markets were analyzed in the study by Anand and Madhogaria (Citation2012). The authors focused on determining the correlation between the investment in gold and the main indices on the capital markets. The relationship between these variables was defined as weak, meaning that investment in gold can be seen as an investment to diversify the portfolio. The study was conducted in the period 2002–2011. In addition to these markets, the capital markets in the USA, Japan, India and China were also analyzed.
A study focussed on UK capital market Ciner et al. (Citation2013), emphasized that gold can be seen as an investment protecting against sudden turmoil in analyzed market. The authors also studied the US capital market during the period 1990–2010. In addition to investment in gold, investment in crude oil was also assessed, for which the above properties of the hedging investment could not be confirmed.
The role of gold in the context of a single capital market was also analyzed in the study by Potrykus (Citation2015). In this work the author analyzed whether gold can be seen as a safe haven in the context of the Warsaw Stock Exchange (WSE). Based on the research conducted, it was shown that investment in US dollars in gold is an example of a diversifying investment for WSE. This means that there was no moderate positive correlation between the rates of return on these investments in the long run. Furthermore, the investment in gold recorded in Polish zloty is an example of a hedging investment for the Polish capital market. It can, therefore, be concluded that the investment in gold, included in the investment portfolio consisting mainly of shares listed on the WSE contributed to reducing the risk of such a portfolio. This was evidenced by the negative values of the Pearson linear correlation coefficient between the rates of return on these investments. The research period adopted in this study was 1995–2015.
Investments in gold and crude oil were also assessed in the context of capital markets in the paper by Śmiech and Papież (Citation2017). The final conclusions of this work were that the investment in gold had the qualities of an investment made on the capital market in normal market conditions. Importantly, the assessment was made in several sub-periods in the 1995–2015 research period. In addition, the authors noted that gold is positively correlated with bonds while investment in oil is negatively correlated.
Accordingly, research into the strong connections in capital markets can be found in the following papers.
The integration of selected stock markets in Europe was studied by Majewska and Olbryś (Citation2017). The authors analyzed six capital markets, three in developed economies (the United Kingdom, France and Germany), and three in developing economies (Poland, Czech Republic and Hungary). The research period adopted in the work was 1993 to 2015. The work emphasized that there was no basis to reject the null hypothesis about the progressive integration of the markets studied. A statistically significant increase in this integration was noticed especially after the accession of the developing economies to the European Union. Moreover, stronger integration was recorded for the developed markets group than for the developing markets group. Also, in the 2007–2008 crisis, a different value for the integration indicator was recorded than in the pre-crisis period.
The connection of capital markets in times of crisis is also included in the study by Das et al. (Citation2019). The final conclusions of this work point to the heterogeneous impact of global crises on the time-varying value of links between the US stock market and its counterparts in the G7 group. This work analyzes the capital markets of the most developed economies in the world. The main research period adopted in the study is 1863–2010. The authors emphasize that the correlation value of the US stock market and other markets researched increased significantly in the period 1950–2010. There was no such strong interconnection before this period. Furthermore, financial crises increase the relationship between the markets studied. This relationship was observed in particular for the UK and US markets and for the US and Japan markets. A similar effect on correlations was also observed for France, Germany and Italy, but this effect was not statistically significant. Different effects were observed only for the US and Canadian markets.
Increased connections between capital markets in selected European countries are also presented in the study by Gjika and Horváth (Citation2013). The authors emphasize that the correlation between stock markets in Central Europe and between Central Europe vis-à-vis the euro area are strong. Importantly, the strength of these connections increases over time. The correlation increases particularly during periods of financial crisis, which limits the benefits of diversification during market turmoil. The study analyzed the period 2001–2011. Similar conclusions indicating an increase in the dependence of stock markets in times of crisis are drawn from the study by Yang et al. (Citation2014), which explores a larger sample of capital markets.
Saeedian et al. (Citation2019), explore the links between 40 stock markets. The authors note that the strength of the relationship is affected by geographical location and ultimately present three groups of similar markets. These are East Asia, Europe and the continent of America, respectively. The markets that are not assigned to any group are China, Iran, Pakistan, Qatar, Saudi Arabia, and Sri Lanka. The research period is from 2000 to 2015.
In the light of the literature research, which emphasizes the significant connections between stock markets, the shares of investments in gold and/or oil in investment portfolios should be similar in all analyzed markets. The author of this publication did not manage to reach a study analyzing the differences between the share of investments in gold and oil between selected European stock markets and depending on the assumed length of the estimation window. The following studies should, therefore, fill this gap.
3. Research methodology and data characteristics
The study used data for ten selected European stock exchanges. The twenty largest companies in terms of capitalization were selected for each stock exchange, for which all possible combinations of three-element portfolios were created. Portfolios of two types have been designated due to the investment objective, portfolios with minimal risk and maximum efficiency. For each of these portfolios, it was examined how the effectiveness measured by the Sharpe coefficient was shaped. The portfolio composition was determined based on the assumptions of Harry Max Markowitz’s portfolio theory. The most important assumptions of this theory are described by the following formula Jajuga and Jajuga (Citation2006), Sharpe (Citation1994), and Markowitz (Citation1952):
For the calculations, two additional assumptions were made Dębski (Citation2007):
where
ip—portfolio rate of return,
ik—rate of return from investment “k”,
wk—share (weight) of investment “k” in the portfolio,
σp—portfolio risk (standard deviation of the rate of return),
σk—risk of investment “k” (standard deviation of the rate of return),
pkm—coefficient of correlation of return rates on investment “k” and investment “m”,
Sp—effectiveness measure (Sharpe ratio),
if—risk-free rate of return,
n—number of investments in portfolio.
The study assumed that the risk-free rate of return was 2% per year. All returns used were calculated as daily logarithmic returns. The work set out three-element portfolios consisting only of traditional investments (the portfolios have been marked as the “only traditional” strategy). In addition, four-element portfolios were designated, which consisted of three traditional investments and investments in gold (the portfolios were marked as “only gold”) and three traditional investments and crude oil (the portfolios were marked as “only crude oil”). In the last group of five-element portfolios examined, the effectiveness of three traditional investments as well as gold and crude oil were examined (the portfolios were marked as “gold and crude oil”).
The research period adopted in the study was 01.01.2016 to 30.06.2019 and was divided into two windows, the so-called estimation window and an evaluation window. The data obtained in the estimation window was used to determine the optimal weights for each investment portfolio with maximum effectiveness (maximum Sharpe ratio) and minimal risk (minimum standard deviation). Based on the data from the assessment window, the effectiveness of investment portfolios was assessed using weights determined on the basis of the data from the estimation window. However, different lengths were assumed for the estimation window and weights were determined for four such windows, which were in the following date ranges:
• 01.01.2016–31.12.2017 period marked as “1617”
• 01.07.2016–31.12.2017 period marked as “16h17”
• 01.01.2017–31.12.2017 period marked as “17”
• 01.07.2017–31.12.2017 period marked as “17 h”
The assessment window was always from 01.01.2018 to 30.06.2019. As mentioned at the beginning, the work analyzed the shares of the largest companies listed on selected European stock exchanges for investments in gold and oil. Data on the analyzed investments, together with the data source, are presented in Annex A. The twenty largest companies were selected for each stock exchange for which data was available in the research period. The companies were selected based on their capitalization value at the end of the 2019 year.
The results for all designated portfolios were compared with the results for the naive strategy (all shares of each investments in portfolio are equal) achieved for the evaluation window. In total, 45,600 portfolios for the naive strategy were analyzed in the paper. For each index of 4,560 portfolios, the strategies adopted (“only traditional”; “only gold”; “only crude oil”; “gold and crude oil”) number 1,140 which is the number of all possible three-element combinations out of twenty (largest companies) because so many companies were analyzed in each market.
In the case of optimizations, the number of designated portfolios is 364,800, as there are 182,400 portfolios each for strategies with minimal risk and for strategies with maximum effectiveness. A total of 45,600 portfolios were designated, being 1,140 for each of the four strategies (due to the assumed length of the estimation window), for each of the ten markets analyzed. In total, 410,400 investment portfolios were determined in the work for portfolio theory and the naive strategy, which were the basis for the results and conclusions of the research presented below.
The analyzed markets were chosen by the following key. Nine of ten markets are classified as developed markets. The last one (Warsaw Stock Exchange) is classified as an emerging market (source: MSCI market classification https://www.msci.com/market-classification). Such a sample can also answer if the emerging market is the one with the highest alternative investment shares, and can be classified as a market with the highest differences when comparing to other analyzed markets.
The Kruskall-Wallis test was used to assess whether there were differences between the shares of alternative investments in individual markets. The set of hypotheses tested in the study was written as Aczel (Citation2000):
H0: There are no differences between the distributions of calculated shares for analyzed investments
H1: There are at least two groups of investments that do not have the same distributions for shares of analyzed investments.
It can, therefore, be concluded that the null hypothesis assumes stochastic homogeneity of the compared distributions, and the alternative hypothesis stochastic heterogeneity is found for at least two distributions Ruxton and Beauchamp (Citation2008). Adoption of an alternative hypothesis will mean that there is, for at least one test group, a tendency to accept higher (or lower) shares for analyzed alternative investment than for other groups. This can also be written as follows:
H0:
H1:
Where,
k—means the number of groups compared (10 groups were analyzed in the study),
Fj (x)—means the distribution function for the weights determined for the j-th market.
The test was carried out for the significance level of α = 0.05. All calculations were carried out in the Statistica program. The Kruskal-Wallis test statistics are calculated from the formula Chan and Walmsley (Citation1997):
Where,
H—value of statistics,
nj—size of j-th group,
Rj—the sum of ranks of the j-th group.
The above statistics have an asymptotic χ2 distribution with k-1 degrees of freedom. If the calculated value of statistics is greater than the critical value read from the tables, then the null hypothesis is rejected (this will mean that there are no differences between the distributions), in favor of the alternative hypothesis (there are at least two groups of investments that do not have such same distributions). When the value of test statistics is less than the critical value, there is no basis to reject the null hypothesis Stanisz (Citation2006).
4. Research results
shows the average efficiency for all designated portfolios with minimal risk. The data presented in are presented for each of the exchanges examined, for each strategy and for each analyzed length of the estimation window separately.
Table 1. Average value of sharpe ratios for investment portfolios with minimal risk
In addition, the figures on a black background indicate whether the average efficiency value obtained using Markowitz’s portfolio theory proved to be higher than the same value obtained for the naive strategy. The data presented are average values for all designated portfolios for the given conditions. In the case of portfolios with minimal risk it was noted that for investment strategies involving the construction of an investment portfolio consisting of gold and three stock companies, and three stock companies, gold and oil, regardless of the length of the estimation window, the use of portfolio theory obtained, on average, higher efficiency values than for a naive strategy. There was no exception here because the same regularity appeared for all the analyzed exchanges. For three-element portfolios consisting only of shares of listed companies, as well as of three shares of listed companies and investments in oil, it was no longer possible to draw such unambiguous conclusions. It turned out that portfolios containing oil were more effective than the naive strategy, and for portfolios consisting only of traditional investments, the most frequent occurrences were that the naive strategy proved to be more effective than the portfolios built using Markowitz’s theory. However, it can be seen that the longer the estimation window, the higher the efficiency and the lower the chance that the portfolios based on portfolio theory will be less effective than the naive strategy.
presents, in contrast, the average efficiency value for portfolios in which this portfolio parameter was optimized. As before, the value of efficiency is presented against a black background if it was lower than for a naive strategy. The results obtained in combination with the strategy are different for these portfolios than for the previously discussed portfolios with minimal risk. First, for the portfolios in , it can be seen that for the short estimation window, portfolio theory has proved sometimes more effective than a naive strategy. The situations where a naive strategy turned out to be more effective than portfolio theory were recorded for an estimation window length of one year and marked as “17”. For this estimation window, it has been noted six times that naive strategy proved to be more effective than portfolio theory. This happened seven times for the longest estimation window marked “1617” and ten times for the estimation window marked as 17 h, and this situation occurred as many as 23 times for the estimation window marked as 16h17. This last observation is particularly surprising because the evaluation window was symmetrical (same length) to the estimation window (16h17), which had the lowest results in terms of efficiency.
Table 2. Average value of sharpe ratios for investment portfolios with maximum efficiency
The next table shows the average shares of investments in gold and investments in oil depending on the length of the estimation window and broken down into portfolios with minimal risk and maximum efficiency.
Based on the data in , it can be concluded that in the four-element portfolios the designated share of investments in gold was much higher than the average share of investments in oil for portfolios with minimal risk. At the same time, it was not possible to indicate at least one estimation window or stock exchange for which it would be different. Importantly, the share of investment in gold also accounted for more than half of the value of designated weights in these portfolios. For maximum-efficiency portfolios, there were no such explicit conclusions; the share of investments in oil was higher than for those with minimal risk. In the case of gold, there was no such dependence, which was mainly influenced by the results from the 16h17 estimation window, for which very low values of the share of investment in gold in the designated portfolios were observed. For this estimation window, an average rate of return lower than zero was noted, which influenced such a low share of this investment in portfolios with maximum efficiency.
Table 3. Average shares of alternative investments in four-element portfolios
An increase in the share of investments in crude oil and a decrease in the share of investments in gold was also recorded in the portfolios with maximum efficiency, but in five-element portfolios. Also, in these portfolios, a high share of investments in gold was observed in portfolios with minimal risk and a low share of investments in crude oil. Looking at results presented in it is very difficult to assess if significant differences in the share of alternative investments in portfolios exist due to the stock exchanges examined.
Table 4. Average shares of alternative investments in five-element portfolios
To examine whether there were statistical differences between the shares for investments in gold and crude oil, a series of Kruskal-Wallis tests were conducted between the subject exchanges. Portfolios with minimal risk and maximum efficiency were analyzed separately. The table shows the exchanges for which there was no reason to reject the null hypothesis assuming no differences in the distribution of calculated shares for the researched investments.
Most of the assessed stock exchanges had different designated shares for the alternative investments in the optimal portfolios examined. Therefore, despite the strong links between stock markets indicated on the basis of literature studies, the determination of weights for the alternative investments studied shoul d be conducted individually for each stock exchange. Certain relationships that cause the shares of the subject alternative investments to be similar between stock exchanges are presented below.
Based on the data presented in , it can be concluded that the longer the estimation window then the greater the number of exchanges, with the fewer differences were found in the distribution of fixed weights for gold and oil. In the case of four-element portfolios, this relationship was very strong. For portfolios with minimal risk, it can be clearly stated that the longer the estimation window, the more the differences in the share of investment in gold and investment in oil between individual exchanges become blurred. With short windows, there were few estimations of such similarities between the analyzed exchanges or for the shortest estimation window they did not occur at all. For maximum performance portfolios, this relationship was also observed, but it was no longer as pronounced as for the minimum risk portfolios, as confirmed by the number of exchanges presented in . Furthermore, for maximum efficiency portfolios, there were on average four times as many exchanges for which statistically significant differences were observed for the designated weights than in the case of portfolios with minimal risk.
Table 5. Results of the Kruskal-Wallis test of the stock exchange pair for which the null hypothesis was not rejected
A similar relationship also exists for five-element portfolios. In this case, it was also noted that there were more exchanges for maximum efficiency portfolios for which there were no significant differences in the determined distribution of investment shares in gold and silver than in the case of portfolios with minimal risk. For these portfolios, however, the strong relationship between the longer estimation window and the increasing number of exchanges for which there were no differences in the analyzed distributions cannot be confirmed. For portfolios with minimal risk, this number is fixed, and the length of the estimation window has no clear impact on it. However, for portfolios with maximum efficiency, it can be stated that the longer the estimation window, the more exchanges for which there are no differences in the distribution of shares for the examined alternative investments, however, the strength of this relationship is weaker than in the case of the four-element portfolios discussed earlier.
5. Conclusions
Based on the research, it can be concluded that the use of Markowitz’s portfolio theory produces better investment results than using a naive strategy. At the same time, portfolio theory gives better results for portfolios with a risk minimization investment objective than for portfolios with maximum efficiency. Although, also for this second investment goal, it can be stated that portfolio theory enables obtaining better results than using a naive strategy. In addition, the extension of investment portfolios to gold or gold and oil allowed for higher efficiency than for portfolios without alternative investments or for portfolios with oil only. It was also confirmed that, on average, for all the exchanges examined, the longer the estimation window, the higher the efficiency of designated portfolios with lower risk.
With reference to the adopted research hypothesis, it cannot be unequivocally answered whether it is proved. It transpired that the longer the estimation window, the more exchanges have similar shares in investments in gold or investments in oil. For shorter windows, the estimations of similarities in the shares of these alternative investments are declining. The research also shows that the construction of portfolios with maximum efficiency causes an increase in the number of exchanges for which the shares of investments in gold or oil will be devoid of statistically significant differences. On the other hand, the design of portfolios with minimal risk limits the occurrence of similarities in the distribution of investment shares in gold and oil between the exchanges examined. Designation of portfolios with maximum efficiency will, therefore, result in alternative investment shares being closer to each other regardless of the exchange concerned. Additionally, the determination of portfolios with minimal risk leads to statistically significant differences in the designated shares for the analyzed investments in gold and oil. The effects described above were stronger for four-element portfolios than for five-element portfolios.
Despite the strong connection between capital markets, the research indicates that in the construction of the investment portfolio between various national stock exchanges there are significantly statistical differences for the shares of such investments as gold or oil. An investor from the German stock exchange should set a gold share at a different level than an investor from Polish, Portugese and French exchanges. What is also surprising is the fact that the alternative investments examined constitute a significant part of the designated investment portfolios. An investment in gold accounts for four-element portfolios with a minimum risk of over 60% of its value. Oil investment in the same portfolios has an average share of 28%. In the case of portfolios with maximum efficiency, the share of investments in crude oil is about 48% and 43% for investments in gold. Similar relationships, i.e. a greater share of gold in portfolios with minimal risk and smaller share of oil, also occur in portfolios with maximum efficiency in five-element portfolios. Such results indicate that shares of alternative investments can be the main part of an investment portfolio not only the supplement of it. What is more, so high weights of alternative investments point that those investments have better investment characteristics (rate of return, risk) than analyzed in this article traditional investment. These high shares can be the basis for further research.
Additional information
Funding
Notes on contributors
Marcin Potrykus
A graduate of the Faculty of Management and Economics at the Gdańsk University of Technology. From 2008 employee at GUT. In 2015 received a PhD degree. His scientific interests mainly concern the construction of the investment portfolio using not only traditional investments but also less known alternative investments. Member of the Polish Finance and Banking Association since 2013. Organizator of the conference “The First Million” together with Kronenberg Foundation. Member of the Competition Committee in the T.I.M.E.S. Supervisor for the team in the CFA Research Challenge. Mentor in the “School of Eagles” program of Ministry of Science and Higher Education.
References
- Aczel, A. D. (2000). Statystyka w zarządzaniu. PWN.
- Anand, R., & Madhogaria, S. (2012). Is gold a ‘safe-haven’? - An econometric analysis. Procedia Economics and Finance, 1(12), 24–19. https://doi.org/10.1016/S2212-5671(12)00005-6
- Baur, D. G., & Lucey, B. M. (2010). Is gold a hedge or a safe haven? An analysis of stocks, bonds and gold. The Financial Review, 45(2), 217–229. https://doi.org/10.1111/j.1540-6288.2010.00244.x
- Baur, D. G., & McDermott, T. K. (2010). Is gold a safe haven? International evidence. Journal of Banking and Finance, 34(8), 1886–1898. https://doi.org/10.1016/j.jbankfin.2009.12.008
- Chan, Y., & Walmsley, R. (1997). Learning and understanding the Kruskal-Wallis one-way analysis-of-variance-by-ranks test for differences among three or more independent groups. Physical Therapy, 77(12), 1755–1761. https://doi.org/10.1093/ptj/77.12.1755
- Ciner, C., Gurdgiev, C., & Lucey, B. M. (2013). Hedges and safe havens: An examination of stocks, bonds, gold, oil and exchange rates. International Review of Financial Analysis, 29(C), 202–211. https://doi.org/10.1016/j.irfa.2012.12.001
- Das, S., Demirer, R., Gupta, R., & Mangisa, S. (2019). The effect of global crises on stock market correlations: Evidence from scalar regressions via functional data analysis. Structural Change and Economic Dynamics, 50(C), 132–147. https://doi.org/10.1016/j.strueco.2019.05.007
- Dębski, W. (2007). Rynek finansowy i jego mechanizmy. Podstawy teorii i praktyki. PWN.
- Gjika, D., & Horváth, R. (2013). Stock market comovements in Central Europe: Evidence from the asymmetric DCC model. Economic Modelling, 33(C), 55–64. https://doi.org/10.1016/j.econmod.2013.03.015
- Jajuga, K., & Jajuga, T. (2006). Inwestycje. Instrumenty finansowe, aktywa niefinansowe, ryzyko finansowe, inżynieria finansowa. PWN.
- Majewska, E., & Olbryś, J. (2017). The evolution of financial integration on selected european stock markets: A dynamic principal component approach. Comparative Economic Research, 20(4), 45–63. https://czasopisma.uni.lodz.pl/CER/article/view/2591
- Markowitz, H. (1952). Portfolio selection. The Journal of Finance, 7(1), 77–91. https://doi.org/10.2307/2975974
- Potrykus, M. (2015). Investment in gold − safe haven, hedge or source of diversification for polish investor. Nauki O Finansach = Financial Sciences, 3(24), 193–207. https://www.dbc.wroc.pl/dlibra/publication/36341/edition/32727
- Ruxton, G. D., & Beauchamp, G. (2008). Some suggestions about appropriate use of the Kruskal-Wallis test. Animal Behaviour, 76(3), 1083–1087. https://doi.org/10.1016/j.anbehav.2008.04.011
- Saeedian, M., Jamali, T., Kamali, M. Z., Bayani, H., Yasseri, T., & Jafari, G. R. (2019). Emergence of world-stock-market network. Physica A: Statistical Mechanics and Its Applications, 526(15 July 2019), 120792. https://doi.org/10.1016/j.physa.2019.04.028
- Sharpe, W. F. (1994). The sharpe ratio. The Journal of Portfolio Management, 21(1), 49–58. https://doi.org/10.3905/jpm.1994.409501
- Śmiech, S., & Papież, M. (2017). In search of hedges and safe havens: Revisiting the relations between gold and oil in the rolling regression framework. Finance Research Letters, 20(C), 238–244. https://doi.org/10.1016/j.frl.2016.10.006
- Šoja, T. (2019). Gold in investment portfolio from perspective of European investor. The European Journal of Applied Economics, 16(1), 41–58. https://doi.org/10.5937/ejae15-19652
- Stanisz, A. (2006). Przystępny kurs statystyki z zastosowaniem STATISTICA PL na przykładach z medycyny. StatSoft.
- Yang, C., Chen, Y., Niu, L., & Li, Q. (2014). Cointegration analysis and influence rank - A network approach to global stock markets. Physica A: Statistical Mechanics and Its Applications, 400(15 April 2014), 168–185. https://doi.org/10.1016/j.physa.2014.01.011
ANNEX A
Table 1. Characteristics of data sources