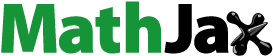
Abstract
The letter examines herding in the most liquid cryptocurrency markets relative to traditional financial markets of 10 emerging economies within the G20. Our results reference significant symmetric crowd and imitation trading, which are dependent on time. Additionally, we report asymmetric herd behaviour in the cryptocurrency and stock markets, indicative that traders of these markets react collectively to extreme return movement with implied high risk and consequences for market informational efficiency.
Keywords:
PUBLIC INTEREST STATEMENT
Crowd trading without recourse to market fundamentals has the potential to usher in high volatility and increase market risk, which may lead to huge investment losses. This letter examines the existence (or otherwise) of herding in the cryptocurrency market and compares such irrational (rational) behaviour to conventional assets of emerging market economies. Evidence of time-dependent herding is detected, with asymmetric herd trading being more pronounced in the cryptocurrency and emerging stock markets.
1. Introduction
Do financial asset investors simply imitate each other's trading strategies or merely follow the crowd during market stress or periods of extreme downturns without recourse to fundamentals?1 Admittedly, these behavioural finance characteristics have received enormous attention, yet until the work of Bouri et al., (Citation2018), the literature was silent on the phenomenon in the new global digital market, cryptocurrency, which has courted unbridled widespread interest mainly due to its price development and prospect for high returns. Nonetheless, a handful of empirical studies on herding behaviour in cryptocurrencies are now gaining gradual momentum in the literature. The works by Calderón (Citation2018), Da Gama Silva et al. (Citation2019), Kaiser and Stöckl (Citation2020), Kallinterakis and Wang (Citation2019), and Stavroyiannis and Babalos (Citation2019) Vidal-Tomás et al. (Citation2018), document different degrees of herding in the new financial market.
Bouri et al., (Citation2018) detected significant herding with an OLS-based rolling-window approach in 14 major cryptocurrencies. Prior to their detection, the authors found no evidence of herding using a static version of the model. Vidal-Tomás et al. (Citation2018) sampled 65 cryptocurrencies and sub-divided them into 5 major and 60 smallest currencies. Using standard testing methods, the authors concluded that “the smallest digital currencies are herding with the largest ones; thus, traders base their decisions on the performance of the main cryptocurrencies.” In another study, Calderón (Citation2018) identified the presence of herding over multiple switching regimes. Meanwhile, a significant herd effect was found missing for the full-sample under a static model. Employing adaptions of the cross-sectional standard deviation (CSSD) and the cross-sectional absolute deviation (CSAD) with Hwang and Salmon’s (Hwang & Salmon, Citation2004) approaches, Da Gama Silva et al. (Citation2019) assessed herding behaviour in 50 cryptocurrencies and found significant herding during down-market periods (with the CSSD); weak herd effect (with the CSAD); and extreme period of adverse herding with the state-space model. With or without incorporating Bitcoin into the sample of cryptocurrencies,Kallinterakis and Wang (Citation2019) reported significant herding effects that happened to be stronger during up-markets, and for days of high volume and low volatility. Elsewhere, Stavroyiannis and Babalos (Citation2019) employed the standard CSSD and CSAD with static models to report herding in 8 largest cryptocurrencies, but later found the absence of such effect using much robust time-varying models. Accounting for potential sampling bias, Kaiser and Stöckl (Citation2020) provided evidence of significant herding in the cryptocurrency market. Coskun et al. (Citation2020) found anti-herding behaviour in the cryptocurrency market using a battery of econometric approaches (i.e., the CSAD, OLS, GARCH, and a time-varying Markov-switching model) under varying sample periods; however, they reported no significant asymmetric behaviour during the “up and down” market periods. Quite differently, Philippas et al. (Citation2020) revealed substantial asymmetries with respect to the intensity of herding in the cryptocurrency market, which the authors claimed to have stemmed from exogenous influences. In their findings on 20 mega-size cryptocurrencies, Amirat and Alwafi (Citation2020), initially detected no herding behaviour in the static CSAD method, but later found significant herding characteristics when a rolling-window approach was employed. Similarly, Youssef (Citation2020) used a static and time-varying version of the CSAD to document, respectively, anti-herding and herding behaviour in the cryptocurrency market, around periods from April 2013 to November 2019.
To provide fresh insight, our study augments the debate by analyzing herding behaviour in the most liquid cryptocurrencies, relative to giant emerging equities and bilateral fiat currencies. Do actors of the cryptocurrency market behave similarly (or otherwise) to those of established emerging economies markets? Although both markets are often described as relatively highly volatile, our study attempts to unlock the puzzle of whether they share (un)common behavioural characteristics.
More so, it is argued that cryptocurrencies are fast becoming a globally accepted medium of exchange (Polasik et al., Citation2015) and a contender for traditional currencies. This viewpoint is boosted perhaps by a report by Barontini and Holden (Citation2019), which claims plans are far-advanced for the issuance of central bank digital currencies in the short or medium term.2 Moreover, with its low transactional cost stemming from non-involvement of financial intermediaries, lack of inflation, absence of bilateral base-currency effect, and the prospects of being adopted as means of money transfers, either internally or via remittances abroad, especially by financial donors, a revolutionary economy of digital currency holds relevance for emerging economies. However, to date, investors and regulators alike are uncertain of the kind of asset that features digital currencies. Therefore, in the lens of behavioural finance, this study contributes to the narrative by comparatively examining behavioural market characteristics, with key emphasis on herding in cryptocurrencies and diverse emerging market assets using OLS- and quantile-based CSAD regression under varying time-samples, including rolling-window estimations. Through such an empirical behavioural lens, could cryptocurrencies be designated in emerging market economies as part of securities (like equities) or monetary assets? Answers to these questions mark the contribution of our letter.
Section 2 describes the data and the empirical methods employed. Sections 3 and 4 respectively discuss the results and conclusion.
2. Empirical methodology
2.1. Data description
After initially considering a universe of 2,092 digital currencies, we filtered 8 most liquid markets (Bitcoin, Ethereum, Ripple, Litecoin, Dash, Ethereum Classic, NEO, and Zcash)3 representing 76.57% of the USD 133 billion combined market value using a three-stage criterion: (i) currencies with market cap above USD 100 million; (ii) markets with trading volumes above the third-quartile volume; and (iii) those with more than 24 months of active trading and reliable data.4 We also considered stocks (BOVESPA, MOEX, NIFTY50, SSE50, FTSE/JSE TOP40, BIST30, KOSPI200, MEXBOL, Indonesian-JCI, and MERVAL) and bilateral currencies (FXs) of emerging economies within the G20,5 except for Saudi Arabia, which we ruled-out for purposes of inconsistency.6 For uniformity, all market variables span 29.10.2016–07.06.20197, sourced in US dollars, and cleaned to synchronize with each other.
2.2. Testing for herding
To measure return dispersion, we employ the cross-sectional absolute deviation (CSAD) approach of Chang et al. (Citation2000):
where denotes the returns of an asset I at time t;
represents the cross-sectional returns average of the asset market portfolio at time t, and N gives the count of asset returns in the portfolio. To allow for a possible non-linear relationship between
and
under periods of market stress, we apply the standard model of Chang et al. (Citation2000):
Aside from the cap-weighted market returns strategy (Bouri et al., Citation2018; Kaiser & Stöckl, Citation2020), we consider the equally weighted:
as in Vidal-Tomás et al. (Citation2018) and portfolio construction based on the global minimum-variance (GMV). Drawing from our selected portfolio of risky assets, the GMV minimizes the variance of the daily returns in an objective function stated as:
Here, denote N × 1 vector of weights, where the optimal portfolio weights are expressed as
. The rationale is to examine the sensitivity of our detection regarding portfolio construction strategies and weight effects. Appendices A.1-A.2 display summary measures of
and
for the aggregate sample, as well as different sub-sample periods of the equally weighted and GMV-based portfolios. Noticeably, the time measures of the former are slightly higher than the latter, and comparatively, the FX market returns recorded the lowest values.
As robustness to the static Least Squares (OLS) estimations for (2), we analyze herding at different quantile distributions.8 Owing to our small time-samples,9 we designate up- and down-markets with the 25th and 75th quantiles and compare the conditional-median QR to the OLS results. Although not new, QR has rarely been applied to quantify herding in cryptocurrencies. The work by Stavroyiannis and Babalos (Citation2019) is however noted, yet the fitted QR largely accounted for herding behaviour in the aggregate time-sample.
To further incorporate the possibility of realizing non-stable regression estimates over moving time-intervals, we augment our herding analysis with a time-varying version of the OLS,10 using a rolling-window of size 80.11 As a rule of thumb, herd trading is absent if the boundary conditions and
are satisfied. Else, we observe anti-herding (herding) if
(
) has a positive (negative) and statistically significant coefficient.
RSE: residual standard error; and AIC: Akaike information criterion. Statistical significance: “*”1%; “**”5%; “***”10%.
3. Results
Of interest to us is the presence of herd trading. display the regression results. From the tables, the estimated coefficient of the OLS is negative for all three asset classes but appears significant only for the stock under the full-sample. Except for the cryptocurrency, the QR estimations report negative and nonsignificant
for the aggregate sample. However, under the sub-sample estimations, the OLS detected a significant herding behaviour for the cryptocurrency and stock markets over 29.10.2016–30.06.2017; the FX and stock for 01.03.2018–31.10.2018; and all asset classes over 01.11.2018–07.06.2019. From the QR results, significant herding is recorded for the cryptocurrencies and stocks over the period 29.10.2016–30.06.2017; and for the stocks at 01.03.2018–31.10.2018. The crowd trading in the emerging FX and stock markets around the 2018s and onwards perhaps could have been influenced by the US–China trade war. The heightened uncertainty that characterised emerging markets during such periods could reasonably inform the trading decisions of inexperienced investors, who as a result might have merely followed the experienced crowd to avoid huge investment losses. Similarly, the 2018 cryptocurrency crash may consequentially account for the herd trade in the digital market between 2018 and 2019. This presupposes that actors of the asset classes react collectively to adverse events.
Table 1. OLS estimates
Table 2. QR estimates
Notably, the slight difference between the OLS and QR results may be attributed perhaps to the location measure used for the return dispersion relationships. As also observed by Calderón (Citation2018) and Da Gama Silva et al. (Citation2019), the high variation patterns may have shredded evidence of herding behaviour in the full-sample, only to be unveiled in the sub-samples, and further corroborated by the rolling-window estimations (refer, Appendix A.3). Based on the rolling-window t-statistics, we could observe significant time-varying herd trading which manifests strongly in the stock market. Although the cryptocurrencies witnessed a lengthy period of anti-herding, traces of herding activity mark periods from 19.10.2017 to 20.02.2018, 02.10.2018–12.11.2018, and 13.11.2018–17.01.2019, of which the herd effect of the former two periods appears somehow weak and nonsignificant.12 In addition, the duration of significant herding in the FX market lasted for fewer days but varied with time.
This behavioural evidence implies that investment decisions are not solely based on individual priors. Meaning, traders imitate each other or merely rally behind the market consensus at different periods. More so, the herd in the fast-embryonic cryptocurrency and the emerging markets is not overly puzzling, mainly because of the low intensity of informational flow, frequent price variations, the rising expectation for higher returns, and the existence of amateur investors, and perhaps adverse economic events. Furthermore, Bouri et al., (Citation2018) found economic policy uncertainty to be among the driving factors of the herd effect in the cryptocurrency market.
Considering periods of up- and down-market, we observe evidence of significant herding behaviour at quantile 0.75 for the cryptocurrencies and stocks over 29.10.2016–30.06.2017; while at the same period, the stocks recorded a significant value of −0.090 under quantile 0.25. is found to be significant for the stock market at quantile 0.75 over the periods 01.03.2018–31.10.2018 and 01.11.2018–07.06.2019. The absence of significant asymmetric crowd trading in the work by Coskun et al. (Citation2020) who investigated herding in leading cryptocurrencies with data from 28.04.2013 to 14.11.2018 is revealed in our sub-sample analysis. The difference between the two results could largely be attributed to the time-sampled data used for the empirical analysis, and perhaps the associated economic events that mark such periods.
Interestingly, in our analysis, the symmetric and asymmetric herding behaviour of the cryptocurrency market coincides with the 2017 price surge and the declaration of the currency by the Japanese Payment Services Act as legal tender.13 This indicates that investors of the market do not only herd during down-market, or more so, exclusively respond to bad news (as found in our earlier findings and also by Vidal-Tomás et al., Citation2018), but besides, they react to good news. Naïve and ill-informed traders who imitate experienced colleagues, as well as others who fear of missing out on prospective higher returns may mostly trigger such reactions.
4. Conclusion
Highlights of the above analysis are provided. (i) Significant herding looks more pronounced in the emerging stock and cryptocurrency markets, especially when considered under the GMV; (ii) Like Bouri et al., (Citation2018) and others, evidence of time-dependent herding is documented, signalling the rationality of investors of these markets in-terms of making trading decisions over-time; (iii) Owing to the absence of significant herding behaviour, the rational asset-pricing model is best used to explain the extreme price variations at the tail distribution of the FX market; and (iv) Comparatively, the behavioural characteristics of the cryptocurrency market are qualitatively less distinctive from that of the stocks. From a behavioural perspective, we could interpret this finding to mean that the new currency, for now, evolves more than an investment asset to market actors instead of a bona fide currency. Therefore, any attempt to design a regulatory framework aimed at widely embracing the new asset into mainstream financial markets of emerging economies should incorporate its evolving featured characteristics.
In summing up, we surmise that herd trading does not just increase investors’ risk but in addition, renders the markets inefficient and reduces the possibility of diversification benefits since individual traders downplay their unique trading strategies to follow other colleagues or herd on the prevailing market performance. This phenomenon fuels speculative trading that culminate to heighten market uncertainty and instability. Potential common internal and/or external drivers that induce herding in these asset markets remain a subject for future research.
Declaration
The authors declare no conflict of interest. All deductions and conclusions are the views of authors and do not in any way represent the standpoint of their affiliations.
Brief profile
Anna and Maurice are final year doctoral students at Wits Business School (WBS). Prior to enrolling with the School, Anna has been working with the Central Bank of Tanzania as a senior financial analyst, while Maurice is an Assistant Lecturer in Statistics. Anna’s main research interest is in areas of financial markets and institutions. Maurice has been publishing articles in several aspects of Statistical Finance.
Imhotep is the grand editor of a conglomerate of journals and a professor of finance at Wits Business School. George is a senior academic at the same school.
Acknowledgements
The authors remain grateful to the two anonymous referees for their valuable comments and criticisms, and to the editorial team. Open Access funding provided by the Qatar National Library.
Additional information
Funding
Notes on contributors
Imhotep Paul Alagidede
Imhotep is a grand editor of a conglomerate of journals and a professor of finance at Wits Business School. George is a senior academic of the same School.

Anna Gustav Lyimo
Anna and Maurice are final year doctoral students at Wits Business School (WBS). Prior to enrolling with the School, Anna has been working with the Central Bank of Tanzania as a senior financial analyst, while Maurice is an Assistant Lecturer in Statistics. Anna’s main research interest is in areas of financial markets and institution. Maurice has been publishing articles in several aspects of Statistical Finance.
References
- Amirat, A., & Alwafi, W. (2020). Does herding behavior exist in cryptocurrency market? Cogent Economics & Finance, 8(1), 1735680. https://doi.org/10.1080/23322039 2020.1735680
- Barontini, C., & Holden, H., 2019. Proceeding with Caution-A Survey on Central Bank Digital Currency. Proceeding with Caution-A Survey on Central Bank Digital Currency. BIS Paper, No.101.
- Bouri, E., Gupta, R., & Roubaud, D. (2018). Herding behaviour in cryptocurrencies. Finance Research Letters, 29, 216–14. https://doi.org/10.1016/j.frl.2018.07.008
- Calderón, P. O., 2018. Herding behavior in cryptocurrency markets. https://arxiv.org/pdf/1806.11348.pdf
- Chang, E. C., Cheng, J. W., & Khorana, A. (2000). An examination of herd behavior in equity markets: An international perspective. Journal of Banking and Finance, 24(10), 1651–1679. http://dx.doi.org/10.1016/s0378-4266(99)00096-5
- Christie, W. G., & Huang, R. D. (1995). Following the Pied Piper: Do individual returns herd around the market? Financial Analysts Journal, 51(4), 31–37. https://doi.org/10.2469/faj.v51.n4.1918
- Coskun, E. A., Lau, C. K. M., & Kahyaoglu, H. (2020). Uncertainty and herding behavior: Evidence from cryptocurrencies. Research in International Business & Finance, 54, 101284. https://doi.org/10.1016/j.ribaf.2020.101284
- Da Gama Silva, P. V. J., Klotzle, M. C., Pinto, A. C. F., & Gomes, L. L. (2019). Herding behavior and contagion in the cryptocurrency market. Journal of Behavioral and Experimental Finance, 22, 41–50. https://doi.org/10.1016/j.jbef.2019.01.006
- Hwang, S., & Salmon, M. (2004). Market stress and herding. Journal of Empirical Finance, 11(4), 585–616. https://doi.org/10.1016/j.jempfin.2004.04.003
- Kaiser, L., & Stöckl, S. (2020). Cryptocurrencies: Herding and the transfer currency. Finance Research Letters, 33, 101214. https://doi.org/10.1016/j.frl.2019.06.012
- Kallinterakis, V., & Wang, Y. (2019). Do investors herd in cryptocurrencies–and why? Research in International Business and Finance, 50, 240–245. https://doi.org/10.1016/j.ribaf.2019.05.005
- Philippas, D., Philippas, N., Tziogkidis, P., & Rjiba, H. (2020). Signal-herding in cryptocurrencies. Journal of International Financial Markets, Institutions and Money, 65, 101191. https://doi.org/10.1016/j.intfin.2020.101191
- Polasik, M., Piotrowska, A. I., Wisniewski, T. P., Kotkowski, R., & Lightfoot, G. (2015). Price fluctuations and the use of bitcoin: An empirical inquiry. International Journal of Electronic Commerce, 20(1), 9–49. https://doi.org/10.1080/10864415.2016.1061413
- Stavroyiannis, S., & Babalos, V. (2017). Herding, faith-based investments and the global financial crisis: Empirical evidence from static and dynamic models. Journal of Behavioral Finance, 18(4), 478–489. https://doi.org/10.1080/15427560.2017.1365366
- Stavroyiannis, S., & Babalos, V. (2019). Herding behavior in cryptocurrencies revisited: Novel evidence from a TVP model. Journal of Behavioral and Experimental Finance, 22, 57–63. https://doi.org/10.1016/j.jbef.2019.02.007
- Su, Y., & Hwang, J. S. (2009). A two-phase approach to estimating time-varying parameters in the capital asset pricing model. Journal of Applied Statistics, 36(1), 79–89. https://doi.org/10.1080/02664760802443871
- Vidal-Tomás, D., Ibáñez, A. M., & Farinós, J. E. (2018). Herding in the cryptocurrency market: CSSD and CSAD approaches. Finance Research Letters (in press). https://doi.org/10.1016/j.frl.2018.09.008
- Youssef, M. (2020). What drives herding behavior in the cryptocurrency market? Journal of Behavioral Finance, 1–10. 1867142. https://doi.org/10.1080/15427560.2020
Appendix
Table 1. Descriptive measures for
Table 2. Descriptive measures for