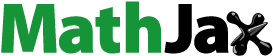
Abstract
This paper analyzes the socio-economic correlates of multiple child deprivations in Kenya using the 1993–2014 KDHS. Conventionally, child well-being is examined from the perspective of income poverty. However, numerous studies have argued that income is just one of the defining characteristics of poverty. Furthermore, examining child well-being only through monetary lens risk leaving behind millions of children because children are not economic agents. Despite global recognition of the importance of having a comprehensive measure of child well-being, there is a dearth of literature on multiple child deprivations. This study utilized data on seven child-specific dimensions of well-being. First, we counted the number of dimensions each child was deprived and then ranked them from those who were not deprived to those who are deprived in four or more dimensions. Second, we examined the distribution of multiple child deprivations by various characteristics. Finally, we investigated factors associated with multiple child deprivations using an ordered logit regression model. The results reveal that rural children, male children and children living in households without access to electricity and under female headship, on average, suffer higher incidence of multiple deprivations than their counterparts. Children with higher birth orders, twins and from rural residence had higher probability of suffering multiple deprivations while higher maternal education, employment of the respondent were associated with lower chances of a child suffering multiple deprivations. The findings of the study suggest the need for integrated policies targeting multiple forms of deprivation and strategies to ensure access to public services in remote areas.
PUBLIC INTEREST STATEMENT
It is acknowledged that well-being is a multidimensional phenomenon yet it has been commonly assessed using income or consumption and expenditure measures. There are a number of drawbacks associated with income measures of well-being especially in a developing country like Kenya with many data issues. Further, the income measures of well-being have not been disaggregated due to age masking the well-being of children. In this study, we assess child deprivations using seven child-specific dimensions namely: nutrition, health, education, shelter, water, sanitation and information. Deprivations experienced by children are disheartening as it may last forever. In order to develop effective policies to reduce child deprivations, it is important to understand the multiple attributes of child development and their causes and consequences. The study affirms that interventions that alter early life trajectories are effective ways to promote child well-being across their life span.
1. Introduction
Child deprivation has received significant attention in the literature since the beginning of the millennium (see for instance, Bastos et al., Citation2004; Gordon et al., Citation2003; UNICEF, Citation2004). This is because child deprivation is acknowledged to be a major contributor to adult poverty and is associated with vulnerabilities such as disability, stunting, early death and waste of human capital (Cockburn & Kabubo-Mariara, Citation2010; Minujin, Citation2012; A. Minujin & Delamonica, Citation2005). This issue is still relevant today in a developing country like Kenya.
Another concern in the literature is to do with determinants of poverty in an attempt to formulate policies to reduce deprivations. For instance, the correlates of poverty are grouped under characteristics like individual (age, sex); household (size of household, education of household head); and community (infrastructural services and location (urban-rural, region)). This approach involves a regression analysis of the various measures of poverty on these characteristics as independent indicators (Achia et al., Citation2010; Y. M Batana et al., Citation2014). However, different measures of poverty may produce silo policies which will not achieve much since poverty is a multidimensional phenomenon in which the dimensions are interlinked (Alkire et al., Citation2021).
Previous studies on poverty in Kenya have analysed well-being using only one dimension (i.e., household income or consumption expenditures) yet it is widely acknowledged that well-being is a multidimensional phenomenon (see Geda et al., Citation2005; Mwabu et al., Citation2000; Oyugi, Citation2000). Using income or consumption expenditures as a measure of well-being has a number of drawbacks (Lanau et al., Citation2020). First, there is an assumption of equal distribution of income to all members of a household but this is not always the case. Second, adjusting the consumption of children using equivalent scales is subjective and arbitrary (Thorbecke, Citation2008). Child poverty is different from adult poverty with children experiencing poverty as a deeply physical, social, and emotional phenomenon (Minujin, Citation2012). In developing countries, measurements of income tend to disregard children’s special needs and hence do not fully capture their experience of poverty (Gordon et al., Citation2003).
In an attempt to circumvent the drawbacks identified in the foregoing discussion, a number of studies have focused on alternative indicators of well-being. Two studies in Kenya focus on child deprivation, albeit taking a unidimensional approach. Mutunga (Citation2007) used the 2003 wave of Kenya Demographic and Health Survey (KDHS), while Kabubo-Mariara et al. (Citation2009) used the 1993–2008 waves to examine determinants of child mortality and nutritional status, respectively. There is consensus that the measurement of child well-being should use multidimensional approaches and, as much as possible, child-specific attributes (De Neubourg et al., Citation2012). However, such empirical evidence of child well-being is still scarce in Kenya (Kenya National Bureau of Statistics, Citation2017).
One outstanding development in the study of well-being is reconceptualization and reformulation of the term so as to cover broader contexts accompanied by the expansion of dimensions to include the use of non-income indicators (Atkinson, Citation2019). Sen (Citation1985) had proposed measurement of deprivation in aspects such as health, education, housing conditions, access to safe drinking water, sanitation and access to information. This view of well-being leads to re-examining well-being in terms of deprivation to guide policy design, improve targeting, increase government allocation and accountability. Understanding child well-being in this perspective is critical to promote well-being over the life course. This study contributes to the growing perspective that child well-being is multidimensional. Two research questions are addressed; what is the distribution of multiple child deprivations in Kenya? What are the key factors associated with risk of multiple child deprivations in Kenya?
Therefore, this study takes the multidimensional approach to analyse child deprivations in nutrition, health, education, shelter, water, sanitation and information dimensions. The objectives of the paper are threefold: first, we construct a multiple-deprivation indicator; two, analyze the distribution of multiple child deprivations and third, investigate the main factors that are associated with multiple child deprivations in Kenya. We use the 1993–2014 waves of KDHS data.
The rest of this paper is organized as follows. Section 2 presents a description of child deprivations in Kenya. Section 3 reviews the relevant theoretical and empirical literature while section 4 discusses the methodology. Section 5 discusses the results, while section 6 concludes and draws policy implications.
2. Child deprivations in Kenya
Kenya is still a young and developing nation. The proportion of population below 18 years was 54% in the 2009 Kenya Housing and Population Census (KPHS) which declined to 50% in the 2019 KPHS (Kenya National Bureau of Statistics, Citation2019). This shows clearly that the future of Kenya lies with the children of today. By 2030, when the Vision 2030 envisages a middle-income economy, children of today will be the ones steering the economy. There is, therefore, huge potential of reaping the fruits of demographic dividend given that most Kenyan population comprises young people.
In the year 2014, under-five mortality rate in Kenya was 52 deaths per 1000 live births, while infant mortality was 39 deaths per 1000 live births (Kenya National Bureau of Statistics and IFC Macro, Citation2015). World Bank projections indicate that under-five mortality declined to 43.2 deaths per 1000 live births, while infant mortality declined to 31.9 deaths per 1000 live births in 2019 (World Bank, Citation2020). When compared with Sustainable Development Goals (SDG) target of 25 deaths per 1000 live births for under-five mortality rate by 2030, these rates are high and of great concern (UNGA, Citation2015).
The percentage of fully immunized children increased marginally from 71% in 2008 to 72% in 2014. However, the coverage of basic vaccination of children declined from 77% in 2008 to 71% in 2014 posing serious challenges for child well-being (Kenya National Bureau of Statistics and IFC Macro, Citation2015). Donfouet et al. (Citation2019), examine inequalities in immunization coverage in Kenya among 1–2 year olds. The results indicate coverage in favour of richest wealth quintile and women possessing secondary education or higher households in Kenya.
Malnutrition places children at high risk of mortality and has also been linked to impaired mental development. Data from the 2014 KDHS shows that between 2008 and 2014, there was an improvement in nutritional status of children with the rate of stunting decreasing from 35% in 2008 to 26% in 2014; wasting declined from 7% in 2008 to 4% in 2014, and the proportion of underweight children declining from 16% in 2008 to 11% in 2014. Malnutrition and micronutrient deficiency disorders still persist with declining or stagnated nutritional status of children, especially in food insecure areas, exacerbating other dimensions like health and education.
Although child mortality and malnutrition rates have declined, there is a likelihood of these outcomes to worsen given the effects of Corona virus (COVID-19) pandemic currently affecting the whole world. The pandemic has affected the food and nutrition and provision of health services, which were already a problem before COVID-19 (Alkire et al., Citation2020). Deprivations in terms of unsafe drinking water, nutrition, and poor housing conditions predict a high risk from COVID-19 in terms of hygiene, weakened immune systems, and respiratory conditions (Alkire et al., Citation2020; WHO, Citation2017, Citation2018, Citation2019). This disastrous pandemic is a wake-up call to policymakers to address poverty in all its forms and especially to the most vulnerable people in our society. The COVID-19 pandemic is devastatingly worsening the multiple deprivations the children are facing.
Child deprivations are a problem to a developing country like Kenya because they have detrimental outcomes. Early childhood is fundamental to growth and instrumental for a child’s life course. During this period, the foundation for life and development is laid. This period is key for the development of cognitive and educational capabilities, mental and emotional health, which are important in their future adult life (A. Minujin & Delamonica, Citation2005). Some form of deprivations may lead to death and/or disability; for example, if a child is not immunized against deadly diseases such as polio, TB or measles, the child may become disabled or die early.
The effects of child deprivations can last over the lifetime as deprived children become poor adults and consequently transfer poverty to their children, thus exacerbating poverty in a society and in turn jeopardizes poverty reduction efforts by governments (Corak, Citation2006). UNICEF have advocated that the effective strategy of poverty eradication in a society is by tackling deprivation among children (UNICEF, Citation2004), while Vandemoortele (Citation2012) pointed out that equity in a society begins with children.
3. Literature
3.1. Theoretical literature
Poverty is both a complex and ambiguous phenomenon whose measurement has puzzled researchers since the beginning of the nineteenth century. Historically, the measurement of poverty was established around the so-called absolute/monetary measures. This approach, which is still in use to date, was pioneered by Booth and Rowntree in London and York in the late nineteenth and early twentieth centuries, respectively (Laderchi et al., Citation2003). However, the approach has attracted heavy criticism for using measures which relate to a means to achieve an end rather than being an end in itself (Sen, Citation1985). Additionally, these measures do not capture the intra-household distribution of income and this can be substantial (Thorbecke, Citation2008). In view of the stated drawbacks, Deaton (Citation1997) sought to develop a measurement approach by exploiting survey data, especially on consumption and expenditure to measure poverty. The author argues that poverty is better measured by consumption and expenditure, which approximates welfare more closely than income per se. Even then, consumption and expenditure measures of poverty have shown considerable fluctuations among the people (Hulme & Mckay, Citation2008).
In the last quarter of the twentieth century, Townsend (Citation1979) proposed the conceptualization of poverty in terms of relative deprivation as an alternative to the absolute poverty approach. This definition paved way for the multidimensional approach to the measurement of poverty.
Townsend (Citation1987, p. 125) defines deprivation as “a state of observable and demonstrable disadvantage relative to the local community or the wider society or nation to which an individual, family or group belongs.” Deprivation, thus, refers to the material and social conditions that are experienced by individuals and households, where these conditions are inadequate relative to what is usually available or experienced in society
The “relative approach” has been criticized prominently by Amartya Sen, who, in spite of acknowledging its importance, also maintains support for the recognition of an irreducible absolutist core in the idea of poverty (Sen, Citation1983, p. 159; Hick, Citation2012). This measurement approach is known in the literature as a capability framework. In the framework, poverty is defined as deprivation or failure to achieve certain basic human capabilities and functionings (Sen, Citation1999). This brief theoretical perspective leads to the concept of deprivation as a multidimensional phenomenon.
The UNDP in collaboration with the Oxford Poverty and Human Development Initiative (OPHI) have been analyzing the global multidimensional poverty index using three dimensions made up of 10 indicators each year since 1990 (Alkire et al., Citation2019). These dimensions are as follows: health measured by nutrition and child mortality indicators; education measured by school attendance and years of schooling indicators; and living standards measured by cooking fuel, sanitation, drinking water, electricity, housing conditions and assets indicators. This study employs seven child-specific dimensions namely nutrition, health, education, shelter, water, sanitation and information to examine multiple child deprivations in Kenya. These dimensions are anchored on the Convention of the Rights of the Child and the Constitution of Kenya.
3.2. Empirical literature
There are three approaches that have been used to measure child poverty. The monetary approach identifies poor children as those living in households with income below the poverty line. Makhalima et al. (Citation2014), for example, used an equivalence scale to determine the poverty line for children in South Africa. Subsequently, a binary logistic regression model was used to estimate the association between child poverty and household total income, employment status, age, and sex of the household head, and the size of the household. The results indicate that the head of the household being in employment, number of people living in the household, and total household income were significant determinants of child poverty.
The monetary measures of child well-being have been vehemently criticized because they are based on adult ideas and fall under unidimensional approaches (A, E Minujin & Delamonica, Citation2012). Although the use of income poverty is vital, it does not tell us the true picture of child poverty. Using income as a measure of child poverty understates the needs of children, which include investment in schools and hospitals, among other facilities undertaken by governments, and these are not captured when measurements are based on household income. Moreover, the rates of poverty among children are substantially higher given that poor households tend to have a higher share of children than non-poor households in developing countries (Y. Batana et al., Citation2013).
The second approach for measuring child poverty is the Bristol deprivation framework (Gordon et al., Citation2003). Gordon et al. (Citation2003) analyzed child poverty from a multidimensional perspective based on the Convention of the rights of the child (UN, Citation1989) and basic needs approach. The dimensions considered in this approach are nutrition, health, education, shelter, sanitation, water, and information. Failure to provide these constitutive rights can be regarded as contributing to child deprivations when access to the basic needs is lacking. This approach has been used by Y. M Batana et al. (Citation2014) for Ugandan children
Y. M Batana et al. (Citation2014) analyzed determinants of multidimensional deprivation among Ugandan children from the year 2000 to 2009 using Uganda Demographic and Health Survey data. They defined child poverty as those children who are simultaneously suffering from two or more deprivations. They adopted a binary logit model to analyze the determinants of child deprivations and estimated three regression models for children deprived in two or more dimensions, three or more dimensions, and four or more dimensions. The results indicate that child, household, and community characteristics were important determinants of child deprivations. Our study adopted a similar conceptualization of multidimensional deprivation but instead used an ordered logit model. The ordered logit model allows child deprivations to have a natural rank that reflects the severity of child deprivations which binary logistic model does not.
Kabubo-Mariara et al. (Citation2012) analyzed child poverty in Kenya using child survival and asset index as measures of child well-being. The authors estimated the determinants of child mortality using a survival model. In another study, Kabubo-Mariara et al. (Citation2011) analyzed multidimensional child poverty in Kenya using a composite indicator of wealth and child health consisting of stunting, wasting and underweight indicators. The analysis was based on a bivariate probit model with the correlates being child, household, maternal, and environmental characteristics. The results of these studies complement each other. Results of the 2011 study, for example, revealed that boys were more malnourished than girls. This finding was also corroborated by the 2012 study which found that boys had a higher probability of dying than girls. The two studies found an association between mothers’ education as well as the physical environment and child well-being. Further, the two studies examined only children below five years of age using data from KDHS 1993–2003. This study attempts to incorporate all the dimensions of child well-being for children aged 0–17 years and uses updated data up to 2014.
The latest study on multidimensional child poverty in Kenya is by Kenya National Bureau of Statistics (Citation2017). The study examined child poverty in Kenya using the multiple overlapping deprivation analysis (MODA) methodology using the 2014 KDHS data. The study found that the deprivation rates were significantly higher for children between 12 and 59 months (29%) compared to children between 0 and 11 months (12%). The deprivation rates in nutrition were 37% between 12 and 59 months and 17% between 0 and 11 months. The highest deprivations among the children aged 0–11 months were sanitation at 54% followed by housing at 53%. Similarly, among the children aged between 5 and 14 years, the highest deprivation was observed in sanitation at 58% followed by housing at 52%. The deprivation rates for health, education and information were 38%, 37% and 27%, respectively. However, the study only assessed child deprivation from KDHS 2014. This study examines child deprivations utilizing five waves of KDHS from 1993 to 2014 using the normative approach and internationally agreed dimensions of child well-being.
Fernandes et al. (Citation2012) presented empirical evidence on the main determinants of overall child well-being in Portugal. The study sought children’s own perspective in engaging in measurement of child well-being. The results show that introducing subjective components based on children’s opinions changes the results of measurement of child well-being, especially in terms of the ordering of the main determinants of overall well-being. Using Ordinary Least Square (OLS), they found that both mother’s and father’s education level contributed positively to the overall well-being of children. Moreover, having unemployed parents strongly reduced overall child well-being while father’s unemployment impacted more severely on the child’s overall well-being than mother’s unemployment. These results support the findings of earlier studies in the same country (e.g., Bastos et al., Citation2004; Bastos & Machado, Citation2009).
Bastos et al. (Citation2004) analyzed child poverty in Portugal from the perspectives of family income and child deprivation-evaluated by non-monetary indicators-to determine if there exist an overlap between the two approaches. They estimated a binary logit model where deprivation status was the dependent variable. The study found that children living in households with only Portuguese members seemed to show better outcomes than children living in households with double nationality with Portuguese or foreigners. However, whereas earlier studies (Kabubo-Mariara et al., Citation2011, Citation2012; Bastos et al., Citation2004; Bastos & Machado, Citation2009) found that boys experienced lower levels of well-being than girls, Fernandes et al. (Citation2012) did not find significant difference between the well-being of boys and girls.
The third approach is known as the composite child well-being indices (see for example, Land et al. (Citation2001), (Citation2007), Bradshaw et al. (Citation2007), and Bradshaw & Richardson (Citation2009)) for the European Union). These studies fall under multidimensional approaches to measurement of poverty. The domains used to capture the well-being of US children are material well-being, health, safety, productive ability, place in community, intimacy, and emotional well-being. In the Europe, child welfare indicators were clustered into eight groups as follows: material situation, housing, health, subjective well-being, education, children’s relationships, civic participation, and risk and safety (Bradshaw et al., Citation2007). The approach relies on large data sets and data for most of the variables are not available for developing countries like Kenya. Therefore, in this study we adopt the domains used by Gordon et al. (Citation2003) to capture the well-being of Kenyan children.
4. Analytical framework
The analytical framework consists of constructing a multiple-child deprivation variable and using it within an econometric framework. The econometric framework relates the probability of a child being at a particular deprivation category to individual, household and community characteristics.
4.1. Measurement of deprivation
The first objective of this study was to examine the distribution of multiple child deprivations by various characteristics. The first step is to examine deprivation at the indicator level. The second step is to examine deprivation at the dimension level and finally the third step is to aggregate the number of dimensions each child is deprived of and determine whether a child is poor or not depending on the child poverty threshold (Gordon et al., Citation2003).
The notation at the indicator level is given as follows;
Where IV, stands for indicator vulnerability, I, is a dummy variable with value 1 if a child is deprived and 0 otherwise, n is the sample of children with which the indicator i is observable.
The notation for dimension deprivation is given by:
Where DV is dimension vulnerability and D is a dummy variable with value 1 if a child is deprived in that dimension and 0 otherwise. A child is deprived in a dimension if he or she suffers deprivation in at least one indicator within that dimension. The notation is given by Equationequation 3(3)
(3) as:
Where d stands for the number of indicators within a specific dimension.
In the literature, there are three approaches used to determine multidimensional deprivation rates. These are union, intermediate and intersection approaches (Alkire & Foster, Citation2011; Atkinson, Citation2003). In this study, we use the union approach to determine the vulnerability of children in each dimension, i.e., if a child is deprived/vulnerable in one of the indicators within a dimension, the child is considered to be deprived in that dimension. Consequently, the children are ranked based on the number of deprivations they are suffering from with a presumption that a child deprived in one dimension is better-off than a child deprived in two, three, four or more dimensions.
4.2. Ordered logit model specification
The second objective of the study was to investigate the main correlates of multiple child deprivations. This was achieved by estimating an ordered logit model (Long & Freese, Citation2001). The children were ranked from those who were not deprived to those who are deprived in four or more dimensions. The study assumed that a child facing deprivation in one dimension is considered better-off than that deprived in two or more dimensions.
The values of the dependent variable, Yi, are ordered integer values from 0 representing children who are not deprived to 4 representing children facing at least four deprivations; Yi {0, 1, …, 4}. The ordinal response model for Y (conditional on explanatory variables X) can be derived from a latent variable model Y*. Following Wooldridge (Citation2002), the continuous unobserved variable is defined as:
Where i indicates the observation and u is the random error and Y* has various threshold points. The relationship between observed outcomes Y and the unobserved outcomes Y* are shown in the equations below. The value of the observed variable Y depends on whether a child has crossed a particular threshold or not. Let α0< α1< α2< α3< α4 be unknown cut-off points (or threshold parameters), and define:
In this essay, the observed ordinal variable Y takes on the values of 0, 1, 2, 3 and 4 and therefore there are 4 cut-off points.
The observed response variables are associated with the unobserved variables as described below:
Where X, is a set of independent variables, which include child, household, locational and communal characteristics. Communal characteristics include the availability of infrastructure and services (e.g., electricity) in the community. is a column vector of parameter estimates and
is the error term. This essay assumed that the distribution of µ is distributed logistically with a mean of 0 and variance of π2/3,
. This modelling leads us to ordinal logit model. We then have the following probabilities.
Where, is the logistic probability distribution function. The maximum likelihood method (MLM) is used to estimate the ordered logit model. In ordered logit model, an underlying score is estimated as a linear function of the independent variables and a set of cut-off points (or threshold parameters). The probability of observing outcome i corresponds to the probability that the estimated linear function, plus random error, is within the range of the cut-off points estimated for the outcome. We are not interested in
, as Y* is an abstract construct and therefore difficult to interpret (Greene, Citation2012). What we are interested in is the marginal effects which are computed differently for continuous and discrete variables.
For the five probabilities above, the marginal effects in the changes of independent variables are as shown in the equations below:
Where , is the probability density function. In our model, we have both binary and continuous regressors. The marginal effects for discrete (i.e., categorical) and continuous variables are calculated differently (Long & Freese, Citation2001). The marginal effects of binary regressors measure the change in probabilities when the binary variable changes from 0 to 1, with all other independent variables at their means values. The marginal effects of a continuous variable measure the amount of change in the dependent variable produced by a one-unit change in the independent variable, with all other variables kept at their means.
The marginal effect of a binary variable is:
4.3. Definition, measurement and relationship between dimensions
The dimensions of child deprivation are based on internationally agreed definitions anchored on the Convention of the Rights of the Child (CRC), which has been acceded to by 194 states to date (UNGA, Citation2014). The only countries that have not ratified the treaty are Somalia, South Sudan and the United States. The CRC gives children the right to survive, develop, participate, and be protected. These rights were adopted in 1989 and recognize the child as the subject of the rights that the state commits to respect, protect, and fulfil, guided by the best interest of the child (Gordon et al., Citation2003; UNICEF, Citation2004; UN, Citation1989). Therefore, the concept of child deprivations embodies violation of these fundamental human rights. presents the dimensions of child well-being and their measurement indicators as well as the age cohort of children in which the dimensions are applicable to and their deprivations cut-offs.
Table 1. Definitions of measurement of dimensions
The first dimension which is vital to child development is nutrition. This study used three indicators to measure nutrition-wasting which reflects acute or short-term undernutrition, stunting which portrays chronic or long-term malnutrition and underweight which is an aggregate indicator of the first two indicators (WHO, Citation2006). The effects of undernutrition are devastating and it is very difficult for children to recover from the consequences of malnutrition (UNICEF, WHO and World Bank, Citation2020). Children who do not meet their minimum nutritional needs have a higher risk of contracting diseases, poor growth development or death. They have been linked also to poor outcomes in school and thus exacerbate poverty. Children may lose weight also due to poor health as they may experience diarrhoea or dysentery.
The second dimension is health where we looked at immunization of children to promote child health and prevention of childhood illness (WHO, Citation2017). A child who is not vaccinated has poor immunity to protect itself from diseases. Children who are sick would not be able to attend school thus affecting the education dimension. At the same time, children who are not well nourished with proper diet may not have immunity to fight diseases and thus impairs child health. Severe health deprivation is closely linked to poverty, with poor children more likely to become sick and die. WHO (Citation1995) stated that the greatest killer and cause of ill health and suffering in the whole world is extreme poverty. Poverty is the main reason why children are not vaccinated; safe drinking water and sanitation are not provided.
Third is the education dimension which examined school attendance of children aged between 6 and 18 years. Education is a basic human right which is embedded in the Kenya’s constitution and other international human rights and development agenda like sustainable development goals (SDGs). This dimension has been argued as the most important to lift people out of poverty (Psacharopoulos & Patrinos, Citation2018). Children who do not have education have fewer opportunities in life (UNESCO, Citation2012). Children of illiterate parents are more likely to have poorer health, to drop out of school themselves and be working rather than attending school. Need is seen to undertake a study on the impact of novel COVID-19 pandemic on education in Kenya. Schools were closed for almost a year and high teenage pregnancies have been reported during the cessation of movement.
Fourth is the shelter or housing conditions of which we examined the type of materials used to construct the roof, walls and floors. A child living in a poorly constructed house with materials which are considered to be poor affect the well-being of the child. It may also affect the psychological emotion of a child when they meet with other contemporaries in school and this may lower their self-esteem and thus inability to maintain a relationship with their peers (Makhalima, Citation2020). Mad floors are critical especially to young children who spent most of their time crawling and playing on the floor and they may end up picking pathogens which affect their health.
Fifth is the water dimension measured by two indicators: source of drinking water and time taken to fetch water. Children who drink water from open sources may contract water borne diseases such as diarrhoea, cholera, dysentery, typhoid, hepatitis A, schistosomiasis and polio thus affecting their health while those who travel long distance to fetch water affect their schooling (WHO, Citation2019). Open water sources may be compounded by poor sanitation facilities which expose children to higher health risks. With the onset of COVID-19, it is very clear that water is very important in combating the pandemic (WHO, Citation2020).
Sixth is the sanitation dimension which we examined the type of toilet facilities present in households where children are living. Improper storage of human waste may cause serious diseases like diarrhoeal diseases which may cause child deaths within a short period. Poor children often live in homes which lack even basic forms of sanitation where people have to resort to open defecation. When lack of sanitation combines with open water sources pose serious health issues in the community (WHO and UNICEF, Citation2005). In Kenya, children from slum areas also experience ‘flying toilets ‘because of poor infrastructure and high population.
Last but not least is the information dimension which we assessed using access of information using either radio or television within the household. This dimension evaluates the exposure of children to the outside world and also consumption of important information being relayed by government on public health matters. During the lock down necessitated by the COVID-19, some schools in Kenya continued to give instructions to pupils/students through radio or televisions and those children living in households without the mass media equipment missed out greatly which will lead to poor educational outcomes. Need is seen on the impact of COVID-19 on schooling in Kenya.
4.4. Explanatory variables
A number of correlates of multiple child deprivations can be identified from the literature including child, household, and community characteristics as given by the theoretical and empirical literature review. presents details of the independent variables.
Table 2. Description of explanatory variables
5. Results and discussions
5.1. Distribution of multiple child deprivations
The first objective of this study was to examine the distribution of multiple child deprivations vis-a-vis various individual, household, and regional characteristics in Kenya. presents the distribution of multiple child deprivations by sex of the child, sex of the household head, area of residence and access to electricity. The results reveal that 6.71% of girls and 6.75% of boys did not suffer any deprivation while 30.45% of girls and 32.04% of boys were deprived in four or more dimensions. Relative to boys, however, more girls were deprived in one, two, and three dimensions. The average number of deprivations were 2.62 for girls and 2.66 for boys.
Table 3. Distribution of multiple child deprivations by sex of the child, sex of the household head, area of residence and access to electricity (%)
In terms of urban-rural disparities, there was a large discrepancy in child deprivation. These results support the spatial phenomenon of poverty revealed in past studies (Kabubo-Mariara et al., Citation2012, Citation2011). Children from rural areas experience more deprivations than their counterparts in urban areas as poverty deepens. The results showed that 20.44% of urban children were not deprived in any dimension compared with only 2.36% from rural areas, whereas 38.36% of rural children were deprived in four or more dimensions as compared to 8.95% from urban areas. Children from rural areas were overrepresented in terms of number of deprivations, supporting findings of past studies that poverty is a rural phenomenon. The average number of deprivations for children from rural areas was 2.97 compared to 1.62 for urban children.
The concentration of poverty in female headed households has led to introduction of the phrase “feminization of poverty” in the literature (Chopra, Citation2020). Comparing the differentials of deprivations between children from female and male-headed households, we found that children from female headed households suffered more deprivations. Specifically, 33.50% of children living in female headed households were deprived in four or more dimensions compared with 30.33% of children from male headed households. There were more children from male headed households not deprived in any dimension relative to their counterparts from female headed households.
Access to electricity has been found to be an important determinant of poverty (Kabubo-Mariara et al., Citation2012). This study found that 37.32% of children from households with electricity were not deprived in any dimension compared with 1.47% of children living in households with no electricity. On the other hand, 36.62% of children living in household without electricity were deprived in four or more dimensions compared with a paltry 0.89% of children from households with electricity. The average deprivations for children without electricity stood at 2.94, while those with electricity was less than 1%. It is evident that having electricity in a household reduces child deprivations.
Closely related to rural-urban disparities are regional disparities. presents the regional disparities in child deprivations. We found that Nairobi region had the largest number of children that were not deprived. Specifically, 29.03% of children from Nairobi region did not experience any deprivation compared with 3.01% of children from North-Eastern region. Nairobi region also had the highest proportion of children that experienced deprivation in only one dimension. Similarly, Nairobi region also had the least number of children deprived in four or more dimensions. Specifically, 1.02% of children from Nairobi region were deprived in four or more dimensions compared with North-Eastern region where more than 60% of children were deprived in four or more dimensions. This observation could be attributed to accessibility to infrastructure and services in Nairobi region (e.g., schools, hospitals, electricity, and piped water in their dwellings) relative to other regions. The average number of deprivations for Nairobi region children was 1.13 compared to 3.30 for North Eastern region children.
Table 4. Distribution of deprivations by region (%)
Table 5. Marginal effects after ordered logit of multiple child deprivations for children aged 0–5 years
Table 6. Marginal effects after ordered logit of multiple child deprivations for children aged 6–17 years
in the appendix presents the distribution of child deprivations by the level of education, marital status and religion of the mother. We found that the well-being of a child improved greatly with the level of education of the mother. For example, 39.01% of children living in households whose mothers had post-secondary education (college and university) were not deprived in any dimension compared with 0.93% whose mothers had no education. More than half of the children that suffered deprivation in four or more dimensions had mothers with no education. These results corroborate past studies (Bastos et al., Citation2004; Bastos & Machado, Citation2009; Kabubo-Mariara et al., Citation2012; Mwabu, Citation2007) which found that a higher level of education of the mother improves child well-being. On average, children whose mothers had higher education were almost non-deprived (less than 1 deprivations) while children whose mothers had no education faced 2.38 deprivations.
The role of the family was also examined and the results show that 40.50% of children from widowed mothers were deprived in four or more dimensions while 21.41% were from mothers who had never been married. On average, children from widowed households suffered three deprivations as compared with those from single mothers facing two deprivations. These results suggest that children from widowed families are more vulnerable to multiple deprivations.
Research on the link of religion on has been well documented (Hoverd & Sibley, Citation2013). Overall, we observed that more children from non-religious families suffered deprivation compared with children from families that profess religion. For example, 60.84% of children with no religion suffered four or more deprivations compared with 47.02% from Muslim, 26.02% from Protestant and 31.19% from Catholic faiths. The average number of deprivations experienced by children in each category was 2.66 for Catholics, 2.52 for Protestants, 2.96 for Muslims and 3.36 for those without religion.
5.2. Descriptive statistics
The details of the descriptive statistics of the variables used for children between ages 0–5 years and between 6 and 17 years are presented in in the appendix. The dependent variable is a categorical variable based on the number of deprivation(s) that a child was facing, with no deprivation considered being the best outcome, while deprivation in four or more dimensions as the worst outcome. We found that about 3.8% of the children below five years of age and 12.4% of those over five years old did not face any kind of deprivation. Conversely, 38.5% of children under five years of age and 32.3% of those above six years faced deprivation in four or more dimensions.
The average age of children below five years was 27.9 months, while 26.9% of children above five years of age were in secondary school. Female-headed households accounted for 28.4% of children below five years and 35.6% of those above five years. This means that, overall, one-third of households in Kenya are headed by females. In terms of mother’s education, 20.3% of children below five years were raised by mothers with no education, 56% by those who had completed primary education, 19% by those who had completed secondary education, and 0.46% by those with college and higher level of education. Access to electricity accounted for 15% and 13.2% of children below five years and above five years respectively. On religion, 61.4% of children were from Protestant households, 21.3% from Catholic households, 14.2% from Muslim households, and 3.0% from households without religion.
Over 80% of mothers were married for the two cohorts of children, while less than 2% were either divorced or separated. About 5.3% of the mothers were single for 0–5 years cohort and 2.59% for the 6–17 years cohort. The rest of the descriptive statistics can be seen in .
5.3. Correlation between dimensions
We estimated the pairwise correlations between dimensions of child well-being. An absolute value of 1 indicates a perfect association of the two dimensions. If correlations are completely independent, then the correlation coefficient is 0. Please refer to in the appendix for correlation matrix between the child dimensions. Generally, the values of the different correlation coefficients do not indicate any strong relationships among the variables. The results, however, indicate that there is a negative and significant correlation between nutrition and health and between health and water. The rest of the dimensions have a positive and significant correlation. The highest correlations were found between shelter and sanitation at 45.88%, between shelter and information at 28.51%, and between water and sanitation at 21.01%. There were no pairwise correlations above 50% suggesting that each dimension was independent and captured a different attribute of child well-being. These results corroborate the literature on section 4.3 of this study.
5.4. Correlates of multiple child deprivations
The second objective of this study was to investigate the main correlates of multiple child deprivations. present marginal effects obtained from an ordered logit model. We present regression results separately for children below five years and for children aged 6–17 years. The estimates are presented in columns labelled 1 to 6. Column 1 gives us the coefficients of the ordered logit as given by Equationequation 4(4)
(4) . In interpreting the coefficients of the ordered logit model, the sign of the coefficients gives the direction of the predicted probabilities of our extreme outcomes that is, for our case, children with no deprivation and those suffering from four or more dimensions of deprivation. Columns 2 to 6 give us the marginal/partial effects of regressors on the response probability as shown in estimating Equationequations 10
(10)
(10) to 14. We discuss the results concurrently.
The findings show that children’s own characteristics influenced the probability of children suffering multiple deprivation. The coefficient of female child was negative and significant in both categories of children, suggesting that girls in a household were more unlikely to suffer multiple deprivations. For children below five years of age, the probability of observing a deprived female child in four or more dimensions was 1.61% lower than that for a male child. In the case of children over six years, the probability of observing a female child deprived in four or more dimensions was 0.78% lower than that of their male counterparts. Past studies in Kenya are consistent with this finding that male children are more likely to face deprivation (e.g., malnourished) than female children (Kabubo-Mariara et al., Citation2009).
The coefficient of age of a child in months had a positive and significant effect on the probability of suffering multiple deprivations. Conversely, the coefficient of age squared had a negative sign indicating that the probability was increasing at a decreasing rate with a turning point at 32.25 months. These results suggest that child well-being is sensitive to factors such as feeding/weaning practices at various stages of growth. Kabubo-Mariara et al. (Citation2009) found similar results where older children were more likely to suffer malnutrition relative to their young, un-weaned counterparts. The authors argue that as children grow older, weaning and less breast milk renders them more vulnerable to malnutrition, but once they are completely weaned, children are more likely to obtain adequate nutrients from regular food intake leading to improved nutritional status. Children aged 6–17 years were categorized into primary and secondary school-aged children with primary school-aged children as the reference group. The coefficient of secondary school-aged children was positive and significant implying that children in this group were more likely to suffer deprivation in four or more dimensions than primary school-aged children. Specifically, chances of finding a secondary school-aged child deprived in four or more dimensions was 1.43% more than the case in primary school-aged child.
The coefficient of birth order of the child was positive and significant at 1 percent confidence level. This finding suggests that the probability of suffering deprivation in multiple dimensions increases with one unit increase in the birth order of the child. The probability of observing a child that is not deprived falls by 0.03%, while the probability of observing a child that is facing deprivation in four or more dimensions increases by 0.69% as the birth order increases. This finding is consistent with Kabubo-Mariara et al. (Citation2009) who found that higher birth order children were more likely to suffer malnutrition than their counterparts of lower birth order.
Regarding the effects of twins or multiple births, the coefficient of a twin child was positive and significant, suggesting that twins have a higher chance of suffering multiple deprivations. For instance, the probability of observing a twin child being deprived in four or more dimensions is 5.55% higher than a singleton. This could be due to the hardships experienced by families having multiple births relative to those with singletons.
The coefficient of the age of the household head was negative and significant with a positive quadratic term for children under five years suggesting that the probability of experiencing deprivation in multiple dimensions is decreasing at an increasing rate (U-shaped curve). The statistics were insignificant for older categories of children.
The effect of female or male headed household on the well-being of a child was also examined. For children over five years old, the coefficient of female headed household was positive and significant suggesting that children from female headed households were more likely to suffer deprivation in four or more dimensions. For instance, the probability of observing deprivation in four or more dimensions was 1.53% higher for children in female headed households than their counterparts from male headed households. However, the statistics on this aspect were insignificant for children under five years.
The coefficient of household size was unexpectedly negative and significant in the cohort of children under five years but insignificant in the cohort of children aged 6–17 years. It appears that each additional household member reduces the probability of a child being deprived in four or more dimensions. The marginal values of observing deprivation increase by 0.02% in the case of one dimension and 0.37% for two dimensions. However, the marginal probabilities of observing a child deprived in three and four or more dimensions reduce by 0.2% and 0.4%, respectively, for a unit increase of household member. This observation contradicts the results of past studies on determinants of poverty where larger households tend to be poorer (Geda et al., Citation2005; Mwabu et al., Citation2000). Kabubo-Mariara et al. (Citation2009) found that household size is inversely correlated with nutritional status of children suggesting competition for food among siblings.
The coefficient of educational attainment of the mother was unequivocal across categories of children. The negative signs of the coefficients suggest that the higher the level of education of the mother, the lower the level of child deprivation. Thus, a child whose mother had primary level education had a 22.39% lower probability of being deprived in four or more dimensions than one whose mother had no formal education. Similarly, a child from a household where the mother had secondary level education had a 30.92% lower probability of being deprived in four or more dimensions compared to one from a household where the mother had no formal education. For a child whose mother was educated up to higher level, the probability of being deprived in four or more dimensions was 32.74% lower than that of a child whose mother had no formal education. Similar results were observed for the cohort of children aged 6–17 years. This result is consistent with findings by Kabubo-Mariara et al. (Citation2009) that education of the mother is an important determinant of nutritional and health status of children. Mwabu et al. (Citation2000) and Geda et al. (Citation2005) also found that lower levels of maternal education or no education at all account for higher probabilities of being poor. Kabubo-Mariara et al. (Citation2012) also found that targeting women through secondary and post-secondary education can make enormous contribution towards reduction of child mortality.
The literature is rife with the concern of maternal age at first birth and child development (Hoffman & Maynard, Citation2008). The coefficient of maternal age at first birth was negative and significant, indicating that as the age of the mother increases the probability of child deprived in four or more dimensions reduces in both cohorts of children. This finding supports the argument that children born to young mothers are more likely to suffer poor health, educational and cognitive outcomes (Cooksey, Citation1997). This study suggests that education of the girl child is key in delaying the age of childbearing.
It was evident that children from divorced families were more likely to suffer multiple deprivations compared to those from married families for children under 5 years. The chances of observing a child deprived in four or more dimensions from a divorced family was 6.08% higher than those from married couples. The marginal effects for other marital statuses were insignificant. For children aged 6–17 years, the probability of observing a child raised by a widowed mother deprived in four or more dimensions was 3.13% higher than a child living with both parents, while the probability of observing a child with separated mother deprived in four or more dimensions was 3.79% higher than a child from married couples.
The effect of locational variables was also examined and the coefficient of rural residence was positive and statistically significant in both categories of children. This observation implies that children from rural areas were more likely to experience deprivation in four or more dimensions than their counterparts from urban areas. Thus, a child under 5 years, from the rural areas had a 13.62% higher probability of being deprived in four or more dimensions than one from urban areas. Similarly, for the 6–17 years cohort, a child from the rural areas had a 14.64% higher probability of suffering deprivation in four or more dimensions relative to one from urban areas. This result is consistent with past studies in Kenya where majority of poor individuals and children live in rural areas (see for example, Mwabu et al., Citation2000; Geda et al., Citation2005; Kabubo-Mariara et al., Citation2009, Citation2011, Citation2012).
Regional dummies were included in the study to capture regional differences. We found that the coefficients of all the provinces were negative relative to Nairobi (reference group) except North Eastern province whose coefficient was positive and significant. This result implies that children from North Eastern region were more likely to experience multiple deprivations compared with children from Nairobi region. Specifically, a child from North Eastern region was 17.73% more likely to be deprived in four or more dimensions than his or her counterpart from Nairobi region. However, a child living in central region was 4.69% less likely to become deprived in four or more dimensions than one from Nairobi region. The probability of a similar occurrence for Nyanza region relative to Nairobi was 4.11%. These results corroborate Kabubo-Mariara et al. (Citation2011) who found that children from all regions were relatively poorer than Nairobi region. Kabubo-Mariara et al. (Citation2011) pointed out that these regions are expected to be poor due to geographical isolation, a low resource base, low rainfall and other vicious climatic conditions.
The respondent’s working status proved to be an important determinant of child well-being. The coefficient of working status of the respondent was negative and significant, suggesting that children from caretakers who are in employment were less likely to experience multiple deprivations compared with those caretakers who were not working. Specifically, children whose caretakers were employed had a 1.15% and 1.69% less chance of becoming deprived in four or more dimensions than those whose parents were unemployed for 0–5 years cohort and 6–17 years cohort respectively.
Access to electricity was used to capture community-level infrastructural development and households’ standard of living. The coefficient of access to electricity in the household was negative and statistically significant. This finding suggests that children living in households with electricity were less likely to suffer multiple deprivations compared with their counterparts without electricity. Specifically, a child who accessed electricity in the home was 19.21% and 22.23% less likely to become deprived in four or more dimensions than their counterparts for 0–5 years cohort and 6–17 years cohort, respectively. This finding supports previous work by Kabubo-Mariara et al. (Citation2011) that community infrastructure is an important factor for lifting people out of poverty.
The year of Survey dummies were included to capture the dynamics of child deprivations over time of which the 1993 survey was our reference point. In the first cohort of children, the coefficients of 1998, 2008 and 2014 surveys were negative and significant, indicating that multidimensional poverty of children declined over the study period. However, the coefficients of the 2003 survey were positive but insignificant. In the 6–17 years cohort of children, the coefficients for 1998 and 2003 surveys were positive, while the coefficients for 2008 and 2014 were negative and statistically significant. Given these trends, we believe that the intergenerational transmission of multidimensional poverty would be curtailed.
6. Conclusions
This paper analyzed the distribution of multiple child deprivations and investigated the main factors affecting multiple child deprivations in Kenya using the 1993–2014 KDHS. The study employed seven child-specific dimensions, namely: nutrition, health, education, shelter, water, sanitation, and information. The empirical results reveal large disparities in multiple deprivation between different socio-economic groups of children. For example, children living in rural areas are more vulnerable to multiple deprivations than their urban counterparts. In terms of regions, children living in North-Eastern and Eastern parts of Kenya are more prone to multidimensional poverty suggesting existence of large horizontal inequalities in Kenya. The share of multiple deprivations was disproportionately high among children whose mothers were widowed compared with children living with both parents. In relation to sex of the child, girls appear to enjoy better outcomes than boys.
A notable household characteristic was the level of education of the mother, which was found to play a key role in reducing child deprivations. The results showed that when mothers’ level of education increases, the number of deprivations in a child decline. Regarding access to electricity, children living in households with access to electricity were less likely to experience multiple deprivations than those living in households without access to electricity.
The results of this study have implications for policy to address multidimensional deprivations in children. We suggest that policy should focus on multiple dimensions of well-being in order to tackle the complex nature of poverty which has many facets. Policies should deliberately target development of rural areas and remote regions where poverty is rampant and aim to provide easy access to essential infrastructural services like schools, electricity, hospitals and water supply schemes. The effect of mother’s education was unequivocal in improving child well-being suggesting policies for promoting the education of every girl child up to the highest level possible. Existing policies like those supporting the provision of sanitary towels in schools should be encouraged to reach all girls and also ensure sustainability. This will ensure that girls’ rights are protected and fulfilled which will greatly enhance the range of life choices available to them as women. Special attention should be given to widows and their children in the society. Existing social security and income transfer policies targeting orphans and vulnerable children should be enhanced and fast-tracked to reach all of them in the country.
This study suggests future research to examine the impact of COVID-19 pandemic on the welfare of children. Due to lock-down, school attendance was greatly affected and a lot of teenage pregnancies were reported, overstretched hospitals due to huge hospitalization of the affected persons.
Disclosure of potential conflicts of interest
No potential conflict of interest was reported by the authors.
Acknowledgements
The authors would like to thank the African Economic Research Consortium (A.E.R.C.) for funding this study. The views expressed in this study are those of the authors and not necessarily those of the African Economic Research Consortium.
Additional information
Funding
Notes on contributors

Isaiah Kiprono Byegon
Isaiah Kiprono Byegon is a PhD Candidate at the University of Nairobi. He is also a Chief Economist at The National Treasury and Planning, Kenya. His interest includes poverty and inequality, food and nutrition security and environmental issues. (corresponding author: [email protected])
Jane Kabubo–Mariara is professor of Economics at the University of Nairobi. She is also the Executive Director of the Partnership for Economic Policy since 2016. Her research interests include multidimensional and child poverty and youth and female labour market outcomes. She has published widely in peer reviewed journals such as the Journal of African Economies, the Journal of Developing Areas among others.
Anthony Wambugu is an Associate Professor of Economics at the University of Nairobi. His research interest include; labour market issues, human capital investment and poverty. He has published in refereed journals like Oxford Bulletin of Economic and Statistics, Economic Letters and many others.
References
- Achia, N. O. T., Wangombe, A., & Khadoli, N. (2010). A Logistic regression model to identify key determinants of poverty using demographic and health surveys data. European Journal of Social Sciences, 13(1), 38–30.
- Alkire, S., Dirksen, J., Nogales, R., & Oldiges, C. (2020). Multidimensional poverty and vulnerability to COVID-19: A rapid overview of disaggregated and interlinked vulnerabilities in Sub-Saharan Africa. OPHI Briefings, Issue 54a, Oxford Poverty and Human Development Initiative, University of Oxford..
- Alkire, S., & Foster, J. E. (2011). Counting and multidimensional poverty measurement. Journal of Public Economics, 95(7), 476–487. https://doi.org/10.1016/j.jpubeco.2010.11.006
- Alkire, S., Kanagaratnam, U., & Suppa, N. (2019). The global multidimensional poverty index (MPI) 2019, OPHI MPI Methodological Note 47, Oxford Poverty and Human Development Initiative, University of Oxford.
- Alkire, S., Oldiges, C., & Kanagaratnam, U. (2021). Examining multidimensional poverty reduction in India 2005/6–2015/16: Insights and oversights of the headcount ratio. World Development, 142, 105454. https://doi.org/10.1016/j.worlddev.2021.105454
- Atkinson, A. B. (2003). Multidimensional deprivation: Contrasting social welfare and counting approaches. Journal of Economic Inequality, 1(1), 51–65. https://doi.org/10.1023/A:1023903525276
- Atkinson, A. B. (2019). Measuring poverty around the world. Princeton University Press.
- Bastos, A., Fernandes, G. L., & Passos, J. (2004). Child income poverty and child deprivation: A study on measurement. International Journal of Social Economics, 31(11/12), 1050–1060. https://doi.org/10.1108/03068290410561168
- Bastos, A., & Machado, C. (2009). Child poverty: A multidimensional measurement. International Journal of Social Economics, 36(3), 237–251. https://doi.org/10.1108/03068290910932738
- Bastos, A., & Nunes, F. (2009). Child poverty in Portugal: Dimensions and dynamics. Norwegian Centre for Child Research, 16(1), 67–87. https://doi.org/10.1177/2F0907568208101691
- Batana, Y., Bussolo, M., & Cockburn, J. (2013). Global extreme poverty rates for children, adults and the elderly. Economic Letters, 120(3), 405–407. https://doi.org/10.1016/j.econlet.2013.05.006
- Batana, Y. M., Cockburn, J., Kasirye, I., Kabubo-Mariara, J., Tiberti, L., & Ahaibwe, G. (2014). Situation analysis of child poverty and deprivation in Uganda, working paper 2014-03, UNICEF and PEP.
- Bradshaw, J., Hoelscher, P., & Richardson, D. (2007). An index of child well-being in the European Union. Social Indicators Research, 80(1), 133–177. https://doi.org/10.1007/s11205-006-9024-z
- Bradshaw, J., & Richardson, D. (2009). An index of child well-being in Europe. Child Indicators Research, 2(3), 319–351. https://doi.org/10.1007/s12187-009-9037-7
- Chopra, S. (2020). Measuring feminization of urban poverty among domestic workers in Delhi, India. Smart Cities and Regional Development, 4(1), 103–124. https://ideas.repec.org/a/pop/journl/v4y2020i1p103-123.html
- Cockburn, J., & Kabubo-Mariara, J. (2010). Child welfare in developing countries: An introduction. In J. Cockburn & J. Kabubo-Mariara (Eds.), Child welfare in developing countries(pp. 1-10).Springer. https://doi.org/10.1007/978-1-4419-6275-1_1
- Cooksey, E. C. (1997). Consequences of young mothers’ marital histories for children’s cognitive development. Journal of Marriage and the Family, 59(2), 245–261. https://doi.org/10.2307/353468
- Corak, M. (2006). Do poor children become poor adults? Lessons from a cross country comparison of generational earnings mobility. Discussion Paper No. 1993, Institute for the Study of Labor (IZA).
- De Neubourg, C., Chzhen, Y., Main, G., Martorano, B., Menchini, L., & Bradshaw, J. (2012). Child deprivation, multidimensional poverty and monetary poverty in Europe, Innocenti Working Papers No. 2012-02, UNICEF Office of Research, Florence.
- Deaton, A. (1997). The analysis of household surveys: A microeconometric approach to development policy. Johns Hopkins University Press, World Bank.
- Donfouet, H. P. P., Agesa, G., & Mutua, M. K. (2019). Trends of inequalities in childhood immunization coverage among children aged 12-23 months in Kenya, Ghana, and Côte d’Ivoire. BMC Public Health, 19(1), 988. https://doi.org/10.1186/s12889-019-7309-9
- Fernandes, J., Mendes, A., & Teixeira, A. (2012). Assessing child well-being through a new multidimensional child-weighting scheme index: An empirical estimation for Portugal, Working Paper, No. 02/2012, Faculdade de Economia e Gestão, Universidade Católica Portuguesa.
- Geda, A., de Jong, N., Kimenyi, M. S., & Mwabu, G., (2005). Determinants of poverty in Kenya: A household level analysis, Economics Working Papers. Paper 200544.
- Gordon, D., Nandy, S., Pantazis, C., Pemberton, S., & Townsend, P. (2003). Child poverty in the developing world. The Policy Press.
- Greene, W., . H. (2012). Econometric analysis (7th ed.). New Jersey: Prentice Hall.
- Heath, A., & Li, Y. (2015). Review of the relationship between religion and poverty: An analysis for the Joseph Rowntree Foundation. CSI Working paper, 2015–01.
- Hick, R. (2012). The capability approach: Insights for a new poverty focus. Journal of Social Policy, 41(2), 291–308. https://doi.org/10.1017/S0047279411000845
- Hoffman, S. D., & Maynard, R. A. (Eds.). (2008). Kids having kids: Economic costs & social consequences of teen pregnancy. The Urban Institute Press.
- Hoverd, W. J., & Sibley, C. G. (2013). Religion, deprivation and subjective wellbeing: Testing a religious buffering hypothesis. International Journal of Wellbeing, 3(2), 182–196.
- Hulme, D., & Mckay, A. (2008). Identifying and measuring chronic poverty: Beyond monetary measures? In N. Kakwani & J. Silber (Eds.), The many dimensions of poverty (pp. 187-214)Palgrave-Macmillan.
- Kabubo-Mariara, J., Karienyeh, M. M., & Kabubo, F. M. (2012). Child survival, poverty and inequality in Kenya. Does physical environment matter? African Journal of Social Science, 2(1), 65–84.
- Kabubo-Mariara, J., Ndenge, G. K., & Mwabu, D. K. (2009). Determinants of children’s nutritional status in Kenya: Evidence from demographic and health surveys. Journal of African Economies, 18(3), 363–387. https://doi.org/10.1093/jae/ejn024
- Kabubo-Mariara, J., Wambugu, A., & Musau, S. (2011). Multidimensional poverty in Kenya: Analysis of maternal and child well-being. UNICEF and PEP.
- Kenya National Bureau of Statistics. (2017) . Child poverty in Kenya: A multidimensional approach.
- Kenya National Bureau of Statistics. (2019). 2019 Kenya population and housing census, volume III: Distribution of population by age and sex. KNBS.
- Kenya National Bureau of Statistics and IFC Macro. (2015). Kenya demographic and health survey 2014. KNBS and IFC Macro.
- Laderchi, C. R., Saith, R., & Stewart, F. (2003). Does it matter that we do not agree on the definition of poverty? A comparison of four approaches. Oxford Development Studies, 31(3), 243–274. https://doi.org/10.1080/1360081032000111698
- Lanau, A., Mack, J., & Nandy, S. (2020). Including services in multidimensional poverty measurement for SDGs: Modifications to the consensual approach. Journal of Poverty and Social Justice, 28(2), 149–168. https://doi.org/10.1332/175982720X15850580703755
- Land, K. C., Lamb, V. L., Meadows, S. O., & Taylor, A. (2007). Measuring trends in child well-being: An evidence-based approach. Social Indicators Research, 80(1), 105–132. https://doi.org/10.1007/s11205-006-9023-0
- Land, K. C., Lamb, V. L., & Mustillo, S. K. (2001). Child and youth well-being in the United States, 1975-1998: Some findings from a new index. Social Indicators Research, 56(3), 241–320. https://doi.org/10.1023/A:1012485315266
- Long, J. S., & Freese, J. (2001). Regression models for categorical dependent variables using stata (2nd ed.). Stata Press.
- Makhalima, J. L. (2020). An analysis of the determinants of child poverty in South Africa. International Journal of Economics and Finance Studies, 12(2), 406–421. Doi: 10.34109/ijefs.202012210
- Makhalima, J. L., Sekatane, M. B., & Dunga, S. H. (2014). Determinants of child poverty in a South African Township: A Case of Boipatong Township. Mediterranean Journal of Social Sciences, 5(1), 235–242. Doi:10.5901/mjss.2014.v5n1p235
- Minujin, A. (2012). Child poverty in East Asia and Pacific: Deprivations and disparities. UNICEF.
- Minujin, A., & Delamonica, E. E. (2005). Children living in poverty: A review of child poverty definitions, measurements and policies. Paper for UNICEF Conference on Children and Poverty: Global Context, Local Solutions, New York, UNICEF, The New School University.
- Minujin, A., . E., & Delamonica, E. D. (2012). Multidimensional child poverty in Tanzania: Analysis of situation, changes and sensitivity of thresholds. In A. Minujin & S. Nandy (Eds.), Global child poverty and context, measurement, concepts, policy and action (pp. 263-286). The Policy Press.
- Mutunga, C. J. (2007). Environmental determinants of child mortality in Kenya. UNU-WIDER Research Paper No. 2007/83, Helsinki: United Nations University World Institute for Development Economics Research.
- Mwabu, G. (2007). Health economics for low-income countries. Handbook of Development Economics, 4, 3305–3374. https://doi.org/10.1016/S1573-4471(07)04053-3
- Mwabu, G., Masai, W., Gesami, R., kirimi, J., Ndeng’e, G., Kiriti, T., Munene, F., Chemengich, M., & Mariara, J. (2000). Poverty in Kenya: Profile and determinants. University of Nairobi and Ministry of Finance and Planning.
- Oyugi, L. N. (2000). The determinants of poverty in Kenya MA Thesis. Department of Economics, University of Nairobi.
- Psacharopoulos, G., & Patrinos, H. A. (2018). Returns to investment in education: A decennial review of the global literature. Education Economics, 26(5), 445–458. https://doi.org/10.1080/09645292.2018.1484426
- Sen, A. K. (1983). Poor, Relatively Speaking. Oxford Economic Papers, 35(2), 235–245. https://doi.org/10.1093/oxfordjournals.oep.a041587
- Sen, A. K. (1985). Commodities and Capabilities. North-Holland.
- Sen, A. K. (1999). Development as Freedom. Oxford University Press.
- Thorbecke, E. (2008). Multidimensional poverty: Conceptual and measurement issues. In N. Kakwani & J. Silber (Eds.), The many dimensions of poverty (pp. 3-19). Palgrave MacMillan.
- Townsend, P. (1979). Poverty in the United Kingdom: A Survey of household resources and standards of living. Penguin, Geneva.
- Townsend, P. (1987). Deprivation. Journal of Social Policy, 16(2), 125–146. https://doi.org/10.1017/S0047279400020341
- UN. (1989). The Convention on the rights of the child. The General Assembly resolution, 44/25. United Nation General Assembly.
- UN.(2014). Status of the Convention on the rights of the child. A/69/260. United Nations
- UN. (2015). Transforming our world: The 2030 Agenda for sustainable development. The General Assembly Resolution 70/1. United Nations.
- UNICEF. (2004). The state of the world’s children 2005: Childhood under threat.
- UNESCO. (2012). Youth skills: Putting education to work, EFA Global Monitoring Report 2012, Paris, UNESCO. http://hdl.voced.edu.au/10707/225656
- Vandemoortele, J. (2012). Equity begins with children. In A. Minujin & S. Nandy (Eds.), Global child poverty and well-being: Measurement, concepts, policy and action (pp. 39-51). The Policy Press.
- WHO. (1995). The world health report 1995: Bridging the gaps. Geneva.
- WHO. (2006). WHO child growth standards: Length/height-for-age, weight-for-age, weight-for-length, weight-for-height and body mass index-for-age: Methods and development. World Health Organization.
- WHO. (2017). WHO recommendations on child health: Guidelines approved by the WHO guidelines review committee. World Health Organization.
- WHO. (2018). Malnutrition. World Health Organization.
- WHO. (2019). Drinking water. World Health Organization.
- WHO. (2020) . WHO global water, sanitation and hygiene: Annual report 2019. World Health Organization.
- WHO and UNICEF. (2005). Water for life: Making it happen. World Health Organization.
- Wooldridge, J. (2002). Econometric analysis of cross section and panel data. The MIT Press.
- World Bank. (2020). http://wdi.worldbank.org/table/2.18. Washington , D.C. :The World Bank
Appendices
Table A1. Distribution of child deprivations by education, marital status and religion of the mother (%)
Table A2. Descriptive Statistics for children
Table A3. Correlation coefficients between dimensions of well-being for children aged 0-5 years