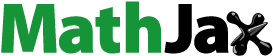
Abstract
Personnel-adjusted added value (PAV) is an index to measure human resource management for internal purposes. In this study, the possibilities and limitations of PAV using external estimates are investigated. To measure the effectiveness of PAV, it is compared with the general added value. This proves the effect of the personnel adjustment. The relationship between future performance and PAV (and added value), including other control variables, are statistically analysed by hierarchical linear modelling. The essence of PAV is to separate the basic labour costs from the company-specific personnel costs. To calculate PAV from outside an enterprise, the basic labour costs should be estimated. For this purpose, in addition to financial data, this study uses survey data on recruitment and employment in Japan. Statistical analysis is applied to the 2008–2016 longitudinal company data of 569 Japanese companies. We find that PAV, a management indicator that includes the adequacy of human resource management, is a useful factor related to future performance.
PUBLIC INTEREST STATEMENT
This study focuses on the effects of PAV, which is an evaluation index that includes the contribution of corporate human capital.
PAV is an index created based on added value. The effect of PAV is measured by analyzing multi-year data using hierarchical linear modeling while comparing PAV with added value.
As a result, it is shown that PAV is more closely related to future profits than added value, and is effective for internal management of companies and estimation from the outside.
1. Introduction
The Organisation for Economic Co-operation and Development notes that labour productivity growth has been weak since the financial crisis of 2007–2008, also known as the global financial crisis and the 2008 financial crisis in its economic surveys and country surveillance in 2018 and 2019. It indicates that labour productivity growth in recent years has been subdued even in Germany and suggests that securing higher living standards requires a revival in labour productivity in the UK and that raising productivity is important to offset the impact of falling labour inputs in Japan (Organisation for Economic Co-operation and Development, Citation2019, pp. 15-18, 58). The Japanese government has also recognised that improving labour productivity is important to maintain and improve the economy in Japan, where the labour force is declining year by year (Japan Ministry of Economy Trade and Industry, Citation2017).
Labour productivity of each company is calculated by added value (AV) divided by the number of employees. AV is defined as the value that each company produces and adds to the products and services generated from various company activities.
There are two methods for calculating AV: subtractive and additive. One is the subtractive method, where the amount paid for the value generated by another company (external cost) is deducted from sales. The other is the summation method, which reflects the sum of the benefits distributed (Haller & Stolowy, Citation1998). In both calculation methods, all expenditures on human capital are included in AV.
The method of calculating AV does not apply the concept of external cost to human capital. Thus, the AV does not change even if the human capital is acquired from outside or trained within the company. However, the quality of human capital has become even more important in a society with a declining workforce. The strategic importance of training to an organization’s human capital development efforts has been asserted (Salas & Cannon-Bowers, Citation2001). The importance of training is especially relevant in today’s rapidly changing era, where organizations spend a lot of time and money improving their employees’ knowledge, skills and abilities to stay competitive (Blume et al., Citation2010). The companies that can provide in-house training to increase the quality of their workforce should be evaluated better than the companies that acquire human resources from outside. Therefore, Personnel-adjusted added value (PAV) is proposed as an indicator that applies the concept of external cost to expenditure on human capital to evaluate the human capital development of companies (Iwamoto & Takahashi, Citation2015).
In the field of training evaluation, by subtracting the salary before receiving training from the salary (including bonuses) after receiving training, it is used as an alternative index of employee performance (e.g., Breuer & Kampkötter, Citation2013). Extending this from individual employees to the corporate level, by subtracting the basic salary from the payment, additional salary is calculated and is used as an alternative index for the results of training. This fact supports the adaptation of external cost concepts to human capital. The essence of PAV is to separate the basic labour costs (BLC) from the company-specific personnel cost.
PAV is proposed as an index to measure human capital management for internal purposes. It is intended to quantitatively measure the outcome of human resource management within an enterprise to evaluate the skill of human capital management. Previous research discussed its principle and structure; however, it did not show the quantitative effectiveness of increasing PAV sufficiently.
Therefore, in this study, the possibilities and limitations of PAV using external estimates are investigated.
We assume that a company having strong human capital can make greater profits in the future than other companies. Therefore, to show the validity and effectiveness of PAV, we examine how PAV affects future profit, which can indicate whether it captures features of human capital. To measure the effect of PAV in more detail, it is compared with the general AV. This proves the effect of the personnel adjustment.
The concept of BLC is introduced in the definition of PAV, where personnel expenses exceeding BLC are considered the subject of human resource management. The key to measuring PAV is to calculate BLC. This study attempts to estimate BLC more precisely than previous research (Iwamoto & Takahashi, Citation2015). For this purpose, in addition to financial data, survey data on recruitment and employment are used.
The following paper is structured as follows: Section 2 reviews the literature related to the PAV and AV; Section 3 describes the research methods, sample, and variables; Section 4 presents the empirical analysis results and a discussion; and finally, Section 5 concludes.
2. Literature review and hypotheses development
2.1. Added value
AV is a measure of economic performance of an economic entity with the traditional roots of economics and used to measure the creation of national wealth, the gross domestic product (Haller et al., Citation2018; Schreuder, Citation1979).
However, one of AV’s major features is that it also has been used for economics, national accounting, financial accounting managerial accounting, and business management. AV has also been discussed and used as a useful economic and performance indicator in these areas (Haller & Stolowy, Citation1998).
Transferred to a company, AV is a measure of its residual return generated through the utilisation of its productive capacity e.g., labour and capital in the broad classical sense. Thus, AV can be described as the value created by corporate activities (Burchell et al., Citation1985).
Another aspect of corporate-level AV is the pre-distribution resources created by the company during the period and distributed to each stakeholder (Haller et al., Citation2018). Top managers distribute AV to help companies improve future performance. Therefore, AV affects future corporate performance.
Although this study does not focus on it, value-added distribution information to corporate stakeholders is considered important from the perspective of corporate social responsibility, and its disclosure is discussed in value-added accounting in social accounting (Burchell et al., Citation1985; Haller et al., Citation2018).
A concept that applies AV to business management and suggests that value-added affects future performance is the value-added intellectual coefficient (VAIC) method (Pulic, Citation2000).
The VAIC method is an example of using AV in an area of intellectual capital. Intellectual capital has many definitions (Poh et al., Citation2018). One definition of intellectual capital is knowledge and the knowing capability of a social collective, such as an organisation, intellectual community, or professional practice (Nahapiet & Ghoshal, Citation1998).
VAIC is a method of measuring contributions using components of intellectual capital, taking AV as a result of intellectual capital management. For example, Ulum et al. (Citation2017) evaluates the effects of intangible assets using modified VAIC and suggests that modified VAIC is a determinant of future performance.
There are many studies that focus on the components of intellectual capital, and there are differences; however, it is common to include the human capital that this study focuses on (Halim, Citation2010; Petty & Guthrie, Citation2000; Saint‐Onge, Citation1996).
PAV, like VAIC, is one of the ways to adapt AV for business management purposes. AV is also used in business management and is suggested to affect future performance.
2.2. Personnel-adjusted added value
As mentioned above, PAV is an adjusted AV. Therefore, PAV is not an index for measuring and capitalising human assets, but an index for measuring company management results, including the results of human capital management, where the adjustment is Personnel adjustment.
PAV is proposed as an indicator in which the concept of external cost is applied to expenditure on human capital to evaluate the human capital development of companies.
PAV is a concept that includes only the part of the labour cost that is the result of the company-specific management, not the whole labour cost (Iwamoto & Takahashi, Citation2015). Assume that in the labour market, employees get wages commensurate with their abilities and attitudes. As a result, the wages paid upon joining the company can be viewed as the result of training external human resources, rather than the result of company-specific human resources management. Labour costs that do not depend on company-specific management are called BLC. The wage increases after joining the company represents an evaluation of the increase in ability and attitude enabled by company-specific management.
Resource based view (RBV) is the concept that a company’s unique and irreplaceable bundle of resources is the basis of a company’s sustainable competitive advantage (Barney, Citation1991; Wernerfelt, Citation1984). Employees at the time of hiring can be replaced, but company-specific management makes it difficult to be replaced. Therefore, by subtracting the BLC, which represents the substitutable workforce, PAV expresses the impact of human capital, which is the basis of competitive advantage.
Zaitouni et al. (Citation2020) shows that continuous training (in other words, company-specific management) is a source of competitive advantage. Measuring training outcomes in increments of payments supports the PAV concept (Breuer & Kampkötter, Citation2013). Company-specific management training is also drawing attention from the perspective of sustainability (Lee et al., Citation2019). Furthermore, Singh et al. (Citation2019) empirically shows that sustainable training leads to a company’s competitive advantage. There is an increasing need to measure company-specific management training results.
This study separates labour costs into BLC and additional labour costs (under company-specific management).
PAV is given as follows:
The difference between personnel expenses and labour costs under company-specific management is the BLC. BLC can be calculated from its own company data in the case of internal management, but estimation is required when calculating PAV from external factors. Estimate BLC from the labour costs of new employees, especially unskilled workers, to calculate PAV from outside of the company.
Previous research on PAV focuses on methods of increasing PAV (Iwamoto & Takahashi, Citation2015). It is important for it to be effective; however, this has not yet been demonstrated quantitatively.
2.3. Relevance between human capital management and future profits
Human capital also has many definitions. A commonly used definition is “the knowledge that employees take with them when they leave the firm” (MERITUM: MEasuRing Intangibles To Understand and improve innovation Management, Citation2002). Due to unit-level influences, replacing human capital is particularly difficult and/or tends to be expensive to imitate due to unit-level influences (Nyberg et al., Citation2018). Thus, human capital has been considered an important factor in human resource theory and intangibles research (e.g., Becker, Citation1964; Edvinsson & Malone, Citation1997; Martinez et al., Citation2017).
Human capital management, among many business activities, is more related to investment management for future performance than current performance (Patrick et al., Citation2001; Wakasugi, Citation1979). Human capital produce performance results through company-specific performance behavior (Ployhart, Citation2021). Therefore, company-specific management is important, and research on the relationship between management and business performance is also being conducted.
For example, (Shin & Konrad, Citation2017) uses a model with a time lag between personnel practices and performance to show that human capital affects future performance. Vomberg et al. (Citation2015) argue that human capital and brand equity are the most important factors of corporate capital and use a psychometric survey to confirm that the interaction of the two capitals affects corporate value. In addition, Iwamoto & Suzuki (Citation2019) using Japanese corporate data, suggests that human capital affects business performance through intermediating effects.
PAV is an indicator supposed to include the contribution of human capital management. Therefore, this study assumes that a high (low) PAV indicates that management is appropriate (inappropriate) and should lead to an increase (decrease) in future profit. In addition, because Personnel adjustment is effective, PAV explains variations in future profit more than AV; it is assumed
H1: A high (low) PAV leads to an increase (decrease) in future profit level.
H2: PAV explains variations in future profit more than AV.
To verify effectiveness of PAV, this study quantitatively analyses the mutual relevance of PAV (or AV) and future profits.
3. Research design and analytical methods
Hierarchical linear modelling (HLM) is performed to test the hypothesis (Raudenbush & Bryk, Citation2002). The analytical approach is determined with reference to previous studies that conducted HLM (Doering et al., Citation2019; Lo & Yeung, Citation2018; Yang & Shyu., Citation2019).
This study creates the following models: Model 1: NULL model and basic model.
Model 2: PAV added to the basic model (PAV model).
Model 3: AV added to the basic model (AV model).
By comparing the basic model and the PAV model, the effectiveness of PAV is verified. By comparing the PAV model and the AV model, the effect of Personnel adjustment is verified.
3.1. Hierarchical linear modelling
PAV can be used as an internal management index. To measure the effect, it is more accurate to measure not only the inter-company-level effect but also the company-level effect. Therefore, the analysis uses longitudinal data instead of cross-sectional data.
HLM explicitly models both individual and between level residuals. This recognizes autocorrelation within the individual company level. In addition, it models the variances of both the individual and between level as well as investigate the influence of between level units on within level outcomes while maintaining the appropriate level of analysis (Hofmann, Citation1997). The parameters of the model are estimated by the maximum likelihood estimation method. Concerns about aggregation bias and poor statistical precision are reduced or eliminated (Bloom & Milkovich, Citation1998). HLM can account for correlations and heterogeneous variances; thus, it overcomes the statistical issues that arise from nested organisational data (Hofmann, Citation1997).
Models with explanatory variables (2 and 3 models) use a common three-step method of HLM analysis and fitting, including assessment of fixed and random effects (Raudenbush & Bryk, Citation2002).
This study first specifies a NULL model that has no predictor variable and has only random intercepts in each company level. The basic models that include the control variables are then specified. Subsequently, a fixed slope model including PAV or AV, which are firm-level predictor variables as an explanatory variable, is designated having no random slope (2 F and 3 F).
Next, a random model (2 R and 3 R model) obtained by adding a random slope assumption to the 2 F and 3 F models is specified. When PAV or AV is effective in the F and R models, it is also important to investigate whether the effect is at the between-company or within-company levels. Thus, a model (2 C and 3 C), which uses a group mean centring on PAV or AV is specified.
Finally, when both the group mean and the difference are significant in the C model, it is not possible to determine whether the PAV and the AV is more effective in each of the means and the difference. Therefore, between models (2B and 3B) using only the group mean, and the difference models (2 W and 3 W) only the difference is used. The validity of explanatory variables and the random slope assumption is evaluated by comparing the fitness of models.
Akaike information criterion (AIC) and Bayesian information criterion (BIC) are used as indices indicating the goodness of model fitting (Bozdogan, Citation1987; Zucchini, Citation2000; Lott & Antony, Citation2012, pp. 32-34). When comparing models by AIC, the model with the lowest AIC is the best model. Similarly, the smaller the BIC, the better the model fit. AIC and BIC are represented by following equation (Zucchini, Citation2000).
refers to the likelihood under the fitted model.
is sample size and
is the number of parameters.
The guidelines for comparing the model and BIC indicate that the change in BIC can be interpreted as weak evidence if it ranges between 0–2, positive evidence if it ranges between 2–6, strong evidence if it ranges between 6–10, and very strong evidence if it is more than 10 (Raftery, Citation1995).
As PAV is related to human capital, it is necessary to verify its effects in the medium term. With reference to the current study, which examines the effects in the medium term, all models use an objective variable with a 1–4-year lag to alleviate the problem of reverse causality (Bao & Bao, Citation1996; Shin & Konrad, Citation2017).
The ‘lme4ʹ package of R is used for analysis. All parameters were estimated with a full maximum likelihood approach, allowing the fit of the model and comparison with different sets of variables.
3.2. NULL model and basic model
The NULL model includes future performance as a dependent variable with values for company and measurement year
. The NULL model has no predictors.
Return on assets (ROA) is a commonly used measure of corporate performance in previous studies, including those using HLM (Karniouchina et al., Citation2013; McGahan & Porter, Citation1997; Yang et al., Citation2019).
The formula for future profit is shown below.
The NULL model is represented by the following equation.
Mixed del:
shows the future performance of company
in year
.
is the future performance average of company
, and is year
’s measurement random error of company
. The total company mean intercept
is constant for all companies; its error term
can vary, defining the deviation of each company mean.
By comparing the fitness of the NULL model with the fitness of other models, this study verifies whether the changes added to the model are more consistent with the data.
Because the data in this study are longitudinal data, which are multi-year data of the same companies, it is obvious that there is a correlation with the data within the company. However, the NULL model is used to confirm that the use of a hierarchical linear model is appropriate. If there is a correlation on data within the company, it is necessary to use a hierarchical linear model that can process this appropriately. The intraclass correlation (ICC) is used to confirm the correlation within the company (Shrout & Fleiss, Citation1979).
Where, ICC is calculated by EquationEquation 4
(4)
(4) .
The possible inflation of the type I error can exceed 30% for groups with more than 50 firms even assuming a relatively low ICC of 0.05, when using a non-hierarchical regression model. If the ICC value is large, it is necessary to use a method that considers intraclass correlation, such as a hierarchical linear model (Kreft & Leeuw, Citation1998, pp. 9, 10).
The basic model is obtained by adding a control variable to the NULL model. Industry dummy and year dummy are used as control variables. The basic model is represented by the following equations.
Mixed model:
indicates the fixed effect of
and
indicates the fixed effect of
.
3.3. PAV model and AV model
The PAV (AV) model is obtained by adding PAV (AV) to the basic model. The fixed slope models (2 F and 3 F) do not include the random effect of PAV (AV), while the random slope models (2 R and 3 R) do.
All PAV and AV are standardised using total assets to eliminate the effect of scale.
The F models and R models are represented by the following equation.
2 F model and 3 F model:
Mixed model:
2 R model or 3 R model:
Mixed model:
PAV and AV are divided into the mean and their differences in each company and used for the centring models (2 C and 3 C). In the centring model, at the company level, the effect of the difference of the PAV and AV from the company mean on the future profit is verified. In addition, the mean within each company is also added to the centring model as an explanatory variable. This verifies whether an increase in the intra-company mean at the between-company level will affect business performance. In addition, comparing the R models and the C models suggests whether the absolute value, the average, or the difference will have a greater effect on future business performance in the
2 C model or 3 C model:
Mixed model:
and
are the mean of PAV and AV for company
,
and
represent the difference between each company’s mean and the corresponding year
‘s PAV and AV.
For the within-company models (2 W and 3 W), only PAV (d) or AV (d), which is the difference of the PAV from each company mean, and for the between-company models (2B and 3B), only the PAV (m) or AV (m), which is each company’s average, is added to the basic model as an explanatory variable. The W and B models are analysed only for the lag-year model for which both the mean and the difference are significant in C models. This is because it is not possible to determine whether PAV or AV will have a greater effect on future performance in either the average or the difference or both, using only the C model.
Estimation of BLC is required when calculating PAV from external factors. In Japan, most companies recruit new graduates simultaneously. If the proportion of mid-career recruitment is low and most employees have been working at the company since they were new graduates, the evaluation before employees received company-specific management would be consistent with the salary of new graduates. Thus, the salary of new graduates can be treated as a BLC. Therefore, the BLC estimated from externals is defined as the starting salary multiplied by the number of employees in this study. Previous research used only the first-year salary for college graduates as the starting salary (Iwamoto & Takahashi, Citation2015). In this research, BLC were estimated more precisely considering the starting salary by graduate school and other educational background. Specifically, the proportion of employees’ academic background is estimated by using the academic background ratio of the recruiters in 2009. All PAVs and AVs are standardised using total assets to eliminate the effect of scale.
3.4. Data
The labour force continues to decrease in Japan. In addition, it is also expected to decrease significantly going forward. In a society where the labour force is declining, human capital management becomes more important (Iwamoto & Suzuki, Citation2019). Thus, Japan is suitable as an environment using PAV. Therefore, it is suggested that Japan is suitable for the environment using PAV but changes according to the same environment in other countries.
According to the AV definition using the deduction method (Breitner & Anthony, Citation1916), PAV is given as follows:
PAV = Sales - Selling and administrative expenses - Total production costs - Goods of purchases + R&Dexpenses + Advertising expenses + Personnel expenses - Basic labor cost
Japan’s environment is by no means special. For example, in China and South Korea, it is suggested that the declining the workforce will be faster in the future than in Japan.
Every sample company satisfies the following conditions: it is listed on one of the Japanese stock exchanges, its data are published in the Employment Quarterly Report 2011 by TOYO-KEIZAI, and it discloses the initial salary of college graduates (Toyo-keizai, Citation2011). The total is 569 companies, including 287 manufacturers and 282 non-manufacturers. The detailed list of industries is presented in .
Table 1. Industry type of target companies
Financial data are taken from the Nikkei NEEDS database from 2008 to 2016. The data source questionnaire published in the Employment Quarterly Report 2011 was conducted in 2009. Owing to changes in the financial statement disclosure system in Japan, acquisition of AV under the same definition can only be obtained prior to 2012. Therefore, PAV and AV from 2009 to 2012 are used. For this reason, for example, the 1-year lag R models use 2009–2012 PAV or AV and 2010–2013 corporate performance data created from the 2009 Employment Quarterly Report and the 2008–2013 Nikkei NEEDS database.
4. Results and discussions
The descriptive statistics of the indicators used in the analysis are shown in . The sample comprises 287 manufacturers and 282 non-manufacturers. As we analyse four years of data at once, the total sample for all models comprises 2,276 observations.
Table 2. Descriptive statistics of indicators
4.1. NULL models and basic models
shows the results of the NULL models for each of the 1–4-year lags.
Table 3. HLM results of NULL models
The gross corporate average (symbol) of future profit of the NULL model is gradually increasing to 0.045, 0.050, 0.052, 0.055 from the 1-year lag in order. The variance of the company average is 0.037, 0.035, 0.035, 0.035 in order from the 1-year lag, and there is almost no change.
The AIC and BIC of the NULL model can be used as criteria for confirming the validity of other models. The ICCs calculated using the NULL model are 0.732, 0.731, 0.720, and 0.738 in order from the 1-year lag. It is suggested that methods that consider the intraclass correlation, such as the hierarchical linear model, should be used. ICC is an index for confirming the correlation of data that is assumed to be hierarchical.
Although ICC can be used for longitudinal data, the correlation between other years’ data of the same company is considered to be higher than that of general hierarchical data (e.g., hierarchical relationship between schools and students), and it is natural that the data greatly exceed the threshold.
shows the basic models results for each of the 1–4-year lags.
Table 4. HLM results of basic models
In , the AIC and BIC are lower in the basic model than in the NULL model (BIC difference exceeds 10). This result indicates that the basic model is more effective than the NULL model. Therefore, a PAV and AV models are created using the basic model’s control variables.
The industry dummy became less significant as the time lag increased. The coefficient indicates that the non-manufacturing industry has a higher ROA than the manufacturing industry. This result is consistent with the fact that the non-manufacturing industry is slightly more advantageous in ROA because there are many industries that require fewer assets, such as properties, than the manufacturing industry. This study uses the Industry dummy to absorb industry-specific effects.
Almost all the year dummies were significant at the 1% level (the 2010 dummy was significant at the 1.6% level in the 3-year-lag model but not significant in the 2-year-lag model). This result indicates that the environment of companies, including economic conditions, is changing year by year. We use this variable to absorb the effects of the corporate environment (e.g., economy). The 2010 dummy in the 2-year-lag model shows that the difference between the ROA averages in 2009–2011 and 2010–2012 is small.
The year dummy absorbs the effects of the corporate environment on a yearly basis.
4.2. PAV models
shows the results of the 2 F models for each of the 1–4-year-lag models.
Table 5. HLM results of fixed PAV (2F) models
In , the AIC and BIC are lower in the 2 F model than in the basic model (BIC difference exceeds 10). This result shows that the 2 F model is more effective than the 1b: basic model. In addition, PAVs are significant at the 0.1% level in all models, and the coefficients of PAV are positive values (in order from a 1-year lag, 0.136, 0.099, 0.089, 0.079). These results indicate PAVs have additional information relevance to future profits. Thus, H1, which states that “A high (low) PAV leads to an increase (decrease) in future profit level”, is supported.
shows the results of the 2 R models for each of the 1–4-year-lag models.
Table 6. HLM results of random PAV (2R) models
In , both AIC and BIC are lower in the 2 R model than in the 2 F (BIC difference exceeds 10).
This result indicates that the 2 R models are more effective than the 2 F models. The random effect of PAV observed means that the impact of PAV varies by company. This variation may be because of differences in the importance of human capital depending on the type of business. PAV is also significant at the 0.1% level in all 2 R models, and all PAV coefficients are positive values. This result also shows that PAVs have additional information relevance to future profits and thus supports H1: A high (low) PAV leads to an increase (decrease) in future profit levels.
shows the results of the 2 C models for each of the 1–4-year-lag models.
Table 7. HLM results of centred PAV (2C) models
The AIC and BIC of the 2 C model are lower than the 2 R model only in the 3-year-lag and 4-year-lag models. The results show that the PAV mean is more suitable for the medium- to long-term (3 years or longer) lag model, and that the originally PAV is more suitable for the short-term (2 years or less) model.
In 2 C models, the coefficients of the PAV mean are positive values of 0.142, 0.134, 0.132, 0.126 in order from the 1-year lag. Like the 2 F and 2 R results, the results of 2 C models support the proposition that PAV has additional information on future corporate performance.
The PAV mean represents the influence of the quality of the average quality of a company’s human capital and ongoing personnel practices. The fact that PAV mean is effective in the medium to long term indicates that company-specific human capital management is related to future profits at the inter-company level. In other words, it suggests that human capital can be the basis of a sustainable competitive advantage over other companies.
The PAV difference is significant only for the 1-year-lag model, and the coefficient is 0.113. It suggests that increasing PAV will improve next year’s corporate performance, not only at the between-company level but also at the within-company level.
Based on this result, if the target is set using the past in the company’s PAV and the target is achieved using the PAV as a management index of the manager who is not at the top level, the next term’s business performance can be improved.
The effect of PAV difference on business performance appears in the short term (1 year), and there is no medium- to long-term lag. This is because when taking the difference in PAV, the influence of human capital that affect the medium to long term, such as personnel practices and established training, is absorbed on mean PAV. Since only the variation in the quality of new attempts and measures in each year appeared as the PAV difference, it is considered that the effect appeared in the short-term lag.
The results in Section 4.2 support H1 and suggest that PAV is effective when used as an internal management index.
4.3. AV models
shows the results of the 3 F models for each of the 1–4-year-lag models.
Table 8. HLM results of fixed AV (3F) models
In , the AIC and BIC are lower for the 3 F models than for the basic model (BIC difference exceeds 10). This result indicates that the 3 F model is more effective than the basic model. In addition, AV is significant at the 0.1% level in all models, and all coefficients of AV are also positive values.
This result supports AVs having additional information relevance to future profits, as shown in previous studies.
shows the results of the 3 R models for each of the 1–4-year-lag models.
Table 9. HLM results of random AV (3R) models
In , the AIC and BIC are lower in the 3 R than in the 3 F model. This result indicates that the 3 R models are more effective than the 3 F model.
The fact that the random effect of AV is recognised means that there is variation in the effect of AV for each company.
shows the analysis results of the 3 C models for each of the 1–4-year lags.
Table 10. HLM results of centred AV (3C) models
The AIC and BIC of the 3 C models are lower than the 3 R model only in the 3-year-lag and 4-year-lag models. Similar to PAV, this result indicates that in the medium to long term (3 years or more), the AV mean is more suitable to use, and in the short term (2 years or less), the original AV is more suitable.
The AV difference is significant only for the 1-year-lag model, and the coefficient is 0.113. This result indicates that AV can be used for management not only between companies but also within companies.
4.4. Comparing PAV and AV models
shows the model with the smallest AIC and BIC among the same lag-year models and the AIC and BIC of the smallest model.
Table 11. The smallest AIC and BIC models in each lag year
The same model is the minimum AIC and BIC for both PAV and AV. The smallest model is the random model for the lag of 1 and 2 years, and the centring model for the lag of 3 and 4 years.
Comparing the minimum AIC and BIC of the PAV and the AV models, the AIC and BIC of the PAV is found to be smaller than those of the AV model in all lag-year data. The model using PAV fits the data better than the model using AV.
This result suggests that PAV has more information relevance than general AV to future profits. It thus supports H2, that PAV explains variations in future profit more than AV.
The impact on human capital management is known to be short- and medium-term. Therefore, even if the time lag is changed to 1–4 years, PAV is more suitable for the model than AV. In addition, this result suggests that human capital leads to a sustainable competitive advantage. PAV may be effective when creating a future performance forecast model considering human capital management. When creating a forecast model, it may be effective to use the PAV itself in the short term and the PAV mean in the medium term.
Since both the mean and the difference are significant in the 1-year-lag model for both PAV and AV, the analysis is performed using the B and W models. shows the results for B and W models.
Table 12. HLM results of the within and between (2B, W and 3B, W) models
The comparison between the 2B and 3B models shows whether the model using the mean PAV or the mean AV has a smaller deviation from the data. The AIC and BIC of the 2B model is −9721.7 and
-9675.8, and that of the 3B model is −9677.3 and −9631.5. Both AIC and BIC are smaller for the PAV model. There is very strong evidence that the PAV model fits the model better than the AV model, because the BIC differs by more than 10.
Top-level managers and shareholders who need to make comparisons with outside companies, should place more emphasis on PAVs than AVs, as the results support the hypothesis that PAVs will explain their future performance.
The comparison between the 2 W and 3 W models shows whether the model using the PAV difference, or the AV difference has a smaller deviation from the data. The AIC and BIC of the 2 W model is −9717.4, −9660.1, and the 3 W model is −9715.4, −9658.1.
Both AIC and BIC are a little smaller for the PAV model. There is positive evidence that the PAV model fits the model better than the AV model because there is also a difference of more than 2 for BIC.
The results of the W models show that using PAV as a management index for managers who manage the company internally rather than comparing with other companies can have a greater impact on future performance than using AV.
It is shown that the model using PAV is more suitable for the model which includes only the mean and the difference as explanatory variables.
When the effects of PAV and AV are divided into the between-company level and the within-company level and compared, PAV is more effective than AV in both cases. This suggests that PAV is more effective than AV for any level of manager.
PAV is an index that applies the concept of external cost to human capital as well. It measures the contribution of human capital, which is the source of a company’s competitive advantage, more precisely. Therefore, it is interpreted that PAV is more closely related to future performance than AV at both the between-company level and the within-company level.
5. Summary and conclusion
5.1. Contribution
To verify the effectiveness of PAV, the relationship between future profit and PAV is examined.
By using HLM for longitudinal data, both the effects of PAV within a company and between companies are discussed.
Analyses are performed using a more refined BLC estimation than in previous studies. Both H1 and H2 are supported by empirical analysis. Thus, PAV estimated from an outside perspective implies that PAV is useful as a key performance indicator for internal control. PAV has a stronger relevance to future profits than AV, and reflects the effectiveness of personnel adjustment.
By using the time lag, the PAV effect is verified to affect future performance, and shown to be effective for at least four years.
5.2. Business implications
This study suggests that companies should use PAV as a performance indicator, which is its initial objective, rather than AV in internal management.
PAV is a useful metric for investors and top managers who need to make comparisons with other companies because improving PAV can have a greater impact on future business results than AV for the medium term. Furthermore, PAV evaluates human resource development rather than focusing on acquiring human resources from outside; therefore, it may be possible to accelerate human resource development within the company.
It is difficult for some departments to directly contribute to profits. However, PAV can directly contribute by developing human resources. It is also possible to improve next term’s performance by boosting the company’s PAV without looking at other companies.
In addition, calculation of PAV is not costly because any company has its own payment data.
As a method of using PAV from an external perspective, if a corporate performance prediction model that uses AV or has AV as a candidate for an index, is used separately from the model of this research, using PAV may provide more accurate forecasts than using AV. Although using PAV requires some costs to collect the information, its use is effective if the user wishes to improve accuracy.
5.3. Further study
While this study uses manufacturing/non-manufacturing categories, there is a possibility of obtaining more sophisticated results by applying or adding other categories focused on human resources. There are two kinds of businesses: one should focus on human resources while the other should not. Therefore, the result may be changed under alternative classifications and analyses.
Research involving human participants and/or animals
This study does not involve any human participants or animals.
Disclosure statement
The author(s) declare that they have no conflict of interests.
Additional information
Funding
Notes on contributors

Hiroki Iwamoto
Hiroki Iwamoto studied and received a bachelor’s and master’s degree at department of industrial and systems engineering, Keio university in Japan.
He is now working as a research associate at the department of industrial & management systems engineering at Waseda university in Japan.
His research interests include corporate social responsibility, including human capital in management systems.

Hideo Suzuki
Hideo Suzuki works as a professor at the department of Industrial and Systems Engineering, Faculty of Science and Technology, Keio university in Japan.
His research area is applied statistics, quality management, and marketing research.
References
- Bao, B. H., & Bao, D. H. (1996). The time series behavior and predictive-ability results of annual value added data. Journal of Business Finance and Accounting, 23(3), 449–21. https://doi.org/10.1111/j.1468-5957.1996.tb01132.x
- Barney, J. (1991). Firm resources and sustained competitive advantage. Journal of Management, 17(1), 99–120. https://doi.org/10.1177/014920639101700108
- Becker, G. S. (1964). Human capital: A theoretical and empirical analysis, with special reference to education. National Bureau of Economic Research.
- Bloom, M., & Milkovich, G. T. (1998). Relationships among risk, incentive pay, and organizational performance. Academy of Management Journal, 41(3), 283–297. https://doi.org/10.2307/256908
- Blume, B. D., Ford, J. K., Baldwin, T. T., & Huang, J. L. (2010). Transfer of training: A meta-analytic review. Journal of Management, 36(4), 1065–1105. https://doi.org/10.1177/0149206309352880
- Bozdogan, H. (1987). Model selection and Akaike’s Information Criterion (AIC): The general theory and its analytical extensions. Psychometrika, 52(3), 345–370. https://doi.org/10.1007/BF02294361
- Breitner, L. P., & Anthony, R. N. (1916). Core concepts of accounting. Pearson.
- Breuer, K., & Kampkötter, P. (2013). Determinants and effects of intra-firm trainings: Evidence from a large German company. Journal of Business Economics, 83(2), 145–169. https://doi.org/10.1007/s11573-012-0650-4
- Burchell, S., Clubb, C., & Hopwood, A. G. (1985). Accounting in its social context: Towards a history of value added in the United Kingdom. Accounting, Organizations and Society, 10(4), 381–413. https://doi.org/10.1016/0361-3682(85)90002-9
- Doering, T., De Jong, J., Suresh, N., & Mastrocinque, E. (2019). Performance effects of supply chain integration: The relative impacts of two competing national culture frameworks. Cogent Business and Management, 6(1), 1610213. https://doi.org/10.1080/23311975.2019.1610213
- Edvinsson, L., & Malone, M. S. (1997). Intellectual capital: Realizing your company’s true value by finding its hidden brainpower. HarperBusiness.
- Martinez, M.G., Zouaghi, F., & Garcia,S. M. (2017). Capturing value from alliance portfolio diversity: The mediating role of R and D human capital in high and low tech industries. Technovation, 59, 55–67. https://doi.org/10.1016/j.technovation.2016.06.003
- Halim, S. (2010). Statistical analysis on the intellectual capital statement. Journal of Intellectual Capital, 11(1), 61–73. https://doi.org/10.1108/14691931011013334
- Haller, A., & Stolowy, H. (1998). Value added in financial accounting a comparative study between Germany and France. Advances in International Accounting, 11(1), 23–51.
- Haller, A., van Staden, C. J., & Landis, C. (2018). Value added as part of sustainability reporting: Reporting on distributional fairness or obfuscation? Journal of Business Ethics, 152(3), 763–781. https://doi.org/10.1007/s10551-016-3338-9
- Hofmann, D. (1997). An overview of the logic and rationale of hierarchical linear models. Journal of Management, 23(6), 723–744. https://doi.org/10.1177/014920639702300602
- Iwamoto, H., & Suzuki, H. (2019). An empirical study on the relationship of corporate financial performance and human capital concerning corporate social responsibility: Applying SEM and Bayesian SEM. Cogent Business & Management, 6(1), 1656443. https://doi.org/10.1080/23311975.2019.1656443
- Iwamoto, H., & Takahashi, M. (2015). A quantitative approach to human capital management. Procedia - Social and Behavioral Sciences, 172, 112–119. https://doi.org/10.1016/j.sbspro.2015.01.343
- Japan Ministry of Economy Trade and Industry. (2017). White Paper on International Economy and Trade 2017, 512–532.
- Karniouchina, E. V., Carson, S. J., Short, J. C., & Ketchen, D. J. (2013). Extending the firm vs. industry debate: Does industry life cycle stage matter? Strategic Management Journal, 34(8), 1010–1018. https://doi.org/10.1002/smj.2042
- Kreft, I. G. G., & Leeuw, J. D. (1998). Introducing multilevel modeling. Sage.
- Lee, C. W., Wu, W., & Yang, C. F. (2019). Employees’ perceptions of training and sustainability of human resource. Sustainability, 11(17), 4622. https://doi.org/10.3390/su11174622
- Lo, C. K. Y., & Yeung, A. C. L. (2018). Quality management standards, institutionalization and organizational implications: A longitudinal analysis. International Journal of Production Economics, 200, 231–239. https://doi.org/10.1016/j.ijpe.2018.03.028
- Lott, J. L., & Antony, J. S. (2012). Multilevel modeling techniques and applications in institutional research: New directions in institutional research. John Wiley & Sons.
- McGahan, A. M., & Porter, M. E. (1997). How much does industry matter, really? Strategic Management Journal, 18(S1), 15–30. https://doi.org/10.1002/(SICI)1097-0266(199707)18:1+<15::AID-SMJ916>3.0.CO;2-1
- MERITUM: MEasuRing Intangibles To Understand and improve innovation Management. (2002). Guidelines for managing and reporting on intangibles.
- Nahapiet, J., & Ghoshal, S. (1998). Social capital, intellectual capital, and the organizational advantage. The Academy of Management Review, 23(2), 242. https://doi.org/10.5465/amr.1998.533225
- Nyberg, A., Reilly, G., Essman, S., & Rodrigues, J. (2018). Human capital resources: A call to retire settled debates and to start a few new debates. International Journal of Human Resource Management, 29(1), 68–86. https://doi.org/10.1080/09585192.2017.1381138
- Organisation for Economic Co-operation and Development. (2019). OECD compendium of productivity indicators 2019. OECD Publishing. https://doi.org/10.1787/b2774f97-en
- Patrick, M. W., Benjamin, B. D., & Scott, A. S. (2001). Human resources and the resource based view of the firm. Journal of Management, 27(6), 701–721. https://doi.org/10.1016/S0149-2063(01)00120-9
- Petty, R., & Guthrie, J. (2000, June). Intellectual capital literature review: Measurement,reporting and management. Journal of Intellectual Capital, 1(2), 155–176. https://doi.org/10.1108/14691930010348731
- Ployhart, R. E. (2021). Resources for what? Understanding performance in the resource-based view and strategic human capital resource literatures (in press). Journal of Management, 1–16. https://doi.org/10.1177/01492063211003137
- Poh, L. T., Kilicman, A., Ibrahim, S. N. I., & McMillan, D. (2018). On intellectual capital and financial performances of banks in Malaysia. Cogent Economics and Finance, 6(1), 1453574. https://doi.org/10.1080/23322039.2018.1453574
- Pulic, A. (2000). VAIC - an accounting tool for IC management. International Journal of Technology Management, 20(5–8), 702–714. https://doi.org/10.1504/IJTM.2000.002891
- Raftery, A. E. (1995). Bayesian model selection in social research. Sociological Methodology, 25, 111. https://doi.org/10.2307/271063
- Raudenbush, S. W., & Bryk, A. S. (2002). Hierarchical linear models: Applications and data analysis methods. Sage Publications.
- Saint‐Onge, H. (1996). Tacit knowledge the key to the strategic alignment of intellectual capital. Planning Review, 24(2), 10–16. https://doi.org/10.1108/eb054547
- Salas, E., & Cannon-Bowers, J. A. (2001). The science of training: A decade of progress. Annual Review of Psychology, 52(1), 471–499. https://doi.org/10.1146/annurev.psych.52.1.471
- Schreuder, H. (1979). Corporate social reporting in the Federal Republic of Germany: An overview. Accounting, Organizations and Society, 4(1–2), 109–122. https://doi.org/10.1016/0361-3682(79)90011-4
- Shin, D., & Konrad, A. M. (2017). Causality between high-performance work systems and organizational performance. Journal of Management, 43(4), 973–997. https://doi.org/10.1177/0149206314544746
- Shrout, P. E., & Fleiss, J. L. (1979). Intraclass correlations: Uses in assessing rater reliability. Psychological Bulletin, 86(2), 420–428. https://doi.org/10.1037/0033-2909.86.2.420
- Singh, S. K., Chen, J., Del Giudice, M., & El-Kassar, A. N. (2019). Environmental ethics, environmental performance, and competitive advantage: Role of environmental training. Technological Forecasting and Social Change, 146, 203–211. https://doi.org/10.1016/j.techfore.2019.05.032
- Toyo-keizai. (2011). Shushoku shiki-ho 2013.
- Ulum, I., Kharismawati, N., & Syam, D. (2017). Modified value-added intellectual coefficient (MVAIC) and traditional financial performance of Indonesian biggest companies. International Journal of Learning and Intellectual Capital, 14(3), 207–219. https://doi.org/10.1504/IJLIC.2017.086390
- Vomberg, A., Homburg, C., & Bornemann, T. (2015). Talented people and strong brands: The contribution of human capital and brand equity to firm value. Strategic Management Journal, 36(13), 2122–2131. https://doi.org/10.1002/smj.2328
- Wakasugi, A. (1979). Ningen shisan kaikei (Human asset accounting) in Japanese. Business Kyouiku Syuppansya.
- Wernerfelt, B. (1984). A resource-based view of the firm. Strategic Management Journal, 5(2), 171–180. https://doi.org/10.1002/smj.4250050207
- Yang, C. S., & Shyu, J., (2019). Do institutional investor and group, firm and time effects matter in enterprise performance in the corporate life cycle? Cogent Business and Management, 6(1), 1606473. https://doi.org/10.1080/23311975.2019.1606473
- Zaitouni, M., Harraf, A., & Kisswani, A. M. (2020). Resources for development: The relationship of HRM practices and continuous learning culture with training success. International Journal of Human Resources Development and Management, 20(1), 75–92. https://doi.org/10.1504/IJHRDM.2020.105099
- Zucchini, W. (2000). An introduction to model selection. Journal of Mathematical Psychology, 44(1), 41–61. https://doi.org/10.1006/jmps.1999.1276