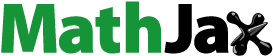
Abstract
The Ethiopian telecommunication market has been expanding slowly due to supply side constraints. However, these days, government has already announced to open market to the private operators and in this environment, the upcoming mobile telecommunication market, where 4 G service was already introduced in 2015, is becoming more substitutive and competitive. Thus, this study aim to forecast the switchers and leapfrogers demand for 3 G and 4 G mobile data services in Ethiopia. In order to do so, firstly, we investigated consumer preferences for mobile data generation services. Secondly, we estimated the mobile phone diffusion using Bass model to predict the future potential market size. Finally, we combined both models and forecasted the switchers and leapfrogers demand for 3 G and 4 G mobile data services. The result reveals that, by 2025, the 3 G and 4 G data service subscribers are forecasted to be 40 and 1.93 million, respectively, including both switchers and leapfrogers adoption. The policy implications are: (1) Service provider should adjust its service supply plan as per the forecasted demand. (2) Service provider should charge its service package strategically considering demand elasticity and finally, the service provider should make proper expansion of 4 G infrastructure since consumers are verging towards 4 G data services.
PUBLIC INTEREST STATEMENT
The role of telecommunication services and ICT has paramount impact on accelerating economic transformation of developing countries. In this study, we have applied a successive generation technology diffusion model in Ethiopian mobile data service market. We have forecasted the switchers and leapfrogers demand for 3G and 4G mobile data services using choice based multigenerational diffusion model. Various policy implications were suggested such as the need for service provider to adjust as per the demand, possibility to adjust price strategies based on mobile data services and the need for infrastructure expansion for future preferred data services.
1.. Introduction
The diffusion of innovation theory explains how, why, and at what rate new ideas and technology spread in to the society (Rogers, Citation2003). Newer technologies are continuously replacing older one through overriding better quality and mode of services compared to its previous generation. Because of successive innovations, for instance, in telecommunication industry, mobile phone services almost replaced fixed line telephone mostly in developing economies, and now internet- based services are about to replace the mobile phone analog voice services and hope will be continue in the future. However, this continues replacement is not taking place overnight, rather it takes time for innovators and imitators to adopt and diffuse it. The latest generation technology version can be diffused to the market in such a way that it may widen the market through expanding its services to previously uncovered areas, and it will also create an opportunity for older technology users to switch to the newer version so that able to benefit with the extra feature of the latest technology services (Norton & Bass, Citation1987). Because of this substitution effect, the older technology may lose its potential users in such a way that the potential customers can leapfrog to the latest version of technology and customers who have been using the older generation service can able to switch to the latest generation services (Norton & Bass, Citation1987). As the latest version of generation introduced to the market within short period interval, the earlier technologies continue to diffuse to the potential buyers while the substitution process continues towards the newer technology. Hence, at the early stage the demand for older technology may also increase as a substitution simultaneously occurs.
Pioneer diffusion model of new product in marketing was developed by Bass (Citation1969) who focused on durable, single product diffusion cases without considering any driving forces of diffusion (Bass, Citation1969). However, since then many scholars have been improved the model and studied on the diffusion pattern of successive generation such as (Norton & Bass, Citation1987). Mahajan and Muller (Citation1996) have developed extension of Bass model to consider the diffusion and substitution pattern simultaneously for each successive generation technology services. Speece and Maclachlan (Citation1995) developed a model-incorporating price as driving force that means as explanatory variable for diffusion process. Islam and Meade (Citation1997) have worked to check the hypothesis of constancy of innovation and imitation coefficient over a successive generation technologies. Jun and Park (Citation1999) developed a model that incorporates choice and diffusion effects to capture simultaneously the substitution and diffusion process for each successive generation of technologies. (Danaher et al., Citation2001) have developed model that incorporates first time sales and time interval-based renewals including marketing mix. Jiang and Jain (Citation2012) proposed two generation product diffusion with explicit diffusion of new purchaser and upgraders by extending work of Norton Bass model. Therefore, the purpose of this paper is to forecast the switchers and leapfrogers demand for 3 G and 4 G mobile telecommunication services in Ethiopia incorporating the marketing elements using the extended generalized Norton Bass model.
2. Review of previous models
2.1. Review of the bass model
Bass diffusion model is the pioneer and widely used model in marketing literature (Radas, Citation2006). According to Bass (Citation1969), the probability of a current purchase linearly depends on the numbers of prior purchasers. More precisely the probability that a given person adopt a new product at time t, provided that he has not adopted yet, is represented by
Where, f(t) is the probability of adoption at time t and F(t) is a fraction of potential, which has adopted by time t, p is the innovation coefficient and q is the imitation coefficient. Adoption of a product is made by the role of innovators and imitators, where the role of innovators is greater at the beginning but diminishing monotonically with time whereas that of imitation effect will increase overtime. The rate of adopters at some point in time is the number of people who have not adopted yet times their probability to purchase the products/services (Bass et al., Citation1994).
Assuming F (0) = 0, the solution to the differential Equationequation (2)(2)
(2) above is;
The density function of Equationequation (3)(3)
(3) above is;
Hence according to the Bass model, the adoption rate for a single durable product in the market is estimated as Equationequation (4)(4)
(4) above. But in real practice, there could be multiple generations of products in the market and hence to improve the original Bass model, Norton and Bass (Citation1987) developed sales diffusion model for multiple generation products, which consists both diffusion and substitution over generations.
2.2. Review of the norton bass model
The Norton Bass model developed in (Norton & Bass, Citation1987) includes both substitution and diffusion effects for multiple generation of products or services. The functional form to represent the diffusion process was proposed by Bass (Citation1969) as in the above Equationequation (3)(3)
(3) and in the case of single generation with no successor, sales would be;
Where is sale, F (t) is cumulative distribution of the adoption rate and m is the upper limit of potential adopters. When the second generation is introduced in to the market then, the sales rate (Bass, Citation1969) is:
For the first generation product;
And the second generation product;
Where, ith is the index of particular generation,
and
. Note that
is the time at which second generation is introduced, and
, qi and pi are imitation and innovation coefficient for ith generation, respectively.
The simultaneous model of Equationequation (6)(6)
(6) and (Equation7
(7)
(7) ) captures both adoption and substitution effects. And for a multiple number of generations, this model can be extended to the nth generation, and the sales of earlier generation will approach to zero as more successive generation is introduced, because of substitution effect.
However, the Norton Bass model assumes the innovation coefficient (pi) and imitation coefficients (qi) are constant over successive generation, which is a strong assumption. It also does not differentiate the leapfrogers adoption from the switchers’ adoption in successive generation of product/services. In order to incorporate these differences (Jiang & Jain, Citation2012) has modified the Norton Bass model and able to propose model for leapfrogging and switching adoption diffusion.
2.3. Review of the Generalized Norton Bass(GNB) model
According to the generalized Norton Bass diffusion model, the new product generation may not immediately got accepted and diffused, rather a potential adopter may keep purchasing an old generation until they become aware of the existence of new generation and decide to adopt it, (Jiang & Jain, Citation2012). Through the process the new generation becomes more known and popular to the potential consumers. Consequently, the fraction of potential consumers/adopters who are skipping previous generation and adopting the latest generation are called leapfrogging adopters. The rate of leapfrogers will increase over time as there will be more information diffusion between leapfrogers and the remain others. In addition to leapfrogers, some adopters of previous generation product may want to switch to latest generation if the additional services obtained from latest generation surpass the cost of switching. This kind of adopters’ behavior become popular in successive generation product markets and termed as switching adopters. Like leapfrogging diffusion, the proportion of switching adoptions also increases over timeFootnote1 (Jiang & Jain, Citation2012).
The generalized Norton Bass model considered two generation case where first generation (G1) introduced in period 1 and second generation (G2) introduced in period t2 > 0. Just before G2 introduction, the diffusion of G1 follows the Bass diffusion model (Bass, Citation1969). After G2 become available, the fraction of potential of G1 who would have adopted G1, if it was the only available in the market could now leapfrog to G2 instead. The GNB assumes the proportion of leapfrogers adoption increase with time, as a result of diffusion of product (G2). That means the probability of leapfrogging from generation 1 to 2 is time varying and influenced by diffusion rate of G2 i.e. .
According to Jiang model, given the leapfrogers multiplier to G2 is, the number of leapfrogers adoption for small time interval change
can be expressed as (Jiang & Jain, Citation2012);
Hence, the instantaneous rate of leapfrogers at time t, denoted by, equals
Where is the derivative of
and represents the diffusion rate of G1 at time t. Hence, the cumulative number of leapfrogers adoption from G1 to G2 by time t is
Besides leapfrogging, switching also begins after time. Hence, switching rate to G2 generation product at time t;
And its cumulative form is
Assuming those who are already using previous generation can adopt the new generation more quickly than new first time purchaser, for instance, Apple insider Hughes (Citation2010) reports shows that 77% of new iPhone 4s purchasers were existing iPhone users, the diffusion rate is across G2 potential adopters. Hence, the rate for those who already adopts G1 (switching multiplier) to G2 is (Jiang & Jain, Citation2012);
According to Jiang and Jain (Citation2012), the switchers and leapfrogers multiplier has similarity with those adopted by Danaher et al. (Citation2001) and Jun and Park (Citation1999). In general, the Norton Bass (NB) model is credited as the pioneering multigenerational diffusion model in marketing science and the Jun and Park (Citation1999) model also credited for choice-based diffusion for successive generation model. Some of the multigenerational diffusion models and their suggested features are summarized in .
Table 1. Review of multigenerational diffusion models and their features
This research was conducted with three successive generation contexts in the case of mobile data service diffusion and filled the gap of multigenerational diffusion models considering leapfrogers and switchersFootnote2 diffusion at a time. Several studies have explored the interesting issues of the telecommunications market of Ethiopia (Negi, Citation2009; Temesgen et al., Citation2009); however, no study has been carried out to forecast the 3 G and 4 G subscribers, switchers and leapfrogers and the price elasticity for 3 G and 4 G data services. Therefore, the purpose of this paper is to fill the knowledge gap and forecast the switchers and leapfrogers demand for 3 G and 4 G mobile telecommunication services in Ethiopia incorporating the marketing element using the extended generalized Norton Bass model. In addition, the specific objectives are: (1) to empirically test the workability of the extended generalized Norton bass model (GNB) by improving a constancy assumption of innovation and imitation coefficient over successive generation diffusion of products, (2) to forecast the switchers and leapfrogers demand for 3 G and 4 G mobile telecommunication services through incorporating consumer choice behavior and (3) finally to know the price and cross price elasticity of market potential for 3 G and 4 G services.
3. Methodology
3.1. Data sources and facts on ethiopia telecommunication services
Ethiopia Telecom is the monopoly telecom operator since its establishment in 1894 and has been providing telephony and internet services. Currently, mobile service penetration is 54.8 percent and internet service users are utmost 24 percent of potential users, which is by far lower than the sub Saharan African standard, which is because of the imbalance between the demand and supply. One alternative to improve and upgrade service provision is via demand forecasting and planning accordingly. Thus, the data used for this study are the subscription rate for 2.5 G since 1999, 3 G since 2009 and 4 G data services since 2015. The data cover monthly subscription data from 1999 to 2018 collected from Ethiopia telecommunication service Provider Company (ETC).
3.2. Model
The study adopts the generalized Norton Bass model and extended to a three successive generations of mobile telecommunication services. The marketing mix is expected to have a significant role in diffusion of successive generation technologies, and hence, the study incorporates pricing variable into the leapfrogers and switchers diffusion model.
3.2.1. Leapfrogging adoption diffusion model
Before the introduction of 3 G, the diffusion of 2.5 G follows the generalized Bass model (GBM) (Bass et al., Citation1994);
Now when 3 G is introduced at time, some of the potential adopters of 2.5 G will leapfrog to 3 G. According to generalized Norton Bass model, the leapfrogging multiplier to 3 G is
since the cumulative market effort of 3 G is expected to influence the leapfrogers to 3 G as well. Hence, considering the successive generation of mobile data services such as 2.5 G, 3 G, and 4 G, the rate of leapfrogers to 3 G service for small change over time
is;
Where leapfrogers to 3 G at is time t, and it is expressed in the rate of diffusion rate as below Equationequation (14)
(14)
(14) :
By the same logic, we also derived for the leapfrogers to 4 G mobile data services as follows:
Where and
are the leapfrogers adoption rate to 3 G and 4 G data services and
are the market potential for 2.5 G and 3 G services.Footnote3 EquationEquation (15)
(15)
(15) implies potential adopters of 4 G data services those who would be willing to adopt 3 G in the absence of 4 G service plus those who already adopts 2.5 G data service but willing to leapfrog to 4 G data services multiplied by 4 G data service leapfrogers multiplier rate.
3.2.2. Switching adoption diffusion model
By the same fashion to the leapfrogging adoption diffusion model, the switchers’ adoption diffusion for the 3 G and 4 G data service is also modelled. The switchers’ multiplier for adoption to 3 G is, as explained in the generalized Norton Bass model, and hence the rate of switching from 2.5 G to 3 G data services is:
Using the Bass exponential density function, the switchers rate to 3 G services is;
Analogous to the 3 G switching adoption diffusion, we also derived for the 4 G switchers’ rate as;
Where and
are switchers adoption rate to 3 G and 4 G services and
are market potential for 2.5 G and 3 G data services, respectively.
It is well known that the marketing mix variables such as promotion and pricing can influence the diffusion of a given technologies. Hence, in this study, we have incorporated the price effect on leapfrogging and switching adoption diffusion of 3 G and 4 G data service. Suppose we specified the price function as (Bass et al., Citation1994);
Where is absolute price for each generation, t is time effect on diffusion of each generation and
is price coefficients of each generation G. Hence, considering Equationequation (19)
(19)
(19) into the leapfrogging and switching adoption diffusion model, the leapfrogers and switchers diffusion rate to 3 G and 4 G data services, taking into account price variable is as follows;
And that of switchers’ diffusion rate to 3 G and 4 G with price variables is;
3.2.3. Consumer choice for mobile data service generation
In multigenerational product diffusion, the sales pattern of new product is based on the choice made by consumer over time, according to Jun and Park (Citation1999) model. Hence, the market share for each generation can be used as a proxy to estimate the choice probability for a given generation. Suppose the choice probability for the kth generation depends on its attributes and attributes of other alternatives in the market. By considering an attributes into aggregate market share (Cooper & Nakanishi, Citation1989).
Where Sj is the market share for jth generation, X’s are attributes of the available generation services. Accordingly, considering the price of internet fee per MB, and cross price as explanatory variable, we estimated the choice probability for each generation services as follows;
By the same fashion similar to Equationequation (25)(25)
(25) , the probability (market share) for 3 G and 4 G services was also calculated. To forecast the future demand for each generation of mobile data services, it requires combining the choice probability and diffusion model. The choice probability for each mobile data communication service is estimated from Equationequation (25)
(25)
(25) above and the diffusion part is from monthly sales data. Accordingly, the consumer choice integrated diffusion models are:
Where is the subscription to ith generation data services at time t,
is the market potential at time t, and
is the previous cumulative adopters of that generation at time t-1.
3.2.4. Leapfrogers demand forecast
In order to forecast the demand for leapfrogers and switchers to 3 G and 4 G services, the choice probability for each generation is derived. For instance, Jun and Park (Citation1999) have estimated choice probability for choice-based diffusion model. Suppose is the market potential for any generation of mobile data services. Let
is the previous adopters (active in use) for total market at time t-1. Then, the total number of non-purchaser at time t-1 is
. As a result, the expected number of leapfrogers for the kth generation product is
Where is the average probability to purchase k-th generation product for the first time at time t, n is the number of generation available. Accordingly, the leapfrogers demand for 3 G data service is as follows:
The leapfrogers demand for 4 G data services, for the first time purchaser is as below Equationequation (29)(29)
(29) ;
Moreover, leapfrogers from 2.5 G service users to 4 G services is as below Equationequation (30)(30)
(30)
Where, is the leapfrogers demand for kth generation at time t, 0 implies no previous generation adopters,
is active in the use of 2.5 G generation services at time t-1.
3.2.5. Switchers demand forecast
Concerning switching adoption diffusion let and
is the number of in use (installed base) for 3 G and 2.5 G data services at time t-1, respectively. Then, the expected number of new upgraders (Zi) from generation (i to k), at time t is
Hence, the switchers demand for 3 G and 4 G data services are modelled as follows;
Where, the above Equationequation (32)(32)
(32) is switchers demand from 2.5 G data services to 3 G data services.
For switchers demand for 4 G data services is:
Hence, using the consumer preference-based diffusion of successive generation in the context of leapfrogers and switchers adoption behavior; we have estimated and tested the workability of this model.
4. Results and discussion
4.1. Extended Generalized Norton Bass (GNB) model result
By relaxing the assumption of constancy of innovation and imitation coefficient over successive generation services, we have estimated the extended GNB model for successive generation of mobile data service diffusion including marketing variable. The result shows that most of the parameters are significant in influencing the diffusion of successive generation of mobile data services except the price coefficients in the model with marketing mix. This may be because of the data was constant over certain periods and there were only three period’s data change within 48 data points. According to Bass et al. (Citation1994), the price variable should have to be changing from period to period to have some relevant impact on diffusion of certain products. Nevertheless, the impact of time on diffusion of all mobile data service generation is positive and significant.
The time coefficient (t2.5, t3 and t4) in shows that as time passes, a consumer valuation for products attributes usually increases given the product diffusion succeeded into the market. As more information become available through advertising, sales promotion and word of mouth results in an increase of product recognition. Furthermore, the time variable may also capture most of the effects of unavailable attributes such as speed, technological evolution and others (Jun & Park, Citation1999). In both model, the innovation and imitation coefficient of each data services are varied over successive generation, which implies that the innovation and imitation rate and capability of consumer may depend on perceived technological attributes. Furthermore, performance of the estimated model with marketing mix variables has better estimates compared to the model without marketing variable. The overall log likelihood values for model with marketing mix has higher value compared to model without marketing mix.
Table 2. Estimated result for extended generalized norton bass model
4.2. Switching diffusion model
Switching adoption is those who cease to use previous version of the generation and switch to the next newest generation services. Considering the price effect on switching adoption diffusion, the estimated result is summarized in .
Table 3. Parameter estimates for switchers’ adoption diffusion model
The imitation coefficient of the switching adoption model in (q2.5, q3 and q4) shows that the internal communication between the adopters and non-adopters were declining over successive generation services, unlike other literature finding such as (Chanda & Bardhan, Citation2008). The inclusion of price effect on switching diffusion model has not improved the fitness of the model compared to its estimates without price effect. Only the price coefficient of 4 G data model is significant in influencing switchers’ rate negatively. The time trend on switching adoption model shows positive insignificant except that of 4 G at 10% significance level.
4.3. Leapfrogging diffusion model
Leapfrogging is a behavior of potential adopters skipping previous generation and directly adopting a newer generation services. This definition is already used by Danaher et al. (Citation2001), Jiang and Jain (Citation2012) in studying diffusion of successive generation of products. According to leapfrogging model specified in methodology section, we have estimated leapfrogers demand for 2.5 G, 3 G and 4 G data services with and without considering price effect.
According to , the parameter estimates for the leapfrogers diffusion of model without price variable are all significant and consistent with other literature findings, (Chanda & Bardhan, Citation2008). That means the imitation coefficient over successive generation shows increment, which implies more effect of internal communication between adopters and non-adopters over successive generation. Concerning time effect, the impact of time on leapfrogers diffusion of 3 G is positive and for that of 4 G services is negative and insignificant. But in model with marketing variable, the price of 3 G services has negative and significant influence on leapfrogers diffusion process whereas price coefficient of other generation are negative insignificant. The overall log likelihood value is maximum for the model with marketing mix.
Table 4. Parameter estimates for leapfrogers adoption model
4.4. Comparison of switching and leapfrogging adoption diffusion
4.4.1. 3 G mobile data services
The introduction of 3 G mobile services brings significant change in the telecom service markets in Ethiopia. Although 3 G data services were introduced in 2009, it shows significant penetration in 2013 and 2014 following big promotion. Following the big promotion of 3 G services, there was network system failure due to congestion. After fixing the network problem the waiting customers’ leads to boom sales volume once again (National Bank of Ethiopia, Citation2015). The monthly subscription rate for 3 G services including switchers and leapfrogers is shown in .
The monthly subscription of 3 G mobile data service shows that initially there were switchers to 3 G services from previous generation users and later first time purchasers started leapfrogging. The cumulative diffusion of 3 G services including both switchers and leapfrogers are shown graphically in .
As we can see from , after the launch of 3 G services into the internet market, the first adopters were those who have been users of 2.5 G services. Since they already have awareness about the use the technology, they were found to be the immediate switchers to 3 G services. However, in the meanwhile the new adopters start to leapfrog to 3 G services instead of adopting 2.5 G internet services. Specially, as the network infrastructures were expanded to previously uncovered area, there would be more leapfrogers. Hence, concerning 3 G data services, the cumulative switcher’s rate is larger than the cumulative leapfrogers rate. From , the gap between switching adoption and leapfrogging adoption seems narrowing overtime which will be tested in the next demand forecast section.
4.4.2. 4 G mobile data services
The 4 G mobile data services was launched in August, 2015. Since its deployment in the capital city, its monthly subscription, switching rate and leapfrogging rate is shown in .
The monthly subscriptions, switchers and leapfrogers rate fluctuates overtime since 2015 because of frequent internet blackout and sales ban to cut information communication from social media based protests from the end of 2015 to 2016. This makes the diffusion of 4 G data services curve more oscillating because of the government obstruction on mobile internet services (Dugo, Citation2016)Footnote6 As we can observe from diagram 4 above, the monthly leapfrogers diffusion to 4 G data services is higher than that of switchers’ adoption diffusion.
From , the diffusion of leapfrogers to 4 G data services is larger than the switchers’ adoption to 4 G services. Furthermore, it gives more insightful implication to make comparison of leapfrogers and switchers diffusion over successive generations including price effect.
4.4.3. Switchers adoption diffusion to 3 G and 4 G data services
Concerning switching adoption diffusion, the switchers’ adoption rate for 3 G is higher than that of 4 G although the time of 4 G data service deployment is very recent compared to 3 G mobile data service diffusion.
The result of shows significant difference between switchers’ adoption diffusion to 3 G and 4 G services. The 3 G data services were deployed earlier in 2009 before the 4 G services deployment. Furthermore, the estimated switching rate of 3 G and 4 G data with, and without price and actual data are almost overlaps, which imply the fitness of the estimated model.
4.4.4. Leapfrogers adoption diffusion to 3 G and 4 G data services
Concerning the diffusion of leapfrogging to 3 G and 4 G data services, the actual leapfrogers to 3 G data services is higher than that of 4 G data services. However, from the estimated result the rate of leapfrogers to 3 G services is declining while that of 4 G services is slowly rising.
According to , with price effect the leapfrogging to 3 G service eventually declining while that of 4 G service is increasing.
Overall, the actual vs estimated (without price effect) switching and leapfrogging adoption diffusion curve shows no significant variation for both 3 G and 4 G data service, which indirectly confirms the workability of the extended generalized Norton bass model. The overall performance of forecasting for switching and leapfrogging adoption over successive generation services is given :
Table 5. Measure of forecasting accuracy
According to the statistical decision rule the lower, the mean absolute percent error the better the model fit. In addition, the higher the value of R square the better the estimated model fit. Hence, using the statistical criterion all model seems to have good fit.
4.5. Consumer choice based demand forecasting
Forecasting mobile data service diffusion is a critical issue for service provider to analyze their customer capacity and to prevent market failure that may arise from over capacity congestion. It also may help to make a long-term plan for supply of the services. Hence, to do so, we need to know the potential data service customers and their choice behavior as well. The study uses the consumer choice-based diffusion model to estimate the future forecast for 3 G and 4 G data services.
4.5.1. Diffusion of mobile phone services
The diffusion model of Bass has been widely used to explain the diffusion process of product or service sales. Let the sales during time t, is, the cumulative sales until t-1 as
, and the market potential as
, the bass model can be represented as follows:
By using the Bass model estimated parameters, we forecasted the number of mobile phone subscribers from 2018 to 2025 (). This forecasted number can be used as future potential subscribers for mobile data services for the forecasting periods.
Table 6. Estimated parameters of mobile phone diffusion
Then according to the choice based model, the choice probability for each mobile data service generations is estimated by multinomial logit model using the market share as proxy variable (Cooper & Nakanishi, Citation1989).Footnote8 Using full information maximum likelihood method, the choice probability for each mobile service generation is estimated in .
Table 7. Estimated parameters for choice-based model
From , we have calculated the choice probability for each generation services.Footnote9 Then, assuming this choice probability is constant for the next eight years, we have forecasted the demand for 3 G and 4 G data services.
As it is expected, the price effect has negative impact on the demand for 3 G and 4 G data services except for 2.5 G data services. For the 2.5 G data services, the real data show that both price of 2.5 G data declines, as same time subscriber to 2.5 G data also declines. This implies that the change in price effect on 2.5 G data service shows positive, which is inferior good or services. Study by Volland (Citation2012), finds consumption expenditure elasticity of beer demand was positive and inelastic, which implied beer was an inferior good for Germany compared to other alcohol before 1965, and after 2004 its elasticity changed to negative and price elastic, implying that consumers become more sensitive towards price change in beer. Another study finding by (Lu et al., Citation2017) on alcohol consumption, shows positive and inelastic price elasticity for some types of wine drinks, which were considered as inferior goods compared to others drinks. Hence, by same fashion, the demand for 2.5 G service can be inferior services compared to others such as 3 G and 4 G data services.
In order to crosscheck the interaction between these successive generations of mobile data services we have included the cross price of alternatives technology service in each generation model. The result shows that, for instance, as a 3 G service price increases the subscriber to 4 G data service increases, which shows the substitution effect.
4.5.2. Demand forecasting for 3 G data services
The demand forecasting for 3 G data service is mainly based on the consumer choice probability for each successive generation and the forecasted mobile phone subscribers. Using reveled preference data, we have calculated the choice probability for each successive generation of mobile data services and it’s assumed to be constant over the coming eight years.Footnote10 shows the forecasted switchers demand and leapfrogers demand for 3 G data services.
According to , the switchers demand for 3 G services is rising whereas the leapfrogers demand for 3 G services is declining over time. This may imply that previous generation users (2.5 G) are more substituting by 3 G data services compared to new purchasers of 3 G services.
4.5.3. Demand forecasting for 4 G data services
By using the same procedure with that of demand forecasting for 3 G data services, the leapfrogers and switchers demand forecast for 4 G services is explained in .
shows that the forecasted switchers demand to 4 G services is almost constant whereas that of leapfrogers to 4 G services is rising. From the very begging, the leapfrogers to 4 G were higher than the switchers to 4 G services. This forecasting may imply that there will be no significant switching adoption to 4 G services, perhaps, because of the absence of perceived difference in value of 4 G and 3 G data services, in addition to other switching cost factor. This forecasting is made assuming 5 G data service will not be launched until 2025 in Ethiopia mobile data service market.
4.6. Price elasticity
Price elasticity measures the percentage responsiveness of demand changes due to percentage change in price of mobile services per megabyte (MB) of data. The price for mobile data internet service may be changed because of technology development or market conditions. Therefore, the price elasticity of the market potential can be derived from the logit model.
In this section, we derive the price elasticity of 3 G and 4 G data services where price represents fees per MB data usage. The elasticity of the nth individual subscription to ith generation probability (Pni) of the fee per MB (Jun et al., Citation2000) is;
Accordingly, we can calculate the elasticity of consumers choosing 3 G services with respect to its price fee per MB data. Just recalling Equationequation (30)(30)
(30) above;
By the same fashion, the price elasticity for 4 G data services can be calculated, but in this case, we are interested in market potential elasticity, which is derived by just averaging elasticity of individual decision maker (Jun et al., Citation2000);
Where, this is the average of individual levels of elasticity weighted by the subscription probability.
4.7. Cross price elasticity
This measure the percentage change in demand for a particular generation services due to percentage change in other generation service prices.
For example, the change for 3 G service subscription due to change in 4 G service price is
Hence by same logic, applying to all generations services, the own price, own network coverage, and cross price elasticity is summarized in .
Table 8. Price and cross price elasticity
For example, if the fee per MB increases by one percent from present level of $0.015 cents, the market potential for 3 G and 4 G data services decreases by −0.370 percent and −0.844 percent respectively. But the price elasticity for 2.5 G data service shows positive which imply a type of inferior goods i.e. when the price fall leads to fall in quantity demanded. Therefore, 2.5 G data services is considered as an inferior good compared to the 3 G and 4 G data services. Studies by Lu et al. (Citation2017) and Volland (Citation2012)) on alcohol consumption behavior confirm positive own price elasticity and those drinks were considered as inferior goods.
Concerning the cross price elasticity, if the price of 3 G service increases by one percent from present level of $0.015 cents per megabyte the subscriber for 4 G increases by 0.148% assuming other things is constant. The reverse is also true i.e. as the price of 4 G service increases by one percent, the subscriber for 3 G increases by 0.019 percent that shows there is a substitution effect.
5. Conclusion and policy implication
There are no studies that forecast the number of 3 G and 4 G subscribers, switchers and leapfrogers demand in Ethiopia, despite the importance of these issues. There are also no studies that estimated price elasticity for 3 G and 4 G data services in the Ethiopia telecommunication market, as far as our knowledge is concerned. This paper forecast the switchers and leapfrogers demand for 3 G and 4 G mobile telecommunication services in Ethiopia, while incorporating the marketing mix element using an extended generalized Norton bass model. The finding of the study reveals that the extended GNB model can fit the forecast for successive generation of the mobile data services. Using choice based diffusion, the forecasted switchers and leapfrogers demand for 3 G and 4 G data services are increasing for the next 7 years. By 2025, the forecasted 3 G and 4 G data service subscribers are around 40 million and 1.93 million including both switchers and leapfrogers adoption. By decomposing to switching and leapfrogging, the forecasted demand for switchers to 3 G service shows increasing whereas that of 4 G services shows constant. By 2025, around 35 million subscribers of 3 G services are switching adopters and around 10 thousand of 4 G services subscribers are switching adopters. However, concerning demand for leapfrogers around 5 million and 1.92 million subscribers are leapfrogers adopters of 3 G and 4 G data services, respectively. The adopters’ responsiveness towards price change via price elasticity for 3 G and 4 G data services shows that the demand are price inelastic, whereas the cross price elasticity shows that both successive generations of mobile data service have substitution effect.
The policy implications of this study are as follows. Firstly, this study helps to make a long-term plan for the supply of the services. According to the study finding, although switching adoption to 3 G mobile data services increases, there is a rising of leapfrogers to 4 G data services. This implies that 4 G network coverage expands to remote areas people can adopt its services, and hence, the service providers can also benefit from huge untouched market given that it can supply services according to the future demand. The second implication can be drawn from own price elasticity point of view. The own price elasticity for 3 G and 4 G mobile telecommunication services are inelastic, so that the service provider can raise revenue by charging for its service packages strategically. The third implication is from the cross price elasticity point of view. Both 3 G and 4 G mobile data services are substitutes for each other according to the finding. Therefore, if the ETC operator can consider its long run plan and invest on 4 G mobile data infrastructure (instead of investing on both) the consumers can easily adopt 4 G services and be benefited from its feature services.
Finally, a few limitations in our approach that can be a future work for other researcher are that we assumed the choice probability for each successive generation to be constant over the coming eight years. Since the consumer, choice probability may be different across users and overtime, this assumption can be relaxed and studied by other interested researcher. Second, during the computation of forecasting demand for 3 G and 4 G data services, we assumed that 5 G would not be introduced in to Ethiopian mobile data service market until 2025. Again, this assumption can be relaxed by any interested researcher and may consider the possible introduction of 5 G data services.
Acknowledgements
This study was supported by Adama Science and Technology University (ASTU). Therefore, we would like to thank Adama Science and Technology University for the invaluable support.
Additional information
Funding
Notes on contributors

Birku Reta Entele
Birku Reta Entele (PhD) received a bachelor degree in Economics from Arba Minch University and MSc degree in Resource and Environmental Economics from Addis Ababa University in 2007 and 2011, respectively. Currently he has PhD in Technology Management, Economics and Policy from Seoul National University, Korea. His research interest includes; valuation of new technology, impact evaluation, consumer behaviour, demand analysis, and demand forecasting.
Bikram Acharya received a B.S. in electronics and communication engineering from Institute of Engineering, Pulchowk, Tribhuwan University. Currently he has PhD in Technology Management, Economics and Policy Program (TEMEP) from Seoul National University, Korea. His research interest includes public policy, demand forecasting, and consumer behaviour analysis in the different facet of ICT.
Notes
1. The advantage of GNB model is that it maintains the desirable mathematical properties such as closed form expression, and continuous time based (Jiang and Jain, Citation2012)
2. Jun and Park’s (Citation1999) Type I model does not explicitly express the rate of leapfrogging.
3. In Leapfrogging and switching adoption diffusion of N generation, we used existing previous generation adopters or potential adopters (N-1) which are potential leapfrogers and switchers to current new generation (Nth). But had the diffusion was normal multigenerational diffusion, we might assume each generation product may has its own market potential and hence we could estimate number of market potential equal to the number of generations. Furthermore, leapfrogers to 4 G is the sum of leapfrogers from 2.5 G and first time 4 G adopters.
4. This assumption is similar to the Meade & Islam (Citation2006) and Tsai (Citation2013), the innovation coefficient across successive generations are assumed to be constant (p1 = p2 = p) while imitation coefficient is varying
5. For both leapfrogging and switching diffusion model, the p is assumed constant over generation; otherwise the model doesn’t fit well. Note that, the leapfrogers to the 4th generation mobile service users are both first time purchaser and leapfrogers from 2.5 G services.
6. Amnesty International. (2016). press release on Ethiopian government blocking of websites during protest widespread. Addis Ababa: retrieved from, https://www.amnesty.org/en/
7. MAPE is mean absolute percent error which shows the prediction accuracy of forecasting mobile phone diffusion.
8. The market share for active in use data is transformed into deviation of logarithm of market share for each in use mobile generation data from the log of non-adopted market share. The price is transformed in to the deviation of absolute value of price from its average for each period for all generation (Basuroy & Nguyen, Citation1998; Brinkman, Coen-Pirani, & Sieg, Citation2015; Cooper & Nakanishi, Citation1989)
9. The market share for each successive mobile data generation was used as a proxy for aggregate MNL to estimate the parameters of the choice attribute. The estimated average choice probability for 2.5 G, 3 G, 4 G and no purchase decision are 0.20248, 0.25644, 0.02622 and 0.51486 respectively.
10. By assuming the consumer average preference is same with social preference and assuming it doesn’t changed over the next eight years, we could forecasted the demand for switching and leapfrogging model.
11. The fee of data service per MB is $0.015 cents in Ethiopia by January, 2018
12. The substitution effect of 4 G service price on 3 G subscribers is less than the vice versa.
References
- Bass, F. M. (1969). A new product growth for model consumer durables. Management Science, 15(5), 215–24. https://doi.org/10.1287/mnsc.15.5.215
- Bass, F. M., Krishnan, T. V., & Jain, D. C. (1994). Why the bass model fits without decision variables. Marketing Science, 13(3), 203–223. https://doi.org/10.1287/mksc.13.3.203
- Bass, P. I., & Bass, F. M. (2001), Diffusion of technology generations: A model of adoption and repeat sales, Working Paper, Bass Economics Inc., Frisco, TX.
- Basuroy, S., & Nguyen, D. (1998). Multinomial logit market share models: Equilibrium characteristics and strategic implications. Management Science, 44(10), 1396–1408. https://doi.org/10.1287/mnsc.44.10.1396
- Brinkman, J., Coen-Pirani, D., & Sieg, H. (2015). Firm dynamics in an urban economy *. International Economic Review, 56(4), 1135–1164. https://doi.org/10.1111/iere.12133
- Chanda, U., & Bardhan, A. K. (2008). Modelling innovation and imitation sales of products with multiple technological generations. The Journal of High Technology Management Research, 18(2), 173–190. https://doi.org/10.1016/j.hitech.2007.12.004
- Chien, C. F., Chen, Y. J., & Peng, J. T. (2010). Manufacturing intelligence for semiconductor demand forecast based on technology diffusion and product life cycle. International Journal of Production Economics, 128(2), 496–509. https://doi.org/10.1016/j.ijpe.2010.07.022
- Cooper, L. G., & Nakanishi, M. (1989). Market-share analysis: Evaluating competitive marketing effectiveness (Vol. 1). Springer Science & Business Media.
- Danaher, P. J., Hardie, B. G. S., & Putsis, W. R. (2001). Marketing-mix variables and the diffusion of successive generations of a technological innovation. Journal of Marketing Research, 38(4), 501–514. https://doi.org/10.1509/jmkr.38.4.501.18907
- Dugo, H. (2016). Violence against free media and knowledge dissemination in Ethiopia: An analysis of the mechanisms of restrictions on information flow. Africology: The Journal of Pan African Studies, 9(10), 395–410.
- Günther, M. (2017), Diffusion of multiple technology generations: An agent-based simulation approach, 2016 Portland International Conference on Management of Engineering and Technology (PICMET), IEEE: Honolulu, HI, USA.
- Guo, Z., & Chen, J. (2018). Multigeneration product diffusion in the presence of strategic consumers. Information Systems Research, 29(1), 206–224. https://doi.org/10.1287/isre.2017.0720
- Hughes, N. (2010). Apple’s recurring revenue stream: 77% of iPhone 4 sales were upgrades. Apple Insider(June 25).
- Hung, H. C., Chiu, Y. C., & Wu, M. C. (2017). A modified lotka-volterra model for diffusion and substitution of multigeneration dram processing technologies. Mathematical Problems in Engineering, 2017. https://doi.org/10.1155/2017/3038203
- Islam, T., & Meade, N. (1997). The diffusion of successive generations of a technology: A more general model. Technological Forecasting and Social Change, 56(1), 49–60. https://doi.org/10.1016/S0040-1625(97)00030-9
- Jiang, Z., & Jain, D. C. (2012). A generalized Norton–Bass model for multigeneration diffusion. Management Science, 58(10), 1887–1897. https://doi.org/10.1287/mnsc.1120.1529
- Jun, D. B., Kim, S. K., Park, M. H., Bae, M. S., Park, Y. S., & Joo, Y. J. (2000). Forecasting demand for low earth orbit mobile satellite service in Korea. Telecommunication Systems, 14(1/4), 311–319. https://doi.org/10.1023/A:1019105920390
- Jun, D. B., & Park, Y. S. (1999). A choice-based diffusion model for multiple generations of products. Technological Forecasting and Social Change, 61(1), 45–58. https://doi.org/10.1016/S0040-1625(98)00049-3
- Kim, N., Chang, D. R., & Shocker, A. D. (2000). Modeling intercategory and generational dynamics for a growing information technology industry. Management Science, 46(4), 496–512. https://doi.org/10.1287/mnsc.46.4.496.12059
- Lin, C., Kilicay-Ergin, Yu, G. E., Kilicay-Ergin, N. H., & Okudan, G. E. (2011). Agent-based modeling of dynamic pricing scenarios to optimize multiple-generation product lines with cannibalization. Procedia Computer Science, 6, 311–316. https://doi.org/10.1016/j.procs.2011.08.057
- Lu, H., Hess, S., Daly, A., & Rohr, C. (2017). Measuring the impact of alcohol multi-buy promotions on consumers‘ purchase behaviour. Journal Of Choice Modelling, 24, 75–95. https://doi.org/10.1016/j.jocm.2016.05.001
- Mahajan, V., & Muller, E. (1996). Timing, diffusion, and substitution of successive generations of technological innovations: The IBM mainframe case. Technological Forecasting and Social Change, 51(2), 109–132. https://doi.org/10.1016/0040-1625(95)00225-1
- Meade, N., & Islam, T. (2006). Modelling and forecasting the diffusion of innovation–A 25-year review. International Journal of forecasting, 22(3), 519–545.
- National Bank of Ethiopia. (2015). Annual Report.
- Negi, R. (2009). User’s perceived service quality of mobile communications: Experience from Ethiopia. International Journal of Quality & Reliability Management, 26(7), 699–711. https://doi.org/10.1108/02656710910975769
- Norton, J. A., & Bass, F. M. (1987). A diffusion theory model of adoption and substitution for successive generations of high-technology products. Management Science, 33(9), 1069–1086. https://doi.org/10.1287/mnsc.33.9.1069
- Radas, S. (2006). Diffusion models in marketing: How to incorporate the effect of external influence? Privredna Kretanja I Ekonomska Politika, 15(105), 30–51. https://hrcak.srce.hr/18398
- Rogers, E. M. (2003). Diffusion of innovations. Free Press. In New York (pp. 551).
- Speece, M. W., & Maclachlan, D. L. (1995). Application of a multi-generation diffusion model to milk container technology. Technological Forecasting and Social Change, 49(3), 281–295. https://doi.org/10.1016/0040-1625(95)00006-V
- Temesgen, Z., Negi, R., & Ketema, E. (2009). An assessment of the marketing strategy for mobile services of Ethiopian telecommunication corporation. Services Marketing Quarterly, 31(1), 50–71. https://doi.org/10.1080/15332960903408443
- Tsai, B. H. (2013). Predicting the diffusion of LCD TVs by incorporating price in the extended Gompertz model. Technological Forecasting and Social Change, 80(1), 106–131.
- Volland, B. (2012). The history of an inferior good: Beer consumption in Germany (No. 1219). Papers on Economics and Evolution. Max-Planck-Institut für Ökonomik.
- Zsifkovits, M., & Günther, M. (2015). Simulating resistances in innovation diffusion over multiple generations: An agent-based approach for fuel-cell vehicles. Central European Journal of Operations Research, 23(2), 501–522. https://doi.org/10.1007/s10100-015-0391-x