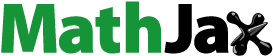
Abstract
Agglomeration economies are the external benefits earned from clustering of industries and people in cities. The study assumes unbridled clustering of population in emerging urban agglomerations turning economies into diseconomies. This study empirically investigates the heterogeneous socioeconomic impacts of agglomeration economies in selected cities of Punjab, Pakistan, from 1998 to 2018, using the Pooled Mean Group and the Mean Group techniques of Panel ARDL. Agglomeration economies are determined by population density, number of registered factories, employment size, and housing, in the cities of Punjab. The study designed four indices for socioeconomic conditions using principal component analysis. These include: education-index, healthcare-index, water & sanitation-index, and economic conditions-index. Research findings reveal pressures of high population density, unemployment, and costly housing on educational & healthcare facilities, poor sanitation & waste management, in cities of Punjab, Pakistan. The study suggests that policy makers and urban planners to develop short term and long term policies and development plans for villages and secondary cities to uplift wellbeing of the local population. Nonetheless, cities need to decentralize for sustainable development and management.
PUBLIC INTEREST STATEMENT
Generally, population and industrial clustering in cities create benefits for individuals and for the economy as a whole. However, in the case of developing economies, due to poor economic infrastructure, and rapid rise in population size, it creates different socio-economic and administrative challenges in absorbing this pressure. The study investigates the short-run and the long-run socio-economic impacts of population clustering in big cities of Punjab, Pakistan. The study tested the impact of population clustering (agglomerations) for 21 years (1998–2018). The findings of the study revealed that pressures of high population density, unemployment, and costly housing are creating pressures on educational & healthcare facilities, sanitation & waste management, and on economic conditions of households, and for city administration. It is suggested for the policy makers to develop both short term and long term plans to control population pressures towards big cities and plan to strengthen administrative infrastructure of the cities.
1. Introduction
Agglomeration economiesFootnote1 are the major characteristic of urbanization, showing structural transformationFootnote2 of a region’s economy. Globally, countries benefit from urbanization by nurturing productivity through clustering of enterprises and people in their cities. Nonetheless, concentration of economic and social activities and migration is a usual phenomenon of big cities. Agglomeration economies and congestion forces come to the surface due to urbanization. Therefore, the process of urbanization poses huge socio-economic, administrative, infrastructural, energy, transport, and environmental problems for the developing world (Arif et al., Citation2019; Rana & Bhatti, Citation2018). Estimates suggest that the urban population of the world would increase by 2.5 billion in 2050 (United Nations, Citation2019). Correspondingly, the urban population of South Asia is projected to increase by 250 million by 2030 (World Bank, Citation2016). Population density, employment size, labor and land prices are the major drivers of agglomerations economies in cities (Glaeser, Citation2010). The knowledge spillover, availability of skilled labor, market access, and good infrastructure, are documented as the micro-foundations of agglomeration economies (Rosenthal & Strange, Citation2001 &, Citation2004; Glaeser, Citation2010). In the long-run, successful urbanization goes along with socioeconomic benefits that spill beyond the urban boundary. Nonetheless, these positive trends are weakened by the rising pressures of the urban population growth on infrastructure, land, housing, basic services, and environment, in the developing economies. Statistics illustrate that around 130 million South Asian residents live in slums and squatters and are deprived of basic infrastructure and services (World Bank, Citation2016). These slum settlements develop peri-urban areas around the city boundary, making rapid modifications to city landscape, and in human activities (Arif & Gupta, Citation2018). These undesired negative effects impeding economic, social and environmental development are termed as over-agglomeration in the economic literature (Kaya & Koc, Citation2019). Nonetheless, the access to information and telecommunication has reduced the importance of Agglomeration Economies (Guiliano, Kang, & Yuan, Citation2019).
Pakistan, with a total land area of 770,880 square km, and population of 220,892,340 people makes 2.83% of the world’s population. It ranks the fifth most populous country in the world with 35.1% urban dwellers . Pakistan is the most urbanized South Asian economy, with 80 million people living in cities (UNDP, Citation2018). The urban population size is growing at 3.3% rate annually as a consequence of structural transformation and migration to urban areas (Rana & Bhatti, Citation2018), during the past few decades (Appendix-I).
The Punjab province is the most urbanized region of South Asia and the recipient of the largest number of migrants from all around Pakistan (Appendix-II), with a consistent demographic shift towards urban areas and cities (; Rana & Bhatti, Citation2018). Punjab alone contributes 50% of the GDP. Lahore, Faisalabad, Gujranwala, Rawalpindi, Multan, and Islamabad are the six cities of Punjab which are among ten largest Pakistani citiesFootnote3 (Appendix-III). These cities cumulatively contribute 55% of the GDP (MGI, 2012Footnote4). Nonetheless, the ten major cities’ share in GDP has also increased to 78%, with a concentration of industrial and services sectors, and they contribute 95% of federal tax revenue (UNDP, Citation2018). This positive growth attracts more people to migrate towards cities. Over the time, the cities are expanding disproportionately in area and in population size (Kanwal et al., Citation2015; Kugelman, Citation2013; Rana & Bhatti, Citation2018) (see map in Appendix-IV). As per urban cluster analysis of Punjab cities, Gujranwala is growing at 8.13% and is expected to be the second largest city in 2040. Faisalabad would be the next largest city in terms of urban expansion (4.02%). More interestingly, the medium cities of Narrowal (12.17%) and Muridke (9.4%) would be the fourth and fifth settlements respectively, and these are expected to be as big as Faisalabad (The Urban Unit, Citation2018).
With the immense rise in growth of cities, costs of basic utilities, administrative services and security arrangements, also rise. It clearly affects the sustainability of urban development, and necessitates strategies for urban growth with emphasis on shift towards development of secondary cities (Ahmed & Ishrat, Citation2020; Malik et al., Citation2020).
The present study has assumed cities as urban agglomerations and investigates the socioeconomic impacts of Agglomeration Economies in five major cities (Lahore, Faisalabad, Gujranwala, Rawalpindi, and MultanFootnote5), of Punjab, Pakistan. The data was collected for 21 years (1998–2018) from different published sources of Pakistan Bureau of Statistics. The pooled mean group (PMG) and the mean group (MG) techniques of panel ARDL approach were used to analyze the relationship of variables of agglomeration economies and socioeconomic conditions. The research attempts to fill gap in empirical literature by providing evidence of both positive and negative socioeconomic impacts of agglomeration economies.
The study is organized in the following sections; section-2 gives review of literature on factors associated with agglomeration economies. Section-3 provides the theoretical background of the study. Section-4 provides the details of model, methodology, data sources and study area. Section-5 demonstrates the results and discussion, and lastly section-6 gives conclusions and recommendations of the study.
2. Literature review
Modern cities attract physical and human capital investments from surroundings. However, weaker economies get depressed with the flight of such capital. For this reason, urban areas become a major competitive advantage in global labor and capital markets. Social infrastructure, e.g., education, healthcare, leisure, culture, entertainment, and transportation attract people from neighborhood that cluster in cities (Ovsiannikova et al., Citation2018; Sun et al., Citation2018).
Housing is a vital issue in the developing world, and around 70% of urban population lives in informal settlements (Malik et al., Citation2020; UN-Habitat, Citation2016). In South Asia, primary citiesFootnote6 and secondary citiesFootnote7 of India, Pakistan, Bangladesh, and Sri Lanka, are facing various urban challenges, e.g., population pressure on infrastructure, housing, basic services and environment. The smallFootnote8 and mediumFootnote9 cities yield positive impact on economic growth; however the primary cities remain statistically insignificant across countries (Deb, Citation2017; World Bank, Citation2016).
In Pakistan, with rising migration towards urban areas, various macroeconomic and socio-economic problems such as urban poverty, overpopulation, environmental pollution, deprivation of education and health, poor sanitation, over-crowded housing, congested traffic, road accidents, and crimes are increasing (Afzal et al., Citation2018; Latif & Yu, Citation2020; A. U. Khan et al., Citation2016). Other urban growth challenges include conversion of farm-lands into residential schemes, squatter settlements, deficient services, and unavailability of clean drinking water (A. A. Khan et al., Citation2014). On the other side, push factors of migration towards cities include; internal war, insecurity, natural disasters, expensive agriculture, un-equal landholdings, oppressive lifestyle (Kugelman, Citation2013). A large number of rural residents have moved to the outskirts of cities, and have undergone structural changes resulting in high density and pressure on infrastructure, resources and urban land (Government of Pakistan. [GOP], Citation2015).
According to a recent labor force survey, urban labor force is 31.5 percent of the total labor force; the province of Punjab holds the highest share that accounted for 57 percent of the total. However, urban labor force participation in Punjab and KPK declined, due to high dependency ratio and rate of migration between 2001–02 and 2013–14 (PES, Citation2018-19). This low urban labor force participation and increased migration show a sluggish expansion of urban economic base that ultimately negates the benefits of urbanization (SPDC, Citation2016). The unbridled urban growth of Lahore has brought a variety of urban environmental annoyances, i.e., untreated industrial and municipal waste, uncollected solid waste, traffic congestion and vehicle exhaust posing serious health risks to the city dwellers (N. Y. Khan et al., Citation2012). Other cities of Punjab; Faisalabad, Gujranwala, Multan, and Rawalpindi, are also facing challenges of inadequate infrastructure and urban management capacities. Water supply, sanitation, and waste management services are unreliable. The aquifers are also over exploited. The congested roads, poor transport, and traffic management system constraint in urban mobility. Housing facilities are also incapacitated for the growing population. This widespread under-performance in service delivery and incapacitate infrastructure affect living conditions, and limit business growth reducing the productive potential of cities (PES, 2018–19).
In 2008, about one-half of the populations of Punjab and Sindh provinces were living in cities, while the figures for Balochistan and KPK were less than 24 percent and 17 percent respectively. Lahore city, the provincial capital of Punjab, has experienced an arbitrary population growth from 6.32 to 11.12 million during 1998 to 2017. The population density rose from 3,566 to 6,606 persons per square Kilometers in 2018, challenging administrative and civic facilities. The city is being further expanded at the cost of productive agricultural lands. This issue has not been resolved by city district government. The urban planners need to develop an integrated plan for infrastructural and socioeconomic development for the city (Rana & Bhatti, Citation2018).
Previous studies suggest that urbanization in Pakistan had dual impact on development of the economy; by encouraging workers to move from agriculture to services sector, and conversely, this caused problems for migrants by depriving them from basic needs (Awan & Iqbal, Citation2010). In addition, urban agglomeration encourages producers and consumers for trade of goods, and a new middle class of over 100 million people emerges, and providing a skilled workforce (Hassan et al., Citation2012). Furthermore, population size, road density, and technically trained labor pool, promoted industrial agglomerations in Pakistan (Burki & Khan, Citation2011). Agglomerations of localized and urbanized economies have a strong impact on formation of new firms, their scale of operation, employment, enrollment levels, and social-inclusion. However, this impact varies with firms’ size (Azhar & Adil, Citation2016; Chaudhry & Haroon, Citation2015).
A few studies claim that the urban population growth rate of a consistent 2% during previous 19 years (1998–2017), is understated in the government statistics. Despite this high rural-urban migration and immense expansion of urban areas boundaries exhibits a rising trend in population growth and demands associated with it. The static population growth might be responsible for de-population or urban-rural migration. The official boundaries of urban areas are expanded immensely. However, the administrative boundaries data fail to delineate the extent of cities where urbanization is rapid, and can cause biases (Bosker et al., Citation2020; Duranton, Citation2015).
Around the globe, studies have investigated the micro-foundations of agglomeration economies (Rosenthal & Strange, Citation2001, Citation2003; Glaeser, Citation2010), benefits of industrial clustering in cities, economies and diseconomies associated with agglomerations. However, in the case of Pakistan, this area is not explored much. Few empirical studies have examined the effects of agglomeration economies; on formation of new firms; on efficiency of manufacturing industries; and on firms’ turnover (Burki & Khan, Citation2011; Haroon, Citation2013; Nasir, Citation2017). Furthermore, two studies identified positive impact of district agglomerations on firm efficiency, social inclusion, and economic outputs, in case of Punjab, Pakistan (Azhar & Adil, Citation2016; Chaudhry & Haroon, Citation2015). The developmental progress of cities of Punjab shows that the environmental conditions of the cities are poorer than the economic and social conditions. However, none of the cities attained the position of a sustainable city, showing the poor condition of infrastructure of the major cities in Punjab (Ghalib et al., Citation2017). The negligence towards the development of secondary cities is one of the causes of over urbanization and environmental degradation in big cities and metropolitan areas. However, secondary cities can play a fundamental role to attain sustainable development at local, regional and national level in Pakistan (Kalwar et al., Citation2016). However urban un-sustainability; economic imbalances, high rents, air and noise pollution are serious challenges to sustainable development and require urban resilience and community engagement (Latif & Yu, Citation2020).
3. Theoretical foundations of the study
The theoretical foundation of the Agglomeration Economies originates from Marshall’s concept of scale economies. A city generates economies as well as diseconomies of scale, as the effects of agglomeration, due to its physical growth (Marshall, Citation1920). Myrdal (Citation1957), Hirshman (Citation1958), and Kaldor (Citation1970) discuss the classical issues of convergence and divergence in a dynamic spatial economy. These studies provide that diverging tendencies dominate converging ones in a growing market economy. The theory of AEs put forward that firms achieve positive externalities from the spatial clustering of economic activities. These benefits arise from intra-and inter-industry clustering, referred as localization and urbanization economiesFootnote10 (Fujita et al., Citation1999; Fujita & Thisse, Citation2002; Melo et al., Citation2009).The mobility of goods, people, and capital enhance this divergence. Conversely, the mobility of knowledge could not get much attention. It is also observed that producers of goods and services are sensitive to urban congestion, through its impact on business cost and productivity levels of outputs (Weisbrod et al., Citation2003).
However, Solovian growth theory (1957) proves that incomes converge under constant returns, and this convergence accelerates by any sort of mobility. Thus, in these theories, information mobility is ignored from the beginning. Krugman (Citation1991) developed a novel theory of the New Economic Geography (NEG), to explain the formation of various economic agglomerations in a geographical space. The NEG theory presents agglomeration benefits and location choices in a formal, general equilibrium framework through the interaction between transportation costs and scale economies. The earlier literature provides evidence supporting NEG theory (Breinlisch, Citation2006; Hansen, Citation2005; Head & Mayer, Citation2004; Redding, Citation2009). NEG is used to describe the spatial agglomerations of industry and population and the economic performance (Fan & Scott, Citation2003). The main features of NEG models are product differentiation, increasing returns to scale and transport costs, mutually creating economic externalities. The three building blocks increase cumulative causation and agglomerations, along with either factor mobility or intermediate inputs. However, the orthodox and heterodox schools of economics have criticized the monopolistic modelling logic of NEG. These critiques focus on the immeasurability of some concepts of increasing returns of NEG frameworks, such as the static nature of some of its assumptions, the specific focus on the representative firm, presence of the pecuniary economies and the absence of human capital and technological spillovers as externalities (McCann & Oort, Citation2019). Besides, the balanced growth theory describes, urban structure is the margin that reduces increasing returns, to earn constant returns to scale in aggregate, sufficient to deliver balanced growth (Hansberg & Wright, Citation2007). This theory produces Zipf’s Law, to describe the city-size distribution, under certain assumptions (Arshad et al., Citation2019), where Zipf’s Law for cities is described as a striking pattern of agglomerations (Gabaix, Citation1999).
4. Model, methodology and data
4.1. Model specification
This section provides the details of model specification of socio-economic conditions (SEC)Footnote11 along with the independent variable of agglomeration economies (AE) i.e. Equation-(1).The model is given below:
The study used population density, number of registered firms, employment size of firms, and housing as proxies for agglomeration economies.
Then, it is further explained with the construction of following four equations:
Where, the dependent variable, socioeconomic conditions (SEC), is represented by four different indices, e.g., education-index (EI), healthcare-index (HI), water & sanitation-index (WSI), and economic conditions-index (ECI). The agglomeration economies (AE) are measured by population density as persons per square kilometer (DEN), number of factories (FAC), size of employment in factories (EMP), and housing (HOU), from Equationequations (2)(2)
(2) to (5).
4.2. Methodology
Panel data techniques of pooled OLS, fixed and random effects, are considered inappropriate for non-stationary data. These are static models, for having common slope and variance. The time series and cross sectional effects can only be observed by including dummy variables, which reduces the degree of freedom (Baltagi, Citation2008). Furthermore, in presence of endogeneity, and correlation between regressors and error term, fixed effects parameter estimates become biased (Campos & Kinoshita, Citation2008). Similarly, the Random effects model has shortcomings of time invariance. Thus, static panel estimators cannot provide the short-run and long-run relationships (Loayza & Ranciere, Citation2006). These models also assume homogeneity of lagged dependent variable coefficient (Holly & Raissi, Citation2009). Thus the static panel techniques are unable to estimate the dynamic models.
In PMG, error variance becomes heterogeneous across individual units. The efficiency, consistency and validity of PMG require a long-run relationship among variables, the coefficient of error correction term must not be less than minus 2. Moreover, the residuals of the error correction model should be serially uncorrelated and explanatory variables be considered as exogenous.
A long-run relationship among variables exists only if all variables are stationary at the same order of integration, whereas the panel ARDL can be applied on variables with different orders of integration (Phillips & Hansen, Citation1990). The ARDL regression, through pooled mean group estimation, provides consistent estimators, whether the variables of the model are integrated of order one I (1), or integrated of order zero I (0) (Pesaran et al., Citation1999). Like the time series ARDL method of estimation, the PMG estimator also cannot be applicable if any variable in the model is integrated of order two, I(2) (Pesaran et al., Citation1999). This is the reason the unit root test is applied before the selection of estimation technique.
The panel ARDL is, autoregressive distributed lag model, with selection of lags of order p in the dependent variable and lag of order q in the explanatory variables. The ARDL specification is constructed in equation-(6), (7), (8) and (9):
Where EI is the dependent variable and DEN, FAC, EMP, and HOU represents the set of explanatory variables. The parametric form of error correction of each indicator is represented by Equationequations (10)(10)
(10) to (13).
Where π and ω are the short term coefficients of the lagged dependent and independent variables, while ψ is the long run coefficient. In addition, φ the coefficient of error correction term, represents the speed of adjustment of dependent variables (EI, HI, WSI, ECI) towards the long run equilibrium level, which also ensures changes in independent variables. The negative and significant value of φ (φ < 0) is the evidence of cointegration between independent and dependent variables. The large value of error correction term (φ), shows faster convergence rate towards the long-run equilibrium. Moreover, the positive value of error correction term (φ > 0) shows stable relationship does not exist among variables in the long-run. Thus the long-run coefficient (ψ) and the speed of adjustment (φ) are considered important in the estimation of the model.
In order to estimate the equations of study, the pooled mean group (PMG) and mean group (MG) techniques are applied. This method is flexible and allows individual specific variations in the short-run coefficients. The alternative method (MG), of estimation of error correction term of ARDL, is applied for comparison purpose (Pesaran & Smith, Citation1995). Like PMG estimation, MG is also applied if data is non-stationary and parameters are heterogeneous across the individual units/groups. The MG estimators provide long-run and short-run slope parameters to vary over individual units only.
The selection of appropriate approaches between PMG and MG can be tested by applying Hausman statistics. The MG estimators become inefficient if the long run homogeneity restrictions prove valid. This provides that PMG estimators are more efficient than the MG.
4.3. Data description and sources
This paper attempts to estimate the short-run and the long-run socio-economic impacts of agglomeration economies using annual data from five major cities of Punjab, Pakistan, over the period of 21 years (1998 to 2018). The data has been collected from different published sources of Pakistan Bureau of Statistics (PBS); including development statistics of the Punjab, Multiple Indicator Survey (MICS), Census of Manufacturing Industries (CMI), and compendium of environment. These cities are selected on the basis of their population size (called urban agglomerations). In contrast to developed countries, the time series data on city level is very limited in Pakistan. The motivation for taking data from 1998 to 2018 was to observe the urban agglomerations, as cities have become densely populated during the last few years. In the empirical analysis, most of the data is taken in numbers and some in percentages, as was available with the Bureau of Statistics, Government of Pakistan. The total number of observations is 105.
4.4. Sample area
Undoubtedly, Karachi, capital city of Sindh province, is the largest populated city of Pakistan. However, Sindh province has only two big cities (Karachi and Hyderabad), and Punjab, the most populous province, has six big cities. Urbanization is more obvious in Punjab, and it is the most industrialized province, making the major share to the national GDP. It is an important economic hub in Pakistan. The province is bordered by the other three provinces, i.e. Balochistan, Sindh, and Khyber Pakhtunkhwa, making the inter-provincial movement easier. Punjab is known for its comparative prosperity and the lowest poverty rate among all provinces. Therefore, the study selected five major cities of Punjab province. The shows the map of the study area. However, Islamabad city is excluded because it is federally administered capital city of Pakistan, and not considered in provincial administration of Punjab.
Figure 1. Map of Punjab province showing all major cities (high density)

In Punjab, 40% people are living in its urban areas. According to World Bank, the problems associated with urbanization include education, health, water and sanitation, poor housing quality and affordability, and transportation. According to existing studies, these problems are most apparent in the five major cities of Punjab, i.e. Lahore, Faisalabad, Gujranwala, Rawalpindi, and Multan. During previous twenty years (1998 to 2017), with following increase in population growth i.e. Lahore (116.32 %), Faisalabad (59.49%), Rawalpindi (48.84%), Gujranwala (78.10%), and Multan (56.33%) (UNICEF, Citation2020), these cities have become urban agglomerations.
5. Results and discussion
The section provides the results and discussions on the empirical results of the study.
5.1. Principal component analysis
The study used principal component analysis. The method was initiated from the works of Pearson (Citation1901) and Hotelling (Citation1933), and updated by Jolliffe (Citation1986), to develop four indices. Following Kaiser (Citation1960) rules of retaining the number of components based on eigenvalues, the selected four indices of Education, Healthcare, Water & Sanitation and Economic Conditions are presented in Appendix-V.
5.2. Summary statistics and correlation coefficients
The results of summary statistics and correlation coefficients among independent and dependent variables are given in appendices VI, & VII.
5.3. Unit root tests
It is necessary to pre-test the stationarity of data series before proceeding to econometric estimation of the model. For this, the study run Levin-Lin-Chu and Im-Pesran-Shin unit root tests for all variables, and found that the variables are integrated of mixed order i.e. I(0) and I(1). Pesaran et al. (Citation2001) suggest that with stationary variables of order I(0) and I(1), the pooled mean group and the mean group techniques are appropriate to estimate the model. However, these techniques are inappropriate if a data series is integrated of order 2 or higher. Moreover, while applying PMG and MG techniques, the dependent variables of the models must be integrated of order I(1). For this reason, we run unit root tests (Levin-Lin-Chu and Im-Pesaran-Shin) for the four indices (EI, HI, WSI, & ECI) too, to confirm that PMG and MG techniques are appropriate for the econometric analysis of study data. The findings of unit root test for all variables are provided in Appendix-VIII.
5.4. Pooled mean group and mean group estimates
To estimate the long-run and the short-run impacts of agglomerations on socioeconomic conditions in the cities, we apply both the PMG and the MG estimators, and the maximum lag of one is selected on the basis of Schwartz Bayesian Criterion. Initially, from socioeconomic variables, four different indices were constructed; education index, health index, water & sanitation index, and economic conditions index, using principle component analysis (PCA). Therefore, the study has estimated the impact of agglomeration economies on these four indices using the Equationequations (6)(6)
(6) to (9) and Equationequations (10)
(10)
(10) to (13) respectively.
shows the results of the PMG and MG estimators in four columns (1 to 4). Where, column-1 represents estimates of both PMG and MG for Education Index, column-2 represents estimates for Healthcare Index, column-3 exhibits estimates for Water & Sanitation Index, and column-4 depicts estimates for Economic Conditions Index. On the basis of Hausman test results, hypothesis of poolability of the long-run coefficients is accepted, and an appropriate model is chosen among both PMG & MG. Thus, it is considered that the PMG estimator is efficient and preferable to MG estimator, for education and economic condition indices, the results of PMG are interpreted for these indices. However, for healthcare and water & sanitation indices, MG is preferred over PMG, so only MG results are interpreted for these two indices.
Table 1. Showing the effects of agglomeration economies on socioeconomic conditions of cities
In Education-Index, at an individual level, the variable of population density shows an insignificant relationship in the long-run, but a significant positive relationship in the short-run. Possibly, in the short-run, in high dens areas, more people enroll in schools and colleges for educational attainment, and the number of educational institutions is also increased to accommodate an increased population in cities. In a favorable learning atmosphere, people concentrate in the neighborhood, which reduces travel time and crime rate (Sun et al., Citation2018). However, the number of factories has a negative and highly significant relationship with education in the long-run. The employment in factories has a significant positive impact on education index in the long-run. This implies that with more employment opportunities, the educational attainment increases in population in cities. However, with more labor absorption, high rate of migration of educated labor is also expected towards cities in the long-run.
This result is in line with previous studies confirming localization economies of knowledge diffusion, buyer-supplier network, and a skilled labor-pool (Burki & Khan, Citation2011; Haider & Badami, Citation2010; Hassan et al., Citation2012). The demand for housing also has a significant positive relationship with education, in the long-run. Educated people move to cities for better living conditions (Azhar & Adil, Citation2016). Overall, the results show that AEs have a significant long-run impact on education index. The error-correction (ECT) coefficient estimate of education index is significantly negative (−0.19) shows the deviation from the long-run equilibrium is corrected by about 19% in one year. The value lies within the dynamically stable range for the PMG estimator. Both, Hausman and ECT values confirm that PMG is preferred for Education Index.
Healthcare-Index consists of the variables of provision of healthcare services in the cities. Again, the ECT estimate is significantly negative with a magnitude of −0.42. In addition, under the Hausman test results, MG is preferred over the PMG approach, and the interpretation of this model centers around MG results only. In the long-run, the coefficients of population density and employment in factories show a significantly negative impact on health conditions in the cities. It shows that a high population density creates pressures on availability of healthcare services and overall health conditions in the cities. High population density in the area causes disease prevalence and other mental health issues (N. Y. Khan et al., Citation2012) to the citizens too.
Water & sanitation-index exhibits the coefficient of ECT is significantly negative showing deviation from the long-run equilibrium is corrected by 55% in one year. In the long-run, population density shows a significantly negative impact on water & sanitation conditions. It indicates the pressures of high population density on available water resources, and municipal services. The unavailability of clean drinking water, inadequate disposal of residential and industrial wastes, vector-borne diseases, and vehicle exhaust are the problems of cities along with poverty (A. A. Khan et al., Citation2014). The number of factories, and employment also show a significant negative impact on water & sanitation-index. The consumption of natural resources, pressures on infrastructure, uncollected solid waste and traffic congestion pose serious health risks (N. Y. Khan et al., Citation2012; GOP, 2014), in the long-run. In Pakistani cities, around 40% citizens have access to safe water supply, and nearly 80% have access to sanitation services, full and safe treatment and disposal of wastewater is almost nonexistent (World Bank, Citation2019). The coefficient of housing indicates a significant positive impact on water & sanitation-index. This indicates, as the demand for housing increases, the demand for water & sanitation services also rises, in the long-run. The results are in line with other studies, as the increased number of settlements around water bodies is a major reason for stress on aquatic sources. The total waste water discharges in Pakistan are recorded as 7,590 million cubic meters annually, of which 30% comes from industries and 70% comes from domestic discharges. It is expected that both of these discharges would double by 2025. Previously, only 1% of urban wastewater was treated in Pakistan, and the remaining flows into rivers without any treatment, contaminating the aquatic sources (United Nations Environment Programme [UNEP], Citation2013).
Economic condition-Index consists of the variables of economic status of people in the cities. The significantly negative value of ECT shows the deviation from the long-run equilibrium is corrected by 46% in one year. The ECT and Hausman values prefer PMG model for Economic condition-index. Among agglomerations variables, population density has a significantly negative impact on economic condition in the long-run. A high population density challenges economic, administrative and civic amenities of cities, and cause socioeconomic problems (Afzal et al., Citation2018; Imran et al., Citation2013). The number of factories also shows a negative impact on economic condition. Along with this, the coefficient of employment in factories has also a significantly negative relationship with economic conditions, both in short-run and long-run. The negative sign of these variables may indicate unemployment in cities. Rising unemployment and inflation in cities, increases urban poverty (A. U. Khan et al., Citation2016). However, the variable of housing shows a significant positive impact, may indicate the development of informal housing, and rising number of housing schemes around the cities (Malik et al., Citation2020; UN-Habitat, Citation2016).
Overall findings of the study show that high population density has a significant negative impact on healthcare, water & sanitation and economic conditions of the cities, in the long-run. The rising number of factories has significant negative impact on all socioeconomic variables (i.e. indices of education, healthcare, water & sanitation, and economic conditions) in cities in the long-run. The variable of employment in factories has significantly negative impact on healthcare, water & sanitation, and economic condition indices, however positive impact on education index. The availability of housing has a positive impact on all indices, in the long-run, for the cities of Punjab, Pakistan. These findings confirm the hypothesis of the study that apart from positive impacts of agglomeration economies, negative impacts are also affecting the socioeconomic conditions of the cities of Punjab, Pakistan.
6. Conclusion and recommendations
The main objective of the present study was to investigate the socioeconomic impacts of agglomeration economies in the major cities of Punjab, Pakistan. For this purpose, five major populous cities (i.e. Lahore, Faisalabad, Gujranwala, Rawalpindi and Multan) of Punjab province were selected for study. The data was collected for previous 21 years from 1998 to 2018, to check the long run impacts of agglomeration economies on socioeconomic conditions of Punjab cities. Initially, four indices were constructed for socioeconomic variables, using PCA technique. Afterwards, PMG and MG techniques of Panel data were applied on all four indices to analyze short-run and long-run impacts. The findings of this research depict that the concentration of industries and population, along with positive economies, create socioeconomic challenges for emerging economies like cities of Pakistan. This study attempts to describe the relationship between city size, availability and provision of socioeconomic infrastructure. The results clearly conclude that unbridled population pressures towards cities, uncontrolled and non-progressive growth in number of factories are creating multiple challenges on the existing social, economic and administrative infrastructure that reduce the ability of cities to address negative externalities.
The private provision of infrastructure can reduce negative externalities, but it is cost-effective only when city population is low or institutions are strong, however, public provision can be more cost-effective in bigger cities. The authors are of the opinion that the government needs to take measures to control the unbridled urbanization through integrated and sustainable initiatives of socioeconomic and infrastructure development along with capacity building, institutional strengthening and development control measures within legal and regulatory framework. Nevertheless, decentralization of large cities and development of secondary cities as an effective urban management tool needs to be opted. Development of regional plans and local infrastructure plan ensure all public amenities, infrastructure facilities and provision of administrative services is also suggested for small towns and villages to control the out-migration, towards big cities. It further suggests the studies on urban public service delivery.
Acknowledgements
The authors acknowledge the valuable comments of the anonymous reviewers in making this work publishable.
Disclosure statement
The authors have no conflict of interest.
Additional information
Funding
Notes on contributors

Naghmana Ghafoor
Ms. Naghmana Ghafoor is a PhD Scholar, at the Superior University, Lahore, Pakistan. She is also serving as Lecturer in Economics, Lahore College for Women University, Lahore Pakistan. Her field of research is development economics and urban economics. The present study is the part of her doctoral research.
Sana Fayyaz
Dr. Sana Fayyaz is Assistant Professor of Economics, Superior University Lahore, Pakistan. She has vast experience of academic and research writing in basic and applied economics.
Mehr-Un- Nisa
Dr. Mehr-Un-Nisa is an assistant professor, Lahore College for Women University, Lahore, Pakistan. Her field of expertise includes public policy, econometric analysis, and macroeconomic modelling.
M. Riaz Akbar
Dr. M. Riaz Akbar completed his doctorate in urban planning from the University of Ulster, UK, and is serving in SNC Lavalin, Candu Energy Inc., Canada. He is also continuing his research in urban planning and management.
Notes
1. Agglomeration economies (AEs) are the unintended benefits that occur with the clustering of firms and people together in an area. These economies arise by improving productivity and job creation, specifically in manufacturing and services sectors.
2. Structural transformation is simply moving from low-productivity areas like agriculture to high productivity and value-added areas like industry.
3. Ten large cities include Karachi, Lahore, Faisalabad, Gujranwala, Multan, Rawalpindi, Hyderabad, Peshawar, Quetta and Islamabad with population size of over one million are also growing at a rate of over 3% annually, are emerging urban agglomerations (Appendix-A).
4. Urban World: Cities and the Rise of the Consuming Class. McKinsey Global Institute (MGI), 2017.
5. The study excluded Islamabad city from empirical analysis, because it is federally administered capital city of orPakistan. So the analysis is run on five cities of Punjab, instead of six.
6. Primary cities (With large Metropolitan areas).
7. Secondary Cities (With population over five million).
8. Small cities (with population of less than 300,000 inhabitants).
9. Medium cities (with 5 million or lesser inhabitants).
10. Localization economies occur when an increased size of industry in a city leads to increased productivity. However, urbanization economies occur when change in size of city leads to increase in productivity (Marshall, Citation1920).
11. The details of the construction of variables of study are given in Appendix-III.
12. Government statistics of migration towards cities are not available.
References
- Afzal, M., Ahmed, S. S., & Nawaz, M. (2018). Macroeconomic Determinants of Urbanization in Pakistan. Growth, 5(1), 6–23. https://doi.org/10.20448/journal.511.2018.51.6.12
- Ahmed, N., & Ishrat, S. (2020). Push and pull factors of internal migration in Balochistan province: A case study. Pakistan Journal of Applied Social Sciences, 11(1), 39–56. https://doi.org/10.46568/pjass.v11i1.435
- Arif, M., & Gupta, K. (2018). Mapping peri-urbanization in a non-primate city: A case study of Burdwan, India. European Economic Research, V(11), 6065–6081. https://euacademic.org/UploadArticle/3466.pdf
- Arif, M., Rao, D. S., & Gupta, K. (2019). Peri-urban livelihood dynamics: A case study from Eastern India. Forum Geographic, XVIII(1), 40–52. http://dx.doi.org/10.5775/fg.2019.012.i
- Arshad, S., Hu, S., & Ashraf, B. N. (2019). Zipf’s law and city size distribution: A survey of the literature and future research agenda. Physica A, 492, 75–92. https://doi.org/10.1016/j.physa.2017.10.005
- Awan, M. S., & Iqbal, N. (2010). Determinants of Urban poverty: The case of medium sized city in Pakistan, PIDE Working Paper: 60. Pakistan Institute of Development Economics (PIDE), Pakistan.
- Azhar, A., & Adil, S. (2016). Effect of agglomeration on socio-economic outcomes: A district level panel study of Punjab. The pakistan development review, papers and proceedings: The 32nd Conference of the Pakistan Society of Development Economists, 13-15th December, 2016, Islamabad, 155–170. https://www.jstor.org/stable/44986481.
- Baltagi, B. (2008). Econometric analysis of panel data. (Fifth ed.). John Wiley and Sons.
- Bosker, M., Park, J., & Roberts, M. (2020). Definition matters. Metropolitan areas and agglomeration economies in a large developing country. Journal of Urban Economics. https://doi.org/10.1016/j.jue.2020.103275
- Breinlisch, H. (2006). The spatial income structure in the European Union- What role for economic geography? Journal of Economic Geography, 6(5), 593–617. https://doi.org/10.1093/jeg/lbl018
- Burki, A. A., & Khan, M. A. (2011). Agglomeration economies and their effect on technical inefficiency of firms: Evidence from Pakistan. Working Paper. International Growth Center, 1–37.
- Campos, N., & Kinoshita, Y. (2008). Foreign direct investment and structural reforms: Evidence from Eastern Europe and Latin America. Working Paper: 26. Washington, DC: International Monetary Fund, IMF Institute.
- Chaudhry, A., & Haroon, M. (2015). The Economic Impact of New Firms in Punjab. The Lahore Journal of Economics, 20(Sep), 143–182.https://EconPapers.repec.org/RePEc:lje:journal:v:20:y:2015:i:sp:p:143-182
- Deb, S. (2017). City systems in South Asian urbanization and growth. Proceedings of the conference on Experiences and Challenges in Measuring Income, Inequality, and Poverty in South Asia. November 23-25, 2017, New Delhi, India.
- Duranton, G. (2015). A proposal to delineate metropolitan areas in Columbia. Desarrollo Y Sociedad, 75, 223–264. http://dx.doi.org/10.13043/DYS.75.6
- Fan, C. C., & Scott, A. J. (2003). Industrial agglomeration and development: A survey of spatial economic issues in East Asia and a statistical analysis of Chinese regions. Economic Geography, 79(3), 295–319. https://doi.org/10.1111/j.1944-8287.2003.tb00213.x
- Fujita, M. M., Krugman, P., & Venables, A. J. (1999). The spatial economy-cities, regions and international trade. the MIT Press. https://doi.org/10.7551/mitpress/6389.001.0001
- Fujita, M. M., & Thisse, J.-F. (2002). Economics of agglomeration-cities, industrial location and regional growth. Cambridge University.
- Gabaix, X. (1999). Zipf’s law and the growth of cities. The American Economic Review, 89(2), 129–132. https://doi.org/10.1257/aer.89.2.129
- Ghalib, A., Qadir, A., & Ahmad, S. R. (2017). Evaluation of developmental progress in some cities of Punjab, Pakistan, using urban sustainability indicators. Sustainability, 9(1473), 1–17. https://doi.org/10.3390/su9081473
- Glaeser, E. L. (2010). Agglomeration economies. In E. L. Glaeser (Ed.), National bureau of economic research (NBER) (pp. 1–14). The University of Chicago Press. http://www.nber.org/books/glae08-1
- Government of Pakistan. [GOP]. (2015). National report of Pakistan for habitat III. Ministry of Climate Change.
- Guilino, G., Kang, S., & Yuang, Q. (2019). Agglomeration Economies and Evolving Urban Form. The Annals of Regional Science, 63, 377–398. https://doi.org/10.1007/s00168-019-00957-4
- Haider, M., & Badami, M. G. (2010). Urbanization and local governance challenges in Pakistan. Environment and Urbanization Asia, 1(81), 81–96. https://doi.org/10.1177/097542530900100107
- Hansberg, & Wright. (2007). Urban structure and growth. Review of Economic Studies, 74, 597–624. https://doi.org/10.1111/j.1467-937X.2007.00432.x
- Hansen, G. H. (2005). Market potential, increasing returns, and geographic concentration. Journal of International Economics, 67(1), 1–24. 1-24. https://doi.org/10.1016/j.jinteco.2004.09.008
- Haroon, M. (2013). The effects of agglomeration on the formation and scale of operation of new firms. Center for Research in Economics and Business [CERB], Lahore School of Economics, Working paper number, 03-13.
- Hassan, M. S., Wajid, A., & Ahmed, K. (2012). Urbanization as a way to open economy: Empirical evidence from Pakistan. World Applied Sciences Journal, 20(7), 931–940. https://doi.org/10.5829/idosi.wasj.2012.20.07.2608
- Head, K., & Mayer, T. (2004). The empirics of agglomertion and trade. In V. Handerson & J.-F. Thisse (Eds.), Handbook of regional and urban economics (Edition. 1, Vol. 4, Chapter 59, pp. 2610–2669). Elsevier.
- Hirshman, A. (1958). The strategy of economic development. Yale University press.
- Holly, S., & Raissi, M. (2009). The macroeconomic effects of European financial development: A heterogeneous panel analysis. Working paper. DIW Berlin, German Institute of Economic Research, No. 1. 4.
- Hotelling, H. (1933). Analysis of a complex of statistical variables into principal components. Journal of Education Psychology, 24, 417–441. https://doi.org/10.1037/h0071325
- Imran, F., Nawaz, Y., Asim, M., & Hashmi, A. H. (2013). Socio-economic determinants of rural migrants in urban setting: A Study conducted at city Sargodha, Pakistan. Academic Journal of Interdisciplinary Studies, 2(1), 71–76. https://doi.org/10.5901/ajis/2013.v2n1p71
- Jolliffe, I. T. (1986). Principal component analysis and factor analysis. In I. T. Jolliffe (Ed.), Principal component analysis. Springer series in statistics (pp. 115–128). Springer. https://doi.org/10.1007/978-1-4757-1904-8-7
- Kaiser, H. F. (1960). The application of electronic computers to factor analysis. Educational and Psychological Measurement, 20, 141–151. https://doi.org/10.1177/001316446002000116
- Kaldor, N. (1970). The case of regional policies. Scttosh Journal of Political Economy, 17, 337–348.
- Kalwar, S., Dali, M. B., Hassan, N. B., Sahito, N., & Memon, I. A. (2016). Urbanization and secondary cities of Sindh Province: Towards more effective and sustainable cities. Proceedings of the conference on “Energy, Environment and Sustainable Development 2016”.
- Kanwal, H., Naveed, T. A., & Khan, M. A. (2015). Socio-economic determinants of rural-urban migration in Pakistan. Journal of Asian Development Studies, 4(3), 72–85. Corpus ID: 46642429.
- Kaya, A., & Koc, M. (2019). Over-Agglomeration and its effects on sustainable development: A case study of Istanbul. Sustainability, 11(135), 1–23. https://doi.org/10.3390/su11010135
- Khan, A. A., Arshad, S., & Mohsin, M. (2014). Population growth and its impact on urban expansion: A case study of Bahawalpur, Pakistan. Universal Journal of Geoscience, 2(8), 229–241. https://doi.org/10.13189/ujg.2014.020801
- Khan, A. U., Saboor, A., Ali, I., Malik, W. S., & Mehmood, K. (2016). Urbanization of multidimensional poverty: empirical evidences from Pakistan. Quality & Quantity, 50, 439–469. https://doi.org/10.1007/s11135-014-0157-x
- Khan, N. Y., Ghafoor, N., Iftikhar, R., & Maalik, M. (2012). Urban annoyances and mental health in the City of Lahore, Pakistan. Journal of Urban Affairs, 34(3), 297–315. https://doi.org/10.1111/j.1467-9906.2011.00585.x
- Krugman, P. (1991). Geography and Trade. MIT Press.
- Kugelman, M. (2013). Urbanization in Pakistan: Causes and consequences. NOREF-Norwegian Peacebuilding Resource Centre.
- Latif, A., & Yu, T.-F. (2020). Resilient urbanization in Pakistan: A systematic review on urban discourse in Pakistan. Urban Science, 4(76), 1–20. https://doi.org/10.3390/urbansci4040076
- Loayza, N. V., & Ranciere, R. (2006). Financial development, financial fragility and growth. Journal of Money, Credit, and Banking, 38(4), 1051–1076. https://doi.org/10.1353/mcb.2006.0060
- Malik, S., Roosli, R., & Tariq, F. (2020). Investigation of informal housing challenges an experience from slums and squatter of Lahore. Journal of Housing and the Built Environment, 35, 143–170. https://doi.org/10.1007/s10901-019-09669-9
- Marshall, A. (1920). Principles of Economics. Macmillan.
- McCann, P., & Oort, F. V. (2019). Theories of agglomeration and regional economic growth: A historical review. Handbook of regional growth and development theories (2nd ed., pp. 6–23). Edward Elgar.
- Melo, P. C., Graham, D. J., Noland, R. B. (2009). A Meta-Analysis of the Estimates of Urban Agglomeration Economies. Regional Science and Urban Economics, 39, 332–342. https://doi.org/10.1016/j.regsciurbeco.2008.12.002
- Myrdal, G. (1957). Economic theory and under-developed regions. Duckworth.
- Nasir, M. (2017). Agglomeration and firm turnover in Punjab. The Lahore Journal of Economics, 22(1), 19–36.
- Ovsiannikova, T., Nikolaenko, M., & Chilbakova, M. (2018). Expansion of urban recreation areas as a factor for human capital renovation. MATEC Web of Conferences, 143, 04011, YSSIP–2017. https://doi.org/10.1051/matecconf/201714304011
- Pakistan Economic Survey. [PES]. (2018-19). Government of Pakistan.
- Pearson, K. (1901). On lines and planes of closest fit to systems of points in space. Philos Mag, 2, 559–572. https://doi.org/10.1080/14786440109462720
- Pesaran, M. H., Shin, Y., ., & Smith, R. J. (1999). Pooled mean group estimation of dynamic heterogeneous panels. Journal of the American Statistical Association, 94(446), 621–634. https://doi.org/10.2307/2670182
- Pesaran, M. H., Shin, Y., & Smith, R. J. (2001). Bound testing approaches to the analysis of level relationships. Journal of Applied Econometrics, 16, 289–326. https://doi.org/10.1002/jae.616
- Pesaran, M. H., & Smith, R. J. (1995). Estimating long-run relationships from dynamic heterogeneous panels. Journal of Econometrics, 68(1), 79–113. https://doi.org/10.1016/0304-4076(94)01644-F
- Phillips, P. C., & Hansen, B. E. (1990). Statistical inference in instrumental variables regression with I (1) processes. The Review of Economic Studies, 57(1), 99–125. https://doi.org/10.2307/2297545
- Punjab growth strategy, government of Punjab, Pakistan. [GOP] (2018). Planning & Development Department, Government of Punjab, Pakistan.
- Rana, I. A., & Bhatti, S. S. (2018). Lahore, Pakistan-urbanization challenges and opportunities. Cities, 72, 348–355. http://dx.doi.org/10.1016/j.cities.2017.09.014
- Redding. (2009). The empirics of new economic geography. Globalization Programme at the ESRC, Center for Economic Performance, London School of Economics. Houghton Street, London, WC2A 2AE, United Kingdom.
- Rosenthal, S. S., & Strange, W. C. (2004). Evidence on the nature and sources of agglomeration economies. In J. V. Henderson & J. Thisse (Eds.), Handbook of urban and regional economics (Vol. 4, pp. 2119–2171). Elsevier
- Rosenthal, S. S., & Strange, W. C. (2001). The determinants of agglomeration. Journal of Urban Economics, 50, 191–229. https://doi.org/10.1006/juec.2001.2230
- Rosenthal, S. S., Strange, W. C. (2003). Geography, Industrial Organization and Agglomeration. The Review of Economics and Statistics, 85(2), 377–393.
- Social Policy and Development Center. [SPDC] (2016). Social Development in Pakistan: Annual Review 2014-15. Karachi, Pakistan.
- Sun, X., Wang, W., Sun, T., & Wang, Y. P. (2018). Understanding the living conditions of Chinese Urban neighborhood through social infrastructure configurations: The case study of Tianjin. Sustainability, 10(3243), 1–17. https://doi.org/10.3390/su10093243
- The Urban Unit. (2018). The urban gazette. 503-Shaheen complex, Edgerton Road, Lahore.
- UN-Habitat. (2016). Urbanization and development: Emerging futures. World Cities Report-2016. Nairobi: United Nations Human Settlements Programme.
- UNICEF. (2020). Report of profiles of slums and underserved areas of five largest cities of Punjab, Pakistan. United Nation Children’s Fund [UNICEF].
- United Nations. (2019). World urbanization prospects. Department of Economic and Social Affairs, Population Division, United Nations, New York, 2019.
- United Nations Development Program [UNDP] for Pakistan (2018). Sustainable urbanization: Development Advocate, Pakistan. Vol.5, issue 4, Islamabad, Pakistan.
- United Nations Environment Programme [UNEP] (2013). The environment and climate change outlook of Pakistan. Regional office for Asia and the Pacific.
- Weisbrod, G., Vary, D., & Treyz, G. (2003). Measuring Economic Costs of Urban Traffic Congestion to Business. Journal of the Transportation research Board, 1839(1). https://doi.org/10.3141/1839-10
- World Bank. (2016). Leveraging urbanization in South Asia: Managing spatial transformation for prosperity and livability.
- World Bank. (2019). Opportunities for a clean and green Pakistan- A country environmental analysis.
Appendices
Appendix-I: Population Size and Growth of the Major Cities of Punjab, Pakistan
Appendix-II: Percentage Distribution of Migrants by Place of Present and Previous Residence
Appendix-III:
Urban Agglomerations in Pakistan
Appendix-D:
Inter-Censal Population Growth in Major Cities of the Punjab, Pakistan
Source: Authors
Appendix-IV:
Expansion of Major Cities of the Punjab from 1995 to 2015
Source: The Urban Unit, Lahore (2019)