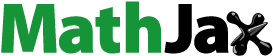
Abstract
Exchange Traded Funds (ETFs) have proven to be extremely popular amongst both retail and institutional investors. The increasing interest in this asset class may incite overconfidence in its’ investor base, which could lead to undesirable market effects such as security mispricing, excess trading volumes, and exacerbated market volatility. This study aims to examine the South African ETF market for presence of investor overconfidence. To achieve this objective, Vector Autoregression (VAR) models and their associated impulse response functions are employed to examine the relationship between the current trading activity and the historical market return. Consistent with the overconfidence hypothesis, a positive and significant relationship between current market turnover and lagged market returns is found for both ETFs with domestic benchmarks and ETFs with international benchmarks. Further analysis of panel VAR models and their associated impulse response functions suggest that the overconfidence bias also influences the trading activities of individual ETFs. These findings have important implications for various market participants.
PUBLIC INTEREST STATEMENT
This study investigates the presence of investor overconfidence in the South African ETF market which, to the knowledge of the authors, has never been done before. This study, therefore, provides an understanding of which biases drive trade decisions in the South African ETF market. By doing so, the results of this study may assist investors in making rational investment choices by reducing the influence of behavioural biases on their trade decisions. Hence, these findings may assist investors and investment management companies in developing strategies that reduce their risk exposures whilst increasing their opportunities for generating high returns. Furthermore, the findings of this study may aid policymakers in developing and implementing policies that seek to improve the efficiency of the ETF market by reducing the behavioural biases that are present in the ETF market.
1. Introduction
In recent years, the investment management landscape has been transformed by the arrival of Exchange Traded Funds (ETFs). An ETF is a pooled fund, based on a preselected basket of securities, which attempts to replicate the risk and return characteristics of a specific benchmark or index (Strydom et al., Citation2015). These ETFs provide access to different financial assets via a single entry point (Petajisto, Citation2017), thus facilitating easier diversification for investors. The different asset classes which can be replicated include corporate bonds, real estate and commodities, with some of these being previously accessible to only institutional investors or investors with a high net worth (Padungsaksawasdi & Daigler, Citation2014). ETFs, therefore, signify a low-cost, easily accessible transformation of an index fund, which also has the added advantage of trading flexibility, as these ETFs can be traded throughout the trading day, can be purchased on margin, or sold short (Mohamad et al., Citation2016; Venkataraman & Venkatesan, Citation2016). It is, therefore, commonly acknowledged that ETFs have become a popular asset class globally, as it represents a cheaper and more convenient saving and investment mechanism (Deev & Linnertová, Citation2014; Aldridge, Citation2016; Da & Shive, Citation2018).
Whilst the inception of the ETF has led to many improvements to the financial market, there is also growing evidence that this market tends to attract mostly short-term (noise) traders, which indicates that that the trade decisions of ETF investors may not always be rational (Ben‐David et al., Citation2018; Broman, Citation2016; Da & Shive, Citation2018; Madura & Richie, Citation2004). Behavioural studies such as Ma et al. (Citation2018) report evidence of the presence of investor overreaction in ETF markets while Bahadar et al. (Citation2019) report evidence of the presence of investor herd behaviour in ETF markets. These irrational ETF investment decisions may cause investors to allow their emotions to overrule logic, and subsequently, these investors may trade too aggressively, have bad market timing, or miscalculate the probability of their success (Chen et al., Citation2007). As a result, there could be an increase in the volatility of ETF returns and trading volumes which could adversely impact the efficiency of ETF markets. Therefore, it is possible that the exponential growth of ETF markets could pose a threat to global financial systems by creating instabilities in broader financial markets because ETFs have exposures to a variety of asset classes, including real estate, bonds, commodities, stocks, and currencies.
Other evaluations of the equity market have overwhelmingly found evidence of the overconfidence biasFootnote1 affecting investment decisions (Baker et al., Citation2019; Jlassi et al., Citation2014; Meier & de Mello, Citation2020; Zaiane & Abaoub, Citation2009). The presence of investor overconfidence has been examined for markets of different asset classes, including stocks (Gupta et al., Citation2018), commodities (Yung & Liu, Citation2009), real estate (Lin et al., Citation2010) and index funds (Bailey et al., Citation2011). Kansal and Singh (Citation2018) mention that the overconfidence bias is probably the most scrutinised behavioural bias in terms of its existence, determinants, and effects. However, to the authors’ knowledge, the presence of investor overconfidence in ETF markets has not been investigated. Therefore, the objective of this study is to determine whether investors are overconfident when trading in the South African ETF market.
The motivation for investigating the presence of investor overconfidence in ETF markets stem from the exponential growth of ETF markets in recent years. Specifically, if investors generate positive returns from investing in ETFs then they may attribute these returns to their own abilities, and as a result, these investors may become overconfident in their security valuation skills. ETFs remain a popular investment vehicle in South Africa and, as a result, the South African ETF market has grown exponentially in terms of its size and number (Steyn, Citation2019). Furthermore, the choice of the South African ETF market is supported by the findings of Griffin et al. (Citation2007). Specifically, Griffin et al. (Citation2007) report that overconfident trading is more prevalent in countries with high levels of corruption and market volatility. Given that South Africa exhibits both high levels of corruption (Salahuddin et al., Citation2020) and market volatility (Redl, Citation2018), it is plausible to expect overconfident trading by South African investors.
Several anomalies that are present in financial markets may be explained by the presence of investor overconfidence. Therefore, overconfident trading in ETF markets poses a threat to the stability of ETF markets and, thus, broader financial markets. This is because, by overweighting their own private signals relative to the general market consensus, overconfident traders drive asset prices away from their intrinsic values, subsequently, creating excess market volatility by distorting asset prices. Notably, these price distortions could lead to asset price bubbles (Scheinkman & Xiong, Citation2003). Hence, the findings of this study have significant implications for policymakers and regulators who are responsible for promoting the efficiency of ETF markets. Specifically, plausible explanations for the deviation of ETF prices from their fundamental values may be identified by analysing financial data from the perspective of behavioural finance. Therefore, policymakers and regulators can use the results of this study to develop and implement policies that enhance the efficiency of ETF markets by reducing the behavioural biases present in ETF markets.
Overconfident investors may underestimate their risk exposures or continue trading even when the costs outweigh the gains (Trinugroho & Sembel, Citation2011). As such, the findings of this study also have notable implications for investors and investment management companies. Specifically, investors and investment management companies can increase their opportunities for generating higher returns and reduce their risk exposures by understanding which behavioural biases influence investors’ trade decisions. Overall, this study fills a significant gap in existing research by examining whether investors are overconfident when trading in the South African ETF market which, to the knowledge of the authors, has not been studied before.
In this study, the presence of investor overconfidence in the South African ETF market is examined using a sample of South African ETFs tracking domestic benchmarks and a sample of ETFs tracking international benchmarks. This sample division is motivated by the findings of Johnson (Citation2009) and Steyn (Citation2019) who report that ETFs with international benchmarks exhibit higher tracking errors due to unsynchronised trading times, dividend policies, and the volatility of exchange rates. Given that tracking ability is an important factor when making ETF investment decisions, it is plausible to expect that ETFs with domestic and international benchmarks exhibit different trading patterns and, therefore, combining them into one market portfolio could distort the results of this study. For both sample divisions, the results of the Vector Autoregression (VAR) models and their associated impulse response functions indicate that market turnover significantly and positively responds to historical market returns and, thus, provides evidence in support of the presence of investor overconfidence in both the market of ETFs with domestic benchmarks and the market of ETFs with international benchmarks. Further analysis of panel VAR models and their associated impulse response functions reveal that the overconfidence bias influences the trading of individual ETFs and, thus, the market-wide overconfidence found is not a direct summation of the disposition effect.
The rest of the paper is structured as follows: Section 2 reviews existing literature surrounding the presence of investor overconfidence in different financial markets. Section 3 outlines the data and methodology employed in this study. Section 4 presents and discusses the findings of this study. Lastly, Section 5 presents the concluding remarks and recommendations for future studies.
2. Literature review
2.1. Theoretical considerations
Traditional finance theories assume that investors’ trade decisions are rational and, therefore, capital markets are efficient. On the contrary, behavioural finance theories argue that capital markets are not always efficient because investors’ trade decisions could be influenced by investors’ state of minds, beliefs and emotions (Kapoor & Prosad, Citation2017). One of the most pronounced behavioural biases influencing investors’ trade decisions is the overconfidence bias (Baker et al., Citation2019; Meier & de Mello, Citation2020; Trejos et al., Citation2019). Moore and Healy (Citation2008) mention that overconfidence can manifest in three different forms, namely; overestimation, overplacement, and overprecision.
Overestimation occurs when individuals overestimate their own abilities, probability of success, or degree of control (Moore & Schatz, Citation2017). Overestimation may be caused by an illusion of control which occurs when individuals have unwarranted beliefs that they have a higher degree of control and chance of success (Langer, Citation1975) or by the planning fallacy which occurs when individuals are too optimistic about the time it takes them to complete tasks (Buehler et al., Citation1994). Overplacement refers to tendency of individuals to believe that they are better than others (Pikulina et al., Citation2017). Overplacement may be caused by the ‘better-than-average effect’ which occurs when individuals appraise themselves more favourably than others (Alicke, Citation1985) or by the notion of unrealistic optimism which occurs when individuals believe that they are not victims of misfortune (Weinstein, Citation1980). Finally, overprecision, which is the most relevant to this study, refers to the tendency of individuals to exaggerate the accuracy of their information and knowledge, and is caused by subjective probability distributions which are too narrow when individuals omit alternative possibilities from the event space (Alpert & Raiffa, Citation1982). Overall, investor overconfidence is broadly defined as the tendency of investors to overrate the precision of their knowledge and security valuation skills (Kansal & Singh, Citation2018).
According to Odean (Citation1998), the level of trading volume increases as the level of overconfidence rises. This is because investors who are overconfident assume that their private information is more reliable than publicly available information, and as a result, they overreact to subjective and less relevant information but underreact to abstract and highly relevant information (Odean, Citation1998). Therefore, overconfident investors trade in riskier securities because they underestimate their risk exposures (Barber & Odean, Citation1999). Whilst this may result in higher returns being earned, it could also be hazardous to investors’ wealth because investors who are overconfident may continue to trade even when the trading costs exceed the trading gains (Odean, Citation1999). Barber and Odean (Citation2001) mention that overconfidence is more pronounced in males relative to females whilst Gervais and Odean (Citation2001) note that the level of overconfidence decreases as an investor acquires more trading experience.
The overconfidence hypothesis proposed by Gervais and Odean (Citation2001) postulates that investors attribute market gains to their own abilities, and consequently, investors become more overconfident in periods after positive market returns. This being so, periods of positive market returns tend to be followed by increased trading volume because overconfident investors trade more aggressively in periods after positive market returns. Hence, Statman et al. (Citation2006) assert that, in the presence of investor overconfidence, there is a positive relationship between the current trading volume of the market and the historical return of the market. Notably, Chuang and Susmel (Citation2011) contend that investor overconfidence is more pronounced in bull markets during which most stock generate positive returns in comparison to bear markets during which most stocks generate negative returns.
Investor overconfidence can either enhance or worsen market efficiency depending on the manner in which information is distributed to markets (Yeh & Yang, Citation2014). According to Odean (Citation1998), investor overconfidence can improve price quality by revealing more information to the market or it can worsen the quality of prices if investors have heterogenous beliefs about security prices. Overconfident investors with heterogenous beliefs overreact to their own private signals and trade without reference to fundamental information, subsequently, causing security prices to deviate from their fundamental values (Scheinkman & Xiong, Citation2003). Therefore, overconfident trading creates excess volatility in financial markets which may result in the formation of price bubbles (Scheinkman & Xiong, Citation2003). Whilst overconfident trading has several drawbacks, Liu (Citation2015) mentions that the aggressive trading by overconfident trades helps to enhance the liquidity of financial markets.
2.2. Empirical evidence of investor overconfidence
The overconfidence hypothesis proposed by Gervais and Odean (Citation2001) asserts that investors who are overconfident associate positive market returns with their own security-picking abilities and, therefore, trade more aggressively in periods after market gains. As a result, when investor overconfidence is present, historical market returns positively influence current trading activities. In their seminal study of investor overconfidence, Statman et al. (Citation2006) test the overconfidence hypothesis by examining how historical market returns impact current trading activity using Vector Autoregression (VAR) models and their associated impulse response functions. Statman et al. (Citation2006) observe all common stocks trading on the New York Stock Exchange (NYSE) from August 1962 to December 2002. Consistent with the overconfidence hypothesis, Statman et al. (Citation2006) report evidence of a positive relationship between current market turnover and lagged market return.
The authors thereafter postulate that it is also important to distinguish between the overconfidence bias and the disposition effect since both biases are associated with a positive relationship between current trading volume and lagged return.Footnote2 To differentiate between investor overconfidence and the disposition effect, Statman et al. (Citation2006) examine the relationship between the turnover of individual securities and lagged market return. The study reports that individual security turnover is positively related to lagged market return suggesting that, even after controlling for the disposition effect, the trading activity of individual stocks is positively influenced by market return-induced investor overconfidence.
Several studies employ the Statman et al. (Citation2006) model and report similar findings for stock markets. Whilst Griffin et al. (Citation2007) observe 46 different stock markets and find strong evidence of investor overconfidence, studies by Zia et al. (Citation2017) and Alsabban and Alarfaj (Citation2020) also find evidence of overconfidence on the Pakistani and Saudi equity markets, respectively. On the contrary, Zaiane and Abaoub (Citation2009) find weak evidence of investor overconfidence in the Tunisian stock market. Overall, Chuang and Lee (Citation2006), Abbes (Citation2013), and Jlassi et al. (Citation2014) report that trading volume induced by overconfident trading is positively related to market volatility. On the contrary, Sheikh and Riaz (Citation2012) find that trading volume induced by investor overconfidence is not related to the volatility of the Karachi stock market.
Chuang and Susmel (Citation2011) analyse the Taiwan Stock Exchange (TWSE) from 1996 to 2005 and report that investor overconfidence is more pronounced in individual investors in comparison to institutional investors, in which case, individual investors are more overconfident in bull markets relative to non-bull markets. Similar, Metwally and Darwish (Citation2015) report that investor overconfidence is present in the Egyptian Exchange (EGX) from 2002 to 2012, however, investor overconfidence is more pronounced during bullish market conditions. Gupta et al. (Citation2018) find that both Indian and Chinese stock market investors are overconfident, however, Chinese investors are more overconfident than Indian investors. Gupta et al. (Citation2018) further report that both Indian and Chinese investors are overconfident before the 2008 global financial crisis, however, only Chinese investors remained overconfident after the crisis.
Although the majority of studies have investigated the presence of investor overconfidence in stock markets, existing research on the presence of investor overconfidence in markets for alternative asset classes remain scanty and with inconsistent results. Yung and Liu (Citation2009) examine the U.S. futures market for commodities (gold, natural gas, copper, silver, unleaded gas, and crude oil) from 1995 to 2006 and discover that investor overconfidence is not present in all the observed commodity futures markets. On the contrary, Aharon and Qadan (Citation2018) examine the U.S. commodities markets for palladium, gold, platinum, zinc, silver and oil from 2004 to 2017 and find that investor overconfidence influences the trade decisions of investors trading in all the observed commodities except platinum and zinc.
Bailey et al. (Citation2011) investigate the U.S. mutual fund industry from January 1991 to November 1996 and report that the trade decisions of mutual fund investors are influenced by the overconfidence bias. On the contrary, Dowie and Willows (Citation2016) report that South African unit trust investors are underconfident rather than overconfident. Lin et al. (Citation2010) observe all Real Estate Investment Trusts (REITs) in the Center for Research in Security Prices (CRSP) database from 1990 to 2006 and discover that the turnover of the REIT market is positively influenced by investor overconfidence induced by the return of the REIT market. However, the turnover of the REIT market is not significantly influenced by investor overconfidence induced by the return of the stock market. Likewise, Chen and Sabherwal (Citation2019) examine the U.S. equity options market from 1996 to 2005 and report that investor overconfidence is present in the U.S. equity options market.
There is no existing study (to the authors’ knowledge) that specifically tests for the presence of investor overconfidence in the market for ETFs. However, Da Dalt et al. (Citation2019) examine the presence of contrarian behaviour in the Helsinki Stock Exchange (OMXH) and document that Finnish investors display less contrarian behaviour when trading in ETFs relative to trading in common stocks. According to Da Dalt et al. (Citation2019), these findings indicate that investors are less overconfident in their ability to identify undervalued ETFs. This study, therefore, fills a gap in existing literature by testing for the presence of investor overconfidence in the ETF market, specifically, the South African ETF market. The next section outlines the data and methodology employed in this study.
3. Data and methodology
3.1. Data
This study aims to investigate the presence of investor overconfidence in the South African ETF market. However, Kyrychenko and Shum (Citation2009) find that investors are more overconfident when trading in assets with foreign exposures because they overestimate their knowledge about assets with foreign exposures. On this premise, it is plausible to expect that investors trading in South African ETFs tracking international benchmarks are more overconfident than investors trading in South African ETFs tracking domestic benchmarks. On the contrary, Steyn (Citation2019) finds that South African ETFs replicating domestic benchmarks track their benchmarks more efficiently relative to South African ETFs replicating international benchmarks. Given that South African ETFs tracking domestic benchmarks exhibit higher tracking efficiency, it is plausible to expect that investors may be more overconfident when trading in South African ETFs tracking domestic benchmarks. Hence, the current analysis of investor overconfidence in the South African ETF market is segmented into a sample of ETFs tracking domestic benchmarks and a sample of ETFs tracking international benchmarks.
Given that the first South African ETF with a domestic benchmark was launched in November 2000 and the first South African ETF with an international benchmark was launched in October 2005, the sample periods for the market of South African ETFs with domestic benchmarks and the market of South African ETFs with international benchmarks starts in November 2000 and October 2005, respectively. The sample periods end in August 2019 for both markets. In order to avoid the survivorship bias, delisted ETFs are also included in this study’s sample. However, only ETFs that were registered on the Johannesburg Stock Exchange (JSE) for at least 30 consecutive months are included in the sample. This results in a total sample of 55 South African ETFs which includes 49 of the 78 listed ETFs and 6 of the 9 delisted ETFs as at 30 August 2019. Notably, data that is of a monthly frequency is employed in this study because Odean (Citation1999), Gervais and Odean (Citation2001), and Statman et al. (Citation2006) argue that investor overconfidence is more evident over monthly horizons. Secondary data relating to closing prices, number of shares traded, and number of shares outstanding are obtained from the IRESS database whilst data on dividends paid are obtained from the Infront Analytics database.
3.2. Methodology
The overconfidence hypothesis proposed by Gervais and Odean (Citation2001) asserts that historical market returns positively influences current trading activity in the presence of investor overconfidence. Accordingly, the presence of investor overconfidence is detected by examining the relationship between historical market returns and current trading activity in the market. However, Statman et al. (Citation2006) argue that the number of outstanding shares fluctuates over time and, therefore, needs to be taken into account when examining the trading activity in a market. As such, the turnover ratio is used as a proxy of trading activity in this study.
Following Statman et al. (Citation2006), the presence of market-wide investor overconfidence is investigated by examining the relationship between current market turnover and lagged market returns using Vector Autoregressive (VAR) models and their associated impulse response. Notably, this study’s analysis is conducted on two markets, specifically, the market of South African ETFs tracking domestic benchmarks and the market of South African ETFs tracking international benchmarks, and the VAR model for each market follows the following specification:
In EquationEquation (1)(1)
(1) above, the endogenous variables are market turnover (
) and market return (
). The market turnover for month
,
, is computed as the average turnover on a market value-weighted portfolio which comprises of all ETFs in the respective market at time t.Footnote3 The market return for month
,
, is computed by aggregating the daily dividend-adjusted returns on the market value-weighted portfolio comprising of all ETFs in the respective market. The exogenous variables in EquationEquation (1)
(1)
(1) are market return volatility (
) and market return dispersion (
) which are included to control for alternative explanations of trading volume, such as, the volume-volatility relationship and potential rebalancing activities, respectively. The market return volatility for month
,
, represents the monthly realized volatility of the returns from the value-weighted ETF market portfolio.Footnote4 The market return dispersion for month
,
, represents the cross-sectional standard deviation of the market returns.
In EquationEquation (1)(1)
(1) ,
and ε represent vectors of intercepts and residuals, respectively whilst
and
represent coefficient estimates. The optimal lag lengths of the endogenous and exogenous variables are denoted by
and
, respectively. Formal overconfidence theories do not specify a time period for the lead-lag relationship between current market turnover and lagged market returns, therefore, the optimal lag lengths of the endogenous and exogenous variables are selected using the information criterion. UnreportedFootnote5 information criterion values select
= 6 and
= 4 for the VAR model estimated for market of ETFs replicating domestic benchmarks and,
= 3 and
= 7 for the VAR model estimated for market of ETFs replicating international benchmarks. Notably, upon estimation of the respective VAR models for each market sample, a positive and significant relationship between current market turnover (
) and lagged market return (
) is consistent with the overconfidence hypothesis introduced by Gervais and Odean (Citation2001). A generalized least squares (GLS) approach is used to estimate the models’ parameters.
As noted before, a positive relationship between current trading activity and lagged returns is consistent with the overconfidence hypothesis, as well as the disposition effect. Therefore, it is difficult to completely disentangle the overconfidence bias from the disposition effect (Statman et al., Citation2006). Despite this drawback in interpretation, an analysis of the trading activity of individual ETFs will confirm whether or not the observed overconfidence effect is a direct sum of the aggregate disposition effect (Gupta et al., Citation2018; Statman et al., Citation2006).
Following Statman et al. (Citation2006), an individual security VAR model is estimated to ensure that the observed overconfidence effect is not a direct summation of the aggregate disposition effect. However, the individual security VAR model follows a panel data approach, and each panel contains all individual ETFs in the respective market, that is, the market of South African ETFs tracking domestic benchmarks and the market of South African ETFs tracking international benchmarks. The individual security VAR model follows the following specification:
In EquationEquation (2)(2)
(2) , there are three endogenous variables:
which represents the turnover of the
th ETF for month
,
which denotes the return on the
th ETF for month
, and
which represents ETF
’s respective market return for month
. The single exogenous variables in EquationEquation (2)
(2)
(2) is
which represents the volatility of ETF
’s return for month
. Market dispersion, which controls for portfolio rebalancing activities, is dropped as a control variable because the individual security VAR model is estimated for individual ETFs in the respective market.
and
represent coefficient estimates while
and
denote the optimal lag lengths of the endogenous and exogenous variables, respectively. Notably, the optimal lag lengths of the endogenous and exogenous variables in the individual security panel VAR models follow the optimal lag lengths which was selected for the respective market VAR models. According to Statman et al. (Citation2006), this uniformity in the lag length ensures consistency in the comparison of individual ETFs since the optimal lag lengths may vary substantially across individual ETFs. The models’ parameters are estimated using the GLS estimator.
The disposition effect is associated with investors’ attitudes towards individual securities in their portfolios, and therefore, a positive relationship between current individual security turnover () and lagged individual security return (
) provides evidence of the presence of the disposition effect (Statman et al., Citation2006). On the contrary, the overconfidence bias is associated with investors’ attitudes towards the general ETF market, and therefore, a positive relationship between current individual security turnover (
) and lagged market return (
) provides evidence of the presence of the overconfidence bias (Metwally & Darwish, Citation2015).
According to Swanson and Granger (Citation1997) and Dewachter et al. (Citation2015), individual VAR coefficients do not fully capture the influence of other variables in the system. On the contrary, impulse response functions fully capture the effect of other variables in the system because they trace the effect of a residual shock by using all the VAR coefficient estimates. Therefore, to supplement the results of the VAR models specified in EquationEquations (1)(1)
(1) and (Equation2
(2)
(2) ), impulse response functions associated with these models are illustrated and analysed.
4. Empirical results
This section is twofold. Firstly, the regression results of the VAR models estimated to examine the presence of market-wide investor overconfidence are presented and discussed. Thereafter, the regressions results of the panel VAR models estimated to investigate the influence of investor overconfidence on the trading of individual ETFs in the market are presented and analysed. Notably, preliminary diagnostics testsFootnote6 indicate that heteroskedasticity and autocorrelation is not present in the estimated VAR and panel VAR models.
4.1. Market-wide investor overconfidence
The VAR model specified in EquationEquation (1)(1)
(1) is estimated for the market of South African ETFs tracking domestic benchmarks and for the market of South African ETFs tracking international benchmarks and the results are presented in and , respectively. In , the dependent variables (
and
) appear in rows whilst the coefficients for the lagged dependent and exogenous variables are organised in columns.
Table 1. VAR model estimates for the market of ETFs with domestic benchmarks
Table 2. VAR model estimates for the market of ETFs with international benchmarks
The results presented in indicate that, after controlling for lagged market turnover, market volatility, and market dispersion, market turnover is dependent on lagged market returns. Specifically, when market turnover is the dependent variable in the VAR model estimated for the market of ETFs with domestic benchmarks, the first and second lagged market return coefficients are equal to 0.1143 and 0.1500 and are statistically significant at a 10% and 5% level of significance, respectively. However, the lagged market return coefficients are not statistically significant from the third and higher lags. Similarly, for the market of ETFs with international benchmarks, the first and third lagged market return coefficients are not statistically significant. However, the second lagged market return coefficient is equal to 0.1037 and is statistically significant at a 5% level of significance.
For both, the market of South African ETFs with domestic benchmarks, as well as the ETFs with international benchmarks, the positive and statistically significant relationship between current market turnover and lagged market returns provides evidence in support of the overconfidence hypothesis, thus, indicating the presence of investor overconfidence in the respective markets. This key empirical finding is discussed further in the context of impulse response functions.
also suggest that the market turnover in both markets is autocorrelated since the current market turnover is significantly dependent on lagged market turnover coefficients. With regards to the exogenous variables, shows that the current market turnover in the market of ETFs with domestic benchmarks is significantly dependent on lagged market volatility and market dispersion. Likewise, indicates that the current market turnover in the market of ETFs with international benchmarks is significantly dependent on lagged market dispersion and both current and lagged market volatility.
For completion, show that there exists significant explanatory variables in the regressions with market return as the dependent variable, thus, indicating that the surveyed ETF markets are not even weak-form efficient. Specifically, for the market of ETFs with domestic benchmarks, market return is significantly dependent on historical market return and market volatility and both current and lagged market dispersion. Notably, for the market of ETFs with international benchmarks, market return is only significantly dependent on the lagged exogenous variables, that is, lagged market volatility and market dispersion. Notably, lagged market turnover is an insignificant explanatory variable for the market return of both markets.
In order to supplement the results of the VAR models presented in , the associated impulse response functions are illustrated for the market of ETFs with domestic and international benchmarks in and , respectively.
Figure 1. Impulse response functions for the VAR model estimated for the market of ETFs with domestic benchmarks

Figure 2. Impulse response functions for the VAR model estimated for the market of ETFs with international benchmarks

In , particular importance is given to Panel B which illustrates the response of market turnover to market return. Panel B in both figures illustrates that, for both markets, a one standard deviation shock in market return does not generate any response in the market turnover during the next month. Remarkably, market turnover responds positively to a one standard deviation shock in market return from the second month, and this positive response remains for at least 10 months in both markets. Notably, only the third period response of market turnover to market return is statistically significant in the market of ETFs with domestic benchmarks whilst, in the market of ETFs with international benchmarks, the third and sixth period responses of market turnover to market return are statistically significant.
Overall, for both markets, the positive and statistically significant response of market turnover to shocks in market return provides evidence in support of a positive and significant lead-lag relationship between current trading activity and historical market returns. This positive relationship between current trading activity and historical market returns indicates that an increase (decrease) in historical market returns leads to an increase (decrease) in current trading activity because investors’ confidence in their security valuation skills increases (decreases) after periods of market gains (losses). Therefore, the results of the VAR models and the impulse response functions accept the overconfidence hypothesis proposed by Gervais and Odean (Citation2001) which asserts that an increase in historical market returns produces overconfident investors who trade more aggressively after periods of market gains.
These findings indicate that investor overconfidence is present in both the market of South African ETFs tracking domestic benchmarks and in the market of South African ETFs tracking international benchmarks. This presence of investor overconfidence in the South African ETF market suggests that the high trading volume present in the South African ETF market may be caused by overconfident investors who believe that their own skills and signals are more reliable than it actually is (Odean, Citation1998). When investors are overconfident in their own abilities, they tend to underestimate their forecast errors (Daniel et al., Citation1998). Hence, this influence of the overconfidence bias on investment decisions made by investors trading in the South African ETF market indicates that investors are irrational, and that the South African ETF market is not efficient. Furthermore, Daniel et al. (Citation1998) argue that the presence of overconfident trading may lead to excess market volatility and, therefore, the overconfidence present in the South African ETF market could further deteriorate the efficiency of the South African ETF market, and could fuel the formation of ETF price bubbles. Nevertheless, the presence of investor overconfidence in the South African ETF market is consistent with the findings from alternative asset classes, such as, mutual funds (Bailey et al., Citation2011), REITs (Lin et al., Citation2010), and stocks (Statman et al., Citation2006). On the contrary, whilst Dowie and Willows (Citation2016) document that South African unit trust investors are underconfident, this study finds that South African ETF investors are overconfident. This inconsistency may be because, relative to ETFs, unit trusts are more costly to trade on average (Steyn, Citation2019).
For completion, Panel A in both indicate that, for both markets, there are periods in which the market turnover significantly responds to its own shocks. Likewise, Panel D in both indicate that, for both markets, there are periods in which the market return significantly responds to its own shocks. Noteworthy is that the significant, positive autocorrelation of the market returns suggests the presence of a momentum anomaly which could last for up to 7 months in the market of ETFs with domestic benchmarks but only 1 month in the market of ETFs with international benchmarks. Notably, Panel C in both show that market return does not significantly respond to shocks in market turnover. These results are consistent with the results obtained from the VAR models presented in .
4.2. Investor overconfidence in individual ETFs
The results presented in Section 4.1 indicate that investor overconfidence is present in both the market of South African ETFs with domestic benchmarks and in the market of South African ETFs with international benchmarks. However, to ensure that the market-wide investor overconfidence reported in Section 4.1 is not a direct summation of the aggregate disposition effect, panel VAR models are estimated to examine the relationship between the historical market return and the current trading activity of individual ETFs in the respective market after accounting for the disposition effect. presents the results of the panel VAR model estimated for the market of South African ETFs with domestic benchmarks whilst provides the results of the panel VAR model estimated for the market of South African ETFs with international benchmarks. Notably, in , the dependent variables (,
, and
) appear in rows whilst the coefficients for the lagged dependent and exogenous variables are organised in columns.
Table 3. Panel VAR model estimates for ETFs with domestic benchmarks
Table 4. Panel VAR model estimates for ETFs with international benchmarks
shows that, after controlling for market volatility and the disposition effect, the current turnover of individual ETFs does not exhibit any significant relationship with the lagged return of the market and the lagged return of individual ETFs in the market. Therefore, the findings of the panel VAR model in indicate that the turnover of individual ETFs in the market of ETFs with domestic benchmarks is not influenced by the overconfidence bias and the disposition effect. However, impulse response functions need to be analysed in order to provide a more reliable result.
shows that, after controlling for market volatility and the disposition effect, the turnover of individual ETFs in the market of ETFs with international benchmarks is significantly related to both market return and the return of individual ETFs in the market. Specifically, the turnover of individual ETFs is negatively and significantly related to the third lag of individual ETF return. However, this negative relationship is not consistent with the disposition effect. Moreover, the results in show that the turnover of individual ETFs in the market is positively related to the third lagged market return, and this relationship is significant at a 1% level of significance. This positive and significant relationship between historical market return and the current turnover of individual ETFs in the market indicate that the overconfidence bias influences the turnover of individual ETFs in the market of South African ETFs with international benchmarks. The influence of market return and individual ETF return on individual ETF turnover is further analysed in the context of impulse response functions.
For completion, also show that the turnover of individual ETFs in both markets is significantly autocorrelated because the current individual ETF turnover displays significant relationships with its historical turnover. Additionally, shows that the turnover of individual ETFs in the market of ETFs with international benchmarks is significantly influenced by both contemporaneous and historical volatility of individual ETFs. On the contrary, shows that the turnover of individual ETFs in the market of ETFs with domestic benchmarks is not significantly influenced by the current and historical volatility of individual ETFs. Notably, and show that the individual ETF return and market return in both markets are inefficient because these returns can be significantly explained by either historical individual ETF turnover, historical ETF return, historical market return, or individual ETF return volatility.
The impulse response functions associated with the panel VAR models presented in are illustrated in , respectively. For simplicity, illustrate the response of individual ETF turnover to its own shocks, shocks in individual ETF return, and shocks in market return in Panels A, B, and C, respectively.Footnote7
Figure 3. Impulse response functions for ETFs tracking domestic benchmarks

Figure 4. Impulse response functions for ETFs tracking international benchmarks

Consistent with the results of the panel VAR model in , Panel B in shows that the turnover of individual ETFs in the market of ETFs with domestic benchmarks does not significantly respond to shocks in individual ETF return. Consequently, the results of this study imply that the disposition effect does not influence the trading activities of South African ETFs with domestic benchmarks. On the contrary, this study finds that the disposition effect significantly influences the trading activities of South African ETFs with international benchmarks because the turnover of individual ETFs tracking international benchmarks significantly and positively respond to a one standard deviation shock in individual ETF return during periods 2, 3, 4, 5, and 8.
With regards to the overconfidence bias, Panel C in both show that, for both markets, a one standard deviation shock in the market return does not generate any response in the individual ETF turnover during period 1. From period 2 onwards, shocks in market return generate positive responses in individual ETF turnover on average. However, for the market of ETFs with domestic benchmarks, only the response in periods 5, 6, 8, 9, and 10 are statistically significant. For the market of ETFs with international benchmarks, only the response in period 4, 7, and 10 are statistically significant. Nevertheless, for both markets, the positive and statistically significant response of individual ETF turnover to shocks in market return imply that investor overconfidence influences the trading activities of individual ETFs in the respective markets even after controlling for the disposition effect.
According to Dewachter et al. (Citation2015), VAR models do not fully capture the influence of other variables in the system. Given this, impulse response functions are more reliable because they fully capture the influences of other variables in the system. Therefore, this study concludes that, based on the impulse response functions associated with panel VAR models, only the overconfidence bias influences the trading activities of individual ETFs in the market of ETFs with domestic benchmarks. However, the disposition effect and the overconfidence bias exist concurrently in the market of ETFs with international benchmarks, and the overconfidence bias explains a portion of the ETF trading activity that cannot be explained by the disposition effect. Nevertheless, the positive and statistically significant response of individual ETF turnover to past market returns even after controlling for the disposition effect, confirm that, for both markets, the market-wide investor overconfidence observed in Section 4.1 is not a direct summation of the aggregate disposition effect.
5. Conclusion
The arrival of ETFs has transformed the investment management landscape in recent years, and the popularity of these funds continue to soar despite the increased market volatility brought about by the COVID-19 pandemic. However, ETF investment choices may not always be rational, and irrational ETF investment decisions may pose a threat to the stability of ETF markets and financial markets as a whole. Therefore, the objective of this study is to investigate the presence of investor overconfidence in the South African ETF market. Using a sample of South African ETFs tracking domestic benchmarks and a sample of South African ETFs tracking international benchmarks, the findings of this study conclude that investor overconfidence is present in both markets. This is because, the VAR models estimated for both markets and their associated impulse response functions show that current market turnover is positively and significantly related to historical market return. This association holds for market-wide turnover and individual ETF turnover, and even after accounting for other possible explanations of trading activity.
These findings have significant implications for investors, investment management companies, and policymakers and regulators. Specifically, investors can avoid large losses by avoiding ETFs that are highly traded because of overconfident investors. Therefore, investors and investment management companies need to understand different anomalous market behaviour and what biases drive investment decisions so that they can reduce their risk exposures and increase their opportunities for realising higher returns. It is also important for regulators and policymakers to adopt policies that enhance the efficiency of the South African ETF market by mitigating the investor overconfidence present in South Africa’s ETF market. For instance, regulators should focus on the disclosure of unbiased and reliable information about ETFs and their constituents. Policymakers can also focus on improving the ETF creation and redemption process in order to limit manipulation of ETF prices.
Areas for future research include examining the presence of investor overconfidence in other South African financial markets (such as the stock and bond markets), testing the presence of other biases (such as anchoring and herding) in the South African ETF market, as well as investigating the presence of investor overconfidence in the South African ETF market under changing market conditions.
Disclosure statement
This work was developed from Damien Kunjal’s Master’s thesis which was supervised by Faeezah Peerbhai.
Additional information
Funding
Notes on contributors
Damien Kunjal
Damien Kunjal is a PhD candidate and part-time lecturer in the School of Accounting, Economics and Finance, University of KwaZulu-Natal, South Africa. He is also a full-time lecturer in the Department of Business Management, University of the Free State, South Africa. His research interests include behavioural finance, investment analysis, financial risk management, and portfolio optimization.
Faeezah Peerbhai
Faeezah Peerbhai (Ph.D.) is a Finance lecturer in the School of Accounting, Economics and Finance, University of KwaZulu-Natal, South Africa. Her research interests include asset pricing, market microstructure, behavioural finance, and exchange-traded funds.
Notes
1. Overconfidence refers to the tendency of investors to overestimate their knowledge, abilities, and security valuation skills (Odean, Citation1998).
2. According to Shefrin and Statman (Citation1985), the disposition effect refers to the tendency of traders to sell winning positions and to hold onto losing positions. As such, the first difference between the disposition effect and the overconfidence bias is that the disposition effect only provides an explanation for the sell-side of a trade whereas the overconfidence bias explains both the sell-side and the buy-side of a trade (Chou & Wang, Citation2011). The second difference between the two biases is that the disposition effect is associated with an investor’s attitude towards a particular security or the securities in their portfolio whereas the overconfidence bias is associated with an investor’s attitude towards the market in general rather than a specific security or securities (Statman et al., Citation2006).
3. The ETFs included in the respective market value-weighted portfolios are continuously rebalanced to account for the listing or delisting of any new ETFs in the respective market.
4. Following French et al. (Citation1987), is computed as follows:
where
is day
’s market return and
is the number of trading days in month
5. Results available on request.
6. Results available on request.
7. Unreported impulse response functions for the response of the individual ETF return and market return are available on request.
References
- Abbes, M. B. (2013). Does overconfidence bias explain volatility during the global financial crisis? Transition Studies Review, 19(3), 291–18. https://doi.org/10.1007/s11300-012-0234-6
- Aharon, D. Y., & Qadan, M. (2018). What drives the demand for information in the commodity market? Resources Policy, 59, 532–543. https://doi.org/10.1016/j.resourpol.2018.09.013
- Aldridge, I. (2016). ETFs, high-frequency trading, and flash crashes. Journal of Portfolio Management, 43(1), 17–28. https://doi.org/10.3905/jpm.2016.43.1.017
- Alicke, M. D. (1985). Global self-evaluation as determined by the desirability and controllability of trait adjectives. Journal of Personality and Social Psychology, 49(6), 1621–1630. https://doi.org/10.1037/0022-3514.49.6.1621
- Alpert, M., & Raiffa, H. (1982). Judgement under uncertainty: Heuristics and biases. Cambridge University Press.
- Alsabban, S., & Alarfaj, O. (2020). An empirical analysis of behavioral finance in the Saudi stock market: Evidence of overconfidence behavior. International Journal of Economics and Financial Issues, 10(1), 73–86. https://doi.org/10.32479/ijefi.8920
- Bahadar, S., Mahmood, H., & Zaman, R. (2019). The herds of bulls and bears in leveraged ETF market. Journal of Behavioral Finance, 20(4), 408–423. https://doi.org/10.1080/15427560.2019.1553177
- Bailey, W., Kumar, A., & Ng, D. (2011). Behavioral biases of mutual fund investors. Journal of Financial Economics, 102(1), 1–27. https://doi.org/10.1016/j.jfineco.2011.05.002
- Baker, H. K., Kumar, S., Goyal, N., & Gaur, V. (2019). How financial literacy and demographic variables relate to behavioral biases. Managerial Finance, 45(1), 124–146. https://doi.org/10.1108/MF-01-2018-0003
- Barber, B. M., & Odean, T. (1999). The courage of misguided convictions. Financial Analysts Journal, 55(6), 41–55. https://doi.org/10.2469/faj.v55.n6.2313
- Barber, B. M., & Odean, T. (2001). Boys will be boys: Gender, overconfidence, and common stock investment. The Quarterly Journal of Economics, 116(1), 261–292. https://doi.org/10.1162/003355301556400
- Ben‐David, I., Franzoni, F., & Moussawi, R. (2018). Do ETFs increase volatility? The Journal of Finance, 73(6), 2471–2535. https://doi.org/10.1111/jofi.12727
- Broman, M. S. (2016). Liquidity, style investing and excess comovement of exchange-traded fund returns. Journal of Financial Markets, 30, 27–53. https://doi.org/10.1016/j.finmar.2016.05.002
- Buehler, R., Griffin, D., & Ross, M. (1994). Exploring the planning fallacy: Why people underestimate their task completion times. Journal of Personality and Social Psychology, 67(3), 366–381. https://doi.org/10.1037/0022-3514.67.3.366
- Chen, G., Kim, K. A., Nofsinger, J. R., & Rui, O. M. (2007). Trading performance, disposition effect, overconfidence, representativeness bias, and experience of emerging market investors. Journal of Behavioral Decision Making, 20(4), 425–451. https://doi.org/10.1002/bdm.561
- Chen, H. S., & Sabherwal, S. (2019). Overconfidence among option traders. Review of Financial Economics, 37(1), 61–91. https://doi.org/10.1002/rfe.1048
- Chou, R. K., & Wang, Y. Y. (2011). A test of the different implications of the overconfidence and disposition hypotheses. Journal of Banking and Finance, 35(8), 2037–2046. https://doi.org/10.1016/j.jbankfin.2011.01.018
- Chuang, W. I., & Lee, B. S. (2006). An empirical evaluation of the overconfidence hypothesis. Journal of Banking and Finance, 30(9), 2489–2515. https://doi.org/10.1016/j.jbankfin.2005.08.007
- Chuang, W. I., & Susmel, R. (2011). Who is the more overconfident trader? Individual vs. institutional investors. Journal of Banking and Finance, 35(7), 1626–1644. https://doi.org/10.1016/j.jbankfin.2010.11.013
- Da Dalt, C., Feldman, D., Garvey, G., & Westerholm, P. J. (2019). Contrarians or momentum chasers? Individual investors’ behavior when trading exchange‐traded funds. Journal of Futures Markets, 39(5), 553–578. https://doi.org/10.1002/fut.21979
- Da, Z., & Shive, S. (2018). Exchange traded funds and asset return correlations. European Financial Management, 24(1), 136–168. https://doi.org/10.1111/eufm.12137
- Daniel, K., Hirshleifer, D., & Subrahmanyam, A. (1998). Investor psychology and security market under‐and overreactions. Journal of Finance, 53(6), 1839–1885. https://doi.org/10.1111/0022-1082.00077
- Deev, O., & Linnertová, D. (2014). The determinants of ETFs short selling activity. Procedia - Social and Behavioral Sciences, 109, 669–673. https://doi.org/10.1016/j.sbspro.2013.12.526
- Dewachter, H., Iania, L., Lyrio, M., & De Sola, P. M. (2015). A macro-financial analysis of the euro area sovereign bond market. Journal of Banking and Finance, 50, 308–325. https://doi.org/10.1016/j.jbankfin.2014.03.011
- Dowie, G., & Willows, G. (2016). An investigation of investors’ estimates of returns earned and the effect of anchoring on these estimations. South African Journal of Accounting Research, 30(1), 29–40. https://doi.org/10.1080/10291954.2015.1021559
- French, K. R., Schwert, G. W., & Stambaugh, R. F. (1987). Expected stock returns and volatility. Journal of Financial Economics, 19(1), 3–29. https://doi.org/10.1016/0304-405X(87)90026-2
- Gervais, S., & Odean, T. (2001). Learning to be overconfident. The Review of Financial Studies, 14(1), 1–27. https://doi.org/10.1093/rfs/14.1.1
- Griffin, J. M., Nardari, F., & Stulz, R. M. (2007). Do investors trade more when stocks have performed well? Evidence from 46 countries. The Review of Financial Studies, 20(3), 3. https://doi.org/10.1093/rfs/hhl019
- Gupta, S., Goyal, V., Kalakbandi, V. K., & Basu, S. (2018). Overconfidence, trading volume and liquidity effect in Asia’s giants: Evidence from pre-, during-and post-global recession. Decision, 45(3), 235–257. https://doi.org/10.1007/s40622-018-0185-9
- Jlassi, M., Naoui, K., & Mansour, W. (2014). Overconfidence behavior and dynamic market volatility: Evidence from international data. Procedia Economics and Finance, 13, 128–142. https://doi.org/10.1016/S2212-5671(14)00435-3
- Johnson, W. F. (2009). Tracking errors of exchange traded funds. Journal of Asset Management, 10(4), 253–262. https://doi.org/10.1057/jam.2009.10
- Kansal, P., & Singh, S. (2018). Determinants of overconfidence bias in Indian stock market. Qualitative Research in Financial Markets, 10(8), 381–394. https://doi.org/10.1108/QRFM-03-2017-0015
- Kapoor, S., & Prosad, J. M. (2017). Behavioural finance: A review. Procedia Computer Science, 122, 50–54. https://doi.org/10.1016/j.procs.2017.11.340
- Kyrychenko, V., & Shum, P. (2009). Who holds foreign stocks and bonds? Characteristics of active investors in foreign securities. Financial Services Review, 18(1), 1–21. http://citeseerx.ist.psu.edu/viewdoc/download?doi=10.1.1.336.4303&rep=rep1&type=pdf
- Langer, E. (1975). The illusion of control. Journal of Personality and Social Psychology, 32(2), 311–328. https://doi.org/10.1037/0022-3514.32.2.311
- Lin, C. Y., Rahman, H., & Yung, K. (2010). Investor overconfidence in REIT stock trading. Journal of Real Estate Portfolio Management, 16(1), 39–57. https://doi.org/10.1080/10835547.2010.12089866
- Liu, S. (2015). Investor sentiment and stock market liquidity. Journal of Behavioral Finance, 16(1), 51–67. https://doi.org/10.1080/15427560.2015.1000334
- Ma, J. Z., Ho, K. C., Yang, L., & Chu, C. C. (2018). Market sentiment and investor overreaction: Evidence from New York listed Asian country exchange traded funds. Emerging Markets Finance and Trade, 54(11), 2455–2471. https://doi.org/10.1080/1540496X.2018.1464907
- Madura, J., & Richie, N. (2004). Overreaction of exchange-traded funds during the bubble of 1998-2002. The Journal of Behavioral Finance, 5(2), 91–104. https://doi.org/10.1207/s15427579jpfm0502_3
- Meier, C., & de Mello, L. (2020). Investor overconfidence in experimental asset markets across market states. Journal of Behavioral Finance, 21(4), 369–384. https://doi.org/10.1080/15427560.2019.1692845
- Metwally, A. H., & Darwish, O. (2015). Evidence of the overconfidence bias in the Egyptian stock market in different market states. The Business and Management Review, 6(4), 178–198. https://www.ijbed.org/cdn/article_file/i-9_c-93.pdf
- Mohamad, A., Jaafar, A., & Goddard, J. (2016). Short selling and exchange-traded funds returns: Evidence from the London stock exchange. Applied Economics, 48(2), 152–164. https://doi.org/10.1080/00036846.2015.1076146
- Moore, D. A., & Healy, P. (2008). The trouble with overconfidence. Psychological Review, 115(2), 502–517. https://doi.org/10.1037/0033-295X.115.2.502
- Moore, D. A., & Schatz, D. (2017). The three faces of overconfidence. Social and Personality Psychology Compass, 11(8), e12331.
- Odean, T. (1998). Volume, volatility, price, and profit when all traders are above average. The Journal of Finance, 53(6), 1887–1934. https://doi.org/10.1111/0022-1082.00078
- Odean, T. (1999). Do investors trade too much? American Economic Review, 89(5), 1279–1298. https://doi.org/10.1257/aer.89.5.1279
- Padungsaksawasdi, C., & Daigler, R. T. (2014). The return‐implied volatility relation for commodity ETFs. Journal of Futures Markets, 34(3), 261–281. https://doi.org/10.1002/fut.21592
- Petajisto, A. (2017). Inefficiencies in the pricing of exchange-traded funds. Financial Analysts Journal, 73(1), 24–54. https://doi.org/10.2469/faj.v73.n1.7
- Pikulina, E., Renneboog, L., & Tobler, P. N. (2017). Overconfidence and investment: An experimental approach. Journal of Corporate Finance, 43, 175–192. https://doi.org/10.1016/j.jcorpfin.2017.01.002
- Redl, C. (2018). Macroeconomic uncertainty in South Africa. South African Journal of Economics, 86(3), 361–380. https://doi.org/10.1111/saje.12198
- Salahuddin, M., Vink, N., Ralph, N., & Gow, J. (2020). Globalisation, poverty and corruption: Retarding progress in South Africa. Development Southern Africa, 37(4), 617–643. https://doi.org/10.1080/0376835X.2019.1678460
- Scheinkman, J. A., & Xiong, W. (2003). Overconfidence and speculative bubbles. Journal of Political Economy, 111(6), 1183–1220. https://doi.org/10.1086/378531
- Shefrin, H., & Statman, M. (1985). The disposition to sell winners too early and ride losers too long: Theory and evidence. Journal of Finance, 40(3), 777–790. https://doi.org/10.1111/j.1540-6261.1985.tb05002.x
- Sheikh, M. F., & Riaz, K. (2012). Overconfidence bias, trading volume and returns volatility: Evidence from Pakistan. World Applied Science Journal, 18(12), 1737–1748.
- Statman, M., Thorley, S., & Vorkink, K. (2006). Investor overconfidence and trading volume. The Review of Financial Studies, 19(4), 1531–1565. https://doi.org/10.1093/rfs/hhj032
- Steyn, J. (2019). The tracking efficiency of exchange-traded funds listed on the Johannesburg stock exchange. Journal of the Southern African Institute for Management Scientists, 28(4), 2–14. https://hdl.handle.net/10520/EJC-19993c0a22
- Strydom, B., Charteris, A., & McCullough, K. (2015). The relative tracking ability of South African exchange traded funds and index funds. Investment Analysts Journal, 44(2), 117–133. https://doi.org/10.1080/10293523.2014.994454
- Swanson, N. R., & Granger, C. W. (1997). Impulse response functions based on a causal approach to residual orthogonalization in vector autoregressions. Journal of the American Statistical Association, 92(437), 357–367. https://doi.org/10.1080/01621459.1997.10473634
- Trejos, C., Van Deemen, A., Rodríguez, Y. E., & Gómez, J. M. (2019). Overconfidence and disposition effect in the stock market: A micro world based setting. Journal of Behavioral and Experimental Finance, 21, 61–69. https://doi.org/10.1016/j.jbef.2018.11.001
- Trinugroho, I., & Sembel, R. (2011). Overconfidence and excessive trading behavior: An experimental study. International Journal of Business and Management, 6(7), 147–152. https://doi.org/10.5539/ijbm.v6n7p147
- Venkataraman, R., & Venkatesan, T. (2016). Evaluation of growth of mutual funds and exchange traded funds in India. SDMIMD Journal of Management, 7(1), 41–47. https://doi.org/10.15533/sdm/2016/v7i1/90221
- Weinstein, N. D. (1980). Unrealistic optimism about future life events. Journal of Personality and Social Psychology, 39(5), 806–820. https://doi.org/10.1037/0022-3514.39.5.806
- Yeh, C. H., & Yang, C. Y. (2014). How does overconfidence affect asset pricing, volatility, and volume? In Advances in computational social science (pp. 107–122). Springer.
- Yung, K., & Liu, Y. C. (2009). Implications of futures trading volume: Hedgers versus speculators. Journal of Asset Management, 10(5), 318–337. https://doi.org/10.1057/jam.2009.31
- Zaiane, S., & Abaoub, E. (2009). Investor overconfidence and trading volume: The case of an emergent market. International Review of Business Research Papers, 5(2), 213–222.
- Zia, L., Sindhu, L. M., & Hashmi, H. S. (2017). Testing overconfidence bias in Pakistani stock market. Cogent Economics and Finance, 5(1), 1. https://doi.org/10.1080/23322039.2017.1289656