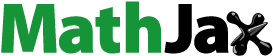
Abstract
This study explores the redundancy of the value premium by conducting a Fourier analysis. The results illustrate periodicity in the value premium and merges the Adaptive Market Hypothesis with the Efficient Market hypothesis. The value premium is considered to be redundant due to structural economic changes, persistently low global interest rates and value investing’s underperformance relative to growth investment strategies. The Adaptive Market Hypothesis suggests that market efficiencies vary over time as risk and behavioral biases change with market conditions. We conducted a Fourier analysis and found a three-month cycle, a six-month cycle and a 10-year cycle in the value premium. The Fourier analysis illustrates the predictability of the value premium and the study explains the short-term cyclicality as behavioral biases. Furthermore, the longer cycles are better explained by rational asset pricing, perceived market risks and market efficiency. Historic value factor returns were sourced from value portfolios that were constructed by their rankings associated with their book to market ratios. Additionally, a combination portfolio of value and momentum was formed including returns from portfolios ranked on past price performances to value portfolios. The combination portfolio held an equal weighting in value and momentum.
PUBLIC INTEREST STATEMENT
Value investing is one of the world’s first structured investment strategies. A radical shift in market expectations, market participants, business models and global monetary policy over the last ten years has caused market participants to speculate whether value investing is redundant due to the strategy’s wide public exposure. The Efficient Market Hypothesis explains that the strategy may not provide fund managers with risk adjusted returns due to its public exposure, but the Adaptive Market Hypothesis explains that market efficiency changes as market participants adapt to new biases and risks associated with the change in the market environment. This work uses spectral analysis to test the predictability and cyclicality of the value premium to determine whether the investing strategy is redundant. The results of the study harmonise the Efficient Market Hypothesis and the Adaptive Market Hypothesis with robust mathematical backing.
1. Introduction
Value investing is one of the world’s first structured investment strategies and has been pivotal to the investment universe since it was introduced to the general public by Graham (Citation1973). The value premium is the product of cheaper securities outperforming more expensive securities on average (Asness et al., Citation2015) and is achieved by going long on cheap securities and shorting expensive securities. The value premium has been sought after by investors for centuries as the strategy produced risk adjusted returns (Zhang, Citation2005). It is important to recognise that there are nuanced differences between “pure value” and value investing that is explained by Graham (Citation1973). Graham (Citation1973) suggests that abnormal profits are achieved by buying profitable, but undervalued companies. Pure value strategies are defined by relatively lower prices of securities compared to accounting fundamentals such as book value or earnings (Graham, Citation1973). Therefore, the practice of combining investment strategies with value investing plays an important role in defining the history of value investing and determining its future.
The main objective of this study is to determine whether the value premium is redundant in US equity markets by testing cyclicality through the execution of Fourier analysis. Another important factor that will be studied is the periodicity of the value premium and how it has adapted intertemporally. The results of this study will speak to how behavioral biases and risk factors affect the value premium over time. Furthermore, there is limited documented academic research investigating the use of Fourier analysis for this purpose. Therefore, the study may aid money managers and investors with a robust market timing tool and a more nuanced understanding around the cyclicality or non-cyclicality of the value premium.
The value premium was 1.17% per year on average in the US and it was not statistically different from zero between 1991 and 2019. Fama and French (Citation2020) suggested that we cannot confidently conclude that the US value premium has declined or disappeared, meaning that all out-of-sample value premiums are equal to in-sample expected premiums. However, for the three years ending December 2019, we see value stocks trailing the market by 6.88% per year on average. For the decade ending 2019, value stocks trailed the market by 2.24% per year on average (Bloomberg, Citation2020a). Fama and French (Citation2018) used a bootstrapping method to simulate 100,000 time periods of varying length to estimate the probability of the value premium being positive over a given period. They found that over a three-year period there was a 23% chance of a negative value premium. Additionally, for a ten-year period it was found that there is a nine percent chance of a negative value premium (Fama & French, Citation2018).
illustrates the downward trend in the US value premium from January 2000 to 2019. This downturn has caused most money managers to speculate whether the value premium will make a return. The possible reasoning behind the lackluster performance of the value premium is that the returns represent a pure risk premium and investors are rewarded for these risks. The risk premium may be eroded on the back of improved asset pricing models and investor pricing abilities. The Fama and French (Citation2015) Five Factor Model illustrated an improvement on the Three Factor Model (Fama & French, Citation1993) that illustrates the power of effective asset pricing modelling. Secondly, a behavioral finance explanation describes an over and under reaction in new information leading to irrationality. This suggests that value stocks are cheap due to human error and will revert to the mean price (Shiller, Citation2017).
Figure 1. Downward trend of the HML value premium that was tested against the Fama and French (Citation1993) Three Factor Model.Source: Bloomberg (Citation2020c) and author calculations.

2006 is the tipping point for value investing as the trend depreciated significantly from that point onward as growth outperformed value (Shiller, Citation2017). illustrates value investing’s significant underperformance since 2006 on an annual basis.
Figure 2. Historic performance of the value investing strategy against the performance of growth stocks. The relative performance is calculated by dividing the MSCI World Value Net Total Return USD Index by the MSCI World Growth Net Total return USD Index (Bloomberg, Citation2020a).Source: Bloomberg (Citation2020c) and author calculations

The a priori assumption of this analysis is that the value premium is explained by a risk premium and irrationality, and that the value premium is cyclical due to its strong correlation to fluctuations in Gross Domestic Product (GDP) figures (Black & Fraser, Citation2003). As a result, the Fourier analysis will connect the Efficient Market Hypothesis (Fama, Citation1970) with the Adaptive Market Hypothesis (Lo, Citation2004). This will be tested by reviewing the Efficient Market Hypothesis (EMH) (Fama, Citation1970) and how the Fama and French (Citation2015) Five Factor asset pricing model has rendered the value premium as a dormant factor. In addition, a review of the AMH will be conducted as this study investigates the risk and behavioural finance elements that link the EMH (Fama, Citation1970) and AMH (Lo, Citation2004) with a specific focus on the value premium. Finally, the literature considering the value premium, as a sound investment tool, will be reviewed by introducing the nature of the value factor and how it reacts to momentum elements. Spectral analysis has been used to determine the trend movements of economic variables such as GDP. The capacity of Spectral Analysis to determine the cyclicality of the value factor is also explored. The remainder of this article proceeds as follows; section 2 presents a comprehensive literature survey analysing the EMH, AMH, rational asset pricing theories, behavioural finance and market timing theories. Section 3 details the methodology and data by describing the relevance of the Fourier analysis and the data used in the analysis. Furthermore, the study results and discussion points are illustrated in section 4, while section 5 provides the conclusion and suggestions for future work.
2. Literature survey
2.1. The efficient market hypothesis
A capital market is said to be efficient if it fully and correctly reflects all relevant information in determining security prices (Malkiel, Citation2003). The EMH (Fama, Citation1970) is tested through event study or portfolio study methods. This examines excess returns with respect to information events and market reactions. Portfolio studies entail building portfolios with idiosyncratic characteristics that will be compared to expected returns from a specific asset pricing model such as the three factor and five factor asset pricing models (Fama, Citation2014). If the investment strategy provides excess returns then it is considered to be an anomaly to the EMH (Fama, Citation1970).
Business cycles are products of GDP fluctuations. Thomson and van Vuuren (Citation2016) illustrated that Fourier analysis can successfully determine the phase and frequency of business cycles. It is intuitive that if there is regularity associated with business cycles then a stock market’s price index should also illustrate some form of regularity (Bodie, Kane, & Marcus, Citation2011). Listed securities are priced by looking at growth potential as defined by the EMH. When leading indicators predict an upturn in economic activity the stock market would have reacted already (Bodie et al., Citation2011). Auerbach (Citation1981) discovered that leading indicators are not reliable over time. This phenomenon is well in line with the EMH.
The weak form EMH and the semi-strong EMH are tested with different techniques (Fama, Citation1970). To prove the weak form EMH; stock returns are to be accurately predicted by changes in new or past public information. Shleifer (Citation2000) suggests that this can be done by event studies that determine whether significant risk adjusted returns are achievable after adjusting the returns for transaction fees. Event studies illustrate the tempo at which prices adjust to new information. Lo and MacKinley (Citation1999) suggest that if a market is efficient then it would be impossible for investors to achieve superior risk-adjusted returns that are adjusted for fees after a public announcement.
Random walk theory, introduced by Pearson (Citation1905) emerges as the result of investors reacting instantaneously to new information. Dupernex (Citation2007) among others, demonstrated that a more efficient market would have more random sequences in price movements across time. The semi-strong form of the EMH is tested by analysing the cross-sectional distribution of returns (Guerrien & Gun, Citation2011). The significant pitfalls to the testing techniques are related to analysts mistaking correlation for causation and data mining by studying a biased universe of data (Oseni & Nwosa, Citation2011). Also, the random walk and the EMH are different as the random walk theory suggests that price changes are independent of one an-other. Shleifer (Citation2000) suggests that the weak form of the EMH leads to the random walk hypothesis, but the semi-strong and strong forms do not.
In conclusion, the EMH (Fama, Citation1970) and the random walk hypothesis (Lo & MacKinley, Citation1999) suggest that the expected returns on a stock should be identical to the actual return and are difficult to predict due to their random elements. Thus, technical analysis and market timing cannot provide risk adjusted returns. Passive investment strategies are superior to any form of active management if the EMH is robust (Malkiel, Citation2003).
2.2. The adaptive market hypothesis
The Adaptive Market Hypothesis (AMH) explains that markets can both be efficient and inefficient due to market participants experiencing different market conditions over time (Lo, Citation2004). Exploring the predictability of the value premium illustrates how strategies perform as market conditions change.
The main critique of the AMH is that it is not robustly supported by mathematical models: most academic literature focusses on identifying adaptive behaviour in stock performance rather than analytically describing it. The portmanteau bicorrelation test (a diagnostic tool used to ascertain the adequacy of volatility models in describing stock market returns generating processes) has been applied in 11 emerging markets (Lim & Brooks, Citation2006) and two developed markets (Ito & Sugiyama, Citation2009) and they concluded that market efficiency is time dependent. Time-varying S&P500 returns have also been reported.
These studies illustrate that the degree of market efficiency is time dependent. Kim, Shamsuddin, and Lim (Citation2011) observed the time-varying predictability of DJIA data between 1900 and 2009 using an automatic variance ratio test and automatic portmanteau. Strong evidence for time varying predictability was found suggesting that economically distressful times provided high levels of return predictability while market crashes provided no return predictability.
Another rolling window variance ratio test explored 15 European emerging stock markets and three developed markets and found that return predictability varied considerably. The global financial crisis provided a highly predictable period for return outlooks in the UK, Slovakia, Poland, Portugal, Croatia and Hungary (Smith, Citation2012). Box Pierce tests, bootstrapped automatic variance ratio tests (through rolling estimation) and finite sample variance ratio tests were applied to three major US stock indices, two Bulgarian stock price indices and 13 South East European indices (Lim et al., Citation2013). Strong evidence of time varying return predictability was found. Urquhart and McGroarty (Citation2014) used a rolling window analysis and identified over four calendar anomalies indicating that market efficiency varies somewhat predictably over different market conditions. Ito et al. (Citation2016) used a non-Bayesian time-varying model and found that the US stock market has fluctuations of market efficiency with periodicity between 30 and 40 years.
2.3. Rational asset pricing
It is paramount to introduce the synergy between the EMH and the Five-Factor asset pricing model (Fama, 2015). According to Fama (Citation2014); the EMH and an asset pricing model is to be used in tandem, as the credibility of the asset pricing model can only be supported by testing it against the EMH. Fama (Citation2014) explains that market efficiency suggests that returns on factor-based portfolios are proxies for risk. Therefore, an abnormal return is the result of abnormal risks associated with the securities in a specific portfolio. This ultimately suggests that real economic profits cannot be achieved when trading based on a new information set. Shleifer (Citation2000) illustrated that there is no causal relationship between outdated released information and future prices of listed securities. This is in-line with the weak form of the EMH that states all prices fully reflect all historical events such as trading data, rates of return and price movements (Fama, Citation1970).
The semi-strong form of the EMH (Fama, Citation1970) also suggests that all market related and non-market related public information is fully reflected in share prices. Thus, it is intuitive that investment decisions made on new information after its publication will not result in risk-adjusted returns. Fama (Citation1970) continued to define the strong-form of the EMH as an assumption that markets are perfect as all information is widely available to everybody at the same time and therefore prices reflect all public and private information.
The initial asset pricing model that represented the market equilibrium was the Capital Asset Pricing Model (Sharpe, Citation1964). This model only captures market risk in the form of beta, meaning that it suggests that expected asset returns are only dependant on the market risks associated with the portfolio. However, Fama (Citation2014) suggests that the model can be improved by the Three Factor Model and the Five Factor model as they have additional risk factors that describe expected return from specific risk proxies. The Three-Factor Asset Pricing Model—developed by Fama and French (Citation1993)—is
The model is tested by the time-series regression:
Where
The HML factor is the most fitting proxy for a pure value effect. It illustrates the difference between the returns from highly ranked stocks and low ranked stocks in terms of their respective B/M values (Fama & French, Citation1993). The relevance of the value factor was justified by its ability to explain the returns with regard to the associated risk when tested against the EMH (Fama & French, Citation2015). The model is considered an empirical asset pricing model as it captures all the sensitivities to portfolio returns. However, with the introduction of profitability (RMW) and an investment (CMA) factor, the value factor has petered out and has brought forward the notion that the value premium is redundant.
The Five-Factor model is tested by the time series Regression 3.
where
Fama and French (Citation2015) explain that the value factor (HML) was redundant after conducting the Five-Factor model test. The value factor provides no explanatory value when considering average returns. The value factor loses its potency when profitability and investment factors are added to the model. Over the period between 1963 and 2019 they recorded a reduction in the value premium from 0.36% to 0.05%. This conclusion is in line with the EMH as investors trade away the value premium as the information becomes more available to the public (Fama & French, Citation2020).
It is evident that money managers and investors still target a large value premium in average returns. Fama and French (Citation2010) suggest this is because there is a large value premium present in average returns when looking at the three-factor model. The results illustrated that in terms of the HML regressions, the explanatory value of the HML factor was absorbed by RMW and CMA. When controlling for the investment factor; value stocks behave like stocks that are ranked highly in terms of profitability even though value stocks tend to be less profitable. This conclusion is flawed as the model only illustrates that value is no longer needed to explain the returns of portfolios and that the combination of factors may provide better explanatory value. The value premium can thus be explained by other variables (Asness et al., Citation2015).
An important factor to consider is that Fama and French (Citation2015) used lagged prices to form the HML factor and most importantly, they disregarded momentum in their analysis. Finally, Fama and French (Citation2020) conducted tests to determine whether the value premium declined after it was established as a market beating premium and investment strategy. The value premium was tested between 1963 and 2019. It was confirmed that the value premium dropped from 0.42% during the period between 1963 and 1991 to 0.11% per month between 1991 and 2019. The value premium has produced high levels of volatility and prevented Fama and French (Citation2020) from rejecting the null hypothesis that the expected value premiums are the same over the period of 1963 to 1991 and 1991 to 2019.
Momentum is defined as an investment strategy that allows securities that have performed well, compared to peers on average, to continue outperforming their peers (Fama & French, Citation2012). Securities that have relatively underperformed against their peers; would continue to underperform. Jegadeesh and Titman (Citation1993) published the first significant study on the momentum effect. They studied US stocks and found that the stocks with the best performances over a 36-month period outperformed the worst performers over the preceding year. Momentum strategies between six and 12 months illustrated that the momentum effect was present. Further studies tested the momentum effect across foreign equities, currencies, commodities and fixed income securities. Fama and French (Citation2012) illustrated that the momentum effect was present in every stock market they studied except for the Japanese stock market. They went on to show that stocks with significant price momentum characteristics consistently outperformed those with the lowest levels of stock price momentum. Another interesting point is that stocks with smaller market capitalisations tended to have a more robust momentum effect, but had higher trading costs associated to them (Fama & French, Citation2012).
The value effect and the momentum effect are widely studied in the efficient market debate. Asness et al. (Citation2013) introduced a new facet to the body of knowledge by examining the joint returns of the momentum effect and the value effect. Significant return premiums were found across eight different asset classes and markets. The evidence also illustrated co-movement between momentum and value strategies across diverse asset classes. That means that momentum strategies are positively correlated across the respective asset classes and value strategies are positively correlated across different unrelated asset classes. When comparing momentum and value; it was evident that the two factors are negatively correlated and may provide diversification effects. Asness et al. (Citation2013) used the Three-Factor model and did not account for transaction fees. It is evident that value and momentum as a joint factor outperforms the two factors as individual features. If markets were truly efficient then the combination of value and momentum should not have resulted in actual returns that exceed expected returns. Asness et al. (Citation2013) did not consider transaction fees in their analysis. Another important factor is that no empirical literature suggests that market timing techniques beat all three version of the EMH (Shleifer, Citation2000).
2.4. Behavioural finance
Behavioural finance is defined as the study of how investors react when they are exposed to new information regarding their investments (Barberis & Thaler, Citation2003). Behavioural finance has been widely used to explain anomalies that were derived from market inefficiencies or inadequacies in asset-pricing theory. Investor biases may be the reason why the value premium seems redundant. The main behavioural finance biases associated with value investing’s redundancy are overconfidence, the gambler’s fallacy, conservatism and Myopic Loss Aversion (Tilson, Citation2005).
Overconfidence is the result of investors overestimating their stock pricing abilities and is the main driving force behind investors trying to time markets (Singh, Citation2012). The gambler’s fallacy is derived from overconfidence in the future direction of a trend in market pricing. Due to market efficiency; anomalies tend to be traded away as they are made available to the public domain. This philosophy can be related to price multiples that have historically benefited from the value premium (Bouman & Jacobsen, Citation2002). Thaler (Citation1987) proved that this has been the case with the January Effect. Stock prices reflect the risks and information associated with the anomalies robustly. Furthermore, Fama (Citation1998) described that share prices experience a lag before fully reflecting information in prices due to over and under reactions to new information exacerbated by the band wagon effect. Barberis and Thaler (Citation2003) explain that this falls in the availability bias category as the investment decision is based on the latest information.
Myopic Loss Aversion entails a reluctance to sell investments after they have fallen in value (Fama, Citation1965). The concept of noise traders was explained by Friedman (Citation1953) as investment decisions that are dependent on psychological factors as opposed to robust investment theory and management. According to Hirshleifer (Citation2001); irrational trading strategies cannot be sustained in the long run and may result in a cyclical phenomenon. Irrational trades are random in nature and are uncorrelated; this suggests that asset prices are left unchanged after trading. These arguments support the claim that the market may be rational, even though individual investors may not.
When investors reflect loss aversion tendencies they hold on to negative yielding investments for longer and ultimately end up taking up more risk (Singh, Citation2012). Additionally, investors tend to regret certain steps in the decision-making process when they fail to deliver expected returns, Quiggin (Citation1994) labelled this as regret aversion. Investors may attempt to manage situations to avoid regret. Conservatism results in investors failing to update their beliefs as they ultimately under-react to new information (Hirshleifer, Citation2001). Another important factor to consider is that when investors fail to process information correctly, future returns and probability distributions may be incorrect.
In conclusion, behavioural finance introduces the idea of overreactions and under reactions experienced in stock prices as investors process information incorrectly due to a plethora of biases that result in suboptimal decisions. This makes it possible for investors to use spectral analysis tools to time the market for seasonal anomalies.
2.5. Market timing
The value premium illustrates that expensive securities underperform cheaper securities (Asness et al., Citation2015). The existence of these patterns in financial markets leads to confirmation biases and may motivate investors to try and time the market (Asness et al., Citation2017). Welch and Goyal (Citation2008) suggest that market timing tools lack robustness as methodologies are riddled with data-mining and selection biases. Earlier market timing studies focused on statistical forecasting power as opposed to outperformance measures against a benchmark. However, a small amount of predictive power may unlock meaningful economic gains for investors (Campbell & Thompson, Citation2008). The correlation between subsequent returns and valuation is stronger in the longer term. That means market timing is easier over a five to 10-year period as opposed to a quarterly exercise (Asness et al., Citation2017).
Asness et al. (Citation2017) tests market timing robustness by ranking securities by their CAPE ratios. They used data from 1900 to 2015 to illustrate that lower valuations consistently predict higher returns. However, their analysis has built in biases as they constructed the quintiles with full hindsight history present. Real time investors do not know how cheap securities are relative to the future market. Asness et al. (Citation2017) adjusted their methodology by comparing their CAPE ranked market timing portfolios with a simple buy and hold portfolio. They evaluated risk adjusted returns and their results suggested that a value-based timing strategy can-not beat the Sharpe Ratio (Sharpe, Citation1994) of a buy and hold portfolio. This is because the market timing strategy was underinvested since 1958 as the average CAPE ratio drifted higher on average over the time period observed.
However, value and momentum as an investment style has illustrated success in out-of-sample tests. Asness et al. (Citation2017) uses the mean of value and momentum signals to time the market. The strategy achieved a relatively higher Sharpe Ratio than a pure value timing strategy. In addition, the strategy outperformed the simple buy and hold portfolio as well. The evidence suggests that momentum should be added as a signal when conducting contrarian market timing strategies. The next section reviews spectral analysis as a tool to identify trends in the value premium.
2.6. Spectral analysis: fourier analysis
According to Hayes (Citation2009), frequency domain analysis is a statistical methodology that determines the strength of a particular frequency within a time series. Therefore, the output from spectral analysis will be illustrated as peaks and the magnitude of the peaks indicate the amplitudes of the frequencies. Furthermore, transforms are the tools used to move data from the time domain into the frequency domain. Chaudhuri and Lo (Citation2016) suggest that there is a rebirth in the use of spectral analysis in finance and economics. However, it is evident that current applications of spectral methods are relatively rare (Chaudhuri & Lo, Citation2016). The tool with the most potential is the Fourier series. The Fourier transform allows data to be transformed from a function of time to a function of frequency. The procedure produces a linear combination of trigonometric functions and is dependent on the Discrete-Time Fourier transform (Chaudhuri & Lo, Citation2016).
Stationarity suggests that time series data hold a joint probability distribution that does not change with time. Benhmad (Citation2013) suggested that the assumption of stationarity is the reason why spectral analysis has not been successful when used with share data. It is evident that share data are non-stationary as they are impacted by business cycles and other macro-economic variables. In addition, after using wavelet transforms to test co-movements between international stock markets; Benhmad (Citation2013) concluded that the Fourier Analysis is not useful for the analysis of periodic and stationary signals. An interesting concept was introduced by Gabor (Citation1946) wherein which the Short Time Fourier Transform was used to analyse a fixed window when the actual non-stationary signal is assumed to be stationary. Fourier analysis is conducted for each window and the magnitudes and frequencies for every window are added to a matrix. The model presented timing issues as a short window may enhance time sensitive information at the expense of frequency information. A longer window would do the opposite (Benhmad, Citation2013).
The trend element in the momentum factor may result in improved predictive capacity. This will allow money managers to enhance market timing techniques and may resurrect the value premium as Asness et al. (Citation2014) illustrates that momentum and value as a combination factor enhances the value premium. If the Fourier analysis proves that the value factor is cyclical then it may be the product of a behavioural inefficiency and this suggests that the value premium will adapt to over and under reactions. If the value premium is cyclical then its cheapness is a product of investor irrationality. The rational asset pricing theories suggest that the value premium is a product of a rational risk premium as returns are off-set by respective risks. Spectral analysis will play a pivotal role in determining the cyclicality of the value premium. The following section outlines the data points and the methodology used to achieve the outlined research objectives.
3. Data and methodology
3.1. Fourier analysis
Following on from Spectral Analysis; an important tool used to transform data into the frequency domain is known as a Fourier series. The Fourier series is the sum of a number related to the sine or cosine wave frequency components;
where
Zill and Cullen (Citation2000) showed that the Fourier Transform, the Fourier sine transform, and the Fourier cosine transform comprise the three Fourier transform pairs.
Fourier cosine transform;
Inverse Fourier cosine transform;
However, Mathews and Fink (Citation2004) argued that a Fourier transform requires a time series to have an infinitely wide bandwidth to transform it into an infinite number of sine or cosine frequencies. Daily closing prices of listed securities do not satisfy this requirement; therefore, it is advised to use the Discrete Fourier Transform (DFT) to transform the finite list into the frequency domain. The DFT is appropriate as both the input and the output functions of the DFT are finite.
It is important to recognise that the DFT can only accurately determine amplitudes of frequencies that are smaller than over the specified sample period. Therefore, the highest possible frequency is half of the input. Unfortunately, periods longer than the sample period will not be identified by the Discrete Fourier Transform. Daily trades will accumulate to 250 days a year, therefore the DFT will accurately calculate approximately 125 times above the sample period under determination. More importantly, the DFT only accounts for positive integer frequencies.
where
Furthermore, using the fast Fourier Transform is a less complicated tool used to conduct a DFT, since it entails matrix factorisation to reduce computations. Zill and Cullen (Citation2000) suggest that the tool can only be used on a series that is equal to the positive integare power of two. This analysis will use monthly portfolio returns from value investing strategies to nullify the problem of using daily closing stock prices.
3.2. Data
The constructed portfolio returns were sourced from Asness et al. (Citation2013) wherein which US value and momentum long-short portfolios were constructed on respective value and momentum strategies. Asness et al. (Citation2013) filtered stocks by the latest available price ratios to capture most of the information priced in by the respective markets. Fama and French (Citation1993) use lagged data that do not capture the latest relevant risks and that may highlight market inefficiencies more accurately (Asness et al., Citation2013). Furthermore, stocks with high book-to-market ratios were longed and stocks with low book-to-market ratios were shorted to form value portfolios. This strategy is in line with the original “HML” value factor that suggests low book-to-market ratios are considered expensive and high book-to-market ratios are considered cheap (Fama & French, 1992). An important factor to consider is that a six-month lag is included in the respective book values to satisfy the assumption that investors had data availability at the time of investing. The momentum portfolios are constructed in line with Jegadeesh and Titman (Citation1993) as the most recent month’s momentum return is not considered when forming the portfolios. Asness (Citation1994) suggests that this prevents short term liquidity issues influencing momentum returns. The momentum portfolios are constructed by going long on recently outperforming stocks and short on recently underperforming stocks. The portfolios are formed by choosing a position that is proportional to its cross-sectional ranking subtracted by the entire cross-section’s average rank. This provides a relative momentum strategy and reduces data noise as outliers are alleviated in a dollar-neutral portfolio. This study used US stocks due to the availability of data as far back as 1920. This allows for a holistic analysis due to high quality data.
Asness et al. (Citation2013) built combination portfolios to consider the value and momentum factors as single portfolios. The return portfolio is illustrated as:
Where
at certain time
The data ranged from the 29 February 1972 to 30 November 2020 assembled monthly. This analysis used the US stock universe that is consistent with Asness et al. (Citation2013) and is comprised of common equity with a specific book value recorded on Compustat with a minimum of 12 months of return data. Furthermore, the most illiquid stocks were eliminated from the analysis by cutting off the stocks that fell into the bottom quarter of all stocks ranked by market capitalisation (Asness et al., Citation2000). Additionally, stocks with prices below one US Dollar, REITS, financials, non-US shares and American Depository Receipts were excluded from the analysis. Therefore, the analysis focuses on liquid stocks that make up most of the market capitalisation of the US equity universe (Asness et al., Citation2013). The returns associated with the strategies were recorded monthly in line with the portfolio assemblies.
3.3. Value spreads
Asness et al. (Citation2000) used the value spread to determine the contrast between current value securities against past levels. The value spread brings in the element of magnitude across time. According to Asness et al. (Citation2000), during the tech bubble the value spread reached a level of 16, which is more than 50% larger than the last peak. The value spread is comprised of the difference between expected earnings growth between value portfolios and growth portfolios. Furthermore, the magnitude between valuation multiples between a growth portfolio and a value portfolio (Asness et al., Citation2000). The methodology is built upon the Gordon (Citation1962) model. The model is a breakdown of a specific stock’s expected return and is represented by a valuation ratio and forecasted future earnings:
where
Asness et al. (Citation2000) derived their value spreads by rebuilding the Gordon (Citation1962) model to form different expected returns for value and growth portfolios. The following equations brought forth the value spread:
The value spread is the difference between these two expected returns and is illustrated by Equationequation 12(12)
(12) :
where
The methodology illustrates that growth stocks are defined by low E/P valuation ratios and value stocks are defined by high E/P valuation ratios. This provides a better understanding of how cheap value stocks are relative to past years and illustrates the adaptability of the value premium as behavioural characteristics suggests a level of cyclicality associated with the level of cheapness of a particular stock (Asness et al., Citation2000).
3.4. Methodology
This paper determined the periodicity of the US value premium using Fourier analysis on monthly value return data in three longer tranches. The tranches are divided between 1972 and 1993; 1993 and 2014; and the period between 2014 and 2020 monthly. The returns are based on the pure value return, momentum return, a 50% value and a 50% momentum factor. The combination factors are important to show the evolution of value investing. The periods that the paper studies are in line with the 1999 tech bubble, financial crisis of 2008 and the Coronavirus pandemic as value spreads based on the price-to-book spread spiked in the years 2000, 2008 and 2020 (Asness, Citation2020). Furthermore, the spikes in the value spreads (Asness, Citation2020) will be used as beacons to test the cyclicality of the value premium to derive explanatory power by following the themes of behavioural finance and rational asset pricing theory. To provide macroeconomic and market capitalisation descriptive data, Bloomberg was utilised to source historic US debt to GDP data, risk free rates and the top 10 ranked stocks as per their respective market capitalisations.
4. Results and discussion
4.1. Fourier analysis results
The value premium experienced three consistent cycles between 1972 and 1993. The dominant cycles identified by the Fourier analysis are 5.57-month; 12.80-month and a 128.00-month cycle. The trend is illustrated by . This illustrates a cyclical event around the half year mark, one-year mark and the 10-year mark. shows the amplitudes associated with the dominant cycles. The amplitudes recorded between 1972 and 1993 are 0.99%, 0.94% and 0.90% respectively.
Figure 3. The trend of the value premium as presented by the results of the Fourier transform between March of 1972 and March of 1993.Source: Asness et al. (Citation2020) and author calculations.

Table 1. Results of the Fourier Transform and the respective significant frequencies in terms of their amplitudes related to the value premium between 1972 and 2020 monthly
The value premium’s cyclicality changed between 1993 and 2014. The stronger cycles were presented by 3.66 months; 2.98 months and 5.82 months. This is relatively shorter when compared to earlier years such as the period between 1972 and 1993. This result has been distorted as the technology bubble of 2000 resulted in a muted value premium. The trend in excess returns between 1993 and 2014 are presented by .
Figure 4. The trend of the value premium as presented by the results of the Fourier transform between June of 1993 and June of 2014.Source: Asness et al. (Citation2020) and author calculations.

The relatively shorter period between 2014 and 2020 recorded monthly cycles of 2.91 months, 5.33 months, and 3.05 months. The analysis used a smaller sample size and prevents the Fourier analysis from selecting longer term cycles associated with the value premium. The analysis was therefore extended to a period from 1999 to 2020 (). The respective amplitudes between 2014 and 2020 are stronger than earlier years at 1.4%, 1.1% and 1.1% respectively.
Figure 5. The trend of the value premium as presented by the results of the Fourier transform between October of 2014 and October of 2020.Source: Asness et al. (Citation2020) and author calculations.

The period between 1999 and 2020 is the most significant to this analysis as the value premium peaks computed by Asness (Citation2020) occur approximately every 10 years from 1999 to 2020. The peaks in value premiums indicate that relative cheapness in value stocks were highest in 2000 during the technology bubble, the year 2008 when the subprime mortgage crisis lead into a financial crisis and, 2020 where global widespread economic shutdowns attempted to curb the spread of the coronavirus. The relative value spreads are 10.10 times; 11.70 times and 12 times for the years 2000, 2008 and 2020, respectively. This result suggests that value was at its cheapest in those years between 1999 and 2020. represents the cycles associated with the time under analysis. The dominant cycles are 36.57 months, 7.76 months, and 32.00 months with amplitudes of 1.33%, 1.28% and 1.24% respectively.
Figure 6. The trend of the value premium as presented by the results of the Fourier transform between September of 1999 and March of September 2017.Source: Asness et al. (Citation2020) and author calculations.

illustrates the trend associated with the value and momentum premium between 1999 and 2020. An interesting observation is that this trend suggests that there is a 128-month cycle present with an amplitude of 0.48%. This suggests that there is a return cycle present every 10 years and this falls in line with the significant spikes recorded in the value spreads (Asness, Citation2020). The year 2000 marks the year of the tech bubble, 2008 marks the year of the global financial crisis and 2020 marks the year of the coronavirus pandemic. This suggests that the value and momentum premium is associated with an over and under reaction to information that is publicly available. illustrates the dominant cycles associated with the value and momentum premium. Namely, 3.01 months, 5.82 months, and 64 months with amplitudes of 0.58%, 0.57% and 0.49% respectively.
Figure 7. The trend of the combination of 50% value and 50% momentum premium as presented by the results of the Fourier transform between September of 1999 and March of September 2017.Source: Asness et al. (Citation2020) and author calculations.

Table 2. Results of the Fourier Transform and the respective significant frequencies in terms of their amplitudes related to the combination of the value premium and momentum premium between 1999 and 2020 monthly
The results of the Fourier transform suggest that the dominant and reoccurring cycle is around three and six months. Firstly, the value premium between 1993 and 2014 signalled a frequency of 3.66 months and 2.98 months as dominant cycle. Secondly, the value premium between 2014 and 2020 signalled cycles of 2.91 months and 3.05 months. Thirdly, the combination premium of value and momentum signalled a cycle of 3.01 months. Finally, the five-month cycle was signalled by Fourier analysis consistently from 1972 to 2020 for the value premium. The 5.57-month cycle was signalled between 1972 and 1993; the 5.82-month cycle was signalled between 1993 and 2014 and the 5.33-month cycle was presented between 2014 and 2020. The six-month cycle is present in the value premium between 1972 and 2020.Furthermore, the combination of value and momentum premium is 5.82 months between 1999 and 2020. The following section will add descriptive power to the three-month, six-month, and 10-year cycle that has been identified by the Fourier analysis over the observed period by linking rational asset pricing and behavioural economic theory to the results.
4.2. Discussion
The intuition behind the value premium suggests that investors earn a risk adjusted return by purchasing cheaper stocks because there was a net overreaction from market participants associated with the specific stock. The value investor’s gain is realised when the market realises that the stocks are undervalued and thereafter the price shall return to its intrinsic value (Lev & Srivastava, Citation2019). A plethora of biases and behavioural finance theories explain the three-month, six month and 10-year cycles identified by the Fourier analysis.
The six-month cycle is explained by a combination of the January Effect (Thaler, Citation1987) and the SAD effect (Kamstra et al., Citation2003). Between 1904 and 1974 monthly returns in January equated to 3.5% wherein which the other months only produced returns of about 0.5%. Another important point is that approximately one third of returns were realised in January (Thaler, Citation1987). Kamstra et al. (Citation2003) suggest that the seasonal and environmental changes have a psychological impact on investor reactions, and this has given rise to the SAD effect. One important fact to consider is that the three to six months of winter leads to a higher level of risk aversion and ultimately a muted stock market performance (Kamstra et al., Citation2003). These effects run between three and six months and speaks to fluctuations in risk associated with value investing strategies. These seasonal effects are the products of overreactions in the market due to external seasonal effects. The familiarity bias, confirmation bias, anchoring and loss aversion may lead to the consistent mispricing of value stocks as they are products of the SAD effect (Kamstra et al., Citation2003) and the January effect (Thaler, Citation1987).
Furthermore, the 10-year cycle that was identified by the Fourier analysis can be described by rational risk and behavioural finance theories. Value stocks are riskier than growth stocks in bad economic times and are only slightly less risky than growth stock during improved economic conditions (Zhang, Citation2005). This is because cheaper companies are associated with higher levels of leverage and uncertainty around future cash flow generation capacity (Chen & Zhang, Citation1998). These higher levels of risk ultimately lead to higher expected returns associated with value stocks. Optimism levels have a strong impact on overreactions, and it is evident that value stocks are persistently under-priced and growth stocks are overpriced due to optimism (Piotroski & So, Citation2012).
It is important to link the respective behavioural and risk associated explanations to the 10-year cycle that has been highlighted by the Fourier analysis. The main driver of optimism is the business cycle and ultimately results in mispricing due to overconfidence or under confidence. Shiller (Citation2017) explains economic fluctuations as results of narratives, specifically when an overreaction from market participants take place. Popular narratives have evolved over time and were present through the crash of 1929; the crash of 2000 and the financial crisis of 2008. It is intuitive to suggest that narratives are driving the stock market and global economy in 2020. It is interesting to note that the value spread (Asness, Citation2020) spikes all occurred in the years 2000, 2008 and 2020. These years are also the years wherein which a specific narrative has driven investor sentiment. Shiller (Citation2017) compared the crash of 1929 to the crash of 1987 and found that the crash of 1929 was initiated with a daily drop of 12.8% in the Dow Industrial Average and the crash of 1987 experienced a 22.6% drop. Furthermore, in the year 2008 the narrative around the stock market was that the economy might collapse completely as all banks would be bankrupt (Shiller, Citation2017). This signifies the potency of narratives and explains why value stocks have reached high levels of cheapness compared to historic levels in the years 2000, 2008 and 2020.
The narrative around the stock market in 2020 is that low global interest rates, increased government spending and quantitative easing has erased perceived risk associated with the stock market. This has led to astronomically higher returns for growth stocks over value stocks on the US stock exchange and has given rise to a sharp spike in the cheapness of value stocks (Israel et al., Citation2020). illustrates the cycle associated with the macroeconomic fiscal and monetary policies of the US Federal Reserve Bank. Since value stocks are riskier (Zhang, Citation2005), a value investing strategy would benefit from higher risk adjusted returns. The narrative around the economy in 2020 reduced the expected return of a value investment strategy as perceived market risk has waned.
Figure 8. Historic US debt to GDP ratios and associated risk-free rates that shows the macroeconomic policies supporting the narrative that risk has been disassociated from the US stock market.Source: Bloomberg (Citation2020d) and author calculations.

Another interesting narrative around the value premium is that investors have lost the ability to identify value stocks since the companies listed on the NYSE have changed over time. illustrates the changes in companies listed on the NYSE during 2000, 2008 and 2020. To determine a company’s cheapness a value multiple that identifies a price against a company’s assets or earnings must be established to rank the stock accordingly (Fama & French, Citation1993). When determining the cheapness of a stock by using the BM/MV (Fama & French, Citation1993), the investor’s ability to capture value characteristics was diminished between 1999 and 2020 as the nature of companies and their book market value changed significantly. The assets of the top 10 NYSE listed stocks in 2020 are not as fixed as the top 10 listed stocks in 1999. In 1999 manufacturing companies had large manufacturing plants to build cars, cellular phones, and pharmaceutical products. In 2008 oil companies dominated the NYSE and had a significant amount of fixed assets. A value investing strategy in 1999 and 2008 would have been more effective as price was easily compared to fixed assets. However, in 2020 the top 10 stocks are dominated by financial service stocks and technology-based stocks. Service based companies are more difficult to identify as human capital and intangible assets prove difficult to measure and ultimately leaves a lot of room for error. However, Fama and French (Citation2020) suggest that the value premium was muted after the value effect (Fama & French, Citation1993) was exposed to the market, but they could not produce significant evidence that it has disappeared.
Table 3. A list of the top 10 NYSE listed companies assorted in descending order by respective market capitalisation for 1999, 2008 and 2020
4.3. Conclusion and opportunities for future research
Fama (Citation2014) suggests that to test market efficiency a respectable asset pricing model has to be used. Fama (Citation2014) believes that the three-factor model and the five-factor models are the best representatives of expected returns based on their respective risk proxies. Therefore, it is important to test whether an investment strategy associated with the cycles outlined in this analysis can form factors that consistently provide risk adjusted returns against the five-factor and three-factor models. Furthermore, value investing is not idiosyncratic to the US equity markets. The analysis is to be extended to international equities, bonds, options, and currencies to identify whether value investing is an effective investing strategy internationally.
The study was conducted to determine the nature of the US value premium. The Fourier analysis has the ability to identify whether the value premium is redundant or whether it has only been dormant momentarily. This study has touched on the cyclicality of the value premium and its characteristics explained by both rational asset pricing theories and behavioral finance theories. Although Fourier analysis methods are now commonly used in finance to identify and characterise variable cyclicality, we have been unable to find any applications of the Fourier approach to the value premium in the literature. We thus contribute to the literature with this study and we add further value by identifying that the value premium does indeed exhibit cyclical behaviour with returns consistently described by three-month, six-month and 10-year cycles.
The return predictability associated with value premium changes, as investors are faced with EMH calendar anomalies over a three month and six-month period. These short-term cycles are explained by the January Effect (Thaler, Citation1987) and the SAD effect (Kamstra et al., Citation2003) while the 10-year cycle could be explained by intertemporal effects or the impact of long (roughly 7–10 years) business cycles. Value stocks are riskier than growth stocks in adverse economic conditions and are only slightly less risky than growth stocks during favourable ones (Zhang, Citation2005). This is because cheaper companies are associated with higher levels of leverage and uncertainty around future cash flow generation capacity (Chen & Zhang, Citation1998).
This work is novel as Fourier analysis has not been used before to substantiate the AMH, neither has the AMH been used to explain the cyclicality of the value premium. The value premium is partially predictable, implying that, in time and after a period of poor performance, the strategy will generate superior performance again. These results suggest that further research into anomalies of the EMH could illustrate how different investment strategies perform as market conditions change over time.
The Fourier analysis has identified a three-month cycle, a six-month cycle and a 10-year cycle associated with the value premium. The 10-year cycle is the product of a combined value and momentum premium. The cyclicality associated with the value premium suggests it adapts as markets change, thus combined value investing strategies may unlock the value premium more consistently. The 10-year cycle in the value and momentum premium aligns with historically cheap periods for value stocks and strong economic narratives. The value premium was robust in the 1920s into the early 1990s when most companies had hard fixed assets that were easily identified. However, technological revolutions, digitisation and high availability of information has weakened the value premium at the expense of the growth premium. This was driven by intertemporal changes in consumer spending patterns, business cycles and macroeconomic policies. Identifying value stocks has become difficult as NYSE listed stocks hold high levels of intangible assets and human capital; which are ultimately more difficult to price.
The value premium is not a product of luck as its returns illustrate cyclicality and do not resemble random noise. Identifying cheap and expensive stocks has become challenging and requires investors to change their value screening processes. This suggests that investors are required to innovate the multiples they use to identify the cheapness of stocks to effectively price in risks and expected returns. The value premium is a product of rational asset pricing theory and behavioural finance theory as expected returns are dependent on perceived risk levels and narratives that influence investor sentiment, which in turn are time sensitive. Historic narratives associated with downturns in economic activity such as the Spanish Influenza pandemic, the Great Depression, the Technological Bubble crash and the Financial Crisis of 2008 have affected markets. The relative cheapness of value stocks coupled with the 10-year cycle in the value premium suggests that the narratives have affected value investing.
Another important factor to consider is that because of the cyclicality of the value premium, a simple underperformance does not suggest that the value premium is redundant. The value premium is dependent on investor confidence that is stimulated by macroeconomic narratives and adapts over time. Narratives drive the value premium as expected returns are lower due to higher risks associated with value investing during downturns in the business cycle as investor risk aversion intensifies. This cyclical phenomenon suggests that value investing is not dead, the strategy has been punished by overreactions and narratives that have made investors expect lower returns from value strategies.
The value premium has underperformed growth stocks since 2016. The leading narrative in 2020 around value investing is that this underperformance is a result of redundancy in the strategy because structural economic changes prevent investors from screening for value with outdated value multiples. Furthermore, low interest rates and high levels of government spending benefited growth stocks. However, the Fourier analysis has identified a 10-year cycle, a six-month cycle and a three-month cycle that shows the adaptability of value investing. Value investing strategies have been unattractive since 2006, but remain unbowed.
Disclosure statement
No potential conflict of interest was reported by the author(s).
Additional information
Funding
Notes on contributors

Gary van Vuuren
John-Morgan Bezuidenhout is a buy-side investment analyst at Sanlam Investments Namibia. He completed his Honours degree at the University of Cape Town, South Africa specialising in financial analysis and portfolio management in the School of Economics in 2021. In addition, he holds an Honours degree specialising in Economics from the University of the Western Cape and is a registered Johannesburg Stock Exchange securities trader.
Gary van Vuuren is an extraordinary professor at the Centre for Business Mathematics and Informatics at North-West University, South Africa, where he lectures in various topics in risk management and supervises postgraduate research. He is an ex-nuclear physicist and works for EY Johannesburg as a private contractor.
References
- Asness, C. (1994). Variables that explain stock returns (PhD thesis). University of Chicago.
- Asness, C. (2020). Is (systematic) value investing dead? AQR, Cliff’s Perspectives. Retrieved September, 2020, from https://www.aqr.com/Insights/Perspectives/Is-Systematic-Value-Investing-Dead/
- Asness, C., Frazzini, A., Israel, R., & Moskowitz, T. (2014). Fact, fiction, and momentum investing. The Journal of Portfolio Management, 40(5), 75–23. https://doi.org/10.3905/jpm.2014.40.5.075
- Asness, C., Frazzini, A., Israel, R., & Moskowitz, T. (2015). Fact, fiction, and value investing. The Journal of Portfolio Management, 42(1), 34–52. https://doi.org/10.3905/jpm.2015.42.1.034
- Asness, C., Ilmanen, A., & Maloney, T. (2017). Market timing: Sin a little resolving the valuation timing puzzle. Journal of Investment Management, 15(3), 23–40. https://www.aqr.com/Insights/Research/White-Papers/Market-Timing-Sin-a-Little
- Asness, C. S., Friedman, J. A., Krail, R. J., & Liew, J. M. (2000). Style timing: Value versus growth. The Journal of Portfolio Management, 26(3), 50–60. https://doi.org/10.3905/jpm.2000.319724
- Asness, C. S., Moskowitz, T. J., & Pedersen, L. H. (2013). Value and momentum everywhere. The Journal of Finance, 68(3), 929–985. https://doi.org/10.1111/jofi.12021
- Asness, C. S., Moskowitz, T. J., & Pedersen, L. H. (2020). Value and momentum everywhere: Factors, monthly. Retrieved August 6, 2020, from Value and Momentum Everywhere: Factors, Monthly: AQR.com
- Auerbach, A. J. (1981). The index of leading indicators:” Measurement without theory,” Twenty-Five Years Later (No. w0761). National Bureau of Economic Research. The Review of Economics and Statistics. https://doi.org/10.2307/1923943
- Barberis, N., & Thaler, R. (2003). A survey of behavioral finance. Handbook of the Economics of Finance, 1(2), 1053–1128. http://dx.doi.org/10.2139/ssrn.327880
- Benhmad, F. (2013). Bull or Bear markets: A wavelet dynamic correlation perspective. Economic Modelling, 32(4), 576–591. https://doi.org/10.1016/j.econmod.2013.02.031
- Black, A. J., & Fraser, P. (2003). The value premium: Rational, irrational or random. Managerial Finance, 29(10), 57–75. https://doi.org/10.1108/03074350310768517
- Bloomberg. (2020a). Data derived from: ‘EQS SPX Index Data 1925-2020ʹ Retrieved August 6, 2020, from
- Bloomberg. (2020b). Data derived from: ‘GDDBUNST Index data 1925-2020ʹ Retrieved August 6, 2020, from
- Bloomberg. (2020c). Data derived from: ‘M1WO000V Index/M1WO000G Index data 1975-2020’ Retrieved August 6, 2020, from
- Bloomberg. (2020d). Data derived from: ‘USGG10YR index data 1925-2020ʹ Retrieved August 6, 2020, from
- Bodie, Z., Kane, A., & Marcus, A. J. (2011). Investments and portfolio management (9th ed.). New York: McGraw-Hill.
- Bouman, S., & Jacobsen, B. (2002). The Halloween indicator,” Sell in May and go away”: Another puzzle. American Economic Review, 92(5), 1618–1635. https://doi.org/10.1257/000282802762024683
- Campbell, J. Y., & Thompson, S. B. (2008). Predicting excess stock returns out of sample: Can anything beat the historical average? The Review of Financial Studies, 21(4), 1509–1531. https://doi.org/10.1093/rfs/hhm055
- Chaudhuri, S., & Lo, A. W. (2016). Spectral portfolio theory. http://doi.org/10.2139/ssrn.2788999
- Chen, N. F., & Zhang, F. (1998). Risk and return of value stocks. The Journal of Business, 71(4), 501–535. https://doi.org/10.1086/209755
- Dupernex, S. (2007). Why might share prices follow a random walk. Student Economic Review, 21(1), 167–179. https://doi.org/10.1080/1331677X.2018.1456358
- Fama, E. F. (1965). The Behavior of Stock-market Prices. The Journal of Business, 38(1), 34–105. https://doi.org/10.1086/294743
- Fama, E. F. (1970). Efficient Capital Markets: A review of theory and empirical work. The Journal of Finance, 25(2), 383–417. https://doi.org/10.2307/2325486
- Fama, E. F. (1998). Market efficiency, long-term returns, and behavioral finance. Journal of Financial Economics, 49(3), 283–306. https://doi.org/10.1016/S0304-405X(98)00026-9
- Fama, E. F. (2014). Two Pillars of Asset Pricing. American Economic Review, 104(6), 1467–1485. https://doi.org/10.1257/aer.104.6.1467
- Fama, E. F., & French, K. R. (1993). Common risk factors in the returns on stocks and bonds. Journal of Financial Economics, 33(1), 3–56. https://doi.org/10.1016/0304-405X(93)90023-5
- Fama, E. F., & French, K. R. (2010). Luck versus skill in the cross‐section of mutual fund returns. The Journal of Finance, 65(5), 1915–1947. https://doi.org/10.1111/j.1540-6261.2010.01598.x
- Fama, E. F., & French, K. R. (2012). Size, value, and momentum in international stock returns. Journal of Financial Economics, 105(3), 457–472. https://doi.org/10.1016/j.jfineco.2012.05.011
- Fama, E. F., & French, K. R. (2015). A five-factor asset pricing model. Journal of Financial Economics, 116(1), 1–22. https://doi.org/10.1016/j.jfineco.2014.10.010
- Fama, E. F., & French, K. R. (2018). Volatility lessons. Financial Analysts Journal, 74(3), 42–53. https://doi.org/10.2469/faj.v74.n3.6
- Fama, E. F., & French, K. R. (2020). The value premium (Fama-Miller Working Paper (20–21)). http://dx.doi.org/10.2139/ssrn.3525096
- Friedman, M. (1953). The case for flexible exchange rates. In Essays in positive economics (Vol. 157, p. 203). Chicago: University of Chicago Press, 1, 953.
- Gabor, D. (1946). Theory of communication. Part 1: The analysis of information. Journal of the Institution of Electrical Engineers-Part III: Radio and Communication Engineering, 93(26), 429–441. https://doi.org/10.1049/ji-3-2.1946.0074
- Gordon, M. J. (1962). The investment, financing, and valuation of the corporation. RD Irwin.
- Graham, B. (1973). The Intelligent Investor 4 (4th rev. ed., pp. 315–316). New York: Harper and Row.
- Guerrien, B., & Gun, O. (2011). Efficient market hypothesis: What are we talking about. Real-World Economics Review, 56(11), 19–30.
- Hayes, M. H. (2009). Statistical digital signal processing and modeling. John Wiley & Sons.
- Hirshleifer, D. (2001). Investor psychology and asset pricing. The Journal of Finance, 56(4), 1533–1597. https://doi.org/10.1111/0022-1082.00379
- Israel, R., Laursen, K., & Richardson, S. A. (2020). Is (systematic) value investing dead? The Journal of Portfolio Management Quantitative Special Issue.
- Ito, M., Noda, A., & Wada, T. (2016). The evolution of stock market efficiency in the US: A non-Bayesian time-varying model approach. Applied Economics, 48(7), 621–635. https://doi.org/10.1080/00036846.2015.1083532
- Ito, M., & Sugiyama, S. (2009). Measuring the degree of time varying market inefficiency. Economics Letters, 103(1), 62–64. https://doi.org/10.1016/j.econlet.2009.01.028
- Jegadeesh, N., & Titman, S. (1993). Returns to Buying Winners and Selling Losers: Implications for Stock Market Efficiency. The Journal of Finance, 48(1), 65–91. https://doi.org/10.1111/j.1540-6261.1993.tb04702.x
- Kamstra, M. J., Kramer, L. A., & Levi, M. D. (2003). Winter blues: A SAD stock market cycle. American Economic Review, 93(1), 324–343. https://doi.org/10.1257/000282803321455322
- Kim, J. H., Shamsuddin, A., & Lim, K. P.(2011). Stock return predictability and the adaptive markets hypothesis: Evidence from century-long US data. Journal of Empirical Finance, 18, 868–879. https://doi.org/10.1016/j.jempfin.2011.08.002
- Lev, B., & Srivastava, A. (2019). Explaining the recent failure of value investing. NYU Stern School of Business (October 25). http://dx.doi.org/10.2139/ssrn.3442539
- Lim, K. P., & Brooks, R. D. (2006). The evolving and relative efficiencies of stock markets: Empirical evidence from rolling bicorrelation test statistics. SSRN931071.
- Lim, K. P., Luo, W., & Kim, J. H. (2013). Are US stock index returns predictable? Evidence from automatic autocorrelation-based tests. Applied Economics, 45(8), 953–962. https://doi.org/10.1080/00036846.2011.613782
- Lo, A. W. (2004). The adaptive markets hypothesis. The Journal of Portfolio Management, 30(5), 115–129. https://doi.org/10.3905/jpm.2004.442611
- Lo, A. W., & MacKinley, A. C. (1999). A non-random walk down Wall Street. Princeton University Press.
- Malkiel, B. G. (2003). The Efficient Market Hypothesis and Its Critics. Journal of Economic Perspectives, 17(1), 59–82. https://doi.org/10.1257/089533003321164958
- Mathews, J. H., & Fink, K. D. (2004). Numerical methods using MATLAB (Vol. 4). Pearson prentice hall.
- Oseni, I. O., & Nwosa, P. I. (2011). Stock market volatility and macroeconomic variables volatility in Nigeria: An exponential GARCH approach. European Journal of Business and Management, 3(12), 43–53. https://www.iiste.org/Journals/index.php/RJFA/article/view/1295/1215
- Pearson, K. (1905). The problem of the random walk. Nature, 72(1867), 342-342. https://doi.org/10.1038/072342a0
- Piotroski, J. D., & So, E. C. (2012). Identifying expectation errors in value/glamour strategies: A fundamental analysis approach. The Review of Financial Studies, 25(9), 2841–2875. https://doi.org/10.1093/rfs/hhs061
- Quiggin, J. (1994). Regret theory with general choice sets. Journal of Risk and Uncertainty, 8(2), 153–165. https://doi.org/10.1007/BF01065370
- Sharpe, W. F. (1964). Capital asset prices: A theory of market equilibrium under conditions of risk. The Journal of Finance, 19(3), 425–442. https://doi.org/10.1111/j.1540-6261.1964.tb02865.x
- Sharpe, W. F. (1994). The Sharpe Ratio. Journal of Portfolio Management, 21(1), 49–58. https://doi.org/10.3905/jpm.1994.409501
- Shiller, R. J. (2017). Narrative economics. American Economic Review, 107(4), 967–1004. https://doi.org/10.1257/aer.107.4.967
- Shleifer, A. (2000). Inefficient markets: An introduction to behavioural finance(pp. 1–216). Oxford University Press. https://doi.org/10.1093/0198292279.001.0001
- Singh, S. (2012). Investor irrationality and self-defeating behavior: Insights from behavioral finance. Journal of Global Business Management, 8(1), 116. https://www.proquest.com/openview/92b3e92b05147b0916f85dc2308bdde/1.pdf?pq-origsite=gscholar&cbl=406316
- Smith, G. (2012). The changing and relative efficiency of European emerging stock markets. The European Journal of Finance, 18(8), 689–708. https://doi.org/10.1080/1351847X.2011.628682
- Thaler, R. H. (1987). Anomalies: The January effect. Journal of Economic Perspectives, 1(1), 197–201. https://doi.org/10.1257/jep.1.1.197
- Thomson, D., & van Vuuren, G. (2016). Forecasting the South African business cycle using Fourier analysis. International Business & Economics Research Journal (IBER), 15(4), 175–192. https://doi.org/10.19030/iber.v15i4.9755
- Tilson, W. (2005). Applying behavioral finance to value investing. Artikel T2 Partners LLC, 1.
- Urquhart, A., & McGroarty, F. (2014). Calendar effects, market conditions and the Adaptive Market Hypothesis: Evidence from long-run US data. International Review of Financial Analysis, 35(2), 154–166. https://doi.org/10.1016/j.irfa.2014.08.003
- Welch, I., & Goyal, A. (2008). A comprehensive look at the empirical performance of equity premium prediction. The Review of Financial Studies, 21(4), 1455–1508. https://doi.org/10.1093/rfs/hhm014
- Zhang, L. (2005). The value premium. The Journal of Finance, 60(1), 67–103. https://doi.org/10.1111/j.1540-6261.2005.00725.x
- Zill, D. G., & Cullen, M. R. (2000). Advanced engineering mathematics (2nd ed.). Massachusetts: Jones & Bartlett Publishers.