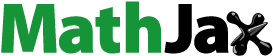
Abstract
While Indonesia has been experiencing relatively considerable and stable economic growth in the last decades, the country is prone to income disparity across regions due to uneven distribution of population, natural resources and the persistent impacts of centralized development imposed by the New Order regime. This study examines the economic growth convergence in East Java, Indonesia, from 2010 to 2019 and explores the influence of government expenditure on education, health, and capital sector on the economic growth convergence. By considering spatial dependence across regions, the result shows no strong evidence of regional income convergence in East Java. Also, this research claims the presence of spillover effect of government expenditure on education and capital sector on regional income growth. Notably, higher government expenditure of the education sector in one region could stimulate higher economic growth of its neighboring regions. Conversely, higher government expenditure on the capital sector in one region may lower the economic growth of its surrounding regions.
PUBLIC INTEREST STATEMENT
Indonesia is prone to regional income disparity due to unequal population and natural resources distribution across regions and past centralized development planning. This study shows that wealthier regions have higher economic growth than poorer regions during the observation, suggesting persistent regional income disparity across regions. Moreover, government spending on the education and capital sector affects the regional income disparity across regions in Indonesia.
1. Introduction
While Indonesia has been experiencing relatively considerable and stable economic growth in the last decades, economic disparity problems remain (Purwono et al., Citation2021). The economy grew on average roughly 5 percent between 2010 and 2019 (World Bank, Citation2020a). However, as an archipelagic country with vast areas, Indonesia is prone to an economic disparity across regions due to uneven distribution of population, natural resources and the long-lasting impacts of past centralized development perpetuated by the New Order regime (Irawan, Citation2014; Resosudarmo & Vidyattama, Citation2006; Sugiharti, Citation2013). It is estimated that more than half of Indonesia’s population lives on Java Island (Dsikowitzky et al., Citation2019) although several provinces such as East Kalimantan, Papua, and Riau are rich in natural resources (Hill et al., Citation2008; Komarulzaman & Alisjahbana, Citation2006). Before the implementation of the decentralization regime in 2001, Indonesia’s development was centralized and the planning stretched for nearly 50 years (Alm et al., Citation2001). Therefore, economic disparity problems across regions remain.
From the perspective of regional development theory, there are two competing views to explain regional incomeFootnote1 behavior across regions. First, the neoclassical growth theory predicts that poorer regions’ incomes tend to grow faster than the richer ones. This condition results in a “catch-up” effect that allows regional income to converge in the end (Barro & Sala-i-Martin, Citation2006). Second, endogenous growth and new economic geography (NEG) theory emphasize the importance of space and the role of spillover in regional growth, claiming that incomes across regions tend to diverge (Wardaya & Landiyanto, Citation2005). These contradictory theories form the basis of the current research, aiming to examine development processes from empirical evidence.
Methodologies to understand regional growth behavior—such as spatial econometrics, which captures spatial dependence among regions (Anselin, Citation2010)—have been developing alongside the theories. Regions are believed to interact with each other in many channels like technology-spillovers and the mobility of production factors that will lead to an externality in the form of regional income spillovers (Sugiharti, Citation2013). A pioneering study conducted by Rey and Brett attempted to explore regional income convergence in the US by using spatial econometrics (Seya et al., Citation2011). They observed model misspecification in a previous study due to the overlooked spatial aspects. Since then, there have been a growing number of studies examining regional convergence process by considering the spatial aspects.
East Java is a good case to understand the regional income convergence process. The province holds significant geopolitical importance for Indonesia (Irawan, Citation2014) and one of the most significant contributors to Indonesia’s economy—reaching 15 percent of national GDP in 2019 (Statistics Indonesia, Citation2020a). The province showed higher economic growth than national’s growth for a decade; for example, in 2010 the growth was 6.68 percent, which was higher than the 6.22 percent national growth, and in 2019 it was 5.52 percent, which was also higher than the 5.02 national growth (Statistics Indonesia, Citation2020b). Still, there was income disparity, indicated by the relatively high Gini coefficient—reaching 0.415 in 2015 and 2017, the highest since 2012 (Statistics Indonesia, Citation2020c).
There are studies attempting to explain the regional income convergence in East Java. For example, Wardaya and Landiyanto (Citation2005) investigated the convergence by considering the spatial aspect and found that there were two spatial regimes in East Java with diverging tendencies from 1983 to 2002. Sugiharti (Citation2013) explored the spatial dependence and spatial regimes of regional income convergence from 2004 to 2010; and found that there are two spatial regimes with convergence tendencies. Meanwhile, Irawan (Citation2014) explored the regional income convergence in East Java from 1980 to 2005 by considering the role of government consumption (excluding government’s expenditure on capital) and human capital that were proxied by the number of people with a diploma degree compared to the total population. Irawan (Citation2014) concluded that spatial dependence does not apply across regions in East Java and government consumption does not affect growth, but human capital does influence the regional growth convergence.
However, such studies did not consider the role of government policy in both physical and human capital investment. This research, therefore, aims to analyze this factor in the regional income convergence in East Java from 2010 to 2019—filling in the gap in the literature by incorporating government policies on physical and human capital investment, such as education and health. These are proxied by using government expenditure on capital, education, and the health sector.
The remaining of the paper is organized as follows: in part 2, the literature is reviewed; in part 3, the data and methodology are outlined; in part 4, the empirical result and discussion are presented; in part 5, the conclusion is drawn.
2. Literature review
2.1. Convergence concept
By definition, income convergence, in the most general sense, is a process of equalization or the decreasing trend of income disparity among different entities, e.g., countries or regions (Paas et al., Citation2007). This proposition comes from the neoclassical theory postulated by Solow (1956), which claims that poorer regions or economies grow faster than their richer counterparts. By holding the assumptions of constant return to scale, diminishing marginal return to capital and labor, and perfect mobility of factors of production, the theory predicts that the regional income inequality tends to evaporate over time (Fischer & Stirböck, Citation2004; J. Yildirim et al., Citation2009).
According to Capello (Citation2016), regional income convergence could be interpreted as a result of regional interaction in capital and labor. In relatively richer regions, higher capital accumulation yields lower return to capital, and therefore capital moves to poorer regions. At the same time, labor moves from lagging regions to the leading ones due to higher wage levels. This process eventually equalizes regional income growth’s trajectory between richer and poorer regions. Knowledge spillover is another potential channel for poorer regions to grow faster, catch up with richer regions’ income, and reach a convergence (Rey, Citation2004). Richer regions are usually ahead in innovating production processes, so poorer regions follow and adopt the innovation. Adoption is cheaper because there is no cost needed for research and development. In addition, knowledge may also spill over in the form of positive externalities produced by firms in specific locations, which affect the production process of firms in other locations.
There are two main approaches to examining income convergence across regions: σ-convergence and β-convergence (Shankar & Shah, Citation2003; Soukiazis & Castro, Citation2005; Tian et al., Citation2016). The σ-convergence refers to the declining dispersion of real per capita income across groups of economies over time (Young et al., Citation2008). This approach views regional incomes converging at the same rate (Gezici & Hewings, Citation2004). The σ-convergence is related to the behavior of cross-sectional standard deviation or the coefficient of variation of per capita output over time (Gömleksiz et al., Citation2017).
The second approach is β-convergence, which refers to a faster income growth rate of poorer regions relative to richer regions that eventually results in the disappearance of regional income differentials (Dey & Neogi, Citation2015). The presence of β-convergence is estimated by regressing the per capita growth rate on its initial value (Dey & Neogi, Citation2015; Soukiazis & Castro, Citation2005). There are two types of β-convergence: 1) absolute or unconditional β-convergence and 2) conditional β-convergence. The absolute β-convergence assumes that technological progress and the steady-state level are homogeneous across regions (Kangasharju, Citation1998). Therefore, it is expected that there will be a negative relationship between the per capita growth rate and its initial income levels in the β-convergence regression even without controlling other variables (Sugiharti, Citation2013).
Nevertheless, the conditional β-convergence assumes that the technological progress and steady-state level may differ across regions. Thus, the estimation of the β-convergence could be calculated by adding variables that reflect the technological progress and steady-state level differences in the β-convergence regression equation. For the empirical purpose, the technological progress differences are usually assumed to be exogenous and not proxied by any variables. Meanwhile, the steady-state level differences are usually proxied by the saving rate, the population growth rate, and the percentage of skilled workers (Kangasharju, Citation1998).
Both σ-convergence and β-convergence are related to each other. The faster income growth of poorer regions relative to richer regions (β-convergence) tends to produce a declining income gap across regions (σ-convergence) (Dey & Neogi, Citation2015). However, the presence of β-convergence does not necessarily imply the existence of σ-convergence. The β-convergence may coexist with a stable or increasing income coefficient variation due to region-specific shocks, which impact regional growth rates in a way that offsets the β-convergence (Dey & Neogi, Citation2015; Rey & Montouri, Citation1999; Shankar & Shah, Citation2003). According to Young et al. (Citation2008), β-convergence becomes necessary but not sufficient for σ-convergence. For instance, let us consider two regions: A and B that have the same level of income at the beginning, with the assumptions: 1) B starts at its balanced growth path and A starts quite far from its balanced growth path; 2) the β-convergence holds, i.e., region A outgrows region B; and 3) the initial variance or coefficient of variation is zero. The variance or coefficient variation will rise over time as A grows faster than B and approaches a higher level of balanced growth path. It indicates that β-convergence generates a rising variance or coefficient of variation (Young et al., Citation2008). Therefore, the empirical analysis of income convergence should consider both approaches (Shankar & Shah, Citation2003).
Research has explored regional income convergence in different contexts and time frames. For instance, a study by Kangasharju (Citation1998) investigated the existence of σ-convergence and β-convergence (absolute and conditional) in Finland from 1934 to 1993. The results show that σ-convergence existed, i.e., the coefficient variation of per capita income decreased during the period of observation; and that there was absolute β-convergence. The study also added regional dummies and shares of the agricultural sector to explore the conditional β-convergence, concluding that the conditional β-convergence existed. Likewise, Young et al. (Citation2008) examined the occurrence of σ-convergence and absolute β-convergence in 3,058 counties in the US from 1970 to 1998. They found absolute β-convergence with convergence rate falling between 6.3 and 9.8 percent; and no σ-convergence. The estimated standard deviation of real per capita income in 1998 was 5.8 percent higher than in 1970.
Rapacki and Próchniak (Citation2009) investigated σ-convergence and absolute β-convergence in 27 formers socialist (transition) countries in 1990–2005. They found that β-convergence did not occur in 1990–2005, but it did in 2000–2005 with a rate of 1.39 percent annually. As for the σ-convergence in 1990–2005, the evidence was not substantial; but in 2000–2005, it was. Dobrinsky and Havlik (Citation2014) examined convergence in 27 country members of the European Union (EU) and also 10 new EU state members (called NMS countries) from 1995 to 2011. Overall, they concluded strong evidence of absolute β-convergence and σ-convergence among the 27 EU countries. The standard deviation of regional income (σ-convergence) of the NMS countries tended to rise until about 2007 before it started to decline. They also observed absolute β-convergence among the NMS countries, even though the relationship was weak. Dobrinsky and Havlik (Citation2014) also investigate conditional β-convergence by adding several independent variables into the convergence regression equation: 1) unit labor cost; 2) domestic saving; 3) domestic and foreign saving; and 4) export performance. The estimation shows that conditional β-convergence existed among the 27 EU countries with a convergence speed of about 1.6 percent to 2.9 percent per year. Dey and Neogi (Citation2015) studies σ-convergence and absolute β-convergence of seven country members of the South Asian Association for Regional Cooperation (SAARC) before (1970–1984) and after the cooperation (1985–2011). Their finding reveals no σ-convergence before the formation and σ-convergence after. There was no substantial evidence of absolute β-convergence before the formation, but there was β-convergence after. Meanwhile, more recent studies have extended the application of the convergence concept for poverty analysis (López-Calva et al., Citation2021; Ravallion, Citation2012), mortality rate (Goli & Arokiasamy, Citation2014; Janssen et al., Citation2016), and social capital (Sarracino & Mikucka, Citation2017).
At the opposite end, endogenous growth theory, which relies heavily on the assumption of increasing return to scale claims that income disparity among regions is undeniable; and that the source of regional income differences is mainly due to human capital and technology level (Gardiner et al., Citation2004). It implies that regional incomes behavior depends on the extent to which low-technology regions follow high-technology regions or advanced regions, the degree of geographical diffusion of technology and knowledge, and the mobility of workers in knowledge-based activities (Rumayya, 2005). The more knowledge or technology progress and the more workers in knowledge-based activities are localized in the more advanced regions, the regional income disparity will be likely to persist.
Meanwhile, NEG, which stresses the significant roles of space and agglomeration believes that the source of income disparity is localized increasing return to scale from spatial agglomeration or specialized economic activities. The theory argues that economic activities in the form of backward and forward linkages, production factors mobility and knowledge spillovers push spatial agglomeration and clustering, leading to core-periphery equilibria (Gardiner et al., Citation2004). For instance, in the forward linkages, firms tend to locate in regions with the higher market potential to reduce transportation costs and maximize economic scale. Later, the firms attract more workers who will spend their income locally, leading to a rising market size in the regions. This condition will, in turn, attract more firms to locate in the same or nearby regions, creating clustered regions. Furthermore, backward linkages push firms to locate around inputs suppliers to minimize their production costs, including transportations and inputs costs. When many firms minimize their production costs by shortening their input linkages, there will be a tendency of firms to cluster in space. Eventually, this will induce persistent regional income inequalities across regions (Källström, Citation2012).
Several empirical works have been conducted to test the theory of NEG, many of which pay attention to wage equilibrium, i.e., whether wage level is higher in regions with higher market potential (Fallah et al., Citation2011; Fingleton & Fischer, Citation2010). Another theory focuses on assessing whether market potential affects the decision-making of firm locations while another one links workers’ migration decisions and market potential (Fallah et al., Citation2011). A study by Fallah et al. (Citation2011) found a positive relationship between average wage level and market potential in the context of US metropolitan areas. They estimated that one standard deviation of rising market potential is associated with a 2.3 percent point of higher wage level. Likewise, Brakman et al. (Citation2006) also found a spatial wage structure in the context of the EU spanning from 1992 to 2000, as suggested by the NEG. It implies that the wage level is higher in the center of the economy and vice versa. Furthermore, Pons et al. (Citation2007) concluded that workers’ migration to industrial agglomeration regions in Spain had pushed the agglomeration process cumulatively. It suggests a relationship between workers’ localization decisions and the potential market of host regions.
2.2. Spatial dependence and convergence process
Spatial dependence or autocorrelation refers to a functional relationship between a considered indicator (such as GDP per capita) in a region and other neighboring regions (Lim, Citation2016). In other words, it describes the effects that neighboring regions have on each other in geographical proximity. It suggests the interdependence relationships among observations in cross-sectional datasets that include a geographical unit of analysis (Magalhães et al., Citation2000; Niebuhr, Citation2000). According to Tobler (Citation1970), “everything is related to everything else, but near things are more related than distant things.” There are two types of spatial autocorrelation: positive and negative. Positive autocorrelation exists when similar values of a considered indicator are clustered in space. Negative autocorrelation occurs when different values of a variable are clustered in space (Magalhães et al., Citation2000).
There are two sources of spatial dependence: nuisance dependence and substantive dependence (Feldkircher, Citation2006). Nuisance dependence pertains to data measurement errors due to the mismatch between the geographical boundaries of actual economic processes and administrative boundaries (such as a county, city, or state) employed to collect the data. On the other hand, substantive spatial dependence may result from true spatial interactions of a variable of interest across regions (Lim, Citation2016). Substantive dependence reflects the complex interactions of each region with another through many channels such as labor and capital movement and input-output linkages. Consequently, the economy’s performance in one region is affected by its neighboring regions (Capello, Citation2016). Overlooking the two forms of spatial dependence in the regional economy’s performance analysis, including regional income convergence, might lead to biased and inefficient estimates (Magalhães et al., Citation2000).
To recall, the conventional β-convergence analysis employs a cross-sectional regression model with regional income growth as a dependent variable and its initial value as a primary explanatory variable. This traditional model is estimated by using the ordinary least square (OLS) method of estimation. By assuming that the error terms of the OLS regression model are independently and identically distributed (iid) with zero mean, the traditional model estimation provides the best linear unbiased estimator (BLUE) (Wooldridge, Citation2015). Nevertheless, overlooking the presence of spatial dependences in the traditional model may create an estimation issue, such as misspecification problem, reflected in the spatially correlated error terms. It means that the fundamental assumption of the OLS estimation, that is (iid), is violated (Niebuhr, Citation2000). Consequently, the estimates of β-convergence and its convergence speed will be biased (Källström, Citation2012; Magalhães et al., Citation2000). Therefore, recent studies have agreed that spatial dependence is a key determinant in regional growth and its convergence process (Lim, Citation2016).
Many empirical studies have confirmed the bias in the β-convergence analysis due to the negligence of spatial dependence. For instance, a study by Postiglione et al. (Citation2020) found that traditional β-convergence estimates (i.e., ignoring spatial dependence) were lower than estimates obtained from the spatial model in the context of 1,133 EU regions from 1991 to 2014. Notably, the convergence rate obtained from the traditional approach ranged from 2.6 to 2.7 percent, while the estimates from the spatial model reached 2.9 to 3.0 percent annually. Niebuhr (Citation2000) estimated that while the convergence rate from the traditional estimate was 1 percent, the estimates from the spatial model were between 0.5 and 0.7 percent per year in West Germany. Similarly, Magalhães et al. (Citation2000) found that the convergence speed obtained from the traditional model was slightly lower than estimates from the spatial model in Brazil. They further argued that even though the bias was slight, it still indicates the significance of spatial dependence in the regional income modeling.
2.3. The effect of government expenditure on growth
Previous studies have generally found inconclusive findings of the effect of government expenditure on growth (Amusa & Oyinlola, Citation2019; Wu et al., Citation2010). Some observed positive effects of government expenditure on growth. The Keynesian theory claims that the efficient use of public resources could increase the economy’s productive capacity and thus induces economic growth (Dudzeviciute et al., Citation2018). Others argue that government spending might hinder economic growth by crowding out private-sector spending (Amusa & Oyinlola, Citation2019). Meanwhile, others believe that the growth-inducing effects of government spending depend on the types of expenditure. For instance, Hansson and Henrekson (Citation1994) found that government transfer and consumption negatively affect growth, while educational spending boosts growth. Barro (Citation1990) revealed that unproductive governmental expenditure impedes growth, while productive governmental expenditure’s effects are indeterminate, modulated by the government behavior and the amount of the expenditure itself.
One important productive governmental spending is capital investment, both physical and human capital investment. The government expenditure on physical capital is a source of public infrastructure provision in a region. The sufficient provision of public infrastructure pushes the improvement in market access, decreasing unit cost of production and increasing private investment (Lall & Yilmaz, Citation2001), leading to higher economic growth. Similarly, the government expenditure on human capital areas—such as the education and health sector—also contributes to human capital formation, enhancing labor force productivity (Dudzeviciute et al., Citation2018), which increases economic growth. Therefore, the spatial distribution of stock of capital, both physical and human capital, as a result of government expenditure or public investment, to some extent, influence the regional income disparity or regional income convergence process (Lall & Yilmaz, Citation2001).
Government expenditure or investment of one region may also have spillover effects on its neighboring regions. Previous studies confirmed that physical infrastructure investment in a particular region produces positive spillover in adjacent regions by stimulating higher output. However, physical infrastructure supply also creates severe inequality by changing neighboring regions’ comparative and competitive advantages. If production factors can move at zero cost, public infrastructure investment in one location could move a production site away from one region to another or provide access to neighboring regions that are previously not accessible (Lall & Yilmaz, Citation2001). Government expenditure on the education and health sector in one region might also spill over its neighboring regions. By assuming that higher government expenditure in the health and education sector reflects better public services in a region, some may be inclined to move to regions with higher education and health government expenditure (Nguyen-Hoang & McPeak, Citation2010). This will create an unequal spatial distribution of human capital across regions.
Several studies have incorporated the role of government expenditure on regional convergence analysis. Lall (Citation2007) concluded that government expenditure on transport and communication infrastructure has positively affected regional economic growth in 24 regions in India. Such effects are not only influenced by government expenditure made by individual regions but also by the spillover effects of the expenditures by the surrounding regions. Further analysis indicates that the lagging regions exhibited a higher economic growth rate from the transport and communication expenditure (investment) than the leading regions. This conclusion is in line with the findings by Puga (Citation2002), stating that benefits of infrastructure are lower in leading regions relative to lagging regions. This finding suggests that government expenditure on capital (transport and communication infrastructure) induces regional income convergence in India. Likewise, Chiquiar (Citation2005) found that government expenditure on capital (investment) and government consumption influenced growth in Mexico in 1970–1985. Nevertheless, such effect disappeared in 1985–2011.
In contrast, Irawan (Citation2014) found that government consumption (excluding government spending on capital or investment) has no effects on growth in East Java in 1980–2005. Similarly, Drezgić (Citation2011) found an insignificant effect of government capital expenditure on regional income convergence in Croatia in 2000–2007. This may have been caused by the efficiency problems such as a lack of planning and insufficient cost–benefit analysis of the projects.
In short, most studies employed government expenditure on capital as a proxy of government role in the convergence analysis. Meanwhile, the role of human capital in convergence research is usually proxied by a ratio of educated people to population (Irawan, Citation2014), average years of schooling or illiteracy rate (Chiquiar, Citation2005). For instance, Chiquiar (Citation2005) concluded that poorer regions with higher human capital represented by average years of schooling showed a higher growth rate than richer regions, which then led to regional income convergence in Mexico. Other than government expenditure and human capital, previous studies have also explored the role of foreign direct investment (Choi, Citation2004), export (Akram & Rath, Citation2020; Baddeley, Citation2006), and structural policy change (Soukiazis & Castro, Citation2005) in the regional income convergence.
3. Methodology
3.1. Data
The data utilized in this study was: product (GRDP per capita), government expenditure on education sector per capita, government expenditure on health sector per capita, government expenditure on a capital sector per capita, the proportion of agriculture in the economy, average years of schooling, and the interaction of average years of schooling and GRDP per capita base year. All these variables, except for the proportion of agricultural sector, average years of schooling, and the interaction of average years of schooling and GRDP per capita base year, were represented in Indonesia Rupiah (IDR) nominal currency and then was deflated into the constant price of year 2010 to obtain its real value. Afterward, it was converted into a natural logarithm form. The data covered all 38 regions across East Java from the year 2010 to 2020.Footnote2 These data were obtained from Statistics Indonesia (www.bps.go.id). The descriptive statistics of the variables used in the analysis is provided in the .
Table 1. Descriptive statistics
3.2. Analytical Framework
The first part of the analysis focuses on σ-convergence and β-convergence without considering spatial dependence across regions. The σ-convergence is measured by the evolution of coefficient variation (CV) of regional income per capita among economies or regions over time. The increasing trend of CV overtime suggests rising regional income inequality across regions and vice versa. The formula of CV in a given year or time is provided below:
where is standard deviation of real GDP per capita across regions in time t and
is mean or average of real GDP per capita across regions at period t. From the equation (1) above, the standard deviation of regional income per capita is estimated as follows:
where is the number of regions in the study and
is real GDP per capita of region i at period t. Following Young et al. (Citation2008), a variance ratio test (F-ratio test) is also applied to explore the statistical differences of standard deviations of regional income per capita overtime.
Furthermore, following Rey and Montouri (Citation1999), the analysis of the absolute β-convergence for cross-sectional data is estimated through the regression equation below
where Ln shows logarithm natural, represents real GDP per capita of region i at time t (end of observation),
indicates real GDP per capita of region i at initial observation. Thus, the dependent variable of the regression equation above shows the growth rate of GDP per capita of region i during the observation. Meanwhile,
and
are model coefficients to be estimated and
is error term. The regression equation (3) is estimated by using OLS with a robust standard error option to avoid heteroscedasticity. The negative sign of coefficient of
and its statistical significance suggest the presence of
convergence (Magalhães et al., Citation2000).
Meanwhile, the conditional β-convergence is obtained by adding several essential variables in the β-convergence regression into equation (3). Specifically, the conditional β-convergence is estimated through the equation below:
where is vector of k additional region i’ characteristic at initial observation (2010) that affect regional income’s steady state differences. Following the work of Chiquiar (Citation2005),
consists of share of agriculture, average years of schooling, interaction variable of GDP per capita base year and average years of schooling. Furthermore,
includes government expenditure on capital, health, and education in the base year (2010). Meanwhile,
denotes the estimated coefficients of k variables.
3.3. Spatial weight matrix and spatial modelling
The exploration of spatial dependence starts with defining spatial weight matrix (usually denoted as “W”) and Moran I-Statistics calculation. Literature in spatial econometrics provides various methods to specify spatial weight matrix: Rook contiguity, Queen contiguity, K-nearest neighborhood, and Great Circle Distance, among others. A statistical procedure called Moran I Statistics could be utilized to determine one of those ways in specifying the appropriate spatial weight matrix. The formula of Moran I-statistics is given as follows:
where Xit is variable of interest, i.e., GDP per capita in region i and year t, N is the number of regions, is the spatial weight, and Nb is the sum of all weight.
To capture a regional interaction through complex mechanisms such as knowledge spillovers, input-output linkage and production factors mobility, a spatial dependence model is constructed based on convergence framework. There are some variations of spatial dependence models such as spatial autoregressive model (SAR), spatial cross-regressive model (SCRM), spatial lag of X model (SLX) and spatial Durbin model (SDM) (Sugiharti, Citation2013). The choice of spatial model specification depends on the Moran I statistics calculation and diagnostic test such as the Lagrange multiplier (LM) test (Florax et al., Citation2003; Ojede et al., Citation2018).
Anselin (2001) describes that the SAR model is appropriate when the spatial autocorrelation indicates a global interaction among the dependent variable at the observed area. Anselin (2001) also claims that SAR can provide both direct and indirect effect from every independent variable in a given area. On the other hand, LeSage and Pace (Citation2009) claim that SDM is a suitable model when the disturbance terms and dependent variables exhibit spatial spillover. The SDM is also able to exploits both direct and indirect effect from dependent variables and the error term. Following the work of Llorca-Rodríguez et al. (Citation2021) and considering the aims of this research, the current study employs the spatial lag of X (SLX) model (LeSage & Pace, Citation2009). The model is the most flexible choice to illustrate the spatial spillover effects of the explanatory variables i.e., the government expenditure of neighboring regions. Moreover, it has the advantage of a straightforward interpretation: the direct effects are represented by non-spatial variables, while the spillover effects are indicated by spatially lagged independent variables (Llorca-Rodríguez et al., Citation2021). Therefore, the formulation of the spatial model is constructed as the extension of the equation (5) and expressed as follows:
where represents the k spatial model parameter to be estimated and
consists of k independent variables, i.e., government expenditure on capital, health, education, share of agriculture and share of manufacturing sector of region i at initial observation or base year, i.e., 2010.
4. Result and discussion
4.1. Conventional convergence process in East Java
Firstly, the convergence process in East Java is estimated by using the σ-convergence. This analysis is conducted along with the examination of coefficient variation (CV) over time. We split the σ-convergence analysis into two: 1) all regions (38 regions in East Java); and 2) 37 regions excluding the City of Kediri as it has a far higher GDP per capita compared to other regions in East Java. The city has the fifth-largest tobacco manufacturer in Indonesia. It is estimated that 80 percent of the GDP comes from the tobacco industry (Statistics Indonesia, Citation2018d). Thus, we treat the City of Kediri as an outlier. The result of σ-convergence is shown in below:
Table 2. Estimation of sigma convergence in East Java from 2010–2020
Based on , the coefficient of variation (CV) of real GDP per capita of the 38 regions was 0.03884 in 2011, and it decreased to 0.03858 in 2014 before increasing from 2015 onwards, except in 2020 (during the COVID-19 pandemic) when it decreased 1.2 percent. It means that regional income disparity tends to decline during the economic downturn caused by the COVID-19 pandemic, which could potentially end up in regional income convergence although some researchers like Martinho (Citation2021) believes otherwise, stating that COVID-19 has hindered income convergence in OECD countries. Nevertheless, the variance ratio test shows no statistical difference of standard deviation in regional income per capita during the period of observations, as indicated by the insignificant p-value in . It suggests no significant change in regional income distribution in East Java in 2010–2020; and that the coefficient variation of regional income dynamics from 2010 to 2020 is a short-run behavior.
In contrast, the coefficient of variation (CV) of real GDP per capita for the 37 regions shows an increasing trend in 2010–2019, with a declining coefficient of variation in 2020. In 2010, the CV was 0.03145, much lower than the coefficient of variation obtained from the analysis for the 38 regions. In 2019, the coefficient of variation reached 0.03261 before declining to 0.03226 in 2020. The statistical test also suggests no difference of standard deviation in regional income per capita during the observations. The visualization of σ-convergence could be seen in the figure below:
shows the CV trend across regions. Visually, we may see a general rising trend, which tentatively indicates the presence of σ-convergence, but this trend is statistically insignificant, as indicated by the variance ratio test in the last part. This may be due to the short period of the analysis. Future study, therefore, may benefit from covering more extended period of observation. Secondly, the convergence process is estimated through conventional β-convergence—the absolute or unconditional β-convergence; and the conditional β-convergence. The absolute β-convergence analysis employs a simple linear regression model, as shown in equation (3). Before running the formal regression, we inspect the relationship between regional income growth rate and initial GDP per capita in East Java. We visualized of the relationships in (A) 38 regions in 2010–2020; (B) 37 regions excluding City of Kediri in 2010–2020; (C) 38 regions in 2010–2019; and (D) 37 regions in 2010–2019. The duration analyses, i.e., 2010–2020 and 2010–2019, aimed to distinguish the negative shock effect caused by the COVID-19 pandemic on regional income growth in 2020.
Figure 1. Comparison of sigma convergence of the 38 regions (part a) and the 37 regions (part b) in East Java in 2010–2020

shows how the initial GDP per capita could be positively associated with income growth rate, even though the positive relationship in the figure (A) and (C) is relatively weak. Figure (B) and (D) indicate a stronger positive relationship, implying that wealthier regions in initial observations show a higher regional income growth rate than the poorer regions. In other words, there is a divergence tendency of regional income growth rate across regions in East Java. To formally check the presence of absolute β-convergence in the cross-sectional dataset, we run simple linear regressions, which is presented in equation (3) with the results as shown in the
:Table 3. Absolute beta convergence from cross-sectional Regressions
Overall, the regression results in model (A), (B), (C) and (D) indicate that the CV of GDP per capita in the base year is positive, but in model (A) and model (C) it is not statistically significant. It is only in model (B) and (D) that focus on the 37 regions, the values are statistically significant. The coefficient in the base year in the model (D), which excludes the period of COVID-19 is larger than model (B), which includes the period of the pandemic in the analysis. In general, the findings above suggest the absence of absolute β-convergence in East Java. Wealthier regions in East Java in 2010 showed higher average regional income growth rate than poorer regions—a tendency of income divergence. The larger coefficient of GDP per capita in the base year in model (D) compared to model (B) shows a severe regional income disparity in the 37 regions before the pandemic COVID-19 started in 2020. In a sense, the pandemic may have slowed down the divergence across the 37 regions. This finding is consistent with the σ-convergence analysis, which shows the declining CV of regional income per capita in 2020.
The following analysis investigates the conditional β-convergence by adding several explanatory variables in the convergence growth model as indicated by equation (4) to capture different steady-state income levels across regions. We split the regressions analysis into two: model (1) to model (5) covers the 38 regions in East Java, and model (6) to model (10) focus on the 37 regions by excluding the City of Kediri. Both models employ datasets from 2010 and 2019. The exclusion of 2020 is to avoid the pandemic effect on the economy. The results of the regression are shown in .
Table 4. Conditional beta convergence from cross-sectional regressions without spatial aspect
In general, the CV of GDP per capita in the base year is positive and statistically significant across models. It means that there is no sufficient evidence of conditional β-convergence. Income growth in regions in 2010 was faster in wealthier regions than in poorer regions, meaning income disparity was persistent. The result of conditional β-convergence analysis corroborates the findings of absolute or unconditional β-convergence. The variable of share of agriculture to GDP shows positive and statistically significant signs across models, which means that the agricultural sector is influential in modulating regional income growth rates. This finding is consistent with the prior work by Chiquiar (Citation2005), which found a positive effect of the agricultural sector on growth in Mexico in 1970–1985. In developing countries like Indonesia, the agriculture sector still has a significant proportion in the economy’s structure. According to the World Bank (Citation2020b), the agriculture sector accounted for roughly 4 percent of global GDP, and in many developing countries, the sector accounted for more than 25 percent of GDP. This sector plays a vital role in creating backward and upward linkages in the economy and in advancing regional income (Nugroho, Citation2016; World Bank, Citation2020b).
Human capital, proxied by average years of schooling, also shows statistical significance with a positive sign across all models, which is in line with previous studies by Chiquiar (Citation2005) in Mexico, stating the education boosts economic growth. Regarding the average years of schooling and GDP per capita, in the base year, there was a negative and statistically significant sign across models. It suggests that holding GDP per capita in the base year constant, poor regions with higher average years of schooling will grow faster and catch up with richer regions, leading to regional income convergence (Chiquiar, Citation2005).
There was no statistical evidence of the role of government expenditures on regional income growth across models. Expenditure on education, health and capital did not affect the regional income growth in East Java. We argue that this finding might be explained by the inefficient government expenditure on education, health, and capital. Drezgić (Citation2011) believes that public expenditure may have adverse effects or no effect on growth due to the lack of planning of infrastructure provisions, improper cost-benefit analysis of projects and corruption. According to Butkiewicz and Yanikkaya (Citation2011), institutional quality determines the impact of government expenditure on growth. Keefer and Knack (Citation2007) mentioned that good governmental institution might spend less on productive investment, but it yields a notable economic return. In contrast, poor government may invest a lot on public sector that is unproductive. For instance, government expenditure on capital might be “pork barrel” expenditure, i.e., building infrastructure or roads to nowhere. A good governmental institution will know how to avoid “pork barrel” expenditure, while a weak governmental institution might thrive on such spending (Butkiewicz & Yanikkaya, Citation2011).
4.2. Spatial dependence across regions in East Java
Spatial weight matrix was determined to formally test the occurrence of spatial dependence. Based on Moran I’s statistics, this study found that the spatial weight matrix of the five-nearest neighborhood was the fittest way to specify the spatial model. The rationale is described in . Moran I’s statistics measured the spatial distribution of every variable in the model. With this, we identified the weight matrices, which explain the spatial distribution of every variable. The various results for every weight matrix were presented in Appendix A. We found that 15 nearest neighbors can explain the spatial autocorrelation of government expenditure on education.
Table 5. Spatial weight matrix of 5-nearest neighbors
Meanwhile, agricultural sector size, average years of schooling and GDP per capita base year were explained by five nearest neighbors. The variable of GDP per capita at the base year could be explained by neighbors within 200 km. The variable of GDP per capita growth rate could be explained by neighbors within 125 km. However, the variable of government expenditure on capital and government expenditure on health had no spatial autocorrelation. This warrants further research on the impact of spatial dependence within the observed variables across East Java.
We corroborated the model’s spatial autocorrelation by conducting Lagrange Multiplier (LM) test to construct the appropriate spatial model. We used the closest five neighbors (K5) weight matrix because of its appropriateness in explaining most of the spatial autocorrelation among the independent variables. Based on the result of the LM test as shown in , we conclude that there is no clarity between the spatial lagged values and the error term in the model. Therefore, following the work of Halleck Vega and Elhorst (Citation2015), Llorca-Rodríguez et al. (Citation2021), and the consideration of the objective of this study to explore the spillover effect of government expenditure, we employ the spatial lag for X (SLX) model.
Table 6. LM Test for spatial model specification
The results of the SLX model estimation are shown in . In general, the results consistently show that the coefficient of Ln GDP Per capita base year is positive across models (full and reduced model). It suggests that the 38 regions in East Java experienced a tendency of regional income divergence. Nevertheless, the coefficient of Ln GDP per capita in the base year in both models is statistically insignificant. Moreover, by comparing the Akaike’s information criterion (AIC) and Bayesian information criterion (BIC) as well as the loglikelihood ratio test, we found that the full model was the most appropriate model to explain the regional income convergence in East Java between 2010 and 2019.
Table 7. Conditional beta convergence from cross-sectional regressions with spatial dependence
Overall, it can be concluded that both models of β-convergence with and without spatial dependence aspects indicate the same conclusion: 38 regions in East Java experienced regional income divergence in 2010–2019. This finding was consistent with the previous research conducted by Wardaya and Landiyanto (Citation2005) and Sugiharti (Citation2013), which argued that regions in East Java tended to grow towards wider income inequality. In addition, this finding was consistent with and support, albeit indirectly, the proposition of new economic geography (NEG) and endogenous growth theory. These two theories, in general, argue that locations play a crucial role in economic activities, which creates different levels of agglomeration and creates an unequal distribution of per capita income across regions. Wardaya and Landiyanto (Citation2005) specifically mentioned that the regional income divergence resulted from the unequal spatial distribution of the manufacturing industry sector across 38 regions in East Java. Some regions were leading in per capita income due to large shares of manufacturing sector, which also means higher productivity.
Furthermore, we found that the variable of average years of schooling and the interaction term of average years of schooling and GDP per capita base year were statistically significant. This finding strengthens the conclusions obtained from the conventional conditional β-convergence regression, as shown in . It means that human capital is essential for growth, and it promotes regional income convergence across regions. The share of agriculture, however, was statistically insignificant, opposing the previous result from the conditional β-convergence regression without the spatial aspects.
Similarly, the spatial models reveal that the government expenditure on education, health and capital statistically did not affect regional income growth in East Java. These findings are consistent with the previous results of conditional β-convergence without accounting for the spatial dependence in . It is also in line with prior research by N. Yildirim et al. (Citation2011) in Turkey and Al-Faris (Citation2002) in the context of Gulf Cooperation Council (GCC) countries. As previously discussed, there are several potential explanations behind the ineffective government expenditure in growth promotion. For instance, Chandra (Citation2010) argued that the return of investment may not be available in a short term. The impact of government expenditure on human capital may take time to impact the income growth since it is a long-run investment (Shafuda & De, Citation2020; Tayebi & Abbaslou, Citation2008). Thus, it is crucial for future research to employ a longer period of analysis.
Eggoh et al. (Citation2015) added several major related factors that might delay the return of investment: inefficient planning, corruption, and underinvestment. A significant proportion of government expenditure in productive sectors that are not supported with strong institutional quality and governance will not be fruitful (Jeyhoon Tabar et al., Citation2017; Shi et al., Citation2017). In the context of developing countries like Indonesia, efficiency may be commonplace; for example, Solihin et al. (Citation2017) found that government expenditure on the education sector in East Java from 2007–2014 was inefficient; hence, it yielded no significant outcomes. Another potential explanation is pervasive corruption. It prevents investment in strategic sectors such as education and health from reaching the targeted groups and programs. Currently, Indonesia’s corruption index ranked 102nd out of 180 countries in 2020 (KPK, Citation2020)), so it is not impossible that there was malpractice in the implementation.
The other factor is underinvestment. Eggoh et al. (Citation2015) argued that the impact of government spending on growth might emerge after passing a certain threshold of expenditure; otherwise, such effects might not be significant. In Indonesia’s case, the enactment of Law No.20/2003 and Law No.36/2009 have mandated the minimum allocation of 20 percent and 10 percent of general government expenditure for the education and health sector, respectively (Breuer et al., Citation2018). It suggests that underinvestment is not an issue in Indonesia, at least for the education and health sector. By contrast, public spending on capital in Indonesia is relatively low relative to its peers. For instance, the total infrastructure spending of Indonesia was 2.2 percent of GDP in 2016, while other emerging countries in Asia were 5.1 percent of GDP (Breuer et al., Citation2018). Thus, we argue that the insignificant effects of government expenditure of education, health, and capital on growth might be attributed to the problem of time lags, underinvestment (for capital or infrastructure), efficiency and potential corruption. In addition, World Bank (Citation2020c) and Breuer et al. (Citation2018) stated Indonesia needs to improve the efficiency in government expenditure since it has limited available financial resources due to a low share of revenue to GDP and tight adherence to fiscal deficit.
Interestingly, the spatial model also shows the existence of spillover effects of government expenditure on education and capital on growth. Particularly, the coefficient of the neighbor’s government expenditure on education is positive and statistically significant at the level of 10 percent. Meanwhile, the coefficient of the neighbor’s government expenditure on capital is negative and statistically significant at the level of 10 percent. These findings are similar to previous studies by Qi (Citation2016) in China, López et al. (Citation2017) in Spain, and Lall (Citation2007) in India. The positive sign of the neighbor’s government expenditure on education suggests that higher expenditure on education in one region could stimulate higher economic growth in its neighboring regions; and a negative sign of the neighbor’s expenditure on capital implies that higher expenditure on capital in one region could lower the economic growth in its neighboring regions.
There are some potential explanations behind the presence of this spatial spillover. Higher government expenditure on education in one region may produce a more educated labor force. Without sufficient job openings, this labor force will probably migrate to another region for better opportunities (McCormick & Wahba, Citation2005), which subsequently impacts its economic growth. It means that the interregional migration of educated labor forces might serve as one of the driving factors causing spatial spillover of government expenditure of the education sector.
Meanwhile, the negative spillover of public spending of capital on growth could be attributed to the shift of comparative and competitive advantages of neighboring’s economies (Lall & Yilmaz, Citation2001). Higher spending on capital that is reflected by more developed infrastructure in one region may attract companies or other production factors from other regions. Boarnet (Citation1998) claimed that regions with higher capital stock may draw factors of production from other regions. Additionally, higher spending on capital is often perceived as a good signal that the regional government is business friendly (Lall, Citation2007). Thus, the negative spillover of public spending on capital suggests the competition relationship across the 38 regions.
4.3. Policy Implication
The regions that do not progress well economically mostly rely on agriculture sector, which often has low productivity. Meanwhile, the progressing regions are more advanced in their manufacturing sector, which could be associated with higher productivity. The government could promote investment in agricultural-based industries in the former regions. The development of agricultural-based industries could improve productivity of the agriculture sector, leading to rising regional income. Second, the low economic growth effects from government expenditure on education, health, and capital calls for the improvement of government spending efficiency by establishing better governance, accountability, and corruption prevention. Third, spillover effects of government expenditure on growth call for better coordination in constructing regional development plans.
5. Conclusion
By employing the district/regional-level data of East Java—Indonesia, the current research has two main objectives: (1) to explore the regional income convergence and (2) to investigate the role of government expenditure on education, health, and capital sector on regional income convergence. This was observed by using σ-convergence, absolute β-convergence, and conditional β-convergence approaches. This study also uses SLX model to account for the spatial dependence across regions.
We find an increasing trend of CV of GDP per capita across the 38 regions in 2010–2019. This finding implies the absence of σ-convergence in East Java. Furthermore, the estimation of absolute β-convergence also shows that the coefficient of GDP per capita base year is positive, suggesting regional income divergence. Similarly, the estimations of traditional conditional β-convergence and spatial conditional β-convergence reveal the same conclusions: the richer regions grew faster than the poorer ones. Therefore, it is evident that the 38 regions in East Java experienced regional income divergence, which is in line with the prior studies by Wardaya and Landiyanto (Citation2005) and Sugiharti (Citation2013).
Second, the estimation of traditional conditional β-convergence and spatial conditional β-convergence show that government expenditure on the education, health and capital sectors does not affect growth. This finding is also similar to previous works by N. Yildirim et al. (Citation2011) and Al-Faris (Citation2002). Some tentative explanations behind this conclusion are inefficient public spending, time lags, potential corruption, and underinvestment.
Third, the result of the spatial conditional β-convergence analysis indicates the presence of spillover effects of government expenditure on education and capital sector on growth. Specifically, the public spending on education of one region could stimulate the higher output in its neighboring regions. Meanwhile, higher public spending on the capital in one region might reduce the output in its neighboring regions. Some prior studies by Qi (Citation2016) and Lall (Citation2007) also documented the spillover effects of public spending on growth.
Based on the findings above, the government might narrow the regional income gaps in East Java by promoting and facilitating new investment in agricultural-based industries in lagging regions. The new investment in agricultural-based industries is expected to create the “catch-up” effect for poorer regions. Moreover, the government also needs to improve public spending efficiency by applying better governance and accountability. Better coordination in establishing regional development planning is also essential to mitigate the adverse effects of government expenditures triggered by the spillover effects.
Lastly, it is worth noting that the scope of the analysis in this study is limited, i.e., only East Java province. Further research may extend the analysis by exploring broader areas in Indonesia. Additionally, the time duration of the analysis in this study is relatively short for regional convergence analysis (only 10 years). Future research may extend the time duration of the analysis.
Disclosure statement
No potential conflict of interest was reported by the author(s).
Additional information
Funding
Notes on contributors
Achmad Solihin
Dr.Achmad Solihin is a senior lecturer at the Department of Economics – Universitas Airlangga. His research interests are public economics and finance.
Wahyu Wisnu Wardana
Wahyu Wisnu Wardana is a lecturer at the Department of Economics – Universitas Airlangga and Research Associate at Research Institute of Socio-Economic Development (RISED) - Surabaya. He works at the intersection of development and regional economics.
Erfan Fiddin
Erfan Fiddin is Head of Loan and Grant Planning and Allocation Section of Directorate General Financing and Risk Management – Ministry of Finance of Indonesia. His research interests are regional economics and spatial econometrics.
Ni Made Sukartini
Dr.Ni Made Sukartini is a senior lecturer at the Department of Economics – Universitas Airlangga. Her research interests are public economics and health economics.
Notes
1. In this study, we refer to regional income as economic growth.
2. The analysis of σ-convergence and traditional absolute β-convergence (without considering the spatial aspect) employed GRDP per capita data throughout 2010–2020. Due to the adverse impact of pandemic COVID-19 on regional growth in 2020, the conditional β-convergence analysis utilized the datasets spanning from 2010–2019 only.
References
- Akram, V., & Rath, B. N. (2020). Does export diversification lead to income convergence? Evidence from cross-country analysis. Buletin Ekonomi Moneter Dan Perbankan, 23(3), 319–24. https://doi.org/10.21098/bemp.v23i3.1251
- Al-Faris, A. F. (2002). Public expenditure and economic growth in the Gulf Cooperation Council countries. Applied Economics, 34(9), 1187–1193. https://doi.org/10.1080/00036840110090206
- Alm, J., Aten, R. H., & Bahl, R. (2001). Can Indonesia decentralise successfully? Plans, problems and prospects. Bulletin of Indonesian Economic Studies, 37(1), 83–102. https://doi.org/10.1080/000749101300046537
- Amusa, K., & Oyinlola, M. A. (2019). The effectiveness of government expenditure on economic growth in Botswana. African Journal of Economic and Management Studies, 10(3), 368–384. https://doi.org/10.1108/AJEMS-03-2018-0081
- Anselin, L. (2010). Spatial econometrics: Methods and models. Kluwer Academic.
- Baddeley, M. (2006). Convergence or divergence? The impacts of globalisation on growth and inequality in less developed countries. International Review of Applied Economics, 20(3), 391–410. https://doi.org/10.1080/02692170600736250
- Barro, R. J. (1990). Journal of political economy. Government Spending in a Simple Model of Endogeneous Growth, 98(5, Part 2), S103–S125. https://www.journals.uchicago.edu/doi/pdf/ 10.1086/261726
- Barro, R. J., & Sala-i-Martin, X. (2006). Economic growth. The MIT Press. & Massachusetts Institute of Technology.
- Boarnet, M. G. (1998). Spillovers and the locational effects of public infrastructure. Journal of Regional Science, 38(3), 381–400. https://doi.org/10.1111/0022-4146.00099
- Brakman, S., Garretsen, H., & Schramm, M. (2006). Putting new economic geography to the test: Free-ness of trade and agglomeration in the EU regions. Regional Science and Urban Economics, 36(5), 613–635. https://doi.org/10.1016/j.regsciurbeco.2006.06.004
- Breuer, M. L. E., Guajardo, M. J., & Kinda, M. T. (2018). Realizing Indonesia’s economic potential. International Monetary Fund.
- Butkiewicz, J. L., & Yanikkaya, H. (2011). Institutions and the impact of government spending on growth. Journal of Applied Economics, 14(2), 319–341. https://doi.org/10.1016/S1514-0326(11)60017-2
- Capello, R. (2016). Regional economics (2nd ed. ed.). Routledge.
- Chandra, A. (2010). Does government expenditure on education promote economic growth? An econometric analysis. University Library of Munich.
- Chiquiar, D. (2005). Why Mexico’s regional income convergence broke down. Journal of Development Economics, 77(1), 257–275. https://doi.org/10.1016/j.jdeveco.2004.03.009
- Choi, C. (2004). Foreign direct investment and income convergence. Applied Economics, 36(10), 1045–1049. https://doi.org/10.1080/0003684042000246759
- Dey, S. P., & Neogi, D. (2015). Testing sigma and unconditional beta convergence of GDP for SAARC countries: Can inclusion of China further consolidate the convergence? Global Business Review, 16(5), 845–855. https://doi.org/10.1177/0972150915591643
- Dobrinsky, R., & Havlik, P. (2014). Economic convergence and structural change: The role of transition and EU accession(wiiw Research Report, No. 395). Vienna: The Vienna Institute for International Economic Studies (wiiw).
- Drezgić, S. (2011). Public investments and regional income convergence: Empirical analysis of Croatian regions. Social Research, 3(24), 43–55.
- Dsikowitzky, L., Damar, A., Ferse, S. C. A., Irianto, H. E., Jennerjahn, T. C., Lukas, M. C., Nordhaus, I., Pohlmann, T., Schwarzbauer, J., Sugama, K., & Sumiono, B. (2019). Chapter 21: Java Island, Indonesia. In C. Sheppard (Ed.), World seas: An environmental evaluation. The Indian Ocean to the Pacific, 2 (pp. 459–490). London, UK: Academic Press. https://doi.org/10.1016/B978-0-08-100853-9.00029-4
- Dudzeviciute, G., Šimelyte, A., & Liucvaitiene, A. (2018). Government expenditure and economic growth in the European Union countries. International Journal of Social Economics, 45(2), 372–386. https://doi.org/10.1108/IJSE-12-2016-0365
- Eggoh, J., Houeninvo, H., & Sossou, G. A. (2015). Education, health and economic growth in African countries. Journal of Economic Development, 40(1), 93. https://doi.org/10.35866/caujed.2015.40.1.004
- Fallah, B. N., Partridge, M. D., & Olfert, M. R. (2011). New economic geography and US metropolitan wage inequality. Journal of Economic Geography, 11(5), 865–895. https://doi.org/10.1093/jeg/lbq016
- Feldkircher, M. (2006). New Regional Economics in Central European Economies: The future of centrope. In Workshop organized by eurosystem (No. 9).
- Fingleton, B., & Fischer, M. M. (2010). Neoclassical theory versus new economic geography: Competing explanations of cross-regional variation in economic development. The Annals of Regional Science, 44(3), 467–491. https://doi.org/10.1007/s00168-008-0278-z
- Fischer, M. M., & Stirböck, C. (2004). Regional income convergence in the Enlarged Europe, 1995-2000: A spatial econometricperspective (ZEW-Centre for European Economic Research Discussion Paper (04-042)).
- Florax, R. J., Folmer, H., & Rey, S. J. (2003). Specification searches in spatial econometrics: The relevance of Hendry’s methodology. Regional Science and Urban Economics, 33(5), 557–579. https://doi.org/10.1016/S0166-0462(03)00002-4
- Gardiner, B., Martin, R., & Tyler, P. (2004). Head. Regional Studies, 38(9), 1045–1067. https://doi.org/10.1080/0034340042000292638
- Gezici, F., & Hewings, G. J. (2004). Spatial analysis of regional inequalities in Turkey. European Planning Studies, 15(3), 383–403. https://doi.org/10.1080/09654310601017091
- Goli, S., & Arokiasamy, P. (2014). Maternal and child mortality indicators across 187 countries of the world: Converging or diverging. Global Public Health, 9(3), 342–360. https://doi.org/10.1080/17441692.2014.890237
- Gömleksiz, M., Şahbaz, A., & Mercan, B. (2017). Regional economic convergence in Turkey: Does the Government really matter for? Economies, 5(3), 27. https://doi.org/10.3390/economies5030027
- Halleck Vega, S., & Elhorst, J. P. (2015). The SLX Model. Journal of Regional Science, 55(3), 339–363. https://doi.org/10.1111/jors.12188
- Hansson, P., & Henrekson, M. (1994). A new framework for testing the effect of government spending on growth and productivity. Public Choice, 81(3–4), 381–401. https://doi.org/10.1007/BF01053239
- Hill, H., Resosudarmo, B. P., & Vidyattama*, Y. (2008). Indonesia’s changing economic geography. Bulletin of Indonesian Economic Studies, 44(3), 407–435. https://doi.org/10.1080/00074910802395344
- Irawan, A. (2014). Regional income disparities in Indonesia: measurements, convergence process, and decentralization (Doctoral dissertation, University of Illinois at Urbana-Champaign).
- Janssen, F., van den Hende, A., de Beer, J., & van Wissen, L. J. (2016). Sigma and beta convergence in regional mortality: A case study of the Netherlands. Demographic Research, 35, 81–116. https://doi.org/10.4054/DemRes.2016.35.4
- Jeyhoon Tabar, F., Najafi, Z., & Sistani Badooei, Y. (2017). The impact of educational expenditures of government on economic growth of Iran. AD-minister, (30), 217–235. https://doi.org/10.17230/ad-minister.30.11
- Källström, J. (2012). An empirical study of regional convergence, inequality, and spatial dependence in the enlarged. European Union.
- Kangasharju, A. (1998). Beta convergence in Finland: Regional differences in speed of convergence. Applied Economics, 30(5), 679–687. https://doi.org/10.1080/000368498325660
- Keefer, P., & Knack, S. (2007). Boondoggles, rent-seeking, and political checks and balances: Public investment under unaccountable governments. The Review of Economics and Statistics, 89(3), 566–572. https://doi.org/10.1162/rest.89.3.566
- Komarulzaman, A., & Alisjahbana, A. (2006). Testing the natural resource curse hypothesis in Indonesia: Evidence at the regional level (No. 200602). Department of Economics, Padjadjaran University.
- KPK (The Corruption Eradication Commission of Indonesia). (2020). Corruption Perception Index (CPI) 2020, Skor Indonesia Menurun. Accessed from https://www.kpk.go.id/id/berita/berita-kpk/2040-corruption-perception-index-cpi-2020-skor-indonesia-menurun
- Lall, S. V. (2007). Infrastructure and regional growth, growth dynamics and policy relevance for India. The Annals of Regional Science, 41(3), 581–599. https://doi.org/10.1007/s00168-006-0112-4
- Lall, S. V., & Yilmaz, S. (2001). Regional economic convergence: Do policy instruments make a difference? The Annals of Regional Science, 35(1), 153–166. https://doi.org/10.1007/s001680000035
- LeSage, J., & Pace, R. K. (2009). Introduction to spatial econometrics. Chapman and Hall/CRC.
- Lim, U. (2016). Regional income club convergence in US BEA economic areas: A spatial switching regression approach. The Annals of Regional Science, 56(1), 273–294. https://doi.org/10.1007/s00168-015-0739-0
- Llorca-Rodríguez, C. M., Chica-Olmo, J., & Casas-Jurado, A. C. (2021). The effects of tourism on EU regional cohesion: A comparative spatial cross-regressive assessment of economic growth and convergence by level of development. Journal of Sustainable Tourism, 29(8), 1319–1343. https://doi.org/10.1080/09669582.2020.1835930
- López, F. A., Martínez-Ortiz, P. J., & Cegarra-Navarro, J. G. (2017). Spatial spillovers in public expenditure on a municipal level in Spain. The Annals of Regional Science, 58(1), 39–65. https://doi.org/10.1007/s00168-016-0780-7
- López-Calva, L. F., Ortiz-Juarez, E., & Rodríguez-Castelán, C. (2021). Within-country poverty convergence: Evidence from Mexico. Empirical Economics, 1–40.
- Magalhães, A., Hewings, G., & Azzoni, C. R. (2000). Spatial dependence and regional convergence in Brazil (Mimeograph). University of Illinois at Urbana-Champaign.
- Martinho, D. V. J. P. (2021). Impact of Covid‐19 on the convergence of GDP per capita in OECD countries. Regional Science Policy & Practice,1–18. https://doi.org/10.1111/rsp3.12435
- McCormick, B., & Wahba, J. (2005). Why do the young and educated in LDCs concentrate in large cities? Evidence from migration data. Economica, 72(285), 39–67. https://doi.org/10.1111/j.0013-0427.2005.00401.x
- Nguyen-Hoang, P., & McPeak, J. (2010). Leaving or staying: Inter-provincial migration in Vietnam. Asian and Pacific Migration Journal, 19(4), 473–500. https://doi.org/10.1177/011719681001900402
- Niebuhr, A. (2000). Convergence and the effects of spatial interaction (No. 110). HWWA Discussion Paper.
- Nugroho, R. Y. Y. (2016). Linkages, potential and spatial efficiency of paddy production in East Java. JDE (Journal of Developing Economies), 1(1), 29–43. http://dx.doi.org/10.20473/jde.v1i1.1737
- Ojede, A., Atems, B., Yamarik, S., Atems, B., & Yamarik, S. (2018). The direct and indirect (spillover) effects of productive government spending on state economic growth. Growth and Change, 49(1), 122–141. https://doi.org/10.1111/grow.12231
- Paas, T., Kuusk, A., Schlitte, F., & Võrk, A. (2007). Econometric analysis of income convergence in selected EU countries and their nuts 3 level regions. SSRN Electronic Journal. https://doi.org/10.2139/ssrn.1078863
- Pons, J., Paluzie, E., Silvestre, J., & Tirado, D. A. (2007). Testing the new economic geography: Migrations and industrial agglomerations in Spain. Journal of Regional Science, 47(2), 289–313. https://doi.org/10.1111/j.1467-9787.2007.00510.x
- Postiglione, P., Cartone, A., & Panzera, D. (2020). Economic convergence in EU NUTS 3 regions: A spatial econometric perspective. Sustainability, 12(17), 6717. https://doi.org/10.3390/su12176717
- Puga, D. (2002). European regional policies in light of recent location theories. Journal of Economic Geography, 2(4), 373–406. https://doi.org/10.1093/jeg/2.4.373
- Purwono, R., Wardana, W. W., Haryanto, T., & Khoerul Mubin, M. (2021). Poverty dynamics in Indonesia: Empirical evidence from three main approaches. World Development Perspectives, 23(C).
- Qi, J. (2016). Government education expenditure, third-party spillover effect and economic growth in China. Journal of Sustainable Development, 9(2), 145–152. https://doi.org/10.5539/jsd.v9n2p145
- Rapacki, R., & Próchniak, M. (2009). Real beta and sigma convergence in 27 transition countries, 1990–2005. Post-Communist Economies, 21(3), 307–326. https://doi.org/10.1080/14631370903090616
- Ravallion, M. (2012). Why don’t we see poverty convergence? American Economic Review, 102(1), 504–523. https://doi.org/10.1257/aer.102.1.504
- Resosudarmo, B. P., & Vidyattama, Y. (2006). Regional income disparity in Indonesia: A panel data analysis. In ASEAN Economic Bulletin (pp. 31–44).
- Rey, S. J. (2004). Spatial dependence in the evolution of regional income distributions. In Spatial econometrics and spatial statistics (pp. 194–213).
- Rey, S. J., & Montouri, B. D. (1999). US regional income convergence: A spatial econometric perspective. Regional Studies, 33(2), 143–156. https://doi.org/10.1080/00343409950122945
- Sarracino, F., & Mikucka, M. (2017). Social capital in Europe from 1990 to 2012: Trends and convergence. Social Indicators Research, 131(1), 407–432. https://doi.org/10.1007/s11205-016-1255-z
- Seya, H., Tsutsumi, M., Yoshida, Y., & Kawaguchi, Y. (2011). Empirical comparison of the various spatial prediction models: in spatial econometrics, spatial statistics, and semiparametric statistics. Procedia - Social and Behavioral Sciences, 21, 120–129. https://doi.org/10.1016/j.sbspro.2011.07.025
- Shafuda, C. P., & De, U. K. (2020). Government expenditure on human capital and growth in Namibia: A time series analysis. Journal of Economic Structures, 9(1), 1–14. https://doi.org/10.1186/s40008-020-00196-3
- Shankar, R., & Shah, A. (2003). Bridging the economic divide within countries: A scorecard on the performance of regional policies in reducing regional income disparities. World Development, 31(8), 1421–1441. https://doi.org/10.1016/S0305-750X(03)00098-6
- Shi, Y., Guo, S., & Sun, P. (2017). The role of infrastructure in China’s regional economic growth. Journal of Asian Economics, 49(C), 26–41. https://doi.org/10.1016/j.asieco.2017.02.004
- Solihin, A., Mursinto, D., & Sugiharti, L. (2017). Efficiency and effectiveness of government expenditure on education at districts/cities level in East Java Indonesia. Asian Social Science, 13(8), 91. https://doi.org/10.5539/ass.v13n8p91
- Soukiazis, E., & Castro, V. (2005). How the maastricht criteria and the stability and growth pact affected real convergence in the European Union: A panel data analysis. Journal of Policy Modeling, 27(3), 385–399. https://doi.org/10.1016/j.jpolmod.2005.01.002
- Statistics Indonesia. (2018d). Distribusi PDRB Atas Dasar Harga Konstan/Distribution of GRDP at Constant Price 2010. https://kedirikota.bps.go.id/statictable/2019/07/31/306/distribusi-persentase-pdrb-atas-dasar-harga-konstan-2010-menurut-lapangan-usaha-2010-2018-persen-.html
- Statistics Indonesia. (2020a). Gross regional domestic product of provinces in Indonesia by Industry 2015-2019.
- Statistics Indonesia. (2020b). Laju Pertumbuhan Produk Domestik Regional Bruto Atas Dasar Harga Konstan 2010 Menurut Provinsi (Persen). https://www.bps.go.id/indicator/52/291/1/-seri-2010-laju-pertumbuhan-produk-domestik-regional-bruto-atas-dasar-harga-konstan-2010-menurut-provinsi.html
- Statistics Indonesia. (2020c). Gini ratio by province 2002-2020. https://www.bps.go.id/linkTableDinamis/view/id/1116
- Sugiharti, L. (2013). A Regional Income Convergence Process in East Java (Indonesia): Do spatial dependence and spatial regimes matter? International Proceedings of Economics Development and Research, 76(17), 97–107. https://doi.org/10.7763/ipedr
- Tayebi, S. K., & Abbaslou, Y. (2008). Higher education, real income and real investment in the selected OIC members: evidence from ARDL and panel data approaches. Iranian Economic Review, 13(22), 69–87. https://doi.org/10.22059/IER.2008.32666
- Tian, X., Zhang, X., Zhou, Y., & Yu, X. (2016). Regional income inequality in China revisited: A perspective from club convergence. Economic Modelling, 100(56), 50–58. https://doi.org/10.1016/j.econmod.2016.02.028
- Tobler, W. R. (1970). A computer movie simulating urban growth in the Detroit region. Economic Geography, 46(sup1), 234–240. https://doi.org/10.2307/143141
- Wardaya, W., & Landiyanto, E. A. (2005). Club convergence & regional spillovers in East Java. Parallel Session VB: Regional Economic Development, 17.
- Wooldridge, J. M. (2015). Introductory econometrics: A modern approach. Cengage learning.
- World Bank. (2020a). GDP Growth (Annual %) - Indonesia. https://data.worldbank.org/indicator/NY.GDP.MKTP.KD.ZG?locations=ID
- World Bank. (2020b). Overview of Agriculture and Food. Accessed from: https://www.worldbank.org/en/topic/agriculture/overview#1
- World Bank. (2020c). Indonesia public expenditure review – spending for better results.
- Wu, S. Y., Tang, J. H., & Lin, E. S. (2010). The impact of government expenditure on economic growth: How sensitive to the level of development? Journal of Policy Modeling, 32(6), 804–817. https://doi.org/10.1016/j.jpolmod.2010.05.011
- Yildirim, J., Öcal, N., & Özyildirim, S. (2009). Income inequality and economic convergence in Turkey: A spatial effect analysis. International Regional Science Review, 32(2), 221–254. https://doi.org/10.1177/0160017608331250
- Yildirim, N., Deniz, H., & Hepsag, A. (2011). Do public education expenditures really lead to economic growth? Evidence from Turkey. International Research Journal of Finance and Economics, (65), 13–24.
- Young, A. T., Higgins, M. J., & Levy, D. (2008). Sigma convergence versus beta convergence: Evidence from US county‐level data. Journal of Money, Credit and Banking, 40(5), 1083–1093. https://doi.org/10.1111/j.1538-4616.2008.00148.x