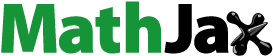
Abstract
In Kenya, very little research has been carried out on the topic of the informal sector. This paper estimates the size of the Kenyan informal sector for the period 1970–2018. In light of our analysis of the stationary properties of the data, which suggests a mixture of I(0) and I(1) variables, this study specifies an Autoregressive Distributed Lag Model (ARDL), which is applied to variables that pose such attributes. In addition, the model is good for small sample size observation, a characteristic that is common among developing countries. Our estimates indicate that this sector is quite large and has grown over time to about 32% of the country’s GDP or nearly one-third of the size of the recorded GDP. These results are consistent with the stylized fact about the Kenyan economy, in particular the large number of individuals employed in small businesses and trading as well as the number of tax returns filed on an annual basis versus the stated level of employment. The finding of a significant informal sector also has implications for the conduct of fiscal policy. The sector should not be wound abruptly since it provides employment to many Kenyans. The fiscal policy should aim at reducing the tax burden so that the participants in the informal sector gradually formalize their businesses.
PUBLIC INTEREST STATEMENT
The informal sector is conspicuous in Kenya. The majority of the employment opportunities are in the informal sector. Although the sector boosts the economy due to the provision of job opportunities, the government fails to get tax revenue from it. This is due to the fact that the sector is hard to tax since transactions occur in cash form. This study, therefore, uses currency demand approach to estimate the monetary value of all the goods and services produced in the sector. The currency demand approach is appropriate given that economic transactions in the sector occur in cash form. The study establishes that the sector accounts for about 32% of Kenya’s GDP. This finding is important to Kenya Revenue Authority because it shows how big the sector is, so that appropriate action is taken to reduce it. Moving some of the participants in the sector to the formal sector will lead to an increase in tax revenue collection in Kenya.
1. Introduction
The informal sector is a type of economy that applies to all income classes and all sectors of the economy. It is comprised of various types of economic activities, spanning from the self-employed such as second-hand clothes dealers, hawkers, dressmakers, wage workers, housekeepers, security guards, carpenters to the registered businesses, for example, law firms, private hospitals, private schools that underestimate their incomes and overestimate their expenditure (Greenidge et al., Citation2009; Ngui et al., Citation2014). However, there have always been problems in attempting to estimate its size. According to Dellas et al. (Citation2017), informal sector data are not included in the national statistics and thus may lead to inaccurate macroeconomic indicators. Not taking into consideration the economic activities in the informal sector can lead to the level of recorded GDP and other important data being underreported. Such data may present an inaccurate impression of an economy. Since recorded GDP is important for international ranking, inaccurate data presents a wrong impression of an economy. Further, Greenidge et al. (Citation2009) asserted that trend estimates might also be affected if the economic activities excluded in the recorded GDP grow at diverse rates from those included. For example, it is often shown that informal sector activities grow at the same time activities in the official economy are shrinking. Therefore, relying on data that does not capture both sectors of the economy may lead to erroneous policy decisions.
This paper estimates the size of Kenya’s informal sector using the ARDL model. This model provides a useful link between long-run equilibrium relationships and short-run disequilibrium dynamics. To the best of our knowledge, this is the first time such an approach has been used to estimate the size of the informal sector in Kenya. This procedure was chosen since the stationarity results revealed a mixture of variables that were integrated of order zero I (0) and one I (1). It is also argued that this model is good for procedure in dealing with small data samples (Pesaran & Shin, Citation1995).
The next section presents the main causes, advantages and disadvantages of the informal sector and an overview of the informal sector in Kenya. Section 3 deals with some of the studies that were conducted on the topic and gives a review of their results. In Section 4, the size of the informal sector in Kenya is estimated using the currency demand approach. The paper concludes with some remarks and policy implications.
2. Causes, advantages and disadvantages of the informal sector
The increase in the size of the informal sector has become a concern among many governments in both developed and developing countries. This growing size of the informal sector is linked to many reasons, for instance, rising tax burdens, poor governance and high levels of corruption in the public sector (Greenidge, Citation2009). A high tax burden widens the gap between effort of the worker and his pay. This, therefore, acts as an incentive for the worker to resort to the informal sector. This therefore implies that a tax regime is a major determinant of the size of the informal sector (Schneider & Enste, Citation2002). Dharmapala, Slemrod & Wilson (Citation2011) showed that a low tax burden could move firms from the informal sector to the formal sector among the developing countries.
This informal sector is essential in the provision of employment among developing countries. The sector provides a conducive environment for entrepreneurs and those who may want to innovate. The sector also gives individuals who lose jobs in the formal sector an opportunity to generate income for their welfare. The sector has also emerged as an alternative source of employment to a growing number of individuals moving from educational institutions. In addition, the proceeds obtained from this sector are spent in the formal sector. Lastly, the sector competes with the formal sectorthus leading to reduced prices and improvement in the quality of the goods and services produced in the formal sector (Muchiri, Citation2014).
Though the informal sector is a source of employment among developing countries, it is not suitable for the economic prosperity of an economy due to tax evasion. Failure by producers and households to declare their economic activities reduce tax revenue raised in an economy (Dellas et al., Citation2017). To bridge the gap in revenue collection, tax authorities usually resort to imposing higher taxes in the formal sector. Such action reinforces the size of the unreported incomes as the taxpayers feel overtaxed and turn to the informal sector, thus exacerbating the situation (Gunes, Starzec & Gardes, Citation2013). This means that tapping tax from the informal sector may be important in sustaining tax morale and tax compliance among larger firms (Joshi, Prichard & Heady, Citation2012). In addition, the informal sector presents a challenge to policymakers by distorting economic information since it overstates key ratios, for instance, unemployment rates and debt-to GDP. The implication of these shortcomings means that policies made in countries with high size of the informal sector may be erroneous, thus causing adverse reactions. The sector also competes unfairly with the official economy, thus reducing the official economy’s income. Lastly, the sector may lead to increased corruption as the participants seek to continue evading taxes (Greenidge, Citation2009).
3. Overview of the informal sector in Kenya
In Kenya, the informal sector started to flourish in the 1970s with the boom in coffee smuggling along the Kenya-Uganda border in search of the market by Ugandans following the imposition of a trade embargo on Ugandan coffee by the USA. Currently, a significant proportion of the informal sector includes activities that are unrecorded and profitable enterprises. This includes self-employed such as second-hand clothes dealers, hawkers, dressmakers, wage workers, housekeepers, security guards and carpenters (Masinde & Makau, Citation2010). According to the Institute of Economic Affairs (IEA)—Kenya (Citation2012), most of these activities are highly profitable and revenue obtained from them exceeds the minimum statutory taxable income of Kshs. 11,135 per month at the same time employing thousands of Kenyans majority who do not keep proper records and do not report their incomes to relevant authorities. Despite the high tax potential, KRA’s effort to reduce the size of the informal sector, for instance, reintroduction of turnover tax in 2019, has been unsuccessful (Republic of Kenya, Citation2019). This inability to bring more individuals into the tax net is attributed to the high administrative costs involved.
In Kenya, the informal sector employs approximately 77% of the total workforce. Of the workforce in this sector, 60% are youth between the age of 18 and 35 years. Of this, 50% are women (Republic of Kenya, Citation2017). The growth of this sector can be linked to liberalization and privatization policies that led to reduced job opportunities in the formal public sector. The formal private sector could not absorb the increasing number of job seekers. Therefore, the informal industry emerged as an alternative source of employment to a growing number of individuals moving from educational institutions. Thus, the informal sector should be supported due to its role in the Kenyan economy (Muchiri, Citation2014). However, it is argued that economic agents choose to operate in the informal sector to evade taxes. Increased tax revenue mobilized from this expansive and untapped tax base would significantly contribute to the country’s GDP, thus alleviating poverty and consequently improving the living standards of the citizen. However, the sector poses tax administrative and compliance challenges. It is argued that the wealthy individuals in Kenya are gradually shifting their wealth to this sector by purchasing motorcycles and hiring youth to ferry passengers at a commission (Wawire, Citation2020). Though the industry is suitable for creating job opportunities, its expansion can lead to low tax revenues, thus negatively impacting the provision of public goods by the government of Kenya (Peters, Citation2017). shows the trends in the size of the informal sector in Kenya.
Figure 1. Trends of size of informal sector (1970–2018). Source of the data: Republic of Kenya; Various Issues of Economic Survey

The trends in corroborate the assertion that liberalization and privatization policies as enshrined in the Structural Adjusted Programmes (SAPs). From this figure, it can be observed that from 1970 to 1988, the number of individuals employed in the informal sector was low. A drastic increase in the number of people employed in the sector is observed from 1989 onwards. This coincided with 1988 and especially 1991 when the Kenyan government embraced these economic and social reforms initiated by World Bank and International Monetary Fund (IMF) commonly referred to as SAPs. The number of people employed in the informal sector adopted a positive trajectory to 2018. This can be linked to an increasing number of individuals exiting tertiary institutions and universities who the formal sector cannot absorb fully, thus turning to the informal sector.
4. The statement of the problem
The extent of the informal sector is a cause of concern in Kenya. The activities of the informal sector have several implications for the country’s sustainable economic management. These activities have implications for the tax revenue and determining what the optimal tax should be. In addition, the informal sector is characterized by unrecorded activities which falsify national accounts, thus rendering policies derived from such statistics inefficient. Generally, the subject of estimating the size of the informal sector has not been widely studied. The few studies that have investigated the size of the informal sector include Bajada and Schneider (Citation2018), Peters (Citation2017), and Greenidge et al. (Citation2009). Locally, very little information is known about the size of the informal sector. Ngui et al. (Citation2014) attempted to investigate the sector, but the study focused mainly on the determinants of the informal sector. It is on this basis that this study sought to estimate the size of the size informal sector in Kenya so as to inform policy to be taken.
5. Empirical literature review
Ahmed and Hussain (Citation2008), while estimating the black economy in Pakistan, revealed that the size of the informal sector in Pakistan was less than 5% of the formal GDP for every year for the period of study running from 1960 to 2003. Greenidge et al. (Citation2009) estimated the size of the informal sector in Barbados. The study established that the informal sector accounted for more than one-third of the country’s GDP in Barbados. In another study, Phiri and Kabaso (Citation2012) studied taxation of the informal sector in Zambia for the period between 1973 and 2010. The study results revealed that the Zambian informal sector accounted for about 42% of the GDP. In another study, Hassan and Schneider (Citation2016) modelled the Egyptian shadow economy for the period running from 1976 to 2013 using the currency demand approach. The study’s findings revealed that the informal sector accounted for about 20% of GDP between 1990 and 2013. The study showed that though there had been a declining trend in the size of the informal sector, the level was still high for Egypt as a developing country and was a stumbling block for economic development. Though the currency model has been widely used in the estimation of size of informal sector, a direct approach such as a survey based on a questionnaire may give a clear pointer to the informal sector.
Another methodology for estimating the size of the informal sector is the Multiple Indicators Multiple Causes (MIMIC). This model was used by Dell’Anno (Citation2007) to estimate Portugal’s size of the shadow economy for the period between 1997 and 2004. The causes of the informal sector considered included the ratio of government employment to the total labour force, ratio of subsidies to GDP, tax burden, ratio of social benefits to GDP, ratio of self-employment to the labour force and unemployment. The indicators considered include real GDP, labour force participation. The study results showed that the informal sector in Portugal decreased from about 30% of the country’s GDP in 1978 to about 18% of the country’s GDP in 2004. Hassan and Schneider (Citation2016) modelled the Egyptian shadow economy by adopting the MIMIC approach for the period between 1976 and 2013. This study adopted a structural equation model in modelling effect of various causes, namely self-employment, share of agricultural sector in GDP and institutional quality on shadow economy. In structural equation model, the shadow economy was represented by the following indicators: real GDP, currency outside banks, and total employment. The study findings revealed that the Egyptian shadow economy was declining over the study period. The size of this economy averaged 30% of GDP for 37 years. Though the structural equation model (SEM) is preferred as an alternative to the currency demand model, it requires a large data size which may not be available among the developing countries.
In another study, Castro and Aranda (Citation2018) estimated the size of the informal sector among OECD members and Latin American countries between 1995 and 2016 using the MIMIC approach. The results on estimation of the size informal sector showed that informal sector in the Latin America countries was 34% of GDP while that of OECD was 19% of GDP. The study attributed the differences in the size of the informal sector between the two regions to differences in quality of institutions and level of economic prosperity. Among causes of the informal sector considered by the study included tax burden, corruption, government integrity and unemployment. The indicators for the informal sector considered in the study had GDP growth, labour freedom and government expenditure on education. This study finding showed that the size of the informal sector is larger among developing countries than in developed countries. This finding motivated the need to estimate the size of the informal sector in Kenya, a developing country but in a different continent.
6. Methodology
6.1. Theoretical framework
Hibbs and Piculescu (Citation2013) theoretical framework informed the model chosen in estimating the size of the informal sector in Kenya. It is postulated that the prevailing tax rate determines the number of output firms decide to produce in the informal sector. The relationship between tax rate and output in an economy is shown in .
Figure 2. The relationship between tax rate and output in an economy.Source: Hibbs and Piculescu (Citation2013)

Patterns of production options as tax rates oscillate around a threshold tax rate t* are illustrated in . From the figure, total output (Q total) represents output produced by formal and informal sectors. The figure region where t < t* illustrates a situation where all production takes place in the formal sector since total output in an economy (Q total) is equal to formal sector output/GDP (Q formal sector). This means the informal sector’s output is equal to zero. As the tax rate t increases beyond t*, firms start to find activities in the informal sector profitable. It can be observed that as the tax rate is beyond t*, production in the formal sector begins to decline and reaches zero (0) when the tax rate reaches one (1). The stage between t* and t = 1 is comprised of the informal production sector (Q formal sector) and informal sector (Q informal sector) simultaneously. From , it is seen that once the tax rate reaches one (1), all economic activities occur in the informal sector; that is, total output in an economy (Q total) is equal to the informal sector output/GDP (Q informal sector). This means the informal output sector is equal to zero implying that the government obtains zero tax revenue.
The same idea can be linked to currency demand. The Keynesian theory states that individuals hold money due to three motives: transaction, precautionary, and speculative (Blankard, Citation2017). According to Hendriks and Myles (Citation2013), most of transactions in the informal sector are in cash. Currency outside the bank can be used to measure output in the informal sector. From , it’s observed that tax rate is the primary determinant of the output in the informal sector and, therefore, must be incorporated in the model. An estimate of currency outside the bank is first obtained when the tax rate is included in the model to estimate output in the informal sector. This can be denoted by . Secondly, an estimate of currency outside the bank is again obtained when the model assumes the tax rate to be zero. This can be denoted by
. From , we expect currency outside the bank to be high when there is a tax rate in the model than when it is assumed to be zero. The difference between
and
gives currency circulating in the informal sector (
), as shown in EquationEquation 1
(1)
(1) .
Assuming that the velocity of money circulating in the informal sector ( is similar to that of the formal sector, an estimate of informal sector output (
) is obtained by getting the product of currency circulating in the informal sector (
and velocity of money (
) as shown in EquationEquation 2
(2)
(2)
To estimate the effect of the size of the informal sector on tax revenue, tax evasion expected utility theory was considered. The theory shows that the choice to evade taxes is made by weighing the gains and losses involved. The theory envisages a preference for a dominant alternative that produces greater utility is picked ones with less utility. This theory makes assumption of decision-makers being aware of multiple outcomes, and can determine a prevailing view (Sebora & Cornwall, Citation1995). Thus, the individuals who play a part in the informal sector act rationally. Their primary interest is maximizing the expected benefit from economic activities they do. For a rational individual who evades tax, the income understated will maximize their net anticipated revenue. This evader compares net gain expected from tax evasion with what would be obtained by doing legal activities informal sector.
6.2. Empirical model
In estimating the size of the informal sector, the currency demand method, as suggested by Tanzi (Citation1983), was adopted. The approach involves specifying currency equations that can infer the effect of tax on demand for currency. The fundamental assumptions made is that high taxes act as an incentive for individuals to shift their economic activities to the informal sector and that currency is the main form of carrying out day to day transactions or used as a store of wealth. In the equation, the natural logarithm of currency outside banks denoted as is dependent variable while tax ratio,
, is independent variable. The control variables are per capita GDP, deposit rate of interest, inflation, and financial innovation denoted as
,
,
and
, respectively. The currency demand function is shown in EquationEquation 3
(3)
(3) .
where ln, subscript and
are natural logarithms, time components, and error terms, respectively.
,
,
,
,
and
are coefficients to be estimated. One (1) is added to independent variable tax to estimate when the tax ratio is assumed to be minimum that is zero. The natural logarithm of the independent variable is then obtained. This is taking cognizant that the natural logarithm of one is zero.
Once EquationEquation 3(3)
(3) has been estimated for 1970–2018, currency demanded can be obtained, assuming that the tax variable assumes a value of zero. The difference between the currencies in the two models gives the currency that circulates in the informal sector. As discussed in the analytical framework section, the informal sector’s size can be estimated by getting the product of excess currency and velocity of money.
6.3. Description of the variables, their measurement and the expected signs
Variables, descriptions, measurements, and a priori expected signs are discussed in .
Table 1. The variables definition, measurement and priori expected signs
6.4. Data source and type
Time series data over the 1970 to 2018 period was used to carry out estimation in the study. This period was preferred due to consistently recorded data on variables. In addition, the observations are enough to handle time-series tests and methods. Data on currency outside banks, tax revenue, per capita GDP, inflation, the interest rate on bank deposits and financial innovation were obtained from KNBS Economic Surveys and abstracts of various years. Data on agriculture share in GDP, manufacturing output, foreign aid were obtained from WDI database. Data on informal sector’s GDP was computed in this study.
6.5. Pre-estimation tests
To avoid cases of spurious regression, the study performed unit root tests. Appropriate methods for testing the presence of unit roots in the data series is the Augmented Dickey Fuller (ADF) test, the Phillips-Peron (PP)-test and Zivot-Andrews (Z-A) test. PP test is the most used alternative to ADF since it is non-parametric. It does not require model specification and lagged parameter in the test regression. However, PP may not be the best test because it is based on asymptotic theory. In other words, PP test is designed to test the unit root in the long time series, which is not possible in reality. Zivot and Andrews (Citation1992) and Pesaran and Pesaran (Citation1997) showed that both ADF and PP tests are asymptotically equivalent. These authors further asserted that both tests have lower power, and invalid null hypothesis is not rejected in the situation where the coefficient in AR (1) process is close to one. Further, these conventional unit root tests do not account for structural breaks in the data. The unit root that overcomes all these drawbacks is the Z-A unit root test. The nature of aggregate time-series data requires that unit root tests be conducted before any regression is carried out. If data is non-stationary, this will lead to spurious or inconsistent regression results. The unit roots tests were performed using the ADF test, the PP test and Z-A test, where the null hypothesis is that the series tested contains at least a unit root. Having established the nature of the stationarity of the variables, the study investigated presence of long-run relationships using the ARDL bounds test (Enders, Citation2015).
7. Results
7.1. The descriptive statistics
The study established measures of central location and spread of the data shown in model 2.4. The study examined the minimum and maximum values as a way of checking for the presence of outliers. The results are shown in .
Table 2. Descriptive statistics
From , it was observed that 49 observations were considered in the study. It was also revealed that the natural log of the currency outside banks has the highest standard deviation implying that the observations are relatively not close to each other. The natural log of tax ratio has the least standard deviation implying that the observed annual observations are not far from each other. The results showed that the minimum natural log of tax ratio was 0.011, while the highest was 0.24. The results also showed that the natural log of per capita GDP deviates from its mean, 9.51 by 1.52.
7.2. The unit root results
The study, therefore, investigated the presence of unit root using ADF, PP and Z-A tests. Results for the first two tests of stationarity are shown in .
Table 3. The augmented Dickey Fuller and Phillips-Perron unit root tests
The results showed that both ADF and PP unit root presented a mix of stationary at level and stationary after differencing once. However, these stationarity tests could not be entirely relied on, especially in the incidences of structural breaks. The variables were thus subjected to a more superior stationarity test, the Zivot-Andrew’s stationarity test. The Z-A unit root tests are shown in .
Table 4. Zivot Andrews unit root test
The Z-A test results corroborate ADF and PP test by presenting variables that integrated different orders where some were stationary while others were not stationary at a level. The Z-A test revealed the presence of structural breaks. According to Murunga (Citation2014), the established structural breaks of most macroeconomic variables in Kenya coincide with identifiable poor and erratic climatic conditions, trade liberalization, economic and political shocks. Having established the presence of both stationary and non-stationary variables at the level, the ARDL framework was adopted. To check for long-run relationship, the ARDL Bounds test was adopted and results are presented in the following section.
7.3. Cointegration results
Based on estimates, ARDL bounds test (cointegration) results in showed a long-run relationship for both models with tax and that with zero tax. This was suggested by the F test being greater than Pesaran, Shin, & Smith (Citation2001) 1(1) bound critical values, thus rejecting H0 of no long-run relationship. Having established the presence of a long-run relationship, the study proceeded to estimate both short-run ARDL and ECM, as discussed in the following sections.
Table 5. ARDL bounds test (cointegration test)
The Short Run Results
shows short-run estimates of ARDL model.
Table 6. Short-run estimates
The short-run results revealed that both models performed well in terms of coefficient of determination. The coefficient of determination indicated that the explanatory variables explained over 90% variation in the dependent variable. In addition, the t values for the model with tax was revealed to be valid as suggested by Breusch-Godfrey results that suggested an absence of autocorrelation. However, the Breusch-Godfrey results for the model without tax proposed the presence of autocorrelation, but the choice of the ARDL model, which introduces lags of both dependent and explanatory variables, act as a remedy to the econometric model.
The results revealed that on average, a 1% increase in the first lag of the natural logarithm of currency outside bank leads to a rise in the natural logarithm of the currency outside the bank by 0.494% and 0.572% when all other factors are held constant for a model with tax and that with minimum tax respectively. Secondly, the results revealed that 1% rise in natural logarithm of tax ratio leads to a rise in the natural log of currency outside banks by 1.307% on average ceteris paribus. In addition, it was revealed that an increase in the natural log of per capita GDP by 1% leads to a rise in the natural log of currency outside bank by 0.627% and 0.558% for a model with tax and with minimum tax, respectively. Further, results showed that increasing financial innovation by a unit leads to a decrease in natural log of currency outside the bank by 21.9% on average, holding all other factors constant for the model with tax. The study revealed that the coefficients of first lag of the natural log of currency outside a bank, natural log of per capita GDP, natural log of tax ratio and financial innovation were significant at a 1% level of significance. This implied these variables were significant determinants of the natural log of currency outside a bank in Kenya in the short run.
7.5. The ECM results
shows the long run results.
Table 7. Long run estimates
The results showed that the error correction term was negative and significant, thus confirming a long-run relationship between explanatory variables and dependent variable for both models with tax and minimum tax. On the one hand, coefficients of regressors were significant for the model with tax. The coefficients of per capita GDP, inflation, tax ratio and financial innovation agreed with the economic theory for the model with tax. For this model, the coefficient of inflation and deposit interest rate did not conform to economic theory. On the other hand, all explanatory variables were found to be essential determinants of the natural log of currency outside banks for the model with minimum tax. For this model, coefficients of natural log of per capita GDP and inflation were in line with economic theory, while interest rate and financial innovation did not conform to economic theory.
Specifically, the results revealed that currency outside banks by 1.12% and 1.28% on average held other factors constant for the model with tax and minimum tax, respectively. The results also revealed that, on average, the natural log of currency outside banks increases by 5.26% if the natural log of tax ratio increases by 1%, holding other factors constant. Furthermore, the results revealed a unit rise in financial innovation leads to 83% decrease in natural log of currency held outside banks ceteris paribus for the model with tax.
Using these coefficients, the study obtained predicted values for currency outside banks for each model. The expected value for currency outside banks with minimum tax was subtracted from the predicted value for currency outside banks with tax. This difference gives the currency that circulated in the informal sector. Assuming velocity of money was same for both formal and informal sectors, the currency in informal sector was multiplied by velocity of money to obtain informal sector’s output. The estimates of informal sector output are presented in .
From , it is evident that the informal sector has been increasing over time. In 1970 the informal sector was KES 2.35 billion out of the GDP of KES 10.33 billion. In 2018, the informal sector accounted for about KES 1.7 trillion out of the GDP of about KES 7 trillion. From the above figure, the informal sector’s size declined from 75% of country’s GDP in 1991 % of GDP to 11% in 2000. This drop can be attributed to tax reforms that had been put in place to strengthen tax administration and combat tax evasion. Some of these reforms include the establishment of KRA as a way of centralizing tax collection. Initially, businesses could get involved in tax evasion due to bureaucracy that was involved in paying taxes since many departments within the Ministry of Finance were involved. In addition, the PIN was introduced in 1997 to make it easy to trace transactions made by individuals or businesses, thus reducing the magnitude of tax evasion.
On average, Kenya’s informal sector accounts for about 32% of GDP. These results are consistent with Medina et al. (Citation2017) findings that showed that the Kenyan informal sector lies between 20% and 40% of GDP while using the MIMIC method.
8. Policy implications
The empirical results in this study present direct implications for tax reform in Kenya. The results paint that the informal sector command a substantial part of the Kenyan economy. Therefore, it may not be advisable to do away with the informal sector completely because it provides a source of employment to the majority of Kenyans. The best policy should aim at removing high tax burden that act as an incentive for individuals to resort to the informal sector. The adoption of lower tax burden can gradually integrate the informal sector into the formal sector.
Disclosure statement
No potential conflict of interest was reported by the author(s).
Additional information
Funding
Notes on contributors
James Murunga
The main author is James Murunga. He is a PhD candidate pursuing a degree that will lead to a degree in Doctor of Philosophy in Economics of the University of Nairobi. His PhD thesis is in form of essays and the article he submitted is the first essay of the PhD thesis. The other two authors are his thesis supervisors. Dr. Moses Kinyanjui Muriithi is a senior lecturer in the Department of Economics, Population and Development Studies of the University of Nairobi. Nelson Were Wawire, is a professor of Economics in the School of Economics of Kenyatta University. James Murunga is the one who wrote the paper andanalysed the data. The role of the other two authors was to assist in shaping the research idea based on their vast experience in the area of public economics.
Reference
- Ahmed, Q. M., & Hussain, M. H. (2008). Estimating the black economy through a monetary approach: A case study of Pakistan. Economic Issues, 13(1), 45–15. https://ideas.repec.org/a/eis/articl/108ahmed.html
- Bajada, C., & Schneider, F. (2018). An international comparison of underground economic activity. In size, causes and consequences of the underground economy. Routledge.
- Blankard, O. (2017). Macroeconomics. Pearson Education Limited.
- Castro, G. R. B., & Aranda, D. A. (2018). The informal economy and its impact on tax revenues and economic growth, analysis of OECD members and Latin America Countries (1995–2016). Pontificia Universidad Católica del Perú.
- Dell’Anno, R. (2007). The shadow economy in Portugal: An analysis with the MIMIC approach. Journal of Applied Economics, 10(2), 253–277. https://doi.org/10.1080/15140326.2007.12040490
- Dellas, H., Malliaropulos, D., Papageorgiou, D., & Vourvachaki, E. (2017). Fiscal policy with an informal sector. CEPR Discussion Paper.
- Dharmapala, D., Slemrod, J., & Wilson, J. D. (2011). Tax policy and the missing middle: Optimal tax remittance with firm-level administrative costs. Journal of Public Economics, 95(9–10), 1036–1047
- Enders, W. (2015). Applied econometric time series. John Wiley & Sons.
- Faal, E. (2003). Currency demand, the underground economy and tax evasion: The case of Guyana. International Monetary Fund.
- Greenidge, K., Holder, C., & Mayers, S. (2009). Estimating the size of the informal economy in Barbados. Journal of Business, Finance & Economics in Emerging Economies, 4(1), 1–33. https://cert-net.com/files/publications/journal/2009_1_4/197_226.pdf
- Gunes, A. T. A., Starzec, C., & Gardes, F. (2013). A new estimation of the size of informal economy using monetary and full expenditures in a complete demand system. Paris: Sorbonne
- Hassan, M., & Schneider, F. (2016). Modelling the Egyptian shadow economy: A currency demand and a MIMIC model approach. Journal of Economics and Political Economy, 3(2), 309–339. doiI:10.1453/jepe.v3i2.788
- Hendriks, J., & Myles, G. D. (2013). Intermediate public economics (2nd ed.). MIT Press.
- Hibbs, D. A., & Piculescu, V. (2013). Tax toleration and tax compliance. Governance, 3(2), 87–99. https://doi.org/10.1111/j.1540-5907.2009.00415.x
- Institute of Economic Affairs (IEA) – Kenya. (2012). Informal sector and taxation in Kenya.
- Joshi, A., Prichard, W., & Heady, C. (2012). Taxing the informal economy: Challenges, possibilities and remaining questions. Brighton: Institute of Development Studies
- Masinde, M. M., & Makau, P. (2010). Unlocking The Revenue Potential in Kenya. Parliamentary Service Commission Policy Working Paper No. 2/2010. Nairobi: PSC.
- Medina, L., Jonelis, M. A. W., & Cangul, M. (2017). The informal economy in Sub-Saharan Africa: Size and determinants. International Monetary Fund.
- Muchiri, B. K. (2014). An analysis of the effect of the growth of the informal sector on tax revenue performance in Kenya [ Unpublished MA project], University of Nairobi.
- Murunga, J. (2014). An Empirical Investigation of Factors Influencing Tax Effort in Kenya. Unpublished master degree thesis, University of Nairobi, Kenya
- Ngui, D. M., Muniu.J, M., & Wawire, N. H. W. (2014, March 27–28). Determinants of informal sector performance in the rural areas of Kenya: Evidence from Makueni county. International Conference on Dynamics of Rural Transformation in Emerging Economies. Nairobi: Kenyattta University.
- Pesaran, M. H., & Pesaran, B. (1997). Interactive econometric analysis. Oxford University Press.
- Pesaran, M. H., & Shin, Y. (1995). An autoregressive distributed lag modelling approach to cointegration analysis
- Pesaran, M.H., Shin, Y., & Smith, R.J. (2001). Bounds Testing Approaches to the Analysis of level relationships. Journal of Applied Econometrics, 16, 289–326
- Republic of Kenya (2019). Communication Authority of Kenya First Quarter Sector Statistics Report for the Financial Year 2018/2019. Nairobi: Government Printer.