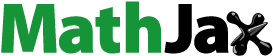
Abstract
Previous research has explored the relationship between carbon emissions, trade openness, and foreign direct investment (FDI), but these studies have not specifically examined carbon emissions using sector-level data. This paper expands upon the existing body of literature by employing a threshold regression approach, utilizing the intensity of carbon emissions as a primary variable to scrutinize the effects of FDI and trade openness on carbon emissions at a sectoral level. Our findings indicate that the impact is contingent upon the chosen thresholds, thereby underscoring the influence of foreign trade openness and FDI on carbon emissions within the industrial sector. The effect of FDI on sector-specific industrial carbon emissions is not constant, with the influence coefficient varying over time. In contrast, trade openness positively and negatively impacts carbon emissions. Specifically, increased foreign trade openness leads to a decrease in carbon emissions in less carbon-intensive sectors. Factors such as the intensity of economic activity, employment levels, independent technical innovation, and per capita GDP significantly influence carbon emissions within industrial sectors.
Reviewing editor:
1. Introduction
The escalating annual increase in carbon dioxide emissions significantly contributes to global warming. This phenomenon poses a serious threat to numerous biological species and the well-being of human populations. In 2018, the United Nations issued a stern warning, emphasizing the urgency of immediate actions to limit climate change to a manageable level of 1.5 degrees Celsius. Without such measures in place by 2040, the global community faces severe consequences. Hence, the worldwide focus has shifted towards controlling carbon emissions and averting further environmental degradation. Notably, China currently holds the position of the world's largest trading nation. As per the China Statistical Yearbook, in 2018, the utilized foreign direct investment was valued at 885.6 billion yuan, while the total value of export and import of goods reached 30.51 trillion yuan. The substantial foreign trade and FDI growth have greatly bolstered China's economic expansion. However, their relationship to carbon emissions has piqued academic curiosity. Most existing research has explored the association between foreign trade or FDI and carbon emissions, underscoring their significant interrelation (Metulini et al., Citation2017). These studies, nonetheless, do not adequately address the connection between carbon emissions, foreign trade openness, and FDI.
Furthermore, there is insufficient focus on carbon emissions from China's industrial sectors. Also, many studies presuppose a linear relationship between FDI, foreign trade, and carbon emissions, overlooking the possibility of variability across sectors with different carbon emission levels. Lastly, divergent views on carbon emissions, foreign trade openness, and FDI largely stem from discrepancies in sample selection and research methodologies.
In line with prior research, this study proposes several novel contributions. Firstly, it explores the relationship between carbon emissions, foreign trade openness, and FDI in China's industrial sector. Secondly, we utilize panel data from specific domestic industries selected from the “China Input-Output Table” and other pertinent data sets. After categorizing all industrial sectors into twenty classifications, we examine the impact of foreign trade and FDI on those most directly associated with carbon emissions. Thirdly, we apply a threshold regression approach to evaluate their influence on varying magnitudes of carbon emissions. A fixed-effects approach was used to investigate the impact of foreign trade openness and FDI on carbon emissions, using panel data from China's industrial sector from 2011 to 2021.
The remainder of the article is structured as follows. The literature review is discussed in the second portion and is presented there. The framework, sources of data, and variables employed in this research are discussed in the third portion. The research findings and analyses are presented in the fourth portion. The summary and results of the study are presented in the final portion.
2. Literature review
The review of available literature led us to identify three major topics of study: foreign trade openness, foreign direct investment, and carbon emissions. Firstly, previous literature investigates the effects of foreign direct investment (FDI) on environmental quality, focusing on the concept of a “pollution haven." Secondly, past literature examines the influence of foreign trade on carbon emissions. Lastly, the previous studies explore the relationship between foreign trade and foreign direct investment.
A substantial amount of research has been conducted on the effects of FDI on the environment in China, presenting three primary perspectives on FDI's impact on carbon emissions. One theory posits that FDI directly creates a “pollution haven.” Differences in environmental policies across nations have given rise to this concept. Developed countries often have stricter environmental regulations than emerging nations, which might not have any or less stringent environmental legislation. Consequently, as developed countries relocate their polluting industries to emerging nations, carbon emissions increase. Pao and Tsai (Citation2011) examined the effects of financial growth and economic development on environmental degradation from 1980 to 2007 using a panel cointegration approach. Their findings support the “pollution haven” hypothesis. Ren et al. (Citation2014) explored the impact of per capita income, imports, exports, foreign trade openness, and FDI on carbon dioxide emissions in China, employing industry panel data and the generalized two-step method of moments (GMM) evaluation technique. Their findings suggested that large FDI inflows exacerbate China's carbon dioxide emissions.
An alternative perspective, known as the “pollution halo” theory, suggests that the influx of FDI brings advanced technology and management methods that could potentially improve the environment. Gohar et al. (Citation2023a) conducted a study using data from the Chinese provincial panel covering 2000 to 2017, employing a panel-corrected standard error (PCSE) approach. They found that FDI adversely affected industrial CO2 emissions at the national level. This negative correlation remained consistent when regional variations were considered for the eastern, central, and western regions. In addition, their study revealed that internal R&D initiatives significantly increased carbon dioxide emissions at both the regional and national levels.
The third perspective considers the interplay between the “pollution haven” and “pollution halo” effects concerning economic growth. The “pollution haven” hypothesis proposes that an increase in FDI could initially cause greater pollution during the early stages of economic growth. Conversely, the “pollution halo” argument contends that as economic growth progresses, FDI indirectly reduces environmental pollution through technological advancements.
The primary focus of studies investigating the impact of foreign trade on carbon emissions aims to ascertain how this effect varies across different regions and categories of carbon emissions. Grossman and Krueger proposed three categorization themes: scale effect, composition effect, and technical effect. These categories have been frequently utilized in empirical studies examining trade and environment relationships. Yan and Yang (Citation2010) estimated the carbon dioxide emissions attributable to China's foreign trade from 1997 to 2007. They found that scale and composition effects amplified carbon dioxide emissions, while the technical effect partially offset this increase. Trade activities, including imports and exports, can also be classified into trade deficit and surplus categories. Using the input-output analysis method, Ren et al. (Citation2014) estimated carbon dioxide emissions in China's foreign trade from 2000 to 2010, highlighting the steady expansion of China's trade surplus as a significant factor in the rapid increase in carbon dioxide emissions.
Bashir, Sadiq, et al. (Citation2022a) analyzed how energy transition and environmental innovation bring environmental sustainability. Bashir, Sadiq, et al. (Citation2022a) examined how industrial and economic indicators contribute to GHG and carbon emissions. Moreover, Lei et al. (Citation2022) analyzed the nexus between board characteristics and firms' environmental performance. Similarly, Bashir (Citation2022) analyzed how the pollution haven hypothesis has evolved. However, these studies provide mixed findings. Chang et al. (Citation2022a, Citation2022b) and Derindag et al. (Citation2022) or Behera and Dash (Citation2017) focus on different geographical areas or nations, while others employ distinct research techniques like the panel quantile regression technique used by Zhu et al. (Citation2016) and the VEC (vector error correction) method used by Derindag et al. (Citation2023a, Citation2023b) and Gong et al. (Citation2023). To address these issues, this study uses panel data from twenty industrial sectors in China, employing a threshold regression approach to examine the interplay between FDI, foreign trade openness, and carbon emissions.
Foreign trade can be divided into two categories: exports and imports. Sulaman et al. (Citation2020) examined the impact of trade along the “Belt and Road” global initiative on carbon dioxide emissions from 2000 to 2016, covering sixty-five countries. They sought to determine the effect of exports on carbon emissions in high-income and low-income countries. They found that exports led to an increase in carbon emissions in low-income countries but a decrease in high-income countries. Additionally, they found that imports increased carbon emissions in low- and middle-income countries and decreased in high- and middle-income countries.
Haug and Ucal (Citation2019) explored how international trade affected carbon dioxide emissions in a regional study of Turkey. His research indicated that while an increase in exports did not have a statistically significant effect, a decrease in exports led to a reduction in per capita carbon dioxide emissions. Conversely, a decrease in imports had no long-term impact, whereas an increase in imports correlated with an increase in per capita carbon dioxide emissions. Further studies by Chen et al. (Citation2022) and Chen et al. (Citation2018) analyzed the relationship between foreign trade and China's per-capita carbon dioxide (CO2) emissions from 1980 to 2014, revealing a negative correlation. Balsalobre‐Lorente et al. (Citation2022) and Shahbaz et al.'s (Citation2019) examination of the environmental Kuznets curve also considered the impacts of scale, technology, composition, and trade openness on carbon emissions in the US. Their findings demonstrated that increased trade openness contributed to reducing carbon dioxide emissions.
Moreover, the main focus of studies on the relationship between international trade and foreign direct investment (FDI) has been the examination of substitution and complementarity. A complementary relationship between foreign trade and FDI is observed when the cost of conducting foreign trade is high. Conversely, José (Citation2007) suggested that an alternative relationship between FDI and international trade occurs when the cost of foreign trade is low. Li and Wen (Citation2013) revealed that FDI positively influences international transactions. The extant literature, however, fails to draw definitive conclusions about the overall relationship, given that international trade and FDI occur across numerous nations and sectors. While Chang et al. (Citation2020a, Citation2020b, Citation2020c) suggest that FDI plays a significant role in promoting trade between the European Union and Central and Eastern European nations, the impact of FDI may vary based on its country of origin. Chang (Citation2020), Ali et al. (Citation2022), Chang et al. (Citation2023a, Citation2023b) found a two-way causal relationship between Singapore's FDI and the total service trade and service imports. However, only marginal support was found for a similar two-way causal relationship in Malaysia. Similar studies have also explored the link between carbon emission reductions, environmental mitigation, economic complexity, and global inequality (Jiang et al., Citation2022; Rafei et al., Citation2022; Sinha et al., Citation2022).
Limited research has been conducted on the association between foreign trade openness, foreign direct investment, and industrial carbon dioxide emissions in China. It is essential to determine whether a connection exists between these elements and, if so, identify its impact's nature. This study applies a threshold panel regression approach to examine the effects of FDI and trade openness on China's carbon emissions. This approach uses panel data from China's industrial sector from 2011 to 2021.
3. Variable description, data, & methodology
3.1. Data source & sample selection
The study sample used in this work includes data from 20 industry sectors spanning 11 years (2011–2021). To guarantee the compatibility and consistency of the data, a categorization approach according to standard practices, like the “China Input-Output Table,” is used. The industrial sector of China is divided into twenty industries, which primarily include wood processing and furniture, instrumentation and cultural office machinery manufacturing, papermaking, printing, cultural and educational sports goods manufacturing, transportation equipment manufacturing, general-purpose and special equipment manufacturing, food manufacturing and tobacco processing industry, manufacturing communication equipment, computer, and other electronic equipment manufacturing, chemical industry, metal products industry, oil and gas extraction, non-metallic mines and other mining and dressing industries, electrical machinery and equipment manufacturing, Metal mining industry, petroleum processing, coking, and nuclear fuel processing industry, electricity and heat production and supply, textile, clothing, shoes, hat, leather, and down and its products manufacturing, coal mining and washing industry, crafts and other manufacturing (waste and scrap), metal smelting and rolling processing industry, and denotes non-metallic mineral products industry.
Data on R&D expenditure was sourced from the “China Science and Technology Statistical Yearbook” (2011–2021), while the “China Science Statistical Yearbook” (2011–2021) provided data on industrial research and development. The “China Energy Statistical Yearbook” (2011–2021) offered information on energy consumption, and the “China Environmental Statistics Yearbook” (2011–2021) delivered data on industrial pollution outputs. Data on foreign direct investment, total exports and imports, and the gross domestic product were obtained from the “China Statistical Yearbook” (2011–2021). Finally, the “China Industrial Economic Statistical Yearbook” (2011–2021) was consulted for the sector's total production value and workforce statistics.
3.2. Explanation of variables ()
Table 1. Variables description.
3.2.1. The explained variable (threshold variable)
The industry k's carbon emission intensity in year t is indicated by the symbol The intensity level is determined by dividing the overall industrial carbon emissions by the industry value of production. The total industrial carbon emissions are calculated by multiplying the industry's energy consumption by the emission coefficient.
3.2.2. Explanatory variables
FDIC, t represents the foreign direct investment in industry k in year The total production worth of companies with foreign funding is selected as a gauge for assessing the effect of foreign direct investment on industry carbon emissions due to the availability of data at a more specific industry level and potential convergence problems in econometric analysis (Li and Liu, Citation2012). The favorable projected value for the predicted coefficient of foreign direct investment's impact on carbon emissions would indicate that growing levels of foreign direct investment in China's industrial sector will raise the country's carbon emissions. It is consistent with the idea that focused investment often causes carbon emissions to increase in emerging nations while decreasing them in advanced countries. In contrast, if the predicted coefficient of foreign direct investment is negative, it means it has been drawn to China's industrial sector to lower carbon emissions. This result would be consistent with the theory of technology spillover influences, according to which foreign direct investment boosts the technical and energy efficiency of the host nation and, as a result, reduces carbon emissions in emerging economies.
During a certain period, t, reflects the degree of trade openness in industry k. It identifies the link between China's economic openness and economic development by comparing its overall imports and exports to the gross domestic product (GDP) ratio to the degree of dependency on international trade openness. The fact that exports are frequently mentioned as the main cause of environmental issues is noteworthy (Dogan et al., Citation2019). The industry's overall export and import worth ratio to its total production value in China was chosen to assess its openness to international trade. If the calculated trade openness coefficient is favorable, it follows that opening up China's industrial sector to overseas commerce would raise carbon emission contamination. If the predicted coefficient is unfavorable, on the other hand, freeing up China's industrial sector to international commerce would help reduce carbon emissions.
The real per capita GDP index for industry k in year t is represented by The industry's carbon dioxide emissions and the extent of its economic growth are strongly related. According to Gohar et al. (Citation2023b, Citation2022a, Citation2022b), Hashmi and Chang (Citation2021) and Hashmi et al. (Citation2021a), the per capita gross domestic product index evaluates how financial growth affects carbon emissions. According to the Environmental Kuznets Curve (EKC) hypothesis, economic expansion reduces environmental degradation after achieving a specific point. It suggests a conflict between environmental pollution and economic growth. A negative U-shaped association between total economic development and carbon emissions was found by Dogan and Inglesi in 2020 (Dogan & Inglesi-Lotz, Citation2020). Nevertheless, the EKC theory's presumptions have not been proven true when the industrial sector makes up a substantial portion of a nation's economic structure, and there is a U-shaped link between carbon emissions and manufacturing financial development.
3.2.3. Control variables
TECk, t, represents the autonomous technological innovation index for business k in year t The development of low-carbon technologies in the host nation comes from inside and outside sources in an open economy. In contrast to outside sources, which discuss worldwide technologies, insider sources discuss independent technical innovation. The capacity of local businesses to incorporate and utilize imported technology is just as important for developing the host nation's productivity and ecologically friendly technology as independent technical innovation and the acquisition of foreign technology. Agreements for the transfer of technological patents, commerce, and cross-border investment are all included in the definition of technology import, with the latter being the most common route. The relationship between TEC and foreign direct investment determines how well local businesses can adopt new technology brought about by FDI. The imported technology emphasizes the importance of foreign corporations in encouraging independent innovation inside domestic businesses and driving reductions in emissions, indicating that domestic companies ought to have a special capacity for learning to gain advantages from foreign technology spillovers. The data on invention patent authorizations, indicating an elevated level of technical innovation, is used as the data for independent technological innovation, drawing motivation from Foss and Laursen (Citation2005).
is the total number of workers in sector k in year t. When creating rules for the business's employment and ecological constraints, authorities should consider this indication. The workforce of a sector significantly influences carbon emissions.
Industry k's research and development activity in year t is represented by the number which significantly impacts the industrial sector's adoption of technology for reducing carbon emissions. The research and development (R&D) activity magnitude is often assessed by looking at the cash allocated to research and development (R&D) operations (Ren et al., Citation2014). The ratio of industry investment in research and development to total production is used to gauge research and development intensity to allay issues with endogeneity and collinearity in regression calculations.
Throughout year t, industry k experienced as the intensity of its economic activity. The intensity of economic activity or ACT indicates the degree of manufacturing activity inside the industry. There is a correlation between rising carbon emissions and higher economic activity levels. Power use may be used as a stand-in for measuring economic activity intensity.
3.3. Variable’s descriptive statistics
Before proceeding with the methodology and empirical research, we performed descriptive statistics on the carbon emissions data from China's industrial sector. The results of these statistics for all variables are displayed in .
Table 2. Variables descriptive statistics.
presents intriguing data: The carbon emission intensity for the twenty industries ranges from a minimum of 1.459 to a maximum of 6.601, with a standard deviation of 2.391. It highlights substantial variations in carbon emissions among the industries, as shown by the nearly 5.142 spread between the highest and lowest figures. Comparable disparities are observed in foreign trade and foreign direct investment (FDI) sectors. The range between the highest and lowest figures for foreign trade is 1002.491, while for foreign direct investment, it is 9.374. These variations underscore the necessity to classify industries based on their carbon emissions volume when assessing the effects of foreign trade openness and direct investment on China's industrial carbon emissions.
presents intriguing data: The carbon emission intensity for the twenty industries ranges from a minimum of 1.459 to a maximum of 6.601, with a standard deviation of 2.391. It highlights substantial variations in carbon emissions among the industries, as shown by the nearly 5.142 spread between the highest and lowest figures. Comparable disparities are observed in foreign trade and foreign direct investment (FDI) sectors. The range between the highest and lowest figures for foreign trade is 1002.491, while for foreign direct investment, it is 9.374. These variations underscore the necessity to classify industries based on their carbon emissions volume when assessing the effects of foreign trade openness and direct investment on China's industrial carbon emissions.
We selected and ordered in ascending order the carbon emission intensity statistics from twenty industries from 2010. delineates four separate intervals—(0.2174–0.5782), (0.5782–1.3411), (1.3411–2.3525), and (2.3525–2.4630)—to illustrate how the intensity of carbon emissions varies significantly across different sectors. The impact of foreign trade openness and foreign direct investment (FDI) on carbon emissions may display a threshold effect at different levels of carbon emission intensity.
Figure 1. In 2010, the twenty industries' carbon emissions intensity.
Note: In , NMPI denotes the non-metallic mineral products industry, MSARPI is used for metal smelting and rolling processing industry, NMAOMADI stands for non-metallic mines and other mining and dressing industry, CMAWI for coal mining and washing industry, CI stands for the chemical industry, EAHPAS stands for energy and heat production and supply, PP stands for petroleum processing, and CANFPI is used for coking and nuclear fuel processing industry, MMI stands for metal mining industry, P,P,C,E,SGM denoted papermaking, printing, culture, education, and sports goods manufacturing, CAOM is used to denote crafts and other manufacturing (waste and scrap), OAGE stands for oil and gas extraction, MPI denoted Metal products industry, T, C, S, H, L, and DAP stand for textile, clothes, shoes, hats, leather, down, and by-products, WPAFTM is used to indicate Wood processing, and furniture manufacturing, FMATPI stands for Food Manufacturing and Tobacco Processing Industry, GASEM stands for general and special equipment manufacturing, TEM stands for transportation equipment manufacturing, EMAEM is used to show denotes electrical machinery and equipment manufacturing, IACOMM is used to show instrumentation and cultural office machinery manufacturing CE, CAOEEM denotes communication equipment, computer and other electronic equipment manufacturing.

3.4. Construction of approach
Given the substantial differences in carbon emissions across various sectors, we divided the Chinese industrial sector into several study areas. We used the carbon emission decomposition variables included in the STIRPAT approach, established by Gohar et al. (Citation2022c, Citation2022d), as the foundation for our empirical approach to investigate the effects of carbon emissions.
(I)
(I)
In our research, the primary variables are carbon emissions, foreign trade openness, and foreign direct investment (FDI). Nonetheless, it is crucial to note that other variables, such as independent technological advancement and per capita production, can further influence carbon emissions, FDI, and trade. To represent the relevant business environment, we incorporate control variables, including the number of industry personnel, industry research, and development intensity, and industry economic activity. To overcome potential issues with data heteroscedasticity, we convert the framework's index data into a natural logarithm form. Through this, we aim to reduce the evaluation's exposure to heteroscedasticity. The revised approach is presented as follows:
(II)
(II)
The fixed-effect method only explores the linear relationship between foreign direct investment, foreign trade openness, and carbon emission intensity. As a result, foreign direct investment and the degree of foreign trade openness are employed as explanatory variables. Meanwhile, carbon emission intensity is used as the threshold variable to construct the Panel threshold model:
(III)
(III)
(IV)
(IV)
4. Estimation & findings
4.1. Simple linear regression
4.1.1. Unit root estimation
This study utilizes data from twenty segments of China's industrial sector from 2011 to 2021. The chosen data's panel structure imbues it with properties of both time series and cross-sectional data, and it is often nonstationary. As a result, the first stage of the analysis verifies the stationarity of the panel data by applying unit root tests. Three commonly used unit root tests are employed—LLC, ADF, and PP tests. All variables stabilize once first-order differencing is applied, as shown in .
Table 3. Findings of unit root estimation.
Table 4. Panel data cointegration estimation.
4.1.2. Cointegration estimation
Cointegration analysis uses the Pedroni and Kao tests. The variables examined include FDI, FTO, LNY, CDE, LNPE, LNACT, TOR, and FDI * TEC. The cointegration test results, presented in Table No. 4, reveal a long-run relationship between industry economic activity intensity, industry research, and development intensity, the number of employees, independent technological innovation indicators, GDP per capita, foreign trade, and foreign direct investment across all industries in the Chinese industrial sector.
4.1.3. Selection of panel regression approach
Panel data analysis often utilizes three primary approaches: fixed-effect methods, random-effect methods, and mixed-effect methods. Multiple tests are compared to identify the most suitable method. This study uses the F, LM, and Hausman tests. The F test compares the fixed-effects and mixed utility methods, while the LM test contrasts the mixed-effect method with the random-effect method. Lastly, the Hausman test is used to compare the fixed-effect method and the random-effect method.
reveals that the null hypothesis is rejected for all industries and highly polluting sectors across all three estimations. The former is preferred between the random and mixed-effect models; when compared to the mixed-effect model, the fixed-effect model is more suitable. Further, it is found that the fixed-effect model is preferable to the random-effect model. Based on these findings, the fixed-effect model is considered the most optimal.
Table 5. The panel regression approach selection estimation findings
4.1.4. Fixed effect approach regression
Control variables are gradually included in the fixed-effect model to analyze the effects of different determinants. These findings are depicted in . The results suggest that foreign direct investment is significant at the 10% level and significantly influences carbon emissions. However, the impact of the foreign direct investment coefficient on carbon emissions changes from negative to positive and back to negative as control variables are introduced. The mechanism through which foreign direct investment impacts carbon emissions is complex and requires further investigation.
Table 6. Fixed effect regression approach.
Contrary to previous studies, the relationship between foreign trade openness and carbon emissions is found to be insignificant. This discrepancy might be caused by the offsetting effects of different time frames, as indicated by the findings of the previous descriptive analysis. Therefore, further research using a threshold regression model is needed. Except for research and development intensity, all other control variables show significance. It suggests that these variables significantly influence carbon emissions.
In summary, we examine the influence of foreign direct investment on carbon emissions and the relationship between foreign trade openness and carbon emissions using threshold regression. This methodology allows us to assess how various levels of foreign trade openness and direct investment impact carbon emissions.
4.2. Threshold regression
4.2.1. FDI (foreign direct investment) is employed as an explanatory variable
Testing various criteria is crucial before a particular methodology is selected. Consequently, we analyze single, double, and triple thresholds using self-bootstrapping. Typically, 1000 sample iterations are used in the Bootstrap calculation for F and P values.
As shown in , all threshold models—single, double, and triple—exhibit significance at a 1% level. It suggests that when carbon emission intensity is taken as the threshold variable, the impact of foreign direct investment on carbon emission intensity displays a triple threshold. Hence, the triple threshold model is chosen. The next steps involve determining the threshold value and performing threshold regression. The results for the threshold values are shown in , while the results for threshold regression are presented in . These findings are supported by Chang et al. (Citation2020a), Chang et al. (Citation2020b), Imane et al. (Citation2023) and Lu et al. (Citation2023), whereas these findings are not supported by Syed et al. (Citation2019) and Hashmi et al. (Citation2021a, Citation2021b, and Citation2022).
Table 7. Threshold influence’s self-sampling estimation.
Table 8. Threshold analysis findings.
Table 9. Threshold regression findings.
and illustrate that foreign direct investment negatively and asymmetric impacts carbon emission intensity. The effects of varying amounts of foreign direct investment on carbon emission intensity can be grouped into four categories, significantly impacted by the total amount of foreign direct investment. An increase in foreign direct investment decreases carbon emission intensity when the carbon emission intensity is less than 1.349. For values of carbon emission intensity between 1.349 and 2.574, the marginal effectiveness drops to −40.193. The marginal effectiveness rises to −30.459 when the carbon emission intensity falls between 2.574 and 3.684. Lastly, the marginal effectiveness reaches zero when the carbon emission intensity exceeds 3.684. Hence, foreign direct investment has the highest absolute impact on carbon emission intensity when it falls between 1.349 and 2.574. These findings align with the results from Bashir, Sadiq, et al. (Citation2022a) but contradict those of Ma et al. (Citation2022) and Shahbaz et al. (Citation2021).
4.2.2. Foreign trade openness was employed as an explanatory variable
The findings of the Bootstrap assessment, which determines the F-value and P-value for each threshold estimation, are shown in . According to the single-threshold estimation, the single threshold is significant at a 1% level, with a p-value of 0.006. According to the double threshold estimation, the double threshold is significant at a 1% level, with a p-value of 0.003. The triple threshold is significant at a 5% level, as indicated by the triple threshold estimation's P-value of 0.039. Previous literature also provides similar findings (Chang et al., Citation2022a, Citation2022b; Maydybura et al., Citation2022; Noman et al. Citation2023; Peng et al. Citation2022).
Table 10. Threshold influence’s self-sampling calculation
The effects of trade openness on carbon emission intensity are shown in and , which are divided into four periods, and all demonstrate a substantial influence of trade openness. A rise in trade openness reduces carbon emission intensity when it falls below 2.1938, with foreign direct investment having a marginal efficiency of 1.192. The marginal efficiency rises to zero for carbon emission intensity levels between 2.1938 and 3.193, indicating that trade openness has little effect in this range. The marginal efficiency increases to 1.4049 when the intensity of carbon emissions is between 3.193 and 3.684. In this range, increased trade openness leads to a rise in carbon emissions intensity. Lastly, the marginal efficiency increases to 3.210 when the intensity of carbon emissions exceeds 3.684. Trade openness significantly affects carbon emission intensity when the intensity of carbon emissions surpasses 3.684. Foreign trade openness generally has a specific suppressive impact on carbon emissions when the intensity of carbon emissions is relatively low. However, trade openness starts to contribute to carbon emissions to some extent as the intensity of carbon emissions rises. These findings contradict Gohar et al. (Citation2022d) and Wang et al. (Citation2022). However, few other studies contradict with the findings of our study. These findings include Salman et al. (Citation2023a, Citation2023b) and Uche et al. (Citation2022a, Citation2022b).
Table 11. Threshold estimated findings.
The fixed-effect assessment reveals that contrary to most other research indicating a substantial influence of foreign trade openness on carbon emissions, there is no substantial symmetric influence of foreign trade openness on China's industrial carbon emissions. For example, Shahbaz et al. (Citation2019) found that foreign trade openness resulted in lower carbon dioxide emissions when they examined United States data from 1965 to 2016. Similarly, Huang et al. (Citation2020) assessed real carbon dioxide emissions in the China-Australia trade using the Bilateral Trade (EEBT) approach and long-term sectoral data. Their findings suggested that while international trade openness might reduce carbon emissions related to the use of green energy sources, technological spillovers could significantly contribute to carbon dioxide emissions associated with a specific trade. Nevertheless, there are significant impacts on carbon emissions from foreign direct investment and trade openness. The research conducted with the threshold regression approach indicates that the impacts of foreign direct investment and foreign trade openness on industrial carbon emissions vary across different carbon emission periods. Therefore, the threshold regression approach may be more suitable than the symmetric regression approach for studying the link between foreign direct investment, foreign trade openness, and industrial carbon emissions in China. It would enable a more comprehensive examination of the conflicting beneficial and harmful influences in the symmetric regression approach.
5. Conclusion & policy implications
The research adopts a panel threshold approach to assess the impacts of foreign direct investment and trade openness on carbon emissions. It analyzes eleven years of data from twenty sectors within China's industrial sector. The empirical evaluation of the research has led to the following findings:
Foreign direct investment hurts carbon emissions, which partially supports the concept of a "pollution halo." Regression results using the panel threshold approach reveal a nonlinear relationship between carbon emissions and foreign direct investment. The magnitude of carbon emission intensity influences the coefficient of foreign direct investment. Overall, foreign direct investment tends to reduce carbon emissions in China's manufacturing sector. The inhibitory effect of foreign direct investment on carbon emissions is particularly pronounced when the intensity of emissions falls within the range of [1.349, 2.574].
The coefficients determining whether trade openness positively or negatively impacts carbon emissions can be positive or negative. Moreover, these coefficients transition from negative to positive as carbon emissions increase. When the carbon emission intensity ranges between [2.3834, 3.1938], the trade openness coefficient is -0.89. Between [2.1938, 3.193], the trade openness coefficient is 0.12. As the carbon emission intensity rises to [3.193, 3.684], the trade openness coefficient increases to 0.102. Ultimately, when the carbon emission intensity reaches [3.684, 4.1370], the coefficient of trade openness rises to 3.19. Foreign trade openness tends to stimulate carbon emissions when the intensity of carbon emissions is lower. At the same time, it tends to mitigate carbon emissions when the intensity of carbon emissions is higher.
Economic activity intensity within the industry is significant at the 5% level, while measures such as per capita gross domestic product (GDP), independent technological advancement, and employment exhibit significance at the 1% level. Economic activity level significantly impacts carbon emissions. Specifically, the coefficients of per capita GDP and industry employment are negative, indicating that an increase in per capita GDP and industry employment leads to reduced carbon emissions from industrial operations.
These are the primary policy implications of this research:
The empirical findings demonstrate that the relationship between carbon emissions and foreign trade openness in China's industrial sector is highly complex, encompassing inhibitory and stimulatory influences on carbon emissions. It is crucial to promote foreign trade openness while concurrently mitigating carbon emissions in the industrial sector through effective environmental management regulations. Optimizing trade openness metrics is of paramount importance. As China's trade operations have evolved from "made in China" to "creation in China" and from labor-intensive industries to technology-intensive ones, the framework of international trade openness needs improvement. It can be achieved by expanding the import and export of environmentally friendly industries, boosting the export of technology-intensive sectors, and enforcing stricter environmental regulations for new businesses.
Increasing foreign direct investment (FDI) strategically is necessary. Priority should be given to attracting foreign investment in low-carbon industries while reducing funding for sectors with high carbon emissions. Implementing relevant policies that encourage foreign direct investment, facilitate the transfer of cutting-edge global technology, and capitalize on the positive effects of technological spillovers for environmental development is crucial. Foreign direct investment and international trade need progressive transformation for China's future economic growth and transition to a low-carbon economy. It can be achieved by implementing a "supply-side reform" in the industrial sector, focusing on reducing carbon emissions. Phasing out excess capacity and high-pollution industries should be part of this restructuring. Simultaneously, research and development of new sustainable technologies should be promoted to create a green industrial environment.
It is advisable to implement a range of subsidy measures that discourage carbon emissions in industries with high emission intensity thresholds while amplifying the adverse impact of foreign trade openness and foreign direct investment (FDI) in sectors with lower carbon intensity. Effectively addressing carbon emissions in different sectors requires close attention to the distinct categorization of carbon emission intensities among the twenty sectors within the industrial sector. Different strategies can be employed for sectors with varying carbon emission intensity, such as export tax rebates and tariffs. These regulations can encourage foreign direct investment (FDI) in sectors with low carbon emissions, foster international trade, and restrict or prohibit trade in sectors with high carbon emissions. This approach aims to enhance the organizational framework of the industrial sector, aligning it with low-carbon emission targets and promoting sustainable growth.
This study has several limitations that should be acknowledged. Firstly, the research is limited to a single country, which may restrict the generalizability of the findings. It is recommended to expand the analysis to include multiple countries and compare the findings across different contexts to enhance the robustness of the results. Additionally, incorporating various panel models, such as GMM and panel ARDL models, would yield valuable insights and bolster the analysis. Furthermore, using advanced time series techniques would provide a more comprehensive understanding of the interrelationships among the variables under investigation. Considering these factors would contribute to a more nuanced and comprehensive exploration of the research topic.
Data.xlsx
Download MS Excel (36.9 KB)Additional information
Notes on contributors
Salim Bagadeem
Dr. Salim Bagadeem has long experience in the academic field as he worked as a researcher at King Abdulaziz University, Saudi Arabia, casual tutor at La Trobe University, Melbourne, Australia and currently associated professor at the Arab Open University KSA. Dr Bagadeem has several publications in economics and applied econometrics topics. Dr Bagadeem has been awarded by several agencies such as, The Saudi Arabian Human Recourse Program (SAHR), King Abdul-Aziz University Jeddah, Teachers College Jeddah, King Abdulla Air Force College Jeddah and Arab Open University.
Raheel Gohar
Dr. Raheel Gohar is working as an Associate Professor of finance at the College of Business, Al Yamamah University. He has more than 15 years’ experience of corporate, teaching and research. He has served in key administrative and leadership positions. His research interests include: corporate finance, corporate governance, family enterprises, financial statement analysis, derivatives and equity valuation. He has more than 20 research papers published in different journals and has presented his research work in conferences around twelve different countries. His research has been published in high quality peer-reviewed international journals, such as the Journal of Business Ethics, Emerging Markets Finance and Trade, International Journal of Intercultural Relations and Afro-Asian Journal of Finance and Accounting.
Wing-Keung Wong
Wing-Keung Wong: Obtained Ph.D. from the University of Wisconsin-Madison, WONG, Wing-Keung is a Chair Professor at the Department of Finance, Asia University. He was a Full Professor at Hong Kong Baptist University and Deputy Director at the National University of Singapore. He appears in “Who's Who in the World”. He is on the list of the "2022 World's top 2% of researchers/scientists" and on the list of the "Best Economics and Finance Scientists”, ranked 788th in the world and ranked the first in Taiwan. He is on the list of top Taiwan economists and Asian economists and top economists by RePEc. He serves as editor for some international journals.
Asma Salman
Professor Asma Salman is a Blockchain Developer and a Professor of Finance at the American University in the Emirates, (AUE), UAE. She served as the Dubai Cohort supervisor for students under the Nottingham Business School DBA program, at Nottingham Trent University, UK for 7 years. She is presently also affiliated as a visiting fellow at the University of Northampton, UK for DBA supervisory responsibilities. She has been actively engaged in scientific research and has published impactful articles in Impact factor Journal, Conferences and Books. Handling editor and board member at Journals of high repute and international bibliography, one of her recent articles on “Bitcoin and Blockchain” has gained wide visibility across the world and won the award for “Blockchain professional of the Year” by Berkley Middle East and served on the Board of Etihad Airlines during 2019-2020. Professor Salman was recently bestowed with the Women Leadership Impact Award by Her Excellency First Lady of Armenia, Research Excellence Award, Women in Education, Leadership Award and the Global Inspirational Women Leadership Award by His Highness Sheikh Juma Bin Maktoum Juma Al Maktoum.
Bisharat Hussain Chang
Dr. Bisharat Hussain Chang is working as Assistant Professor at Sukkur IBA University, Sukkur, Sindh, Pakistan. Dr. Chang has published more than 50 research papers in finance and economics related journals. These articles are published in renowned journals indexed in SSCI, SCIE, Scopus and/or ABDC listed and other reputable indices. Dr. Chang has published articles various sub areas like stock markets, bond markets, foreign exchange markets, oil prices, cryptocurrencies, economic growth, environmental studies, tourism, energy prices, energy demand, energy economics, and other related macroeconomic and financial variables/areas.
References
- Ali, W., Gohar, R., Chang, B. H., & Wong, W. K. (2022). Revisiting the impacts of globalization, renewable energy consumption, and economic growth on environmental quality in South Asia. Advances in Decision Sciences, 26(3), 78–98.
- Baek, J., & Koo, W. W. 2008. A dynamic approach to the FDI-environment nexus: The case of China and India (vol.13). Social Science Electronic Publishing.
- Balsalobre‐Lorente, D., Driha, O. M., Halkos, G., & Mishra, S. (2022). Influence of growth and urbanization on CO2 emissions: The moderating effect of foreign direct investment on energy use in BRICS. Sustainable Development, 30(1), 227–240. https://doi.org/10.1002/sd.2240
- Bashir, M. F., Pan, Y., Shahbaz, M., & Ghosh, S. (2023a). How energy transition and environmental innovation ensure environmental sustainability? Contextual evidence from top-10 manufacturing countries. Renewable Energy, 204, 697–709. https://doi.org/10.1016/j.renene.2023.01.049
- Bashir, M. F. (2022). Discovering the evolution of pollution haven hypothesis: A literature review and future research agenda. Environmental Science and Pollution Research, 29(32), 48210–48232. https://doi.org/10.1007/s11356-022-20782-1
- Bashir, M. F., Sadiq, M., Talbi, B., Shahzad, L., & Adnan Bashir, M. (2022a). An outlook on the development of renewable energy, policy measures to reshape the current energy mix, and how to achieve sustainable economic growth in the post COVID-19 era. Environmental Science and Pollution Research, 29(29), 43636–43647. https://doi.org/10.1007/s11356-022-20010-w
- Behera, S. R., & Dash, D. P. 2017. The effect of urbanization, energy consumption, and foreign direct investment on the carbon dioxide emission in the SSEA (South and Southeast Asia) region. Renewable and Sustainable Energy Reviews, 70(4), 96–106. https://doi.org/10.1016/j.rser.2016.11.201
- Chang, B. H. (2020). Oil prices and E7 stock prices: An asymmetric evidence using multiple threshold nonlinear ARDL model. Environmental Science and Pollution Research International, 27(35), 44183–44194. https://doi.org/10.1007/s11356-020-10277-2
- Chang, B. H., Rajput, S. K. O., Bhutto, N. A., & Abro, Z. (2020a). Asymmetric effect of extreme changes in the exchange rate volatility on the US imports: Evidence from multiple threshold nonlinear autoregressive distributed lag model. Studies in Economics and Finance, 37(2), 293–309. https://doi.org/10.1108/SEF-03-2019-0122
- Chang, B. H., Sharif, A., Aman, A., Suki, N. M., Salman, A., & Khan, S. A. R. (2020b). The asymmetric effects of oil price on sectoral Islamic stocks: New evidence from quantile-on-quantile regression approach. Resources Policy, 65, 101571. https://doi.org/10.1016/j.resourpol.2019.101571
- Chang, B. H., Rajput, S. K. O., Ahmed, P., & Hayat, Z. (2020c). Does Gold Act as a Hedge or a safe haven? Evidence from Pakistan. The Pakistan Development Review, 59(1), 69–80. https://doi.org/10.30541/v59i1pp.69-80
- Chang, B. H., Derindag, O. F., Hacievliyagil, N., & Canakci, M. (2022a). Exchange rate response to economic policy uncertainty: Evidence beyond asymmetry. Humanities & Social Sciences Communications, 9(1), 358. https://doi.org/10.1057/s41599-022-01372-5
- Chang, B. H., Channa, K. A., Uche, E., Khalaf, O. I., & Ali, O. W. (2022b). Analyzing the impacts of terrorism on innovation activity: A cross country empirical study. Advances in Decision Sciences, 26(Special), 124–161.
- Chang, B. H., Auxilia, P. M., Kalra, A., Wong, W. K., & Uddin, M. A. (2023a). Greenhouse gas emissions and the rising effects of renewable energy consumption and climate risk development finance: Evidence from BRICS countries. Annals of Financial Economics, 2023, 2350007. https://doi.org/10.1142/S2010495223500070
- Chang, B. H., Gohar, R., Derindag, O. F., & Uche, E. (2023b). COVID-19, lockdown measures and their impact on food and healthcare prices: Empirical evidence using a dynamic ARDL model. Journal of Economic Studies, 50(5), 1008–1026. https://doi.org/10.1108/JES-12-2021-0627
- Chen, Y., Wang, Z., & Zhong, Z. 2018. CO2 emissions, economic growth, renewable and nonrenewable energy production, and foreign trade in China. Renewable Energy, 131(2), 208–216. https://doi.org/10.1016/j.renene.2018.07.047
- Chen, F., Ahmad, S., Arshad, S., Ali, S., Rizwan, M., Saleem, M. H., Driha, O. M., & Balsalobre-Lorente, D. (2022). Towards achieving eco-efficiency in top 10 polluted countries: The role of green technology and natural resource rents. Gondwana Research, 110, 114–127. https://doi.org/10.1016/j.gr.2022.06.010
- Derindag, O. F., Chang, B. H., Gohar, R., & Salman, A. (2022). Exchange rate effect on the household consumption in BRICST countries: Evidence from MATNARDL model. Journal of International Commerce, Economics and Policy, 13(02), 2250010. https://doi.org/10.1142/S1793993322500107
- Derindag, O. F., Chang, B. H., Gohar, R., Wong, W. K., & Bhutto, N. A. (2023a). Food prices response to global and national factors: Evidence beyond asymmetry. Cogent Economics & Finance, 11(1), 2187128. https://doi.org/10.1080/23322039.2023.2187128
- Derindag, O. F., Maydybura, A., Kalra, A., Wong, W. K., & Chang, B. H. (2023b). Carbon emissions and the rising effect of trade openness and foreign direct investment: Evidence from a threshold regression model. Heliyon, 9(7), e17448. https://doi.org/10.1016/j.heliyon.2023.e17448
- Dogan, E., & Inglesi-Lotz, R. 2020.The impact of economic structure on the Environmental Kuznets Curve (EKC) hypothesis: Evidence from European countries. Environmental Science and Pollution Research, 27(3), 12717–12724. https://doi.org/10.1007/s11356-020-07878-2
- Dogan, E., Taspinar, N., & Gokmenoglu, K. K. 2019.Determinants of ecological footprint in MINT countries. Energy and Environment, 30(6), 1065–1086. https://doi.org/10.1177/0958305X19834279
- Foss, J., & Laursen, K. 2005.Performance pay delegation and multitasking under uncertainty and innovativeness: An Empirical investigation. Journal of Economic Behavior and Organization, 58(2), 246–276. https://doi.org/10.1016/j.jebo.2004.03.018
- Gohar, R., Bagadeem, S., Chang, B. H., & Zong, M. (2022a). Do the income and price changes affect consumption in the emerging 7 countries? Empirical evidence using quantile ARDL model. Annals of Financial Economics, 17(04), 2250024. https://doi.org/10.1142/S2010495222500245
- Gohar, R., Bhatty, K., Osman, M., Wong, W. K., & Chang, B. H. (2022b). Oil prices and sectorial stock indices of Pakistan: Empirical evidence using bootstrap ARDL model. Advances in Decision Sciences, 26(4), 1–27.
- Gohar, R., Chang, B. H., Derindag, O. F., & Abro, Z. (2022c). Nexus between consumption, income, and price changes: Asymmetric Evidence from NARDL Model. ETIKONOMI, 21(2), 213–228. https://doi.org/10.15408/etk.v21i2.23339
- Gohar, R., Osman, M., Uche, E., Auxilia, P. M., & Chang, B. H. (2022d). The economic policy uncertainty extreme dynamics and its effect on the exchange rate. Global Economy Journal, 22(03), 2350006. https://doi.org/10.1142/S2194565923500069
- Gohar, R., Salman, A., Uche, E., Derindag, O. F., & Chang, B. H. (2023a). Does US infectious disease equity market volatility index predict G7 stock returns? Evidence beyond symmetry. Annals of Financial Economics, 18(02), 2250028. https://doi.org/10.1142/S2010495222500282
- Gohar, R., Chang, B. H., Uche, E., Uddin, M. A., & Kalra, A. (2023b). Nexus between energy consumption, climate risk development finance and GHG emissions. International Journal of Financial Engineering, 10(03), 251. https://doi.org/10.1142/S2424786323500251
- Gong, X., Chang, B. H., Chen, X., & Zhong, K. (2023). Asymmetric effects of exchange rates on energy Demand in E7 Countries: New evidence from multiple thresholds nonlinear ARDL Model. Romanian Journal of Economic Forecasting, 26(2), 125.
- Hashmi, S. M., & Chang, B. H. (2021). Asymmetric effect of macroeconomic variables on the emerging stock indices: A quantile ARDL approach. International Journal of Finance & Economics, 28(1), 1006–1024. https://doi.org/10.1002/ijfe.2461
- Hashmi, S. M., Chang, B. H., & Shahbaz, M. (2021a). Asymmetric effect of exchange rate volatility on India’s cross‐border trade: Evidence from global financial crisis and multiple threshold nonlinear autoregressive distributed lag model. Australian Economic Papers, 60(1), 64–97. https://doi.org/10.1111/1467-8454.12194
- Hashmi, S. M., Chang, B. H., & Rong, L. (2021b). Asymmetric effect of COVID-19 pandemic on E7 stock indices: Evidence from quantile-on-quantile regression approach. Research in International Business and Finance, 58, 101485. https://doi.org/10.1016/j.ribaf.2021.101485
- Hashmi, S. M., Chang, B. H., Huang, L., & Uche, E. (2022). Revisiting the relationship between oil prices, exchange rate, and stock prices: An application of quantile ARDL model. Resources Policy, 75, 102543. https://doi.org/10.1016/j.resourpol.2021.102543
- Haug, A. A., & Ucal, M. 2019. The role of trade and FDI for CO2 emissions in Turkey: Nonlinear relationships. Energy Economics, 81(6), 297–307. https://doi.org/10.1016/j.eneco.2019.04.006
- Huang, R., Chen, G., Lv, G., Malik, A., Shi, X., & Xie, X. 2020. The effect of technology spillover on CO2 emissions embodied in China-Australia trade. Energy Policy, 144, 111544. https://doi.org/10.1016/j.enpol.2020.111544
- Imane, E., Chang, B. H., Elsherazy, T. A., Wong, W. K., & Uddin, M. A. (2023). The external exchange rate volatility influence on the trade flows: Evidence from Nonlinear ARDL Model. Advances in Decision Sciences, 27(2), 75–98.
- Jiang, T., Yu, Y., Jahanger, A., & Balsalobre-Lorente, D. (2022). Structural emissions reduction of China's power and heating industry under the goal of " double carbon": A perspective from input-output analysis. Sustainable Production and Consumption, 31, 346–356. https://doi.org/10.1016/j.spc.2022.03.003
- José, P. P. 2007. A non-monotonic relationship between FDI and trade. Economics Letters. 95(3), 369–373.
- Lei, J., Lin, S., Khan, M. R., Xie, S., Sadiq, M., Ali, R., … Bashir M. F., Shahzad, L., Eldin, S. M., & Amin, A. H. (2022). Research trends of board characteristics and firms’ environmental performance: Research directions and agenda. Sustainability, 14(21), 14296. https://doi.org/10.3390/su142114296
- Li, Z. C., & Wen, X. T. 2013. The empirical analysis of the influence of foreign direct investment on the export trade structure-taking Jiangsu province as an example. Technoeconomics and Management Research.
- Li, Z. H., & Liu H. H., 2012. Are there threshold effects of FDI on the environment? Evidence from 220 cities in China. Finance and Trade Economics, 9, 101–108.
- Lu, M., Chang, B. H., Salman, A., Razzaq, M. G. A., & Uddin, M. A. (2023). Time varying connectedness between foreign exchange markets and crude oil futures prices. Resources Policy, 86, 104128. https://doi.org/10.1016/j.resourpol.2023.104128
- Ma, B., Wang, Y., Zhou, Z., Lai, Y., Zhou, Z., & Bashir, M. F. (2022). Can controlling family involvement promote firms to fulfill environmental responsibilities?—Evidence from China. Managerial and Decision Economics, 43(2), 569–592. https://doi.org/10.1002/mde.3403
- Maydybura, A., Gohar, R., Salman, A., Wong, W. K., & Chang, B. H. (2023). The asymmetric effect of the extreme changes in the economic policy uncertainty on the exchange rates: evidence from emerging seven countries. Annals of Financial Economics, 18(02), 2250031. https://doi.org/10.1142/S2010495222500312
- Metulini, R., Riccaboni, M., Sgrignoli, P., & Zhu, Z. (2017). The indirect effects of FDI on trade: A network perspective (working papers).
- Noman, M., Maydybura, A., Channa, K. A., Wong, W. K., & Chang, B. H. (2023). Impact of cashless bank payments on economic growth: Evidence from G7 countries. Advances in Decision Sciences, 27(1), 1–22.
- Pao, H. T., & Tsai, C. M. 2011.Multivariate Granger causality between CO2 emissions, energy consumption, FDI (foreign direct investment) and GDP (gross domestic product): Evidence from a panel of BRIC (Brazil, Russian Federation, India, and China) countries. Energy, 36(1), 685–693. https://doi.org/10.1016/j.energy.2010.09.041
- Peng, B., Yang, B. H., Zhu, L., & C., Chang. (2022). Exchange rate and energy demand in G7 countries: Fresh insights from Quantile ARDL model. Energy Strategy Reviews, 44, 100986. https://doi.org/10.1016/j.esr.2022.100986
- Rafei, M., Esmaeili, P., & Balsalobre-Lorente, D. (2022). A step towards environmental mitigation: How do economic complexity and natural resources matter? Focusing on different institutional quality level countries. Resources Policy, 78, 102848. https://doi.org/10.1016/j.resourpol.2022.102848
- Ren, S, G., Yuan, B., Ma, X., & Chen, X. 2014. The impact of international trade on China’s industrial carbon emissions since its entry into WTO. Energy Policy, 69, 624–634. https://doi.org/10.1016/j.enpol.2014.02.032
- Ren, S., Yuan, B., Ma, X., & Chen, X. 2014. International trade, FDI (foreign direct investment) and embodied CO2 emissions: A case study of China’s industrial sectors. China Economic Review, 28(C), 123–134. https://doi.org/10.1016/j.chieco.2014.01.003
- Salman, A., Razzaq, M. G. A., Chang, B. H., Wong, W. K., & Uddin, M. A. (2023a). Carbon emissions and its relationship with foreign trade openness and foreign direct investment. Journal of International Commerce, Economics and Policy, 14(03) https://doi.org/10.1142/S1793993323500230
- Salman, A., Chang, B. H., Abdul Razzaq, M. G., Wong, W. K., & Uddin, M. A. (2023b). The emerging stock markets and their asymmetric response to infectious disease equity market volatility (ID-EMV) index. Annals of Financial Economics, 2023, 2350008. https://doi.org/10.1142/S2010495223500082
- Shahbaz, M., Bashir, M. F., Bashir, M. A., & Shahzad, L. (2021). A bibliometric analysis and systematic literature review of tourism-environmental degradation nexus. Environmental Science and Pollution Research, 28(41), 58241–58257. https://doi.org/10.1007/s11356-021-14798-2
- Shahbaz, M., Gozgor, G., Adom, P. K., & Hammoudeh, S. 2019. The technical decomposition of carbon emissions and the concerns about FDI and trade openness effects in the United States. International Economics, 159, 56–73. https://doi.org/10.1016/j.inteco.2019.05.001
- Sinha, A., Balsalobre-Lorente, D., Zafar, M. W., & Saleem, M. M. (2022). Analyzing global inequality in access to energy: Developing policy framework by inequality decomposition. Journal of environmental management, 304, 114299. https://doi.org/10.1016/j.jenvman.2021.114299
- Sulaman, M., Long, X, L., Muhammad, S., & Lamini, D. 2020. Effect of urbanization and international trade on CO2 emissions across 65 belt and road initiative countries. Energy, 196, 117102. https://doi.org/10.1016/j.energy.2020.117102
- Syed, Q. R., Malik, W. S., & Chang, B. H. (2019). Volatility spillover effect of federal Reserve’S balance sheet on the financial and goods markets of Indo-Pak Region. Annals of Financial Economics, 14(03), 1950015. https://doi.org/10.1142/S2010495219500155
- Uche, E., Chang, B. H., & Effiom, L. (2022a). Household consumption and exchange rate extreme dynamics: Multiple asymmetric threshold non‐linear autoregressive distributed lag model perspective. International Journal of Finance & Economics, 28(3), 3437–3450. https://doi.org/10.1002/ijfe.2601
- Uche, E., Chang, B. H., & Gohar, R. (2022b). Consumption optimization in G7 countries: Evidence of heterogeneous asymmetry in income and price differentials. Journal of International Commerce, Economics and Policy, 13(01), 2250002. https://doi.org/10.1142/S1793993322500028
- Wang, X., Chang, B. H., Uche, E., & Zhao, Q. (2022). The asymmetric effect of income and price changes on the consumption expenditures: Evidence from G7 countries using nonlinear bounds testing approach. Portuguese Economic Journal, 2022, 1–19.
- Xu, Y. D. 2014. Foreign direct investment, trade openness, and CO2 emissions: Taking Shandong province as an example. Advanced Materials Research, 1073, 2691–2699. https://doi.org/10.4028/www.scientific.net/AMR.1073-1076.2691
- Yunfeng, Y., & Laike, Y. (2010). China’s foreign trade and climate change: A case study of CO2 emissions. Energy Policy, 38(1), 350–356.
- Zhu, H., Duan, L., Guo, Y., & Yu, K. (2016). The effects of FDI, economic growth and energy consumption on carbon emissions in ASEAN-5: Evidence from panel quantile regression. Economic Modelling, 58, 237–248. https://doi.org/10.1016/j.econmod.2016.05.003