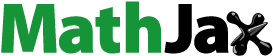
Abstract
The study analyzed the extent of financial cycle synchronization, as represented by the Aggregate Financial Cycle and the Credit and Housing Cycle, in South Africa from 1975q1 to 2023q4 using the Dynamic Conditional Correlation model and the Vector Erro Correction Mechanism. Financial cycles are a significant source of systemic risks monitored by both the Prudential Authority and the South African Reserve Bank through macroprudential policies in South Africa. Understanding the interactions among these cycles can improve the formulation of such policies. The findings indicated a higher level of homogeneity in the stability, size, and movement of financial cycles compared to their relationship with business cycles, suggesting an increased synchronization of financial cycles. Additionally, the results highlighted strong correlations among financial cycles, intensifying during financial crisis episodes. This finding underscores that there are significant contagion effects during times of financial turmoil in South Africa. Moreover, the analysis revealed a positive long-term relationship between financial cycles, reflecting the procyclicality of the South African financial system and its tendency to amplify economic fluctuations, exacerbating both booms and busts. In light of these findings, policymakers should prioritize coordinated macroprudential measures to mitigate systemic risks, enhance financial stability, and bolster the resilience of the overall financial system, especially during times of crisis.
Impact statement
The study looked at how different financial cycles connect over time in South Africa. The study found that during financial crises, problems in one part of the financial sector can quickly spread to others. This shows how risky the South African financial system can be, even when things seem to be going well. It’s like a rollercoaster – both the ups and downs can be dangerous. Our findings mean that it’s really important for the government to have strong macroprudential policy rules in place to protect the financial system, especially when things get tough. By doing this, we can help prevent big problems and make sure our economy stays healthy for everyone.
SUBJECTS:
1. Introduction
The 2007/2009 global financial crisis (GFC) triggered an important discussion on the role of financial cycles in macro-financial dynamics. These cycles – financial cycles – described as the medium-term deviations of financial markets from their longer-term trend – propagate economic shocks (Bemanke & Gertler, Citation1989; Gertler & Karadi, Citation2011; Kiyotaki & Moore, Citation1997), and they can also be a source and an instigator of great recessions and financial disruptions through their impact on business cycles (Borio, Citation2014; Claessens et al., Citation2011; Oman, Citation2019). Moreover, given the financial cycles’ association with financial booms and busts in the financial system, it has been argued that financial cycles have an advantage in predicting systematic financial crises relative to other macroeconomic cycles like the business cycle for instance (Borio et al., Citation2018; Oman, Citation2019; Stremmel, Citation2015). Between scholars and international regulatory bodies such as the Bank of International Settlements and the International Monetary Fund, there is a consensus that financial cycles should be monitored by macroprudential policies to contain the build of financial cycle threats over time (Cerutti et al., Citation2017; Rünstler & Vlekke, Citation2018) and that monetary policies ought to ‘lean against the wind’ of financial cycles given the interplays between the real and financial sectors (Agenor & Da Silva, Citation2019; Borio, Citation2014). Thus, countries that have macroprudential policy frameworks, such as South Africa, state that the objective of their macroprudential policy is to stabilize financial cycles and mitigate the threats they pose (Bosch and Koch, Citation2020). However, as argued by Drehmann et al. (Citation2012), Rünstler and Vlekke (Citation2018), and Adarov (Citation2017), the implementation of monetary and macroprudential policies requires that policymakers understand financial cycles and the interplays that exist between these cycles.
Several contributions have examined financial cycles in advanced economies, but fewer studies have concentrated on emerging market economies such as South Africa. Among others, Amador-Torres et al. (Citation2014), Claessen et al. (Citation2011a), De Backer et al. (Citation2018), Drehmann et al. (Citation2012), Kunovac et al. (Citation2018), Mandler and Scharnagl (Citation2019), Merler (Citation2015), Oman (Citation2019), Rünstler and Vlekke (Citation2018) have investigated financial cycles in the advanced economies. This gap, which is the focus on advanced economies, limits our understanding of interactions of financial cycles from an empirical stand in emerging market economies and can impede the ability of policymakers to effectively conduct and formulate macroprudential policies aimed at stabilizing financial cycles. A common finding in the above-mentioned studies is that while financial cycles experience episodes of expansions and downturns which is like business cycles, financial cycles are much longer and ampler in duration than business cycles (Drehmann et al., Citation2012). Moreover, these studies found that credit and asset prices were the best proxies of financial cycles as the movement of these variables coincided with significant changes in financial conditions in the advanced economies (Claessen et al., Citation2011a). Nevertheless, the studies above rarely considered the issue of financial cycle synchronization. Financial cycle synchronization is not only an academic endeavor, but it also has in-depth macroprudential policy ramifications. Strong synchronization of financial cycles implies that financial markets are prone to simultaneous distress; hence, macroprudential policy ought to be deployed to the whole financial system during a financial disturbance (Adarov, Citation2017). However, the lack of synchronizations means that financial markets are not susceptible to simultaneous distress; hence, macroprudential policy should be deployed to the affected financial sectors only during a financial disturbance.
There is a scant body of literature on financial cycles in South Africa, and these few studies, for instance, Farrell and Kemp (Citation2020), Milan and Botha (Citation2019), Pahla (Citation2019), Nyati et al. (Citation2021), Bosch and Koch (Citation2020), De Wet and Botha (Citation2022) fail to consider the synchronization of financial cycles which this study considers. These studies found that credit, house, and equity prices were the best proxies of financial cycles in South Africa, similar to the advanced economies. Moreover, these studies found that, on average, a financial cycle can have a duration between 10 and 30 years, with its peaks occurring at or around a time of crisis. These studies often use static models such as Bry and Boschan’s (Citation1971) turning point analysis, Bexter-King filter, and Markov Switching Dynamic Regression to examine properties of financial cycles in South Africa. Hence, in light of the above, the objective of this study is to gauge the extent of financial cycle synchronization in South Africa. The contribution of this study is two-fold. First, this study employs a time-varying parameter model, namely, the Dynamic Conditional Correlation (DCC) pioneered by Engle (Citation2002), to assess the synchronization of financial cycles in South Africa between 1975q1 and 2023q4, an adequate period to detect any changes in direction and significance of financial cycle synchronization. Second, and in addition to the DCC, the paper uses a Vector Error Correction Mechanism (VECM) to further shed light on how financial cycles interact and influence each other. The research questions in this paper are: How similar or dissimilar are properties of financial cycles in South Africa? How strong is the correlation of financial cycles in South Africa? Does the correlation of financial cycles vary over time in South Africa? Is there an equilibrium relationship between financial cycles in South Africa? And, how long does it take for financial cycles to adjust back to equilibrium if their relationship is disturbed in South Africa? By examining the relationship and dynamics between various versions of financial cycles in South Africa the findings of this study are expected to contribute towards the formulation of macroprudential policies in South Africa.
The rest of this study is organized as follows. Section two is the literature review. Section three is the data and methodology section. Section four is the results and discussion section. Finally, section five is the summary and conclusion section.
2. Literature review
Understanding the behavior and interconnectedness of financial cycles is crucial to guide the conduct of macroprudential policies. It gained momentum during the 2007–2009 financial crisis (Borio, Citation2014; Claessen et al., Citation2011a; Drehmann et al., Citation2012; Stremmel Citation2015; Agenor & da Silva, Citation2013; Alfaro et al., Citation2007; Boar et al., Citation2017; Kim & Mehrotra, Citation2017; Mishkin & Bank, Citation2011; Paoli & Paustian, Citation2017; Peltonen, Citation2017). The main questions of the present literature review are: what are financial cycles, and why do they matter?
Despite their significance, there is no agreed-upon definition for financial cycles (Oman, Citation2019). The commonly accepted definition is that of Borio (2014), which states that financial cycles are ‘self-reinforcing interactions between perceptions of value and risk, attitudes towards risk and financing constraints, which translate into booms followed by busts’ (Borio, 2014; Juselius et al., Citation2016). In line with this definition, it has been demonstrated that financial cycles can be represented by the co-movement of financial indicators (Oman, Citation2019). A significant body of literature has found that the co-movement of credit and house prices coincides with the booms and busts of the whole financial system; hence, credit and asset prices are the best representation of financial cycles (Claessen et al., Citation2011b; Drehmann et al., Citation2012; Gorton & Ordonez, 2017; Oman, Citation2019; Stremmel, Citation2015; Stremmel & Zsámboki, Citation2015). While it has been accepted that credit and asset prices are the best proxies of financial cycles, Oman (Citation2019), on the other hand, demonstrates that leverage is strongly associated with the movement of credit and asset prices, which makes leverage another suitable proxy for financial cycles. Adarov (Citation2017) demonstrates that share prices and long-term interest rates are also linked to credit and house prices, making them suitable for capturing financial cycles. This is similar to Borio et al. (Citation2016). Therefore, it can be said that financial cycles are co-movements of financial indicators that can explode into booms and busts in the financial system.
Several features set financial cycles apart from conventional business cycles. The duration of financial cycles is longer and ampler than that of business cycles, which is shorter and less ample (Borio, 2014; Drehmann et al., Citation2012). Drehmann et al. (Citation2012) found that in the a typical financial cycle measured by credit and asset prices lasts up to thirty years, whereas a business cycle lasts up to eight years. Similarly, Hiebert et al. (Citation2018) found that in the financial cycles last 15 years on average compared to 6.5 years for business cycles, while Beirne, (Citation2020) found that financial cycles are four times larger than business cycles. These findings highlight that financial and business cycles are distinct from each other in their features. This is why these cycles have different uses. Business cycles anchor price stability targeted by monetary policy onver the short to medium term, whereas financial cycles anchor financial stability, which is the long-term target of macroprudential policy (Agenor & Da Silva, Citation2019). There are several factors behind this distinction, including financial frictions (Bemanke & Gertler, Citation1989; Bernanke et al., Citation1994; Kiyotaki & Moore, Citation1997) and policy regimes (Borio, 2014; Borio et al., Citation2001; Cagliarini & Price, Citation2017). For instance, the shift towards more financially liberal economies since the 1980s has amplified the length and amplitude of financial cycles (Borio & Lowe, Citation2002). This is because financial constraints are relaxed with more financial liberalization, supporting full interplay between financial markets (Borio, 2014). This is why strong capital flows are associated with strong financial booms (Claessen et al., Citation2011a).
As a concept distinct from the business cycle, financial cycles play a crucial role in the macro-financial economy. As early as the 'Debt-deflation’ theory of Fisher (Citation1933), followed by the 'Instability Hypothesis’ of Minsky (Citation1982), it has been accepted that financial cycles influence economic conditions (Fisher, Citation1933; Gurley & Shaw, Citation1955; Minsky, Citation1977, Citation1982). Fisher argued that over-indebtedness causes the velocity of money to slow down, ultimately reducing aggregate spending and the price level (Fisher, Citation1933; Parusel & Viegi, Citation2009). This is because high debt levels reduce the rate at which currency deposits occur, hence the velocity of money (Fisher, Citation1933). In turn, a falling price level causes debt to appreciate in real terms, further putting downward pressure on aggregate spending and the price level (Fisher, Citation1933). Therefore, over-indebtedness causes deflation (Fisher, Citation1933). On the other hand, Minsky argued that the procyclical supply of credit can wreak havoc in the financial system and increase the probability of a financial crisis (Minsky, Citation1977, Citation1982). This is because credit expansion may stimulate investment into riskier assets, which may seem safe during good times, fuelling the buildup of financial imbalances (Parusel & Viegi, Citation2009). Indeed, evidence suggests that credit and asset price booms and busts have coincided with deteriorating global financial conditions (Borio, 2014; Drehmann et al., Citation2012). This explains why, in many economies, including South Africa, macroprudential policy authorities aim to smooth out excessive growth in credit and asset prices in order to prevent cracks from opening up in their financial systems (Dlamini and Ngalawa, Citation2022).
Other studies suggest that financial cycles matter for economic growth. Goldsmith (Citation1969), and Gurley and Shaw (Citation1955), were the first to accentuate that financial developments in credit could lead to economic growth and development. Credit is used to finance household spending and investment by governments and firms (Keynes, Citation1964), which in turn contributes to economic growth. Consequently, credit condition improvement stimulates spending and investment, which improves the economic growth rate (Parusel & Viegi, Citation2009). More recently, it has been observed that the deepening and integration of financial markets can lead to high economic growth (e.g., Adarov, Citation2017; Beck & Levine, Citation2004; Beck, Citation2008). This is because financial deepening can mobilize economic resources toward efficient use (Cagliarini & Price, Citation2017). For instance, financial deepening is associated with increased savings, investment, and economic growth (Bakang, Citation2015). Financial developments can also contribute negatively to economic growth. Following the recent financial crisis, it has been demonstrated that financial developments are associated with deep recessions (Borio, Citation2014; Borio et al., Citation2016; Borio et al., Citation2018; Classen et al., Citation2011a; Drehmann et al., Citation2012; Ma & Zhang, Citation2016). Claessen et al. (Citation2011a) found that financial busts relate to deep recessions, as in the previous financial crisis. During a recession that coincides with a financial bust, financial and economic conditions deteriorate simultenously, which then compounds and intensifies the recession since the financial system cannot finance the recovery phase (Ma & Zhang, Citation2016). Therefore, given their significance, financial cycles have courted a lot of policy attention/interest. In particular, given that they influence financial stability and price and output developments, they are essential to guiding macroprudential and monetary policies (Agenor & Da Silva, Citation2019; Schumpeter, Citation1911).
Following this, several studies investigate financial cycles at the country level to determine if they exist and how they affect the macro-financial economy. Some of these include Adarov (Citation2017), Aikman et al. (Citation2019), Alcidi (Citation2017), Claessen et al. (Citation2011b), De Backer et al. (Citation2018), Drehmann et al. (Citation2012), Harun et al. (Citation2014), Kunovac et al. (Citation2018), Mandler and Scharnagl (Citation2019), Oman (Citation2019). However, these studies mostly focus on advanced economies, neglecting emerging market economies such as South Africa. For instance, Drehmann et al. (Citation2012) focused on Australia, Germany, the United Kingdom, Japan, Norway, Sweden, and the United States, while Oman (Citation2019) focused on the Eurozone, whereas Claessen et al. (Citation2011a) focused on 21 advanced economies. Amongst the fewer studies investigating financial cycles in South Africa, for instance, De Wet and Botha (Citation2022), Farrell and Kemp (Citation2020), Nyati et al. (Citation2021), Bosch and Koch (Citation2020), Pahla (Citation2019), Milan and Botha (Citation2019). Farrell and Kemp (Citation2020) found that credit and asset price ratios were good approximations of South African financial cycles. The study further found that financial cycles have a longer duration and a larger amplitude than the traditional business cycle and that periods, where financial conditions are stressed, are associated with peaks in the financial cycle. De Wet and Botha (Citation2022) found that credit and monetary conditions were significant drivers of financial cycles in South Africa. Whereas Bosch and Koch (2020) found that the average duration of a financial cycle in South Africa is 17 years. However, these studies did not consider the issue of synchronization, which this study considered. The following section introduces the data and methodology employed in this study to address this objective.
3. Data and methodology
3.1. Data and description of variables
The study covers the sample period 1975Q1–2023Q4. It is common in the literature to rely on credit and property prices to represent financial cycles (e.g., Borio, 2014; Drehmann et al., Citation2012). Other studies include share prices and real interest rates (Adarov, Citation2017). This study uses five financial indicators, namely credit from all sectors to the non-financial sector as a percentage of GDP (CRD), credit-to-GDP gap (CRG); housing prices (H.R.); share prices (SHR) and long-term interest rates (L.R.). The first two variables were sourced from the Bank of International Settlements (BIS), while the other three were sourced from the Organisation for Economic Cooperation and Development (OECD). The primary reason the study relies on credit and house prices to identify financial cycles is Stremmel (Citation2015), Drehmann et al. (Citation2012), Richter et al. (Citation2018), Schularick and Taylour (Citation2012), Sukati (Citation2013), and Poggio et al. (Citation2001). These scholars argue that credit and housing market peaks and troughs tend to co-move with each other and are strongly correlated with the financial developments in the overall financial system. Consequently, these variables are better suited to proxy financial cycles (Borio, 2014). Moreover, Adarov (Citation2017) also found that long-term interest rates and share prices are suitable proxies for the financial cycle in addition to credit and asset prices. In the case of South Africa, Farrell and Kemp (Citation2020) found that credit and asset prices were proxies for financial cycles. Nyati et al. (Citation2021) and De Wet and Botha (Citation2022) found that interest rates must also be included as a proxy for financial cycles in South Africa. To make results comparable with other studies (e.g., Claessen et al., Citation2011b; Drehmann et al., Citation2012; Rünstler & Vlekke, Citation2018), the study also uses real GDP to represent the business cycles.
The first step in deriving the financial cycles is to combine financial indicators and then extract the common components. Various methodologies exist in literature to assist with this process, including spectral analysis (Strohsal et al., 2015), dynamic factor modeling (Adarov, Citation2017; Hatzius et al., Citation2010;) and principal components analysis (PCA) (Brave & Butters, Citation2011; Eickmeier et al., Citation2014). Amongst these methodologies, the chief advantage of PCA is that it reduces the curse of dimensionality in the dataset and only focuses on the most relevant features describing the co-movement of variables (Stock & Watson, Citation2011). Consequently, this study follows Brave and Butters (Citation2011) and employs the PCA to construct financial cycles.
The study is interested in the covariances, variances, and correlation of all the financial variables. The information in these variances, covariances, and correlations is crucial to formulating financial cycles. The first step is to look for a linear function of the elements of
financial indicators having maximum variance.
is a vector of
variables
and
denotes transpose, such that:
(1)
(1)
Afterwards, one can look for a linear function uncorrelated with
and having a maximum variance, such that at the
stage, a linear function of
with maximum variance exists and is uncorrelated with
The
th derived variable,
is one of the derived principal components (P.C.s) explaining variations in the three financial variables. There can be an
principal components; however, for the purpose of this study, we focus on the principal components with eigenvalues greater than one to extract financial cycles from financial indicators. The derived principal compoments will represent financial cycle indexes as in Brave and Butters (Citation2011), which could then be used to construct the financial cycles.
Since financial cycles are much like business cycles, this study uses the deviation cycles approach of Lucas (Citation1976) to construct financial cycles. Therefore, a financial index is decomposed into two components: the cyclical and secular components. Voluminous filtering techniques exist in the literature, each with its own attributes. However, the purpose of this study is not to discern between good/or bad techniques. Hence, the discussion of their performance is ignored. The study employs, for comparability purposes, the Hodrick-Prescott filter (HP), which is widely used in the financial cycles literature (Adarov, Citation2017; Drehmann & Yetman, Citation2018; Kuper & Lestano, Citation2007; Nzimande & Ngalawa, Citation2016). The Hodrick-Prescott filter has also been used by other studies to construct financial cycles in South Africa (Bosch and Koch, Citation2020). The HP is the preferred filtering technique for detrending financial variables as suggested by the Basel Committee on Banking Supervision (2010). It has been found to outperform other filtering techniques (Drehmann & Yetman, Citation2018).
For the purposes of the study, assume that a financial index is:
(3)
(3)
Where is the observed series, financial index,
and
is the trend component and cyclical component of the observed series, respectively. It is, moreover, assumed that the secular component is difference stationary, whereas the cyclical component is stationary at levels. An estimate of the trend is obtained by minimizing EquationEquation 4
(4)
(4) ,
(4)
(4)
Where and
are the cyclical and trend components of
respectively.
is the penalty parameter, which is closely related to the smoothness of the estimated trend. The process described in EquationEquation 4
(4)
(4) is used to extract financial cyces from financial indexes. In addition to financial indexes, the H-P Filter is applied to real GDP to extract the business cycle. The penalty parameter is set to
for business cycles, as Hodrick and Prescott (Citation1997) suggested. However, following the literature on financial cycles, the penalty parameter is set to 400,000 (Bosch & Koch, Citation2020; Drehmann et al., Citation2012).
3.2. Dynamic conditional correlation
After financial cycles have been constructed, Dynamic Conditional Correlation (DCC) is employed to address the questions of correlation of financial cycles in South Africa. The choice of the DCC is influenced by its ability to estimate time-varying correlation over other synchronization models, such as simple correlation (Kovacic et al., Citation2017). This feature is consistent with recent literature, which suggests that correlation of economic variables is not static but changes over time (Belke et al., Citation2017; Cerqueira & Martins, Citation2009). The DCC model has been utilized to analyze various aspects of financial markets. In its early stages, the model was used (see Hafner and Reznikova, Citation2012) to analyze the time-varying movements of exchange rates. Billio et al. (Citation2006) used the model to study the connectedness of risk between financers and insurers. The results underscored that there was an interdependence between financial markets. Christoffersen and Errunza (Citation2000) explored the implications of capital mobility and risk management issues using econometric measures derived from the DCC model. In recent years, the model has been used by Ali et al. (Citation2022) to analyze the co-movement of financial markets with cryptocurrency development. Ali et al. (Citation2022) investigated the dynamic correlation of equity markets in Asian economies. These studies demonstrate the versatility of the DCC model in understanding correlations, volatility dynamics, and systemic risk in modern financial markets.
The model used in this study is specified as in Engle (Citation2002), with conditional quasi-correlation parameters that weigh the non-linear combinations of the conditional variances. The DCC approach has convenient and practical advantages. For example, the model ensures that the time-dependent conditional correlation matrix is positive definite for all periods (Engle, Citation2002). Moreover, the number of parameters grows linearly; therefore, the model is relatively parsimonious (Kuper & Lestano, Citation2007). This characteristic of the model will ensure the results are not biased and over-estimated. The study estimates the following model:
(5)
(5)
(6)
(6)
(7)
(7)
(8)
(8)
(9)
(9)
Where is an (m x k) matrix of parameters;
is a (k x 1) vector of independent variables and contains the lags of dependent variables;
is the Cholesky factor of the time-varying conditional covariance matrix
is an
vector of normal, independent, and identically distributed innovations and
is a diagonal matrix of conditional variances:
(10)
(10)
In which evolves according to a univariate GARCH model of the form
by default, where
are ARCH and GARCH parameters, respectively.
is a matric of conditional quasicorrelation,
(11)
(11)
is an m x 1 vector of standardized residuals,
and
are non-negative parameters that govern the dynamics of conditional quasicorrelation and satisfy
If
is stationary, R matrix in (2.8) is a weighted average of the unconditional covariance matrix of the standardized residuals
denoted by
and the unconditional mean of
denoted by
Since
as shown by Aielli (Citation2013),
is neither the unconditional matrix nor the unconditional mean of
For this reason, the parameters in
are known as quasicorrelation (Aielli, Citation2013; Engle, Citation2002).
3.3. Robustness checks: vector error correction mechanism
Finally, the study cast the cycles into a Vector Auto Correction Mechanism (VECM) model to highlight how financial cycles interact. Since Johansen (Citation1988) and Stock and Watson (Citation2011), the VECM has widely been used to assess short and long-run relationships among macroeconomic variables (e.g., Awokuse, Citation2003; Cipamba, Citation2015; Miller & Ratti, Citation2009). They are significantly helpful in identifying long-run co-integrating relationships between these variables (Awokuse, Citation2003). Moreover, the VECM is able to solve the issue of endogeneity; hence it avoids normalizing the cointegrating relations among the variables (Cipamba, Citation2015). Consequently, we proceed in three steps to estimate the VECM. In the first step, we determine if the variables (financial and business cycles) are of the same order of integration through a battery unit root test. Following this, the Johansen (Citation1998) test of cointegration is employed to ascertain if there are long-run relationships among the cycles. Third, The VECM is then estimated to assess the financial and business cycle’s dynamics.
A battery of tests is then used to ensure that the dataset is suitable for the MGARCH DCC VECM techniques. Akaike Information Criteria (AIC), Hannan-Quinn Information Criterion (H.Q.), and Schwarz Information Criterion (SIC), are used to determine the lag ordering of the variables. The Augmented Dickey-Fuller Test (ADF), Philips-Peron Test (P-P), and Kwiatkowski-Phillips-Schmidt-Shin (KPSS) will be used to test for unit roots and determine the order of integration. The Ljung-Box Q Statistics ARCH LM Test will be used to test for autocorrelation.
4. Empirical findings
This section presents the findings of the study. The financial cycles were obtained from the principal component analysis presented in the data and methodology section. From the PCA, two principal components were obtained, satisfying the Kaiser-Meyer-Olkin (KMO) criterion (Pechenizkiy et al., Citation2004) (Appendix A, ). The PCA found that the second component had high loadings on credit and house prices only, in contrast to the first, which had high loadings on all financial indicators (Appendix, ). We label the second component the Credit and Housing Cycle. The first component is labeled the Aggregate Financial Cycle since it explains most variations in all financial indicators. This section commences by presenting summary statistics and diagnostic Analysis for financial and business cycles. Thereafter, the main findings and robustness checks are presented.
4.1. Preliminary analysis
This section concisely presents the statistical properties of the data (refer to ). This is important to highlight the cyclical features of financial cycles. In this section and the following sections, BC refers to the business cycle, while AFC and CHC refer to the Aggregate Financial Cycle and the Credit and Housing Cycle, respectively.
Table 1. Properties of financial and business cycles.
The standard deviations are 0.21, 0.48, and 0.04 for AFC, CHC, and BC, respectively. The standard deviations of financial cycles are larger than the standard deviation of the business cycle, implying that financial cycles are more volatile relative to business cycles, a finding that has already been established in the literature (Drehmann et al., Citation2012). In the context of South Africa, this points to a more unstable financial system characterized by the excessiveness of indebtedness of households, the government and state-owned entities, weak exchange rate, and a steep price level amidst insufficient and unreliable electricity supply. The excessive indebtedness of the government and state-owned entities, coupled with high household debt levels, creates vulnerabilities that could amplify the impact of economic shocks (Mothibi & Mncayi, Citation2019). Additionally, a weak exchange rate and steep price levels contribute to inflationary pressures, further straining household budgets and eroding purchasing power. Moreover, the chronic issue of insufficient and unreliable electricity supply poses a significant constraint on economic activity, hindering productivity and exacerbating the challenges faced by businesses and consumers alike. These factors, together with the findings of the study, paint a picture of heightened financial fragility, with the potential for increased economic volatility and prolonged periods of economic hardship in South Africa.
The means for AFC, CHC, and BC are 3.26E-10, 7.19E-10, and 1E-11, respectively. The size of the means suggests that, for South Africa, financial cycles are larger and ampler in duration than business cycles. This is similar to the finding of Nyati et al. (Citation2021), who found financial cycles to be longer and ampler than business cycles in South Africa. A similar finding was reached by De Wet & Botha (Citation2022). In , financial and business cycles are plotted to trace their movements over time. As expected (Amador-Torres et al., Citation2014; Borio, Citation2014; Claessen et al., Citation2011b) the duration of financial cycle expansions and downturns is longer and ampler than that of business cycles (see red and blue lines). De Wet & Botha (Citation2022), Nyati et al. (Citation2021), Farrell and Kemp (Citation2020), and Bosch and Koch (Citation2020) posit that the business cycle lasts for 7.5 years, while financial cycles last 10–30 years on average in South Africa. Another important observation in is that financial cycle peaks occur at or around a financial crisis period. The figure highlights several significant events that occurred during the time of these peaks. These events include the financial crash caused by COVID-19 in 2019/20, the Euro-debt crisis in 2010, the global financial crisis in 2007/2009, the Dotcom bubble bust in 2002, and the Asian financial crisis in 1997. This observation underscores that, in the case of South Africa, financial cycles are best suited for predicting banking crises and ultimately influence the conduct of macroprudential policies since they are closely associated with episodes of financial turmoil. This is similar to Borio’s (Citation2014) and Oman (Citation2019) findings.
Given the discrepancies in the properties of financial and business cycles, including the differences in their movements, it can be expected that business and financial cycles may not always be synchronized. However, financial cycles demonstrated homogenous features. They are volatile, larger, and ampler than business cycles, and they peaked around episodes of financial distress. This indicates that financial cycles may be more synchronized with each other than they are with business cycles. This cannot, however, be ascertained from eyeball inspection. It requires formal testing, which is done in the subsequent section. displays the scatter plot of financial and business cycles. Despite their differences, financial and business cycles are positively correlated. However, as indicated by the slope of the fitted lines, the correlation between CHC and BC is stronger than between AFC and BC. This supports the initial observation that there can be stronger synchronization between financial cycles than between financial and business cycles. The next sections formally test this synchronization.
4.2. Unit roots and model fit
Employing the DCC requires the series to be stationary and follow a Garch process. below reports two unit root tests. Namely the Augmented Dickey-Fuller test (ADF) and the Philips-Peron test (PP). The ADF and PP test the null hypothesis that a series has unit roots against the alternative hypothesis of no unit roots. The significance of the parameters in the ADF and PP implies that the null hypothesis is rejected. shows that the ADF and PP reject the null hypothesis that financial and business cycles are not stationary. To test whether the cycles follow a GARCH process, we turn to the Ljung-Box Q, which is corroborated by the ARCH LM test.
Table 2. Unit roots results.
The results are reported in . Both tests test the null hypothesis of homoscedasticity against the alternative hypothesis of heteroscedasticity. If the alternative hypothesis is not rejected, then the variable in question follows a GARCH process. The advantage of both tests is that they focus on several lags rather than a single lag. Following the work of Hong and Ramsey (1999), these tests are conducted at lags 12, 24, and 36 lags, respectively, to compare the effectiveness of each test in various lags. shows that the Ljung-Box and the ARCH LM parameters are significant for all the cycles at all selected lag lengths. Therefore, the null hypothesis of homoscedasticity is rejected. Financial and business cycles are heteroscedasticity. Similarly, the Ljung-Box Q and the ARCH LM parameters are significant for the financial cycle. Therefore, both the financial and business cycles follow a GARCH process. The results of the study suggest that business and financial cycles are stationary and follow a garch process. Hence, the study can proceed with estimating the DCC.
Table 3. Autocorrelation results.
4.3. DCC results
This section presents and discusses the DCC results. The lag length is selected using the Akaike Information Criteria (AIC), Hannan-Quinn Information Criterion (H.Q.), and Schwarz Information Criterion (SIC), which suggested one lag. The main results of the DCC are presented in . Panel A of reports the means and variance equations, while panel B reports the correlation parameters and the Wald test. Firstly, a Wald test is conducted to test if there is a relationship between the financial cycles. The Wald test tests the null hypothesis that the parameters on the lags of dependent variables are zero. As demonstrated in , the Wald χ2 test is significant at all levels. Therefore, the study rejects the null hypothesis that the parameters on the lags of the financial cycles are zero and concludes that there is a relationship between the financial cycles. The results of the Wald test provide evidence of synchronization between financial and business cycles in South Africa.
Table 4. Dynamic conditional correlation.
The main reason behind the synchronization of financial cycles is that the South African financial sector is dominated by a few large financial institutions, which means the behavior of a few institutions drives this sector (Financial Sector Conduct Authority, 2023). In particular, in 2013, the banking sectors’, insurers’, and pension funds’ assets to GDP ratios were 77%, 23%, and 83%, respectively. By 2023, the figures were 120%, 68%, and 54%, respectively. In contrast, for the other financial institutions, they were 74% combined. This concentration raises several noteworthy implications for the synchronization of the financial sector in South Africa. Firstly, the behavior of these few institutions holds considerable sway over the broader financial market. Their decisions on lending practices, investment strategies, and risk management can profoundly influence factors such as interest rates, asset prices, and overall market stability. Furthermore, such concentration poses a notable risk in terms of systemic issues. Should any of these major players encounter financial distress, the ramifications could extend throughout the entire financial system, potentially impacting the broader economy. This explains why financial cycles are synchronized in South Africa.
The parameters on the lagged dependent variables are mean relations of dependent variables (Aielli, Citation2013), which are the financial and business cycles in this paper. The parameters provide more insights into the relations between financial and business cycles. The mean for BC is 0.05, whereas it is 0.23 and 0.12 for AFC and CHC, respectively. The mean parameters further confirm prior findings that financial cycles are larger than business cycles (e.g., Drehmann et al., Citation2012). In the Aggregate Financial Cycle and the Credit and Housing Cycle equations, the parameters 0.05 and 0.25 characterize these financial cycles’ mean relations. These values imply a positive link between the financial cycles. The parameters are also statistically significant. This perennial finding implies that the link between financial cycles is significant. The positive association of financial cycles underscores the procyclicality of the South African financial system. This means that, amongst other things, financial segments are susceptible to simultaneous disturbances. This is expected since the South African Financial sector is driven by few financial institutions, as noted above. If these institutions are in distress, the rest of the sector will be in distress.
A typical example of simultaneous distress in South Africa is the small banks crisis that unfolded in South Africa in 2002 and had profound effects on the country’s financial sector. The collapse of several small banks sent shockwaves throughout the sector, leading to increased concerns about stability and trust within the financial system (Hollander & Havemann, Citation2021). Investor confidence plummeted, prompting widespread panic and withdrawals from banks perceived as vulnerable. This crisis highlighted systemic weaknesses in regulatory oversight and risk management practices, prompting authorities to implement stricter regulations and supervision to prevent similar occurrences in the future. Additionally, it underscored the interconnectedness of financial institutions and the potential for contagion, emphasizing the importance of maintaining robust risk management frameworks and ensuring the banking sector’s resilience as a whole (Nkou Mananga et al., Citation2023).
The parameters characterizing the link between financial cycles and the business cycle are statistically insignificant. For example, in the business cycle equation, the parameters 0.01 and 0.02 are positive but not significant. This indicates that financial cycles are less synchronized with business cycles in South Africa. This is expected. In South Africa, business cycles are used to anchor price stability, whereas financial cycles are used to anchor financial stability. The price stability objective is short to medium-term, requiring that the SARB adjusts the repo rate regularly to keep up with business cycle changes. In contrast, the Prudential Authority considers financial stability a long-term objective, in line with the notion of longer and more ampler financial cycles. Due to these factors, the movements of business and financial cycles in South Africa are not homogenous. Financial cycles occur roughly every 10 years, whereas business cycles are roughly seven years (de Wet & Botha, Citation2022). Therefore, these cycles are less synchronized.
also reports the Arch (α) and GARCH (β) parameters. The arch parameters are 0.05 and 0.16 for AFC and CHC, accordingly. The former is not statistically significant, while the latter is significant at all levels. This essential finding implies that only the volatility of credit and housing markets is significant compared to the aggregate financial system. This further confirms that few financial institutions, credit institutions, drive the South African financial sector. This is in line with the literature that suggests that credit and housing markets are significantly helpful in predicting the buildup of financial imbalances in South Africa (Borio, 2014; Claessen et al., Citation2011a; Farrell & Kemp, Citation2020; Oman, Citation2019). Moreover, the arch parameter for the business cycle, 0.11, suggests volatile business cycles. In contrast, the GARCH parameters, 0.85 and 0.88 for AFC and CHC, are both statistically significant at all levels. Their signs imply that good news are likely to affect the volatility of these cycles relative to bad news, while their magnitude implies that the volatility of financial cycles is highly persistent. This is also the case for the business cycle (see ).
The results of the quasi-condititional correlations of financial cycles from the DCC are also reported in . The correlation between the AFC and CHC is 0.41, whereas it is only 0.05 and 0.02 between AFC and BC and CHC and BC. The magnitude of correlation coefficients further provides evidence of more synchronization between financial cycles. The parameters, 0.03 and 0.16, are constraints on correlation values, and combined they lie between zero and one indicating that the correlation parameters are true and stable. plots the time-varying quasi-conditional correlations to assess whether the financial cycle correlation varies with time. The figure shows that correlation varies with time. In particular, in the years around the global financial crisis, correlation between financial cycles increased sharply (see , top left-hand corner). This finding is supported by , which plots the conditional variances. Indeed, the figure shows that the covariance of financial cycles increased sharply in the years around the global financial crisis. In contrast, financial cycles are weakly correlated with the business cycle, especially since the global financial crisis (see ). Moreover, shows a weaker covariance between business and financial cycles.
Figure 3. Conditional quasi-correlation of financial and business cycles. Source: Authors’ estimates.

The sharp increase in synchronization during a financial downfall is due to the contagion effects. During a crisis, South Africa experiences the contagion effects through various interconnected channels. Firstly, disruptions in major financial centers can swiftly transmit to South Africa as an integral part of global financial markets, inducing volatility in local financial markets (Claessens & Kose, Citation2018; Ncube et al., Citation2016). Secondly, the country’s economy is closely tied to global trade flows, making it susceptible to reduced demand for exports in key trading partners during crises. Thirdly, South Africa relies on foreign capital inflows, which can reverse during times of crisis as global investors withdraw investments from emerging markets, leading to currency depreciation and pressure on domestic interest rates (Makrelov et al., Citation2022). Moreover, the interconnectedness of South African financial institutions with global networks means that distress in global financial institutions can spill over, causing liquidity shortages and systemic risks domestically. Additionally, fluctuations in global commodity prices impact South Africa’s export revenues and government finances, further exacerbating economic vulnerabilities. Furthermore, policy responses from major central banks and international financial institutions play a crucial role in mitigating contagion risks, highlighting the importance of coordinated monetary policy actions and financial support measures to stabilize South Africa’s economy during crises (Borio et al., 2020; Kabundi and Rapapali, Citation2019).
4.4. VECM results
The previous section underscored that the movement of financial cycles in South Africa is characterized by a strong level of synchronization, which is high around a crisis period. If this relationship is disturbed, how long does it take for financial cycles to revert to this relationship? How do financial cycles respond to each other’s shocks? This section presents the VECM results, which address these questions. Since unit-roots have already been tested and the variables were found to be stationary, we proceeded to test for cointegration. presents the Johansen cointegration test, which tests the null hypothesis of no cointegration against the alternative hypothesis of cointegration. In , the trace statistics at rank zero and one exceed their critical values of 15.41 and 3.76, respectively. The trace statistics are 129.42 and 48.49, respectively. Therefore, the null hypothesis of no cointegration is rejected. This implies that there are at least two cointegrating relationships between the financial cycles, suggesting a long-run link. This finding is important as two long-run relationships highlight the synchronization of financial cycles in South Africa.
Table 5. Cointegration of financial and business cycles.
Next, the paper analyzed the long-run dynamics of financial cycles. The lagged independent variables in represent the long-run dynamics, while the error correction (E.C. terms) captures the speed of adjustment back to equilibrium. The error terms in the Aggregate Financial Cycle, Credit, and Housing Cycle are −0.76 and −0.77. The signs of the parameters suggest that there is a long-run relationship between the financial cycles. The magnitudes of the parameters are closer to 1, meaning a faster adjustment to equilibrium. This implies that when one financial cycle expands, the other financial cycle catches up faster. By contrast, the E.C. for the business cycle is marginal but significant. This error term is 0.06, meaning that when the financial cycles are expanding, the business cycle adjusts slowly but significantly.
Table 6. VECM short and long-run dynamics.
In South Africa, the existence of a long-run relationship between financial markets can be attributed to various factors, including economic integration, globalization, and financial liberalization. Economic theory posits that in an interconnected global economy, financial markets tend to exhibit co-movement over the long term due to the transmission of shocks and the integration of capital flows. In the case of South Africa, the country’s increasing integration into global financial markets has facilitated the transmission of information, capital, and risk across different segments of the financial system (Grossman & Stiglitz, Citation1980). One significant driver of the long-run relationship between financial markets in South Africa is financial liberalization. Following the dismantling of apartheid-era financial controls in the 1990s, South Africa embarked on a path of financial deregulation and liberalization, opening up its economy to foreign investment and capital flows (Isaacs & Kaltenbrunner, Citation2018). This liberalization process has led to greater interconnectedness between domestic financial markets and global markets, as evidenced by the increased cross-listing of South African companies on international exchanges and the growing presence of foreign investors in domestic capital markets (Temouri et al., Citation2016).
Moreover, developing sophisticated financial instruments and expanding financial intermediaries have further facilitated the transmission of shocks and the co-movement of financial markets in South Africa (Bekaert et al., Citation2011). For instance, the emergence of derivative markets has enabled investors to hedge risks and arbitrage opportunities across different asset classes, leading to increased correlations between equity, bond, and currency markets. Additionally, macroeconomic factors such as inflation, interest rates, and exchange rates play a crucial role in shaping the long-run relationship between financial markets in South Africa (Ho, Citation2019). Changes in these macroeconomic variables can affect investor sentiment, asset prices, and portfolio allocations, thereby influencing the co-movement of financial markets over time (Makau, Citation2021).
In the financial cycle’s equations in , the parameters in the lagged dependent variables are all positive but statistically significant except for the statistically insignificant business cycle (see ; first and the second columns). This finding further supports the DCC findings that a significant positive link exists between financial cycles. This is in line with the notion that financial cycles are 'self-reinforcing’ interactions that can lead to booms and bursts in the financial system (Borio, 2014). In the Business cycle equation in , however, all lagged parameters are statistically significant and positive (see ; third column). This suggests that financial cycles have a significant impact on the business cycle. This is in line with Claessen et al. (Citation2011b), Amador-Torres et al. (Citation2014), and Gomez-Gonzalez et al. (Citation2015).
This study finds that a shock in the Credit and Housing Cycle triggers positive reaction in the Aggregate Financial Cycle. Likewise, a shock in the Aggregate Financial Cycle triggers a positive response in the Credit and Housing Cycle. These finding further demonstrates the procyclicality in the South African financial sector. Procyclicality in the South African financial cycle reflects the tendency of financial variables to amplify economic fluctuations, exacerbating both booms and busts (G. D. Liu & Seeiso, Citation2012). Several factors contribute to this phenomenon, including the procyclical behavior of credit, asset prices, and risk-taking behavior within the financial system (Bernanke et al., Citation1994). One key driver of procyclicality in South Africa’s financial cycle is the behavior of credit. During economic upswings, banks tend to increase lending activities, extending credit to households and businesses at a faster pace (Claessens et al., Citation2011). This credit expansion fuels investment and consumption, driving economic growth further. However, during downturns, banks often tighten lending standards and reduce credit supply, exacerbating the economic slowdown (Fostel & Geanakoplos, Citation2008). This procyclical behavior of credit amplifies fluctuations in economic activity, contributing to the volatility of the financial cycle.
Asset prices also exhibit procyclical tendencies in South Africa’s financial cycle. During periods of economic expansion, asset prices tend to rise as investors become more optimistic about future returns (Reinhart & Rogoff, Citation2009). This can lead to speculative bubbles in asset markets, such as housing or equity markets, where prices detach from underlying fundamentals (Shiller, Citation2000). However, when economic conditions deteriorate, asset prices can experience sharp declines, triggering financial distress and exacerbating the downturn (Borio & Lowe, Citation2002). This procyclical behavior of asset prices amplifies volatility in the financial system, contributing to the synchronization of economic and financial cycles. Moreover, the procyclical nature of risk-taking behavior among financial market participants exacerbates fluctuations in South Africa’s financial cycle. During periods of economic expansion, investors tend to take on more risk, seeking higher returns in riskier assets (Adrian & Shin, Citation2010). This behavior can lead to the buildup of leverage and the accumulation of systemic risks within the financial system (Brunnermeier and Sannikov, Citation2014). However, when economic conditions deteriorate, risk aversion increases, prompting investors to deleverage and reduce exposure to risky assets (Caballero and Krishnamurthy, Citation2009). This procyclical behavior of risk-taking exacerbates financial instability, amplifying the impact of economic downturns.
5. Summary and conclusion
The study investigated the synchronization and dynamics of financial cycles in South Africa using data for the period 1975q1-2023q4. Data descriptive statistics demonstrated that financial cycles, represented by the Aggregate Financial Cycle and Credit and Housing Cycle (CHC), exhibit higher volatility and longer duration compared to the business cycle. These findings suggested that financial cycles are more synchronized with each other than with the business cycle, suggesting homogeneity in their features. A positive correlation exists between financial and business cycles, but financial cycles demonstrate stronger synchronization with each other. Financial cycle peaks often coincide with episodes of financial distress, such as financial crises, indicating their predictive power for banking crises and financial turmoil in South Africa.
Employing Dynamic Conditional Correlation (DCC) analysis, the study confirms the presence of synchronization between financial cycles. This is similar to other studies, for instance, Drehmann et al. (Citation2012), Borio (2014), Adarov (Citation2017), and Oman (Citation2019), among others. Financial cycles in South Africa are characterized by a strong level of synchronization, particularly around crisis periods, indicating a systemic interdependence among financial markets. Given the synchronization and systemic interdependence of financial cycles, policymakers should focus on enhancing macroprudential policies to mitigate systemic risks and ensure financial stability at the level of the overall financial system. Regulatory authorities should closely monitor the behavior of major financial institutions, as they exert significant influence over the financial system, and implement measures to prevent systemic issues. The findings emphasize the importance of maintaining robust risk management frameworks and regulatory oversight to prevent financial crises and ensure the resilience of the banking sector.
The South African Reserve Bank (SARB) should take into account the interaction between financial and business cycles when formulating monetary policy. During periods of financial distress or heightened synchronization among financial cycles, central banks may need to adopt accommodative monetary measures to support financial stability and prevent systemic risks. Regulatory authorities, including the Prudential Authority in South Africa, should enhance macroprudential policies to address systemic risks arising from the synchronization of financial cycles. Macroprudential measures should focus on monitoring and regulating the behavior of major financial institutions to prevent the buildup of excessive leverage, credit imbalances, and asset price bubbles. Stress testing and scenario analysis should be conducted regularly to assess the resilience of the financial system to potential shocks and identify areas of vulnerability.
Policymakers should consider implementing long-term structural reforms to address underlying vulnerabilities in the financial system, such as concentration risk and interconnectedness among financial institutions. Measures to promote competition and diversity in the financial sector, including fostering the growth of small and medium-sized financial institutions, can enhance market resilience and reduce systemic risks. Overall, the findings highlight the importance of proactive and coordinated policy responses to address the synchronization of financial cycles and promote financial stability in South Africa. By implementing appropriate monetary and macroprudential measures and undertaking structural reforms, policymakers can mitigate systemic risks and safeguard the resilience of the financial system against future crises.
The study on the synchronization and dynamics of financial cycles in South Africa provides valuable insights, but it also has several limitations that warrant consideration. Firstly, the Analysis relies on data spanning from 1975q1 to 2023q4, which may not fully capture recent developments or structural changes in the financial system. Additionally, while the study identifies synchronization between financial cycles using Dynamic Conditional Correlation (DCC) Analysis, it may not account for all potential drivers of synchronization, such as international spillover effects or changes in regulatory environments. Moreover, the study’s focus on South Africa limits the generalizability of its findings to other countries or regions with different economic and financial characteristics.
In terms of future research directions, there are several avenues to explore. Firstly, conducting a more granular analysis of the drivers of financial cycles, including factors such as credit growth, asset prices, and regulatory changes, could provide deeper insights into the dynamics of financial cycles and their synchronization. Additionally, exploring the implications of financial cycle synchronization for different sectors of the economy, such as the real estate market or the corporate sector, could enhance our understanding of the transmission channels of systemic risk. Furthermore, incorporating measures of uncertainty and sentiment into the Analysis could help to better capture the psychological aspects of financial cycles and their impact on market dynamics. Future research could also focus on evaluating the effectiveness of macroprudential policies in mitigating systemic risks associated with financial cycle synchronization. By conducting empirical assessments of policy interventions, policymakers can identify the most effective measures for safeguarding financial stability and preventing future crises. Moreover, exploring the role of central bank communication and forward guidance in influencing market expectations and dampening excessive financial cycle synchronization could provide valuable insights for policymakers.
In summary, while the study sheds light on the synchronization of financial cycles in South Africa and its implications for financial stability, there remain important limitations and opportunities for future research. By addressing these limitations and exploring new research avenues, scholars can contribute to a deeper understanding of financial cycle dynamics and inform more effective policy responses to safeguard financial stability.
Acknowledgements
The authors would like to thank Precious Mncayi for her valuable comments which improved the quality of this work.
Disclosure statement
No potential conflict of interest was reported by the author(s).
Data availability statement
Data is available from authors on request.
Additional information
Funding
Notes on contributors
Khwazi Magubane
Khwazi Magubane is a dedicated researcher specializing in economic trends and policy implications. His work primarily focuses on analyzing financial cycles and systemic risks in emerging economies. This paper is an extension of his broader research agenda, examining the synchronization of financial cycles in South Africa. By investigating these dynamics, He contributes valuable insights to the discourse on economic stability and resilience in the region. This research aligns with his overarching goal of informing policymakers and stakeholders about the interconnectedness of financial systems. Through evidence-based analysis, Khwazi Magubane advocates for proactive measures to mitigate risks and promote sustainable economic development. His commitment to advancing knowledge in economics underscores his dedication to shaping informed policy decisions.
References
- Adarov, A. (2017). Financial cycles in credit, housing and capital markets: Evidence from systemic economies. Tech. rep., WIIW Working Paper.
- Adrian, T., & Shin, H. S. (2010). Liquidity and leverage. Journal of Financial Intermediation, 19(3), 418–437. https://doi.org/10.1016/j.jfi.2008.12.002
- Agénor, P. R., & da Silva, L. A. (2013). Inflation targeting and financial stability: A perspective from the developing world. Inter-American Development Bank.
- Agenor, P. R., & Da Silva, P. (2019). Integrated inflation targeting: Another perspective from the developing world. Bank of International Settlements.
- Aielli, G. P. (2013). Dynamic conditional correlation: On properties and estimation. Journal of Business & Economic Statistics, 31(3), 282–299. https://doi.org/10.1080/07350015.2013.771027
- Aikman, D., Giese, J., Kapadia, S., & McLeay, M. (2019). Targeting financial stability: Macroprudential or monetary policy? European Central Bank.
- Alcidi, C. (2017). Fiscal policy stabilisation and the financial cycle in the Euro area. Tech. rep., Directorate General Economic and Financial Affairs (DG ECFIN), European.
- Alfaro, L., Kalemli-Ozcan, S., & Volosovych, V. (2007). Capital flows in a globalized world: The role of policies and institutions. In Capital controls and capital flows in emerging economies: Policies, practices, and consequences (pp. 19–72). University of Chicago Press.
- Ali, F., Bouri, E., Naifar, N., Shahzad, S. J., & AlAhmad, M. (2022). An examination of whether gold-backed Islamic cryptocurrencies are safe havens for international Islamic equity markets. Research in International Business and Finance, 63, 101768. https://doi.org/10.1016/j.ribaf.2022.101768
- Amador-Torres, J. S., Gaitán-Maldonado, C., Gómez-González, J. E., Villamizar-Villegas, M., & Zárate-Solano, H. M. (2014). Credit and business cycles: An empirical analysis in the frequency domain. Borradores de Economía. No. 843.
- Angelini, P., Neri, S., & Panetta, F. (2014). The interaction between capital requirements and monetary policy. Journal of Money, Credit and Banking, 46(6), 1073–1112. https://doi.org/10.1111/jmcb.12134
- Awokuse, T. O. (2003). Is the export-led growth hypothesis valid for Canada? Canadian Journal of Economics/Revue Canadienne D'économique, 36(1), 126–136. https://doi.org/10.1111/1540-5982.00006
- Bakang, M. (2015). Effects of financial deepening on economic growth in Kenya. International Journal of Business and Commerce, 4(7), 1–50.
- Beck, T. (2008). The econometrics of finance and growth. The World Bank.
- Beck, T., & Levine, R. (2004). Stock markets, banks, and growth: Panel evidence. Journal of Banking & Finance, 28(3), 423–442. https://doi.org/10.1016/S0378-4266(02)00408-9
- Beirne, J. (2020). Financial cycles in asset markets and regions. Economic Modelling, 92, 358–374. https://doi.org/10.1016/j.econmod.2020.01.015
- Bekaert, G., Harvey, C. R., Lundblad, C. T., & Siegel, S. (2011). What segments equity markets? Review of Financial Studies, 24(12), 3841–3890. https://doi.org/10.1093/rfs/hhr082
- Belke, A., Domnick, C., & Gros, D. (2017). Business cycle synchronization in the EMU: Core vs. periphery. Open Economies Review, 28(5), 863–892. https://doi.org/10.1007/s11079-017-9465-9
- Bemanke, B., & Gertler, M. (1989). Agency costs, net worth, and business fluctuations. American Economic Review, 79, 14–31.
- Bernanke, B., Gertler, M., & Gilchrist, S. (1994). The financial accelerator and the flight to quality. Tech. rep., National Bureau of Economic Research.
- Billio, M., Caporin, M., & Gobbo, M. (2006). Flexible dynamic conditional correlation multivariate Garch models for asset allocation. Applied Financial Economics Letters, 2(2), 123–130. https://doi.org/10.1080/17446540500428843
- Boar, C., Gambacorta, L., Lombardo, G., & Pereira da Silva, L. A. (2017). What are the effects of macroprudential policies on macroeconomic performance? BIS Quarterly Review. https://www.bis.org/publ/qtrpdf/r_qt1709g.pdf
- Bordo, M. D. (2009). The crisis of 2007: The same old story, only the players have changed. In Globalization and systemic risk (pp. 39–50). World Scientific.
- Borio, C. (2014). The financial cycle and macroeconomics: What have we learnt?. Journal of Banking & Finance, 45, 182–198. 10.1016/j.jbankfin.2013.07.031
- Borio, C. E., Drehmann, M., & Xia, F. D. (2018). The financial cycle and recession risk. BIS Quarterly Review. https://www.bis.org/publ/qtrpdf/r_qt1812g.pdf
- Borio, C. E., Lombardi, M. J., & Zampolli, F. (2016). Fiscal sustainability and the financial cycle. https://www.bis.org/publ/work552.pdf
- Borio, C., & Lowe, P. (2002). Assessing the risk of banking crises. BIS Quarterly Review, 7, 43–54.
- Borio, C., Furfine, C., & Lowe, P. (2001). Procyclicality of the financial system and financial stability: Issues and policy options. BIS Papers, 1, 1–57.
- Bosch, A., &Koch, S. F. (2020). The South African financial cycle and its relation to household deleveraging. South African Journal of Economics, 88, 145–173. 210.1111/saje.12245
- Brave, S. A., & Butters, R. (2011). Monitoring financial stability: A financial conditions index approach. Economic Perspectives, 35, 22.
- Brunnermeier, M. K., &Sannikov, Y. (2014). A macroeconomic model with a financial sector. American Economic Review, 104, 379–421. 210.1257/aer.104.2.379
- Bry, G., &Boschan, C. (1971). Standard business cycle analysis of economic time series. In Cyclical Analysis of Time Series: Selected Procedures and Computer Programs. NBEr,
- Bry, G., &Boschan, C. Standard business cycle analysis of economic time series. In Cyclical Analysis of Time Series: Selected Procedures and Computer Programs
- Caballero, R. J., &Krishnamurthy, A. (2009). Global imbalances and financial fragility. American Economic Review, 99, 584–588. 210.1257/aer.99.2.584
- Cagliarini, A., & Price, F. (2017). Exploring the link between the macroeconomic and financial cycles [Paper presentation]. RBA Annual Conference Volume (Discontinued).
- Cerqueira, P. A., & Martins, R. (2009). Measuring the determinants of business cycle synchronization using a panel approach. Economics Letters, 102(2), 106–108. https://doi.org/10.1016/j.econlet.2008.11.016
- Cerutti, E., Claessens, S., & Rose, A. K. (2017). How important is the global financial cycle. Evidence from Capital Flows. CEPR Discussion Papers, 12075.
- Cerutti, M. E., Correa, M. R., Fiorentino, E., & Segalla, E. (2016). Changes in prudential policy instruments—A new cross-country database. International Monetary Fund. https://doi.org/10.5089/9781475574517.001
- Christoffersen, P., & Errunza, V. (2000). Towards a global financial architecture: Capital mobility and risk management issues. Emerging Markets Review, 1(1), 3–20. https://doi.org/10.1016/S1566-0141(00)00002-9
- Cipamba, P. W. (2015). The export-output relationship in South Africa: An empirical investigation. Studies in Economics and Econometrics, 39, 25–46.
- Claessens, S.,Kose, M. A., &Terrones, M. E. (2011). Financial Cycles: What? How? When?. NBER International Seminar on Macroeconomics, 7(1), 303–344. 10.1086/658308
- Claessens, S., & Kose, M. A. (2018). Frontiers of macrofinancial linkages. BIS Paper.
- Claessens, S., Kose, M. A., & Terrones, M. E. (2011a). Recessions and financial disruptions in emerging markets: A bird’s eye view. Series on Central Banking Analysis and Economic Policies No. 16.
- Claessens, S., Kose, M. A., & Terrones, M. E. (2011b). Financial cycles: What? How? When? NBER International Seminar on Macroeconomics, 7(1), 303–344. https://doi.org/10.1086/658308
- Cochrane, J. H. (2005). The risk and return of venture capital. Journal of Financial Economics, 75(1), 3–52. 10.1016/j.jfineco.2004.03.006
- De Backer, B. R., Dewachter, H. A., Guarda, P. A., Haavio, M. A., Hindrayanto, I. R., & Iskrev, N. I. (2018). Real and financial cycles in EU countries: Stylised facts and modelling implications. https://www.ecb.europa.eu/pub/pdf/scpops/ecb.op205.en.pdf
- De Wet, M. C., & Botha, I. (2022). Constructing and characterising the aggregate South African financial cycle: A Markov regime-switching approach. Journal of Business Cycle Research, 18(1), 37–67. https://doi.org/10.1007/s41549-022-00064-y
- Dlamini, L., &Ngalawa, H. (2022). Macroprudential policy and house prices in an estimated Dynamic Stochastic General Equilibrium model for South Africa. Australian Economic Papers, 61, 304–336. 210.1111/1467-8454.12249
- Drehmann, M., & Yetman, J. (2018). Why you should use the Hodrick-Prescott filter–At least to generate credit gaps. https://www.bis.org/publ/work744.pdf
- Drehmann, M., Borio, C. E., & Tsatsaronis, K. (2012). Characterising the financial cycle: don’t lose sight of the medium term! https://www.bis.org/publ/work380.pdf
- Eickmeier, S., Gambacorta, L., & Hofmann, B. (2014). Understanding global liquidity. European Economic Review, 68, 1–18. https://doi.org/10.1016/j.euroecorev.2014.01.015
- Engle, R. (2002). Dynamic conditional correlation: A simple class of multivariate generalized autoregressive conditional heteroskedasticity models. Journal of Business & Economic Statistics, 20(3), 339–350. https://doi.org/10.1198/073500102288618487
- English, W., Tsatsaronis, K., & Zoli, E., (2005). Assessing the predictive power of measures of financial conditions for macroeconomic variables. BIS Papers, 22, 228–252.
- Farrell, G., & Kemp, E. (2020). Measuring the financial cycle in South Africa. SAJE, 88(2), 123–144.
- Fisher, I. (1933). The debt-deflation theory of great depressions. Econometrica, 1(4), 337–357. https://doi.org/10.2307/1907327
- Fostel, A., & Geanakoplos, J. (2008). Leverage cycles and the anxious economy. American Economic Review, 98(4), 1211–1244. https://doi.org/10.1257/aer.98.4.1211
- Frieden, J. A. (1991). Invested interests: the politics of national economic policies in a world of global finance. International Organization, 45(4), 425–451. 10.1017/S0020818300033178
- Gelain, P., & Ilbas, P. (2017). Monetary and macroprudential policies in an estimated model with financial intermediation. Journal of Economic Dynamics and Control, 78, 164–189. https://doi.org/10.1016/j.jedc.2017.01.010
- Gertler, M., & Karadi, P. (2011). A model of unconventional monetary policy. Journal of Monetary Economics, 58(1), 17–34. https://doi.org/10.1016/j.jmoneco.2010.10.004
- Gertler, M., & Kiyotaki, N. (2015). Banking, liquidity, and bank runs in an infinite horizon economy. American Economic Review, 105(7), 2011–2043. https://doi.org/10.1257/aer.20130665
- Goldsmith, R. W. (1969). Financial structure and development. Yale University Press.
- Gomez-Gonzalez, J. E., Villamizar-Villegas, M., Zarate, H. M., Amador, J. S., & Gaitan-Maldonado, C. (2015). Credit and business cycles: Causal effects in the frequency domain. Ensayos Sobre Política Económica, 33(78), 176–189. https://doi.org/10.1016/j.espe.2015.05.002
- Gorton, G., & Ordonez, G. (2020). Good booms, bad booms. Journal of the European Economic Association, 18(2), 618–665. https://doi.org/10.1093/jeea/jvy058
- Grossman, S. J., & Stiglitz, J. E. (1980). On the impossibility of informationally efficient markets. The American Economic Review, 70, 393–408.
- Gurley, J. G., & Shaw, E. S. (1955). Financial aspects of economic development. The American Economic Review, 45, 515–538.
- Hafner, C. M., &Reznikova, O. (2012). On the estimation of dynamic conditional correlation models. Computational Statistics & Data Analysis, 56(11), 3533–3545. 201210.1016/j.csda.2010.09.022
- Harding, D., & Pagan, A. (2006). Synchronization of cycles. Journal of Econometrics, 132(1), 59–79. https://doi.org/10.1016/j.jeconom.2005.01.023
- Harun, C. A., Taruna, A. A., Nattan, R. R., & Surjaningsih, N. (2014). Financial cycle of Indonesia–Potential forward looking analysis. https://publication-bi.org/repec/idn/wpaper/WP092014.pdf
- Hatzius, J., Hooper, P., Mishkin, F. S., Schoenholtz, K. L., & Watson, M. W. (2010). Financial conditions indexes: A fresh look after the financial crisis. Tech. rep. National Bureau of Economic Research.
- Hiebert, P.,Jaccard, I., &Schüler, Y. (2018). Contrasting financial and business cycles: Stylized facts and candidate explanations. Journal of Financial Stability, 38, 72–80. 10.1016/j.jfs.2018.06.002
- Ho, S.-Y. (2019). Macroeconomic determinants of stock market development in South Africa. International Journal of Emerging Markets, 14(2), 322–342. https://doi.org/10.1108/IJoEM-09-2017-0341
- Hodrick, R. J., & Prescott, E. C. (1997). Postwar US business cycles: An empirical investigation. Journal of Money, Credit and Banking, 29(1), 1–16. https://doi.org/10.2307/2953682
- Hollander, H., & Havemann, R. (2021). South Africa’s 2003–2013 credit boom and bust: Lessons for macroprudential policy. Economic History of Developing Regions, 36(2), 339–365. https://doi.org/10.1080/20780389.2021.1938532
- Hubrich, K., d‘Agostino, A., Cervena, M., Ciccarelli, M., Guarda, P., & Haavio, M.. (2013). Financial shocks and the macroeconomy: Heterogeneity and non-linearities. ECB Occasional Paper.
- Isaacs, G., & Kaltenbrunner, A. (2018). Financialization and liberalization: South Africa’s new forms of external vulnerability. Competition & Change, 22(4), 437–463. https://doi.org/10.1177/1024529418788375
- Johansen, S. (1988). Statistical analysis of cointegration vectors. Journal of Economic Dynamics and Control, 12(2-3), 231–254. 10.1016/0165-1889(88)90041-3
- Juselius, M., Borio, C. E., Disyatat, P., & Drehmann, M. (2016). Monetary policy, the financial cycle and ultralow interest rates. Bank of Finland Research Discussion Paper.
- Kabundi, A., & Rapapali, M. (2019). The transmission of monetary policy in South Africa before and after the global financial crisis. South African Journal of Economics, 87(4), 464–489. https://doi.org/10.1111/saje.12238
- Keynes, J. M. (1964). The general theory of employment, interest and money (1936). The Collected Writings of John Maynard Keynes, 7, 1971–1979.
- Kim, S., & Mehrotra, A. N. (2017). Effects of monetary and macro-prudential policies–Evidence from inflation targeting economies in the Asia-Pacific region and potential implications for China. BOFIT Discussion Paper No. 4/2017
- Kiyotaki, N., & Moore, J. (1997). Credit chains. Edinburgh School of Economics. Discussion Papers, 118.
- Kovacic, Z., Vilotic, M., Scholes, V., Peiris, I., Lee, L., & Huang, G. (2017). Assessing European business cycles synchronization. https://ideas.repec.org/p/pra/mprapa/79990.html
- Kunovac, D., Mandler, M., & Scharnagl, M. (2018). Financial cycles in euro area economies: A cross-country perspective. https://www.econstor.eu/bitstream/10419/264534/1/OBES_OBES12481.pdf
- Kuper, G. H. & Lestano (2007). Dynamic conditional correlation analysis of financial market interdependence: An application to Thailand and Indonesia. Journal of Asian Economics, 18(4), 670–684. https://doi.org/10.1016/j.asieco.2007.03.007
- Liu, G. D., & Seeiso, N. E. (2012). Basel II procyclicality: The case of South Africa. Economic Modelling, 29(3), 848–857. https://doi.org/10.1016/j.econmod.2011.10.015
- Lucas, R. E. (1976). Econometric policy evaluation: A critique. Carnegie-Rochester Conference Series on Public Policy, 1, 19–46. https://doi.org/10.1016/S0167-2231(76)80003-6
- Ma, Y., & Zhang, J. (2016). Financial cycle, business cycle and monetary policy: Evidence from four major economies. International Journal of Finance & Economics, 21(4), 502–527. https://doi.org/10.1002/ijfe.1566
- Makau, M. U. (2021). Macro risk factors, investor sentiments and performance of equity market at Nairobi securities exchange, Kenya [Ph.D. dissertation]. KENYATTA UNIVERSITY.
- Makrelov, K., Davies, R., & Harris, L. (2022). The impact of capital flow reversal shocks in South Africa: a stock-and-flow-consistent analysis. In Capitalism: An unsustainable future? (pp. 145–171). Routledge.
- Mandler, M., & Scharnagl, M. (2019). Financial cycles across G7 economies: A view from wavelet analysis. The Journal of Economic Asymmetries, 26, e00277.
- Merler, S. (2015). Squaring the cycle: Financial cycles, capital flows and macroprudential policy in the euro area. Tech. rep., Bruegel Working Paper.
- Milan, C., & Botha, I. (2019). Characterising cycles exhibited by important financial sections in the South African economy. Journal of Economic and Financial Sciences, 12(1), 1–18. https://doi.org/10.4102/jef.v12i1.433
- Miller, J. I., & Ratti, R. A. (2009). Crude oil and stock markets: Stability, instability, and bubbles. Energy Economics, 31(4), 559–568. https://doi.org/10.1016/j.eneco.2009.01.009
- Minsky, H. P. (1977). The financial instability hypothesis: An interpretation of Keynes and an alternative to “standard” theory. Challenge, 20(1), 20–27. https://doi.org/10.1080/05775132.1977.11470296
- Minsky, H. P. (1982). The financial-instability hypothesis: Capitalist processes and the behavior of the economy. https://www.levyinstitute.org/pubs/wp74.pdf
- Mishkin, F. S., & Bank, R. (2011). How should central banks respond to asset-price bubbles? The ‘lean’ versus ‘clean’ debate after the GFC. https://www.rba.gov.au/publications/bulletin/2011/jun/pdf/bu-0611-8.pdf
- Mothibi, L., &Mncayi, P. (2019). Investigating the key drivers of government debt in South Africa: A post-apartheid analysis. International Journal of Ebusiness and Egovernment Studies, 11(1), 16–33.
- Ncube, M., Ndou, E., & Gumata, N. (2016). Global growth and financial spillovers and the South African macro-economy. Springer.
- Nkou Mananga, P., Lin, S., & Zhang, H. (2023). A network approach to interbank contagion risk in South Africa. https://www.resbank.co.za/content/dam/sarb/publications/working-papers/2023/WP%202309.pdf
- Nyati, M. C., Tipoy, C. K., & Muzindutsi, P.-F. (2021). Measuring and testing a modified version of the South African financial cycle. Tech. rep., Economic Research Southern Africa.
- Nzimande, N. P., & Ngalawa, H. (2016). Is there a SADC business cycle? Evidence from a dynamic factor model. Tech. rep. https://saef.ukzn.ac.za/wp-content/uploads/2018/05/SAEF-Is-there-a-SADC-Business-Cycle-Evidence-from-a-Dynamic-Factor-Model.pdf
- Oman, W. (2019). The synchronization of business cycles and financial cycles in the euro area. International Journal of Central Banking, 15, 327–362.
- Pahla, Z. M. (2019). The determinants of the South African financial cycle. University of Johannesburg (South Africa.
- Paoli, B. D., & Paustian, M. (2017). Coordinating monetary and macroprudential policies. Journal of Money, Credit and Banking, 49(2–3), 319–349. https://doi.org/10.1111/jmcb.12381
- Parusel, M., & Viegi, N. (2009). Economic policy in turbulent times. http://www.nviegi.net/research/crises.pdf
- Pechenizkiy, M., Tsymbal, A., & Puuronen, S. (2004). PCA-based feature transformation for classification: issues in medical diagnostics [Paper presentation]. Proceedings. 17th IEEE Symposium on Computer-Based Medical Systems (pp. 535–540). https://doi.org/10.1109/CBMS.2004.1311770
- Peltonen, T. (2017). Coherent financial cycles for G-7 countries: Why extending credit can be an asset. https://www.esrb.europa.eu/pub/pdf/wp/esrbwp43.en.pdf
- Poggio, T., Lo, A. W., LeBaron, B., & Chan, N. T. (2001). Agent-based models of financial markets: A comparison with experimental markets. https://papers.ssrn.com/sol3/papers.cfm?abstract_id=290140.
- Reinhart, C. M., & Rogoff, K. S. (2009). The aftermath of financial crises. American Economic Review, 99(2), 466–472. https://doi.org/10.1257/aer.99.2.466
- Richter, B., Schularick, M., & Shim, I. (2018). The macroeconomic effects of macroprudential policy. https://www.bis.org/publ/work740.pdf
- Rünstler, G., & Vlekke, M. (2018). Business, housing, and credit cycles. Journal of Applied Econometrics, 33(2), 212–226. https://doi.org/10.1002/jae.2604
- Schularick, M., & Taylor, A. M. (2012). Credit booms gone bust: Monetary policy, leverage cycles, and financial crises, 1870-2008. American Economic Review, 102(2), 1029–1061. https://doi.org/10.1257/aer.102.2.1029
- Schumpeter, J. The theory of economic development. Routledge (1911).
- Shiller, R. J. (2000). Measuring bubble expectations and investor confidence. Journal of Psychology and Financial Markets, 1(1), 49–60. https://doi.org/10.1207/S15327760JPFM0101_05
- Stock, J. H., & Watson, M. (2011). Dynamic factor models. Oxford Handbooks Online.
- Stremmel, H. (2015). Capturing the financial cycle in Europe. https://www.ecb.europa.eu/pub/pdf/scpwps/ecbwp1811.en.pdf
- Stremmel, H., & Zsámboki, B. (2015). The relationship between structural and cyclical features of the EU financial sector. https://www.ecb.europa.eu/pub/pdf/scpwps/ecbwp1812.en.pdf
- Sukati, M. (2013). Cointegration analysis of oil prices and consumer price index in South Africa using STATA software. https://ideas.repec.org/p/pra/mprapa/49797.html
- Temouri, Y., Driffield, N., & Bhaumik, S. K. (2016). A strategic perspective of cross-listing by emerging market firms: evidence from Indonesia, Mexico, Poland and South Africa. Journal of International Management, 22(3), 265–279.