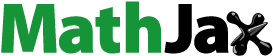
ABSTRACT
Large-scale disasters have exposed vulnerabilities in energy systems and interdependent infrastructure, underscoring the importance of proactively mitigating risks to critical infrastructure. This paper focuses on strengthening power system planning by incorporating the costs associated with prolonged and extensive power interruptions to bolster resilience. To achieve this, duration-dependent customer damage functions are developed, capturing the direct, indirect, and societal impacts of power interruptions, ranging from short, localized events to widespread and long-duration ones. The research methodology is applied to three islanded communities facing substantial resilience challenges with limited data availability. Three customer interruption cost surveys are conducted with local electricity customers, yielding valuable insights into duration-dependent customer damage functions for direct, indirect, and monetizable societal costs. Significantly, the power interruption cost estimates derived from these functions vary considerably from those in the contiguous U.S. and even between the different islanded communities, reflecting their distinct hazard profiles and geographical characteristics. In conclusion, this study demonstrates the potential of duration-dependent customer damage functions to enhance power system resilience. It also identifies several areas for further research, paving the way for a more robust and resilient power infrastructure.
1. Introduction
Islanded populations face an increasing number of short-term risks (e.g., tropical storms, cyclones, and hurricanes) and long-term risks (e.g., sea-level rise, droughts, and flooding) that uniquely impact their energy infrastructure, resulting in long-duration and widespread power interruptions (LDWIs) (Shah, Blechinger, Furlong, et al., Citation2016). In addition, the frequency, intensity, and duration of extreme weather events are expected to increase with a changing climate U.S. Department of Energy, Citation2013, Citation2015; National Academies of Sciences, Engineering, and Medicine, Citation2017, USGCRP, Citation2018; Zamuda et al., Citation2019; Pörtner et al., Citation2022). Recovering from these hazards is particularly challenging due to the remote locations of these communities and their technical, financial, and institutional limitations (To, Bruce, Munro, et al., Citation2021). U.S. agencies including the Federal Emergency Management Agency (FEMA) and the Environmental Protection Agency (EPA) provide some guidance about increasing energy resilience (Klise, Moglen, Hogge, et al., Citation2022). However, more work is necessary to understand island-specific energy vulnerabilities and strategies for increasing resilience. Energy infrastructure is highly capital intensive, and these investments risk becoming stranded if conditions significantly change. Hence, long-term planning has long been a central activity in the electricity sector, placing a premium on the value of new information (Carvallo, Sanstad, & Larsen, Citation2019). While the costs of localized and short-duration power interruptions have been widely incorporated, a resilience-based planning approach does not yet exist in power system planning. Research in this space has focused on defining proper metrics to measure grid resilience (Kintner-Meyer, Homer, Balducci, et al., Citation2017; Vugrin, Castillo, & Silva-Monroy, Citation2017), revising methods to reflect resilience in capacity expansion models (Anderson, Li, Dalvi, et al., Citation2020), or outlining different resilience-enhancing strategies that power system planners can leverage (Mahzarnia, Moghaddam, Baboli, et al., Citation2020). An available approach for resilience-based planning is not designed to be used with LDWI cost surveys and does not reflect common power system planning processes (Stout, Lee, Cox, et al., Citation2019). The missing information on the costs of LDWIs often result in conducting case studies of historical events (see the left of ).
Figure 1. Investments to reduce the risks of power interruptions without and with resilience-based planning and informed power interruption cost estimates.

This paper focuses on the downstream, economic impacts of power disruptions that are a result of damaged utility infrastructure and not on the economic impact of damaged property – to the utility, households, or businesses – resulting from a natural disaster (orange box of the right side of ). Regulatory proceedings and long-term planning processes involving utilities do not account for damage to households or businesses because of the natural disaster. Instead, these processes focus on damage to the utility system (Leibowicz, Sanstad, Zhu, et al., Citation2022) and how this past (or future) damage may impact customers downstream. Utilities and the organizations that regulate them then make decisions about the power system to try and mitigate these impacts in the future. New research from Dormady, Rose, Roa-Henriquez, et al. (Citation2022) indicates that business interruption costs exceed the costs of property damage by more than 900%. Therefore, we focus on the economic and societal impacts of disruptions, given this importance and the general shortfall of power interruption information.
There are well-established methods for assessing the direct costs of localized and relatively short-duration power interruptions. These estimates have been widely used to justify future investments in reliability (e.g., see Larsen, Citation2016). As stated, the costs of LDWIs are often neglected in power system planning processes in part because there is no standardized or agreed-upon method to estimate the value of resilience (Kintner-Meyer et al., Citation2017; Carvallo, Frick, & Schwartz, Citation2022).Footnote1 Including LDWI costs in power system planning would necessitate improvements in the functional forms that have traditionally been used to describe these costs. Most methods of modeling costs of reliability events (i.e., short and localized events) rely on average costs ($/event) and static values ($/kWh) that do not change over the duration of the outage. However, these static values are not appropriate inputs for analyzing resilience investments, which are concerned with mitigating costs from LDWIs. It is more suitable to consider power interruption costs that increase with the duration and geographic scope using duration-dependent customer damage functions (CDFs).
In the cases where duration-dependent values for reliability costs exist, they are typically limited to disruptions lasting under 24 hours (e.g., the Interruption Cost Estimate (ICE) Calculator).Footnote2 To address this, researchers from National Renewable Energy Laboratory (NREL) proposed a framework that offers a duration-dependent implementation of the value of lost load (VOLL) that separates power interruption costs into three categories: (1) fixed costs that do not depend on outage duration; (2) flow costs which accrue as outage duration increases, and (3) stock costs, which are losses of perishable inventory of goods after some point in time (Anderson, Li, Dalvi, et al., Citation2020; Ericson & Lisell, Citation2018). Although these authors demonstrated that duration-dependent CDFs are superior for modeling longer-duration power interruptions, their framework suffers from three major limitations. First, the researchers relied on coarsely-reported data to construct the CDFs. Second, the functional form of CDFs is hypothetical and deterministic. Finally, their framework lacks sufficient customer valuation data to estimate parameters and aggregate individual CDFs to the sector level.
In this paper, we specifically focus on islanded communities. Island populations face unique energy security and resilience challenges due to complications posed by limited redundancy in existing infrastructure systems, logistical challenges complicating disaster response and recovery, and often have limited resources for capital improvements. Ensuring power system resilience is of critical importance for islanded communities. Unfortunately, there is little research systematically estimating power interruption costs for islanded communities, thus requiring immediate attention. More research is needed to understand the direct (damage to electricity systems) and indirect (disruptions from power outages to productivity and emergency management) economic impacts on islanded populations from LDWIs so that utilities, governments, and community stakeholders can make more informed decisions. To date, little research has been done to estimate the economic impacts of power outages on islanded systems (Klise, Moglen, Hogge, et al., Citation2022). Rather, these valuation studies (customer interruption cost (CIC) surveys and regional economic modeling) focus on the contiguous U.S. Many islanded communities are required to use the default VOLL provided by FEMA in their benefit-cost analysis tool to justify energy resilience projects (FEMA, Citation2020). These values are based on outdated data specific to the contiguous U.S. that do not account for the ways in which islanded populations vary in their capacity for economic development, grant fundraising, and energy consumption (Bundhoo, Shah, & Surroop, Citation2018).
This paper offers three main contributions. First, we present a generalizable state-of-the-art CIC methodology that can elicit the costs of power interruptions ranging from short and localized events to LDWIs for different types of electricity customer segments. Second, in collaboration with electricity service providers and local survey partners, we apply the method in three different islanded communities. Finally, we show how planners can collaborate with electricity service providers and local stakeholders to apply these methods in the study regions. We wrap up with a few thoughts on the importance of having survey-informed, duration-dependent VOLL estimates for islanded communities that account for the difference in the power interruption costs between the contiguous U.S. and islanded communities.
2. Method to estimate power interruption costs and construct customer damage functions
In this section, we propose a systematic method that (1) designs realistic power interruption scenarios ranging from a common event to the worst possible event; (2) estimates the costs of power interruptions ranging from short and localized events to LDWIs from each customer segment, and (3) constructs duration-dependent CDFs that estimate the average direct costs of power interruptions for each customer segment. See below for the complete process of power interruption cost estimation and CDF constructions. Further details on the survey design, cost elicitation, and CDF construction methods are described in the following subsections.
Figure 2. Diagram of the steps of eliciting informed customer interruption cost (CIC) estimates and constructing duration-dependent customer damage functions.

2.1. Scenario development
The first step of eliciting informed estimates of power interruption costs starts with developing detailed and realistic power interruption scenarios. For scenario development, we propose the following steps:
Identify the hazard profiles of the study regions
Analyze the impacts of the identified hazards on their electric grid topology
Obtain information about strategies the community has adopted to mitigate risks (e.g., how much backup power does the study region secure and how they utilize the backup power generation system during power interruptions)
Project electric outage durations and geographic scope through simulations or through expert judgments
Develop scenarios that could impact energy infrastructure ranging from common events to the worst-possible case
Have the scenarios reviewed by energy officials, utility personnel, regulators, and relevant stakeholders to identify threats of greatest concern and make the scenarios most relevant.
A region faces several different types of threats, and the range of temporal and geographic scope of power outages can be broad. Nevertheless, we cannot cover all combinations as this would increase survey length considerably. Instead, we rely on two key assumptions to limit the number of scenarios and estimate the CDFs efficiently and effectively: (1) duration and geographic extent of the power interruptions and damage to the power systems are correlated with one another, and 2) the weather condition is fixed to the weather when the economic and societal power interruption costs are highest.
2.1.1. Customer interruption cost survey design
CIC studies typically segment electricity customers into three classes based on their consumption characteristics and the magnitude of impacts they experience. Extending the classic segmentation of electricity customers, we reorganize electricity customers into four classes based on the cost and saving components and the magnitude of economic and societal impacts: (1) residential; (2) small and medium non-residential (SMNR); (3) large non-residential (LNR); and (4) public sector customers.
In the case of residential customers, whose interruption costs are dominated by intangible and non-monetary costs (e.g., lack of comfort and inconvenience), surveys assess the maximum willingness-to-pay (WTP) to avoid the impacts of hypothetical interruptions. Interruption costs of non-residential customers are primarily tangible and measurable. Thus, surveys of SMNR and LNR customers elicit the value of lost revenue, costs incurred, and savings realized during hypothetical power interruptions. In the case of public sector customers, the surveys elicit the interruption-related costs and the impacts of power interruptions on their operations. summarizes the cost and saving components the survey assesses from each customer class.
Table 1. Power interruption cost and saving components that need to be elicited from each customer segment. Asterisks indicate the costs or saving components that are not captured in the traditional CIC surveys.
To elicit the respondents’ informed power interruption cost estimates, we developed an elicitation framework that helps respondents think systematically about the value they attach to resilient electric services. Extending the state-of-the-art customer survey instruments implemented in Baik, Davis, and Morgan (Citation2018, Citation2020) and Sullivan, Collins, Schellenberg, et al. (Citation2019), our approach employs detailed and realistic blackout scenarios, information about the consequences of initiating events, questions about operations/activities that could be sustained during the outages, and assessments of the value of lost production, interruption-related costs, interruption-related savings (non-residential surveys) or WTP to avoid outages to their homes and their communities (residential surveys).Footnote3,Footnote4 See for the elicitation design for residential, SMNR/LNR, and public customers.
2.2. CDF construction
In general, there are three types of power interruption costs: direct, indirect, and societal. The first type is the direct economic costs of power interruptions to electricity customers. The second cost component of a power interruption is the indirect costs, which are further broken into (1) economic losses that accrue due to the absence of that business (or industry), specifically the lost sales from businesses upstream in the production process from the directly affected business; and (2) economic losses arising from changes in spending patterns due to changes in household income as a result of the affected businesses not operating. These costs are estimated from multipliers of regional economic models. Finally, critical private and public organizations’ responses to the expected increase in morbidity and mortality during power interruptions can be used to estimate the societal costs of power interruptions.
Lastly, we construct duration-dependent CDFs using the elicited informed cost and saving estimates. The most important step in CDF construction is finding the appropriate functional form. We employ an empirical approach and select the forms for each customer segment that satisfy the following criteria. First, the costs of momentary outages should be greater than or equal to zero. Second, the increase in power interruption costs for localized, short power interruptions is more gradual than that of LDWIs. This assumption is built on the fact that the geographic extent of localized, short power interruptions is narrower than that of LDWIs, and the critical infrastructure dependent on electricity will become scarce as the duration and geographic extent increase. Third, the expected numbers of injuries or deaths for localized, short power interruptions are less than or equal to those of LDWIs. Finally, the functional forms and cost estimates should be ‘in the ballpark’ of those published in the literature (Sullivan, Mercurio, Schellenberg, et al., Citation2010; Apt, Morgan, Hines, et al., Citation2004; Hanna, Disfani, Haghi, et al., Citation2019; Shuai, Chengzhi, Shiwen, et al., Citation2018; Sullivan, Schellenberg, & Blundell, Citation2015).
3. Case studies: eliciting the duration-dependent CDFs of islanded communities
To develop a generalized set of customer damage functions, we administered the surveys to a small number of electric utility service territories with significantly different characteristics: the Gulf of Alaska, parts of Southeast and Interior Alaska, and a U.S. territory in the South Pacific.Footnote5 Below, we summarize the characteristics of the communities and their electricity consumption:
The first study region, located in the Gulf of Alaska, is a coastal community (Region 1). Its economy relies heavily on the commercial fishing industry. Strong coastal storms often impact the community, and it receives a significant amount of precipitation every year in the form of both rain and snowfall.
The second study region includes parts of Southeast and Interior Alaska (Region 2). Communities in Southeast Alaska are mostly coastal communities. The biggest threats to these communities are tsunamis, earthquakes, and aging infrastructure. On the other hand, communities in Interior Alaska suffer more from fuel supply chain issues, winter storms, and (diesel) generator failures.
The third study region is located in a U.S. territory in the South Pacific, which experiences a number of natural disasters, including earthquakes, floods, storm surges, tsunamis, and tropical storms (Region 3).
The first step of tailoring the generalizable elicitation frameworks starts with designing realistic power interruption scenarios of varying severities. In collaboration with electric service providers for the study regions and local stakeholders, we identified the sets of initiating events, determined the consequences of the power interruptions, and incorporated the feedback of the utilities and stakeholders who represent communities. summarizes the initiating events and power interruption scenarios that we use in the surveys.Footnote6 The survey instruments used in the actual survey are provided in Appendix B to E.
Table 2. Summary of the initiating events and power interruption scenarios.
We conducted three rounds of surveys with convenience samples of electricity customers with assistance from university research partners and staff at the electric utilities. The first study was conducted in a Gulf of Alaska community from June to October 2021. The second study was conducted across parts of Southeast and Interior Alaska from January to February 2022. The third study was conducted in a U.S. territory in the South Pacific from February to April 2022. To participate in the residential surveys, the respondents were required to (1) be at least 18 years old; (2) live in the study region; (3) receive electricity from the electric utility partners; and (4) be aware of their electricity bills. To participate in the non-residential surveys: (1) the respondents were required to work for an organization that has a facility located in the study region; (2) the organization must receive electricity from the electric utility partners; (3) the respondents should be responsible for the organization’s facility management and operation during normal conditions and power outages; and (4) the respondents must be aware of the organization’s electricity bills.
We advertised the study through several channels, including having the electric service providers send emails, local social media community pages, postcards, flyers, radio advertisements, and direct communications (e.g., phone calls and emails from the research collaborators and local hires).
3.1. Direct costs of power interruptions
We elicited the power interruption costs for three different scenarios using the surveys. We first performed a consistency check to validate the collected estimates. Two-sample Kolmogorov-Smirnov tests for all the possible combinations of power interruption cost cumulative distributions within each customer segment and study region suggest that the power interruption costs significantly increase as the duration and the geographic extent of the outage increase (all p-value < .05). With that finding, we used the elicited power interruption costs to construct the duration-dependent CDFs for direct power interruption costs.
We tested linear, exponential, and quadratic functional forms to develop the duration-dependent CDFs. Our analysis comparing the CDFs and macroeconomic estimates suggests that the linear fit underestimates and the exponential fit overestimates the long-duration interruption costs. A quadratic fit, shown as red lines in , provides a reasonable balance of long-duration cost representation and short-duration costs. See for the summary of direct CDFs for each customer segment and study region.
Figure 4. Distribution of the interruption cost estimates collected from surveys and the fitted quadratic CDFs for the part of Southeast and Interior Alaska.

Table 3. Duration-dependent customer damage functions (CDFs) for the direct power interruption costs.
3.2. Indirect costs of power interruptions
Indirect losses include two components. First, they include economic losses that accrue due to the absence of that business (or industry), specifically, the lost sales from businesses upstream in the production process from the directly affected business. Second, they include economic losses arising from changes in spending patterns due to changes in household income as a result of the directly and indirectly affected businesses not operating. Short-duration and localized power interruptions are expected to result in little to no indirect impacts on the economy, but LDWIs could impose substantial indirect costs that can be far greater than the direct costs.
In this paper, we used two different methods to estimate the indirect impacts. The first method is to use the multipliers of the regional economic models (see Ehlen, Vargas, Loose, et al., Citation2011; Santos, Roquel, Lamberte, et al., Citation2022 as examples). The U.S. Bureau of Economic Analysis’s (BEA) regional input-output modeling system (RIMS II) multipliers estimate the potential impact of an initial change throughout the economy (Bureau of Economic Analysis, Citation2013). Using the weighted average of the sector-level demand multipliers (which are the RIMS Type II Multipliers subtracted by one) and the relative weights of industry sectors (which are represented by the number of customer responses), we calculated the indirect demand multiplier for the study region. See , left column, for the indirect CDFs calculated using the study region’s RIMS II multipliers.
Table 4. Duration-dependent CDFs for the indirect power interruption costs.
The other approach utilizes a range of multipliers used in previous studies. This method relies on previous regional economic modeling studies’ multipliers. Sullivan and Schellenberg (Citation2013) review the previous studies and conclude that one-half to two times the direct cost estimates is a reasonable range for indirect costs. Following this approach, we used the range of multipliers (the multiplier of one-half to two) to estimate the indirect power interruption costs in two other study regions. The center and right columns of report the summary of CDFs for the ranges of indirect CDFs calculated using the range approach.
3.3. Monetizable societal costs of power interruptions
Finally, the surveys allowed the research team to assess health and safety impacts of power interruptions. More specifically, the surveys for public customers asked critical private and public organizations who were responsible for emergency services (e.g., hospitals, police and fire stations, schools/emergency shelters, and governments) questions about the expected increase in response time, mortality rate, and morbidity rate due to the natural hazards and LDWIs that followed. summarizes the answers to the health/safety-related questions from the critical public and private organizations.
Table 5. Expected percent increase in morbidity and mortality provided by the critical private and public organizations in the study regions.
While separating the impacts of natural hazards and power interruptions is difficult, we can convert the responses into morbidity- and mortality-related costs under a set of assumptions. To estimate the number of deaths and injuries associated with the power interruptions, we assumed that (1) the expected percentage increase in the number of deaths is more associated with the initiating events, but the expected percentage increase in the number of injuries (i.e., non-fatal injuries and injury-related deaths) is related to power interruptions; and (2) the numbers of non-fatal injuries and injury-related deaths are proportional to the duration of power interruptions.
As an illustrative example, we calculated the health and safety impacts on the study region in the Gulf of Alaska, where we could find sufficiently granular information required for the calculation. Under the assumptions summarized in , we can construct the health and safety duration-dependent CDFs using equations 1 through 5.
Table 6. Key inputs and assumptions used to determine the health/safety CDFs for the Gulf of Alaska.
where Population represents the number of residents living in the study region, t represents the duration of a power interruption, and
represent the expected number of deaths and injuries due to the initiating event and power interruption that last for t hours, Injury death rate represents the number of deaths due to injury per 100,000 population,
represents the number of residents who are hospitalized due to injuries per 10,000 population, and
represent the expected increase in injuries due to the two-day-long and seven-day-long power interruption.
4. Policy implications, research needs, and conclusion
In this paper, we presented a three-step methodology that (1) develops power interruption scenarios in collaboration with utilities and stakeholders; (2) elicits the power interruption costs and health/safety impacts from different electricity customer segments; and (3) constructs the duration-dependent CDFs which can be used to estimate the direct, indirect, and societal costs of power interruptions for a given duration of power interruption.
The methodology we proposed is novel in three aspects. First, the elicitation framework covers a range of scenarios, from common power interruptions to the worst possible scenario, thus providing reliable inputs to construct CDFs without using any extrapolations beyond what was intended. Second, the elicited preferences are used to construct CDFs for direct economic impacts as well as indirect economic impacts and monetizable societal impacts, which can be far greater than direct economic impacts during longer-lasting and widespread power interruptions. Finally, the CDFs can estimate the impacts of power interruptions on the community and therefore can assist in the holistic assessment of potential resilience options.
4.1. Importance of having informed VOLL in islanded communities
For several decades, the costs of short and localized power interruptions have been assessed and used in utility planning. To the best of our knowledge, there are no CIC survey studies covering U.S. electric utility territories outside the contiguous U.S. For these regions, studies often extrapolate the VOLL estimated in different regions of the contiguous U.S. The most representative example of this issue is the FEMA’s benefit-cost analysis (BCA) tool.Footnote7 The FEMA standard value for loss of utility function is measured as the amount (in dollars) per person per day of lost service – labeled Value of Unit Service ($/person/day). According to FEMA’s ‘Benefit-Cost Analysis Sustainment and Enhancements − 2020 Standard Economic Valuation Methodology Report,’ the standard value for electricity is determined by the impact of that utility on the regional economy and on residential customers (FEMA, Citation2020).
FEMA calculates regional economic impacts of lost electric power service by estimating the economic impact per day to commercial and industrial customers using daily national Gross Domestic Product (GDP) dollar values (BEA, Citation2020); importance factors for each economic sector, determined by ATC Publication 25 (FEMA, Citation1991); and U.S. population values (Census Bureau, Citation2020). FEMA estimates regional economic impacts from a power interruption to be $144.32/Capita-Day ($2019). Next, FEMA estimates the impact of the loss of electrical service to residential service by utilizing survey data from a customer’s WTP to avoid an outage. The data used for the FEMA estimate comes from a Lawton, Sullivan, Van Liere, et al. (Citation2003) study, which reviews six survey studies conducted over a 15-year period, covering five utility service territories with a total sample size of 11,368 residential customers. This study concludes that the average WTP to avoid a 12-hour outage is $26.27 ($2002). FEMA extrapolates this amount to be $75.05 ($2019) for a 24-hour outage. As the surveys estimate WTP for households to avoid a power outage, FEMA divides this value by the average household occupancy of 2.54 (U.S. Census, 2019) to arrive at a per capita WTP of $29.78 ($2019). Finally, FEMA estimates the total value of a unit of electric service by adding the regional economic impacts from a power outage, $144.32 ($2019), to the impacts on residential customers, $29.78 ($2019). They arrive with a total economic impact of $174 ($2019) per capita per interrupted day. However, these estimates are inappropriate for estimating resilience benefits for islanded service territories because: (1) the value is static, and often FEMA’s BCAs cover periods of multiple days, where a duration-dependent CDF would yield a different benefit estimate; and (2) the data informing FEMA’s VOLL is outdated and is based on surveying regions within the contiguous U.S.
The proposed method and duration-dependent CDFs developed for islanded systems are immediately useful for two reasons. First, islanded communities have unique populations, economies, and outage profiles. Therefore, extrapolating the power interruption costs estimated in the contiguous U.S. cannot represent the power interruption costs of electricity customers served by islanded systems (more discussion about the differences follows in the next section). The island-specific, duration-dependent CDFs are more representative of non-contiguous U.S. regions since they reflect the actual preferences of residents living within islanded communities.
Second, the duration-dependent CDFs will reflect the temporal arc of LDWIs, which is critical for resilience investment decision-making. The duration-dependent CDFs can provide critical input to a benefit-cost analysis that determines not only power system reliability investments but also power system resilience investments. For instance, decision makers and stakeholders can conduct an ex-ante analysis that justifies future investments in electric power systems. An example of this approach is the recent valuation analysis study conducted for a remote community in Alaska, where a utility partnered with a developer to deploy networked microgrids and advanced control technologies to enhance the power system resilience of the community. The distribution of benefit-cost ratios of the technology deployment under different scenarios was presented to the utility and other stakeholders to illustrate the cost-effectiveness of the technologies under different assumptions. Another way to employ these CDFs would be to use them for an ex-post analysis, which evaluates the value of reliability or resilience after the investment has been made. Larsen (Citation2016) evaluated the costs and benefits of a past decision to completely underground power lines in the community of Cordova, Alaska.
4.2. The differences in power interruption costs between the contiguous U.S. and islanded communities
While there is a general understanding of the differences in risks to electric utility systems and risk preferences within and outside of the contiguous U.S., there are no direct comparisons between these two regions from the perspective of power interruption costs. We drew this comparison using the survey-informed, duration-dependent CDFs constructed for the islanded communities with the power interruption costs estimated within the contiguous U.S. Although there are some differences in how the power interruption costs were elicited across these studies, we are still able to draw some significant insights.
We selected two studies for high-level comparisons of economic impact resulting from one-week-long power interruptions. The first study is Sullivan and Schellenberg (Citation2013), which assesses the total economy-wide impacts of LDWIs lasting from 24 hours to 7 weeks in downtown San Francisco using customer surveys and a range of indirect cost multipliers. This study represents the average costs per outage event per customer for a one-week-long power interruption in the U.S. territory (specifically the U.S. territory in the South Pacific). The second study is Industrial Economics (2018), which employs regional economic modeling to estimate the direct and indirect economic benefits of a microgrid that serves the Village of Rockville Centre. The microgrid serves ~ 3,000 residential customers, 500 commercial and industrial customers, and 26 critical service providers. For the direct comparison, we assumed that the power interruption costs could be linearly interpolated, and 10% of Alaska’s commercial and industrial customers are large industrial customers (i.e., 450 SMNR customers and 50 LNR customers, to match the customer populations served in the Village of Rockville Centre). summarizes the comparison results with Sullivan and Schellenberg (Citation2013), and summarizes the comparison results with Industrial Economics (Citation2018).
Table 7. Summary of the comparison of the islanded communities’ direct and indirect power interruption cost estimates (calculated from the duration-dependent CDFs) and those elicited from San Francisco, California.
Table 8. Summary of the comparison of the islanded communities’ direct and indirect power interruption cost estimates and those elicited from the Village of Rockville Centre, New York.
Both results demonstrate that the power interruption costs calculated from CDFs for islanded communities differ from those of the contiguous U.S. The power interruption costs show large differences even within the islanded communities. Ultimately, these differences underscore the need to elicit power interruption costs separately for islanded communities and use the island-specific CDFs for islanded power systems’ infrastructure investment decision-making.
4.3. Enhancing electric power system resilience using the duration-dependent customer damage functions
As previously discussed, power system reliability is determined based on operating reliabilityFootnote8 and resource adequacyFootnote9in many cases. Power system planners use long-term planning to determine the required investment in capacity that achieve specified reliability (and potentially resilience) outcomes at the lowest cost under normal operating conditions, which exclude low-probability and high-consequence events (e.g., earthquakes, tsunamis, volcanic events, ice storms, physical/cyber attacks). Considering that LDWIs and their initiating events are often excluded from ‘normal operating’ events, it is difficult to justify the investments in resilience under the current power system planning scheme. While there have been studies estimating the costs of short and localized power interruptions, the costs of LDWIs have not been sufficiently explored nor considered in utilities’ investment decision making. Without having those estimates, decision makers often rely on other proxies such as historical impacts on power systems and their costs (see the left of )
Our proposed method can enhance electric power system resilience against changing grid conditions and external threats. Incorporating the costs of LDWIs could substantially change the portfolios to enhance power system resilience. First, the study characterized not only past threats but also future threats by identifying strategies to mitigate their impacts. Second, the duration-dependent CDFs can help estimate the impact on power systems and economic/community costs more accurately with and without strategies at both the customer- and utility-levels (Shah, Blechinger, Furlong, et al., Citation2016). Finally, with additional information detailing how proposed (or actual) investments in reliability (resilience) mitigate the impact of power disruption, utilities and decision-makers can understand the benefits of these actions. Thus, the method described here can enable resilience investment decision making for islanded communities by minimizing total costs and maximizing the avoided economic and social impacts (see the right of ).
An example of the actual implementation of this framework for islanded communities was presented recently. The FRamework for Overcoming Natural Threats to Islanded Energy Resilience (FRONTIER) tool, a web-based energy system resilience investment decision analysis system, was developed by Lawrence Berkeley National Laboratory (LBNL) and Argonne National Laboratory (ANL) with the collaboration of utilities.Footnote10 FRONTIER allows islanded communities to visualize natural threat scenarios and compare the benefits and costs of proposed resilience strategies. The duration-dependent CDFs were used to estimate the avoided economic and social costs of power interruptions of different geographic scopes and duration (the green box of ) but also can help decision makers map out the necessary full range of informed judgments and enhance the resilience of the electric power system with other information (the adjacent boxes of ).
5. Conclusion and future research opportunities
In this paper, we develop and report duration-dependent CDFs which can be used to estimate the direct, indirect, and societal impacts of power interruptions ranging from short and localized events to widespread and long-duration events. This information was generated specifically for use by long-term planners in islanded communities where little or no information on the costs of power disruptions has ever been reported. The research presented here makes a number of important advances, especially in the valuation of power system resilience for islanded communities. First, the framework used to elicit the responses from utility customers covers a range of scenarios, from common power interruptions to the worst possible scenario. Second, the information collected was used to construct CDFs for direct economic impacts as well as indirect economic impacts and monetizable societal impacts. We have found that these non-direct impacts can be far greater than the direct economic impacts during longer-lasting and widespread power interruptions. Finally, the CDFs can estimate the impacts of power interruptions across the community, and therefore, can be immediately used in long-term planning processes to identify potential resilience options. There are several opportunities for future research.
5.1. Long-term planners need better information on compounded uncertainties and how to incorporate them into planning processes
It is a relatively straightforward exercise to estimate the costs and benefits of past investments in power system resilience and reliability. Information necessary to perform this type of analysis includes the capital and operations and maintenance (O&M) costs of the investment, the designed lifespan, the extent and duration of power disruptions avoided due to the past investment, and values of (avoided) lost load generated from a CDF – among other things. However, an ex-ante analysis – the long-term power system planning and valuation process – is subject to a considerable number of compounded uncertainties about the future that need to be incorporated into the decision-making process. These uncertainties include, but are not limited to, assessing the annual likelihood of:
A natural disaster occurring
Resulting damage to community infrastructure, including the extent of damage to the power grid
Expected community responses to the natural disaster, including coping strategies and power restoration times with and without proposed investments
Furthermore, proper assessments of the costs and benefits of a proposed strategy (or investment) need to be compared to a ‘do nothing’ case (i.e., status quo) (Larsen, Goldsmith, Smith, et al., Citation2008; Melvin, Larsen, Boehlert, et al., Citation2017). Important questions for planners interested in enhancing resilience include: What are the natural hazard-related costs to their system and to utility customers if no investments in resilience are made? How might these costs change if we make a broad or targeted set of investments in the grid? However, it is challenging to properly assess the likelihood of conditional events occurring, difficult for planners to interpret the combined likelihood, and extremely complicated to incorporate this information into single (or multi-criteria) planning processes. Additional research is needed to adequately capture the compounded uncertainties involved in estimating power system- and community-level risk and then incorporate this information into long-term planning tools and processes already being used by industry practitioners.
5.2. Valuation of other critical services dependent on electricity is needed
The methods and results presented in this paper focus on the direct, indirect, and societal costs of power disruptions. These estimates were generated based on surveys of customers and regional economic model multipliers. However, the approach described here does not account for the value of other critical services, which are dependent on having reliable and resilient power. For example, we are unable to account for the costs to water utility customers – or societally more broadly – if a lack of electricity prevents the treatment and distribution of water to the community. These interdependencies between the energy and water sectors should be accounted for if electric (or water) utility planners need a comprehensive assessment of the full economic impact of a power disruption. Having said that, some of the methods presented in this paper could be repurposed to assess the value of lost water due to a power disruption. For example, a survey could be deployed to water utility customers asking them to identify expected impacts if they were without water for one day or longer – and how much they would be willing to pay for a hypothetical service that provided water during this disruption. Assessing the value of sustaining the critical and interdependent infrastructure and making necessary investments will enhance the community’s resilience against natural hazards and power interruptions.
Abbreviations
ANL | = | Argonne National Laboratory |
BCA | = | Benefit-Cost Analysis |
BEA | = | Bureau of Economic Analysis |
CDF | = | Customer Damage Function |
CIC | = | Customer Interruption Cost |
GDP | = | Gross Domestic Product |
EPA | = | Environmental Protection Agency |
FEMA | = | Federal Emergency Management Agency |
FRONTIER | = | Framework for Overcoming Natural Threats to Islanded Energy Resilience |
ICE Calculator | = | Interruption Cost Estimate Calculator |
LBNL | = | Lawrence Berkeley National Laboratory |
LDWI | = | Long-Duration Widespread power Interruptions |
LNR | = | Large non-residential |
MBDC | = | Multiple-Bounded Discrete Choice |
NREL | = | National Renewable Energy Laboratory |
O&M | = | Operation and Maintenance |
RIMS II | = | Regional Input-output Modeling Systems (Type) II |
SMNR | = | Small and medium non-residential |
VOLL | = | Value of Lost Load |
WTP | = | Willingness-to-pay |
Public Customer Interview Protocol.pdf
Download PDF (208.2 KB)Large Non-residential Interview Protocol.pdf
Download PDF (260.5 KB)Residential Survey.pdf
Download PDF (276.3 KB)Small and Medium Non-residential Survey.pdf
Download PDF (251.4 KB)Acknowledgments
We would like to acknowledge the staff at the three electric utilities who provided support throughout the design and implementation of the surveys. We would also like to thank Jennifer Schmidt, Jessica Passini, John Rivera, and Forrest Chargualaf for their help with the administration of the surveys. We would like to thank Reviewers for taking the necessary time and effort to review the manuscript. Finally, we sincerely thank the survey respondents for taking the time to complete these surveys. The U.S. Department of Energy’s Office of Energy Efficiency and Renewable Energy (EERE) provided the funding which made this research project possible.
Disclosure statement
No potential conflict of interest was reported by the author(s).
Data availability statement
The (anonymized) data supporting this study’s findings are available upon request from the authors.
Supplementary material
Supplemental data for this article can be accessed online at https://doi.org/10.1080/23789689.2023.2280870.
Additional information
Funding
Notes on contributors
Sunhee Baik
Sunhee Baik is a Research Scientist in the Energy Markets and Policy Department at Lawrence Berkeley National Laboratory (LBNL). Her interests focus on policy problems where technology, science, and public perceptions play a central role. Her research focuses on characterizing energy system vulnerabilities from natural disasters and emerging threats, obtaining economic and social costs of power outages, and enhancing resilience. Sunhee holds a Ph.D. in Engineering and Public Policy from Carnegie Mellon University (CMU), an M.S. in Industrial and System Engineering from Korea Advanced Institute of Science and Technology (KAIST), and a B.A. in Industrial Engineering from Yonsei University.
Nichole L. Hanus
Nichole L. Hanus is a Research Scientist in the Energy Markets and Policy Department at LBNL. Nichole conducts research aimed at improving electricity grid resilience and reliability. Her work is informed by her background in mechanical engineering, behavioral decision sciences, and public policy. Prior to joining LBNL, Nichole worked as an Energy Engineer at Sieben Energy Associates in Chicago and as a Consultant at E3 in San Francisco. Nichole holds a Ph.D. and M.S. in Engineering and Public Policy from Carnegie Mellon University, as well as a B.S. in Mechanical Engineering from the University of Dayton.
Juan Pablo Carvallo
Juan Pablo Carvallo is a Research Scientist in the Energy Markets and Policy Department at LBNL. Research areas at the lab focus on long-term power system planning, integration and planning of distributed energy resources and electric vehicles, and reliability and resilience valuation. Dr. Carvallo holds Ph.D. and M.S. degrees in Energy and Resources from the University of California, Berkeley, as well as P.E. and B.S. degrees in Electronics Engineering from Universidad Técnica Federico Santa Maria, Chile.
Peter H. Larsen
Peter Larsen is a Staff Scientist and Leader of the Energy Markets and Policy Department at the Lawrence Berkeley National Laboratory. Peter conducts research and analysis on electricity reliability and resilience, energy efficiency, and regional electric system planning. Peter holds a Ph.D. in Management Science and Engineering from Stanford University; M.S. degrees from Stanford University (Management Science and Engineering) and Cornell University (Natural Resource Economics); and a B.A. in Economics from the University of Montana at Missoula.
Notes
1. See Baik et al. (Citation2021) and Gorman (2022) for the methodologies that are used to estimate power interruption costs.
2. The ICE calculator can be accessed at: https://icecalculator.com/home
3. Stated preference studies have several inherent issues, including hypothetical bias. However, a study that is carefully designed and properly conducted is assumed to be able to provide useful input into decision making processes. Under this assumption, we adopted the stated preference method to estimate the WTP or cost/saving estimates while making sure that we followed the suggested guidelines (Arrow, Solow, Portney, et al., Citation1993).
4. The most widely-used techniques under this approach include bidding games, payment card method, open-ended questions, dichotomous choice, and multiple-bounded discrete choice (MBDC) method. See Baik, Davis, and Morgan (Citation2018) for the review of major elicitation techniques and their pros and cons. Among these methods, we selected open-ended questions (for non-residential surveys) and MBDC (for residential surveys) to fit the characteristics of power interruption costs of different customer segments, the number of questions needed to be asked to elicit the costs, and difficulties in answering the questions.
5. We obfuscated the names of the utilities in this study to emphasize the application of the generalized framework and de-emphasize utility-specific circumstances affecting the resilience of the power system.
6. We had the fourth scenario of a one-month-long power interruption scenario as the worst-possible scenario for two of our study regions: Southeast and Interior Alaska and the U.S. territory in the South Pacific. However, the respondents struggled to understand the scenario and infer the economic impacts. Furthermore, societal impacts were hard to estimate as the given situations were too extreme, and the impacts of emergency aid and relief from external organizations are hard to predict. To ensure the reliability of the power interruption cost estimates, we decided to drop the fourth scenario.
7. FEMA’s BCA Tool can be found here: https://www.fema.gov/grants/tools/benefit-cost-analysis
8. Operating reliability is defined by the North American Electric Reliability Corporation (NERC) as ‘(the power system’s) ability to withstand sudden disturbances while avoiding uncontrolled cascading blackouts or damage to equipment’ (North American Electric Reliability Corporation NERC, Citation2020).
9. Resource adequacy is defined as ‘(the power system’s) ability to supply the aggregate electrical demand and energy requirements of the electricity customers at all times’ (North American Electric Reliability Corporation NERC, Citation2020).
10. FRONTIER can be accessed at:https://frontier.lbl.gov.
References
- Anderson, K., Li, X., Dalvi, S., Ericson, E., Barrows, C., Murphy, C., & Hotchkiss, E. (2020). Integrating the value of electricity resilience in energy planning and operations decisions. IEEE Systems Journal, 15(1), 204–214. https://doi.org/10.1109/JSYST.2019.2961298
- Apt, J., Morgan, G., Hines, P., King, D., McCullar, N., Meisterling, K. … Talukdar, S. (2004). Critical electric power issues in Pennsylvania: Transmission, distributed generation, and continuing services when the grid fails. Report for the Pennsylvania Office of environmental Protection,
- Arrow, K., Solow, R., Portney, P. R., Leamer, E. E., Radner, R., & Schuman, H. (1993). Report of the NOAA panel on contingent valuation. Federal Register, 58(10), 4601–4614.
- Baik, S., Davis, A. L., & Morgan, M. G. (2018). Assessing the cost of large-scale power outages to residential customers. Risk Analysis, 38(2), 283–296. https://doi.org/10.1111/risa.12842
- Baik, S., Davis, A. L., Park, J. W., Sirinterlikci, S., & Morgan, M. G. (2020). Estimating what US residential customers are willing to pay for resilience to large electricity outages of long duration. Nature Energy, 5(3), 250–258. https://doi.org/10.1038/s41560-020-0581-1
- Bundhoo, Z. M., Shah, K. U., & Surroop, D. (2018). Climate proofing island energy infrastructure systems: Framing resilience based policy interventions. Utilities Policy, 55, 41–51. https://doi.org/10.1016/j.jup.2018.09.005
- Bureau of Economic Analysis. (2013). An essential tool for regional developers and planners. https://www.bea.gov/sites/default/files/methodologies/RIMSII_User_Guide.pdf
- Bureau of Economic Analysis. (2020). News release: Gross domestic produce by industry, third quarter 2019. Available at: https://www.bea.gov/system/files/2020-01/gdpind319.pdf
- Carvallo, J. P., Frick, N. M., & Schwartz, L. (2022). A review of examples and opportunities to quantify the grid reliability and resilience impacts of energy efficiency. Energy Policy, 169, 113185. https://doi.org/10.1016/j.enpol.2022.113185
- Carvallo, J. P., Sanstad, A. H., & Larsen, P. H. (2019). Exploring the relationship between planning and procurement in western U.S. electric utilities. Energy, 183, 4–15. https://doi.org/10.1016/j.energy.2019.06.122
- Dormady, N. C., Rose, A., Roa-Henriquez, A., & Morin, C. B. (2022). The cost-effectiveness of economic resilience. International Journal of Production Economics, 244, 108371. https://doi.org/10.1016/j.ijpe.2021.108371
- Ehlen, M. A., Vargas, V. N., Loose, V. W., Starks, S. J., & Ellebracht, L. A. (2011). Regional Economic Accounting (REAcct). A software tool for rapidly approximating economic impacts(Report No. SAND2009-6552). Sandia National Lab.(SNL-NM),
- Ericson, S., & Lisell, L. (2018). A flexible framework for modeling customer damage functions for power outages. Energy Systems, 11(1), 95–111. https://doi.org/10.1007/s12667-018-0314-8
- FEMA. (1991). Seismic vulnerability and impact of disruption of lifelines in the conterminous United States. In Earthquake hazard reduction series 58. Applied Technology Council (ATC). https://permanent.fdlp.gov/lps117748/fema_224%5b1%5d.pdf.
- FEMA. (2020, June 30). Benefit-cost analysis sustainment and enhancements. “Standard economic value methodology report – version 9.0.
- Hanna, R., Disfani, V. R., Haghi, H. V., Victor, D. G., & Kleissl, J. (2019). Improving estimates for reliability and cost in microgrid investment planning models. Journal of Renewable and Sustainable Energy, 11(4), 045302. https://doi.org/10.1063/1.5094426
- Industrial Economics, Incorporated. (2018). Estimating the regional economic resiliency benefits of community microgrids. Prepared for New York State Energy Research and Development Authority, 18–32. https://www.nyserda.ny.gov/-/media/Files/Publications/Research/Electic-Power-Delivery/18-32-Estimating-the-Regional-Economic.pdf.
- Kintner-Meyer, M. C., Homer, J. S., Balducci, P. J., & Weimar, M. R. (2017). Valuation of electric power system services and technologies(Report No. PNNL-25633). Pacific Northwest National Lab.(PNNL),
- Klise, K., Moglen, R., Hogge, J., Eisenberg, D., & Haxton, T. (2022). Resilience analysis of Potable water service after power outages in the U.S. Virgin Islands. Journal of Water Resources Planning and Management, 148(12), 05022010. https://doi.org/10.1061/(ASCE)WR.1943-5452.0001607
- Larsen, P. H. (2016). Severe weather, power outages, and a decision to improve electric utility reliability [ Doctoral Dissertation]. Stanford University. http://purl.stanford.edu/sc466vy9575
- Larsen, P. H., Goldsmith, S., Smith, O., Wilson, M. L., Strzepek, K., Chinowsky, P., & Saylor, B. (2008). Estimating future costs for Alaska public infrastructure at risk from climate change. Global Environmental Change, 18(3), 442–457. https://doi.org/10.1016/j.gloenvcha.2008.03.005
- Lawton, L., Sullivan, M., Van Liere, K., Katz, A., & Eto, J. (2003). A framework and review of customer outage costs: Integration and analysis of electric utility outage cost surveys. Lawrence Berkeley National Laboratory Report No. LBNL-54365.
- Leibowicz, B. D., Sanstad, A. H., Zhu, Q., Larsen, P. H., & Eto, J. H. (2022). Electric utility valuations of investments to reduce the risks of long-duration, widespread power interruptions, part II: Case studies. Sustainable and Resilient Infrastructure, 8(sup1), 1–20. https://doi.org/10.1080/23789689.2022.2138163
- Mahzarnia, M., Moghaddam, M. P., Baboli, P. T., & Siano, P. (2020). A review of the measures to enhance power systems resilience. IEEE Systems Journal, 14(3), 4059–4070. https://doi.org/10.1109/JSYST.2020.2965993
- Melvin, A., Larsen, P., Boehlert, B., Neumann, J., Chinowsky, P., Espinet, X., Martinich, J., Baumann, M., Rennels, L., Bothner, A., Nicolsky, D., & Marchenko, S. (2017). Climate change risks to Alaska public infrastructure: Improved estimates of damages and the economics of proactive adaptation. Proceedings of the National Academy of Sciences of the United States of America, 114(2), E122–E131. https://doi.org/10.1073/pnas.1611056113
- National Academies of Sciences, Engineering, and Medicine. (2017) . Enhancing the resilience of the nation’s electricity system. National Academies Press.
- North American Electric Reliability Corporation (NERC). (2020). 2020-2021 Winter reliability assessment. https://www.nerc.com/pa/RAPA/ra/Reliability%20Assessments%20DL/NERC_WRA_2020_2021.pdf
- Peterson, C., Xu, L., & Florence, C. (2021). Average medical cost of fatal and non-fatal injuries by type in the USA. Injury Prevention, 27(1), 1–10.
- Pörtner, H. O., Roberts, D. C., Adams, H., Adler, C., Aldunce, P., Ali, & E. Birkmann, J. (2022). Climate change 2022: Impacts, adaptation and vulnerability. IPCC Sixth Assessment Report
- Santos, J., Roquel, K. I. D. Z., Lamberte, A., Tan, R. R., Aviso, K. B., Tapia, J. F. D., & Yu, K. D. S. (2022). Assessing the economic ripple effects of critical infrastructure failures using the dynamic inoperability input-output model: A case study of the taal volcano eruption. Sustainable and Resilient Infrastructure, 8(sup1), 1–17. https://doi.org/10.1080/23789689.2022.2127999
- Shah, K. U., Blechinger, P., Furlong, H., Philippidis, G., Surroop, D., & Singh, A. (2016). Special issue on energy policy for the future of small island developing states (SIDS). Energy Policy, 100(98), 650–652. https://doi.org/10.1016/j.enpol.2016.09.007
- Sheridan, S. C., Zhang, W., Deng, X., & Lin, S. (2021). The individual and synergistic impacts of windstorms and power outages on injury ED visits in New York State. Science of the Total Environment, 797, 149199. https://doi.org/10.1016/j.scitotenv.2021.149199
- Shuai, M., Chengzhi, W., Shiwen, Y., Hao, G., Jufang, Y., & Hui, H. (2018). Review on economic loss assessment of power outages. Procedia Computer Science, 130, 1158–1163. Singh, P., Amekudzi-Kennedy, A., Ashuri, B., Chester, M., Labi, S., & Wall, T. A. (2022). Developing adaptive resilience in infrastructure systems: an approach to quantify long-term benefits. Sustainable and Resilient Infrastructure, 1-22. https://doi.org/10.1016/j.procs.2018.04.151
- The state of Alaska, Department of labor and workforce development. 2020 census data for redistricting. https://live.laborstats.alaska.gov/cen/2020-census-data.html?geotype_check%5B49%5D=49
- Stout, S. R., Lee, N., Cox, S. L., Elsworth, J., & Leisch, J. (2019). Power sector resilience planning Guidebook: A self-guided reference for practitioners(Report no. NREL/TP-7A40-73489). National Renewable Energy Lab.(NREL).
- Strayer, H., Blake, I., Stevens, I., & Provost, E. (2020). Alaska Native Injury Atlas. Third. Alaska Native Tribal Health Consortium Injury Prevention Program and Alaska Native Epidemiology Center. Available athttp://anthctoday.org/epicenter/publications/InjuryAtlas2020/2020_AlaskaNative_InjuryAtlas_FullReport.pdf.
- Sullivan, M., Collins, M. T., Schellenberg, J., Larsen, P. H., Hanemann, M., Morgan, M. G., Davis, A. L., Baik, S., & Vishnav, P. (2019). Elicitation of electric utility customer power interruption costs: A Roadmap for conducting a National survey. https://icecalculator.com/assets/documents/National-Study-Roadmap.pdf
- Sullivan, M. J., Mercurio, M. G., Schellenberg, J. A., & Eto, J. H. (2010). How to estimate the value of service reliability improvements. In IEEE PES General Meeting, Minneapolis, Minnesota (pp. 1–5). IEEE.
- Sullivan, M., & Schellenberg, J. (2013, March 27). Downtown San Francisco long duration outage cost study. Prepared for Pacific Gas & Electric Company.
- Sullivan, M., Schellenberg, J., & Blundell, M. (2015). Updated value of service reliability estimates for electric utility customers in the United States(Report No. LBNL-6941E). Lawrence Berkeley National Lab.(LBNL).
- To, L. S., Bruce, A., Munro, P., Santagata, E., MacGill, I., Rawali, M., & Raturi, A. (2021). A research and innovation agenda for energy resilience in Pacific island countries and territories. Nature Energy, 6(12), 1098–1103. https://doi.org/10.1038/s41560-021-00935-1
- U.S. Census Bureau. (2020). U.S. And world population clock. Available at: http://www.census.gov/popclock
- U.S. Department of Energy. (2013). US Energy Sector Vulnerabilities to Climate Change and Extreme Weather. Department of Energy Washington DC. https://www.energy.gov/downloads/us-energy-sector-vulnerabilities-climate-change-and-extreme-weather#:~:text=US%20Energy%20Sector%20Vulnerabilities%20to%20Climate%20Change
- U.S. Department of Energy. (2015). Climate Change and the U.S. Energy Sector: Regional Vulnerabilities and Resilience Solutions. Department of Energy Washington DC. https://www.energy.gov/sites/prod/files/2015/10/f27/Regional_Climate_Vulnerabilities_and_Resilience_Solutions_0.pdf
- U.S. Department of Transportation. (2021). Guidance on the treatment of the economic value of a statistical life (VSL) in U.S. Department of transportation analyses - 2021 update. https://www.transportation.gov/sites/dot.gov/files/2021-03/VSL%20Update%202021%20-%20Transmittal%20Memo.pdf
- U.S. Global Change Research Program. (2018). Impacts, risks, and adaptation in the United States: Fourth National climate assessment (Vol. II). https://doi.org/10.7930/NCA4.2018
- Vugrin, E. D., Castillo, A. R., & Silva-Monroy, C. A. (2017). Resilience metrics for the electric power system: A performance-based approach. (report no. SAND2017-1493). Sandia National Lab.(SNL-NM).
- Zamuda, C. D., Larsen, P. H., Collins, M. T., Bieler, S., Schellenberg, J., & Hees, S. (2019). Monetization methods for evaluating investments in electricity system resilience to extreme weather and climate change. Electricity Journal, 32(9), 106641. https://doi.org/10.1016/j.tej.2019.106641