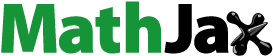
Abstract
During flood events, river topography and fluvial habitats can change drastically, potentially affecting the ecological status. In case of multiple floods, whether each single event modifies the habitat characteristics in the same direction or not, is still an open question. We gathered high quality topographical data of one braided Alpine reach before, between and after multiple floods. Considering the full dynamics of the hydrological regime affected by hydropower production, we calculated water depth and flow velocities distributions for relevant discharge conditions using hydrodynamic modelling. We then calculated four ecological indicators related to habitat diversity, habitat quantity, habitat connectivity and stranding risk. Despite the consistent depositional morphological trend, the habitat diversity and stranding metrics returned to pre-floods values after an initial deviation. The habitat quantity and connectivity metrics did not show a clear trend towards an alternative state. Habitat prevalence varied seasonally and with hydropower water release, and also changed markedly between floods, possibly affecting species composition. We show the possible intrinsic variability in several ecological indicators which can aid in the management and restoration of river floodplains.
1. Introduction
Natural and near-natural rivers are conveyors of water and sediment, and constitute hotspots of aquatic habitats and biodiversity. Erosion and deposition of sediment change river topography (Ashmore Citation1991; Warburton et al. Citation1993; Roghair et al. Citation2002; Milner et al. Citation2013; Parasiewicz et al. Citation2019), which can impact the local biota by altering habitat quality and quantity (Roghair et al. Citation2002; Tamminga and Eaton Citation2018; Parasiewicz et al. Citation2019; Stähly et al. Citation2019), and potentially abundance of fish or invertebrate populations (Elwood and Waters Citation1969; Niemi et al. Citation1990; Parasiewicz et al. Citation2019). Especially during flood events, rivers can transport large quantities of sediment, causing large topographical changes (Sawyer et al. Citation2010). Therefore, these events are capable of modifying existing habitats in a short time frame, representing major disturbances in fluvial ecosystems.
Floods impact the species and communities in the river. Recurring (e.g., seasonal) events allow species to adapt and are therefore usually not harmful (Junk Citation2005). Rare events can directly impact species and communities in a variety of ways (Bischoff and Wolter Citation2001; Carline and McCullough Citation2003; Milner et al. Citation2013, e.g.,). Recovery of species or communities from the immediate effects of flood events can take several months to years (Niemi et al. Citation1990; Pearsons et al. Citation1992; Weng et al. Citation2001; Roghair et al. Citation2002).
Besides their direct, short-term effects, floods can also indirectly affect biota by altering habitat, e.g., areas with a certain combination of parameter values which are suitable for a species or group of species. It is generally assumed that river ecosystems are in equilibrium, i.e., the system is in balance and small changes may lead to an initial deviation from this state, but will ultimately not cause the system to move towards another equilibrium state (e.g., Woodworth and Pasternack Citation2022). However, flood-induced habitat changes can alter this balance and lead to novel ecological states. In this regard, disturbances can be classified by their long-term impact: pulse or press (Reeves et al. Citation1995). The system can either return to the pre-disturbance state after a pulse disturbance, or will move to a new equilibrium after a press disturbance (Reeves et al. Citation1995). This definition has been used to describe the effect of disturbances on a variety of entities, but here we will limit ourselves to the effects of disturbances on habitats. With pulse disturbances, habitat types will persist but might shift in location (termed the shifting habitat mosaic) (Reeves et al. Citation1995; Stanford et al. Citation2005). This process has been validated at river basin (Brennan et al. Citation2019) and at reach scales (Glova and Duncan Citation1985; Van der Nat et al. Citation2003).
Natural variations in weather and hydrology, the immediate flood effects and normal year-to-year variation in abundance of species can mask the long-term flood effects, complicating their measurements. Although many processes can be affected by floods which can have an impact on the long-term ecological status, habitat changes are a good proxy for the long-term ecological changes in a certain reach. Habitat modelling techniques allow for the identification of flood-induced changes to habitats in isolation and are usually employed (e.g., Tamminga and Eaton Citation2018). These procedures aim to measure the habitat changes, assuming these changes affect the biological assemblage. Habitat modelling is often used to analyze the effects of anthropogenic changes, such as river restoration works (e.g., De Jalón and Gortazar Citation2007; Parasiewicz et al. Citation2013; Gostner et al. Citation2021), hence to inform river managers and decision makers.
Using habitat modelling, Harrison et al. (Citation2017) found an increase in the number of pools after a flood, increasing potential Steelhead habitat. Stähly et al. (Citation2019) found that artificial sediment replenishment before floods can increase hydromorphodynamic and habitat diversity. Hajdukiewicz et al. (Citation2016) showed that a flood widened a river reducing habitat diversity in unmanaged reaches, but increasing it in channelized ones.
By determining the long-term ecological effects using habitat modelling, a flood can be classified as a press or pulse disturbance. What happens in subsequent floods is also important. Multiple floods may have consistent effects, changing the habitat template towards a new equilibrium, or subsequent floods may compensate for the previous floods’ changes, reverting the habitat template back to the pre-disturbance state. Multiple flood events have only been investigated sporadically. Glova and Duncan (Citation1985) found that floods did not alter habitat prevalences in braided river reaches, and that the river was in a dynamic equilibrium. Tamminga and Eaton (Citation2018) found that floods generally homogenized the flow conditions in a reach, leading to reduced habitats for the present species. Contrastingly, Roghair et al. (Citation2002) found that multiple floods after an initial extreme flood and debris-flow returned the reach to a state more similar to the pre-event state. Lastly, Woodworth and Pasternack (Citation2022) investigated a long river reach (more than 30 km) and showed how the composition of morphological units was not stationary over multiple floods.
Temporal habitat changes are related to topographical changes, but also to discharge dynamics. The hydrological variation over the year can influence the water depth and flow velocity in a river and cause seasonal habitat changes. This is important to consider since some habitat types are used more in some seasons than others depending on the species and lifestage. In Alpine regions, hydropower production is common and often causes hydropeaking, i.e., sub-daily changes in discharge. This may alter the hydrological regime at multiple temporal scales (Zolezzi et al. Citation2009), and change the temporal dynamics of habitat availability even more (Greimel et al. Citation2015; Holzapfel et al. Citation2017; Judes et al. Citation2021). Additionally, hydropeaking can lead to (rapid) dewatering, which can cause stranding of species or the destruction of redds (Barillier et al. Citation2021). To reduce the effects of hydropower production on biota often limitations on the management of hydropower plants are imposed, such as required minimal flows or limitations on up- and downramping rates (Hayes et al. Citation2019). River reaches with different morphologies are affected differently by hydropeaking (Vanzo et al. Citation2016), and therefore it is likely that hydropeaking can affect the same reach in different ways when it undergoes morphological change. However, the temporal habitat variation due to seasonal or hydropower-induced discharge variation has largely been overlooked in studies identifying flood-induced habitat changes.
In this study, we investigate a braided reach of the Moesa river in southern Switzerland where one major flood was followed by several subsequent floods with a return period between 1 and 5 years. This field site is of particular interest because it is very similar to restored reaches where rivers are widened with the goal of enhancing river ecology (Wohl et al. Citation2015) in response to past artificial narrowing and channelization (Hohensinner et al. Citation2021). Therefore it can be expected that habitat changes occurring at the investigated field site can be representative of those occurring at widened Alpine river reaches. In many rivers, the widened sections are those with the highest ecological value (Rohde et al. Citation2004; Person et al. Citation2014; Schmutz et al. Citation2016) and therefore the natural variability of these reaches is of interest. This is especially the case because more and more rivers are restored, and the amount of river restoration projects is expected to increase (Kurth and Schirmer Citation2014).
We gathered high quality topographical data at this study site before, between and after the floods. We analyzed the flood-induced effects on habitat diversity, quantity and connectivity and stranding potential, considering the full hydrological regime, with the goal of assessing possible long-term effects on fluvial ecosystems. Specifically, we aim to answer the question: is there a trend in the habitat quality and quantity through multiple subsequent floods? We setup a two-dimensional numerical hydrodynamic model for each measured period. Using the numerical model’s output, we modelled the flood-induced habitat changes considering the flow regime’s full dynamics using a novel mesohabitat approach. Additionally, we quantified metrics relating to the change in hydromorphorphological state, connectivity and stranding risk. Finally, we evaluated how the floods changed the reach’s ecological state using these metrics.
2. Study site
The investigated Moesa river reach is located near the village of Cabbiolo, canton Grisons, Switzerland (). The Moesa is a 5th order Alpine stream that flows from the San Bernardino pass to Lumino where it merges with the Ticino river. The reach was never channelized, but is stabilized with side embankments for flood protection. The reach is approximately 800 meters long, has a longitudinal slope of approximately 0.012 m/m and the floodplain’s total width is between 100 and 200 m (). The substrate is gravel with patches of sand.
Figure 1. Overview of the Moesa river. A. The location of the Moesa river, the fieldsite and of nearby gauging stations and towns. B A more detailed view of the fieldsite also showing the hydropower plant’s location and the village of Cabbiolo (source: map.geo.admin.ch: edited). C Monthly discharge of the Moesa river as measured at Soazza Al Pont. The solid line indicates the monthly median discharge and the colored area the discharges between the 5th and 95th percentiles in that month.

Figure 2. Aerial photographs and discharge timeline in the study period. The aerial photographs collected during the field study (see section 3.1). From left to right: 19 December 2018 (pre-floods), 27 August 2019 (not analyzed), 22 January 2020 (inter-floods-1), 16 July 2020 (inter-floods-2), 15 October 2020 (post-floods) and 4 march 2021 (post-floods). The letters indicate areas where an embankment stops the lateral movement of the channel (between A and B), where embankment collapses occurred (C, D, E) or where vegetation was strongly affected by the floods (F, G). Below the residual flow during the study period; the flood events are clearly visible. The horizontal line indicates the approximated discharge where sediment movement is possible.

The nearest gauging station is Soazza Al Pont (Amt für Natur und Umwelt, 2006–Citation2021), approximately 4 km upstream of the fieldsite. A rating curve is available for low discharges, which we extrapolated to high values by calibrating a HEC-RAS model (Brunner Citation2016; Paszti Citation2019). The measured discharge is highest in summer and lowest in winter, averaging approximately 4 and 1.5 respectively (). Hydropeaking, the release of peaks of discharge during hydropower production, is present in the reach. The Soazza power plant is located approximately 1 km upstream from the fieldsite and releases up to 14
of water. It has two turbines with a maximum release of 7
each and regularly runs at partial load.
Two typical Alpine fish species are present: brown trout (Salmo trutta) and bullhead (Cottus gobio) (Flavio Nollo, cantonal Fisheries officer, personal communication). The reach is considered important for trout migration (Flavio Nollo, personal communication).
2.1. Flood events
In 2019 and 2020 multiple floods occurred in the Moesa river (). The first (12 June 2019) was the largest, with an estimated peak discharge of approximately 150 This was the highest flood ever measured at the Soazza Al Pont gauging station. The return period cannot be accurately assessed because the record of water level measurements is only nine years long. Downstream at the Lumino gauging station this flood had a return period of between 100 and 150 years (Bundesamt für Umwelt BAFU Citation2020). More floods occurred later on 12 August 2019, 21 October 2019, 7 June 2020, 29 August 2020 and 3 October 2020 with approximate discharges of 90, 90, 32, 120 and 110
and approximate return periods of 3, 3, 1, 5 and 4 years, respectively. These return periods are based on the Soazza al Pont gauging station and therefore are indicative only, owing to the short available record.
3. Methods
Since floods, especially large ones, are infrequent and poorly predictable, high quality data for pre-flood states is scarce (Turner and Dale Citation1998). When data is available, the data quality often limits the analyses that can be performed. We therefore measured the reach’s topography at several times (Section 3.1). This leads to a full topographical description for four different periods (pre-floods, inter-floods-1, inter-floods-2, post-floods).
Using these topographical models, four numerical hydrodynamic models were setup and run to evaluate the depth and velocity distributions for a set of discharges (Section 3.2). The numerical model’s outputs were used to quantify the floods’ ecological impacts by the calculation of selected metrics, namely: habitat diversity, quantity and connectivity and stranding risk (Sections 3.4-3.7 and supplementary materials).
3.1. Topographical survey and analysis
To measure the river topography, droneflights were carried out, which were georeferenced with ground control points measured using handheld RTK GPS devices. Using structure from motion technique via industry-standard programs (Agisoft Metashape Professional Citation2020; Pix4dMapper Citation2021) the drone images were used to obtain orthoimages and digital terrain models (DTM). The DTM was supplemented with data from below the water surface, which we measured using handheld RTK GPS devices while wading. These measurements were carried out shortly after the drone flight if it was safe to wade and at a later date if not (see also supplementary materials). These points were spaced between 0.5 and 1.5 m apart in transversal direction and 3 to 15 m apart in longitudinal direction. The points were spaced in such a way by the surveyors that interpolating them would yield a good description of the river bed topography. Points measured with the RTK GPS devices had a vertical error up to 0.05 m; horizontal errors were always smaller than vertical errors. Vertical errors up to 0.2 m were allowed under dense vegetation because of poor satellite connections. Points measured with the RTK GPS devices outside the submerged area not used as ground control points, matched the DTM with a vertical error of less than 0.1 m.
We composed four complete DTMs representing four periods: before 12 June 2019 (pre-floods), between 21 October 2019 and 7 June 2020 (inter-floods-1), between 7 June 2020 and 29 August 2020 (inter-floods-2) and after 3 October 2020 (post-floods). In the inter-flood periods of 12 June 2019 − 12 August 2019, 12 August 2019 − 21 October 2019 and 29 August 2020 − 3 October 2020 it was not possible to obtain complete DTMs, owing to the short duration of these periods. They are therefore not analyzed.
To quantify the topographical changes we calculated the difference in elevation among the subsequent states, as used in the hydrodynamic model, for each point in the reach. Areas where higher uncertainties in the DTM are expected (e.g., under dense vegetation), and areas that are dry under ordinary discharge conditions (e.g., side embankments), were excluded from this analysis, totalling approximately 30% of the field site.
3.2. Numerical hydrodynamic modelling
For each investigated period, a numerical hydrodynamic model was setup using BASEMENT v3.1 (Vanzo et al. Citation2021), a freeware tool solving the fully unsteady shallow water equations over two-dimensional unstructured meshes. The computational meshes had between 169,724 and 175,806 cells, and an approximate mean cell size of 0.6 They were created using BASEmesh (Vanzo et al. Citation2021), an open-source plugin.
We considered five different hydraulic roughness categories (rough sediment, fine sediment, vegetated, blockramp and boulders) the areas of which visibly shifted locations between periods and were manually delineated. Fine sediments were primarily located on top of bars and were therefore rarely wetted, but could influence how water was diverted over the side channels. In the model of the first investigated period the roughness values were calibrated by comparing the modeled depths to measured values using five pressure sensors throughout the fieldsite. The Strickler roughness values for the rough sediment, fine sediment, vegetated, blockramp and boulder area were 30, 50, 5, 45 and 20 respectively (see Paszti Citation2019). The remaining hydrodynamic simulations used the same roughness values.
At the downstream boundary a water level was imposed, calculated based on the discharge and local slope, assuming local uniform flow conditions. At the inflow boundary a discharge was imposed. Eleven discharges were investigated (see section 3.3), for each discharge scenario, the models were run until steady flow was reached.
Each of the models’ results were validated by comparing the inundated area visible on the orthoimages with the modelled area, using the discharge scenario closest to the discharge during the drone flight. Since many side channels are shallow, the minimum water depth was set to the low value of 0.005 m, in order to represent the wetted area well.
3.3. Metric calculation and hydrological scenarios
The numerical hydrodynamic model’s output was used to compute various metrics. These metrics relate to the habitat diversity (HMID), the amount of habitat, connectivity within the reach and fish stranding (dewatered area). More details on each of these metrics are given in the following sections.
Each metric is evaluated for each modelled discharge: 1, 2, 3, 5, 7, 10, 12, 15, 17, 20 and 28 These discharges are selected based on the flow duration curve (see supplementary materials). Here we simulate three hydrological scenarios: residual flow, full load (2 turbines in operation) and half load hydropower (1 turbine). We used the hydrograph, calculated from the water levels measured at Soazza Al Pont () as the residual flow, and we consider an additional 7 or 14
to represent the discharge during half load and full load, respectively. The residual flow, half load and full load are well represented by the lower (1 through 7
), the medium (7 through 15
) and the high (15 through 28
) modelled discharges, respectively. For discharge values in the hydrological scenarios that were not simulated, the metrics were linearly interpolated from the two nearest simulated discharges. We present the monthly metric values including the spread in daily values, based on all available discharge data.
We show the depth and flow velocity distributions, as well as the braiding index (i.e., the average number of channels in a cross-section) (Hong and Davies Citation1979). The four metrics we calculated are habitat diversity, habitat quantity, connectivity and stranding risk (Sections 3.4-3.7). Each metric with exception of stranding risk was computed for all topographical states and hydrological scenarios. Since stranding risk is concerned with the difference between two discharge stages, we calculated it for the difference between full load and residual flow.
3.4. Habitat diversity: Hydromorphological index of diversity (HMID)
We used the hydromorphological index of diversity (HMID) to estimate habitat diversity (Gostner et al. Citation2013). The HMID is a measure for the diversity in ecologically relevant hydromorphological parameters in the riverine environment. It is calculated as:
(1)
(1)
where σd and σv are the standard deviation of the depth and velocity distributions, respectively, and μd and μv are the mean depth and velocity, respectively. Sites with an HMID lower than 5, between 5 and 9 and higher than 9 are considered morphologically heavily altered, somewhat altered and pristine, with all possible types of habitats present (Gostner et al. Citation2013), respectively. A higher HMID is thus considered a proxy of higher diversity.
The HMID was developed for mean flow conditions. However, we applied it to all discharge scenarios to consider the full hydrological regime. Therefore, comparing the resulting values with other rivers or the classification values given above may be difficult.
3.5. Habitat quantification: Mesohabitat modelling
We quantified the available habitat types. We did this using a mesohabitat approach, which assumes that habitat choice or quality depends not only on the location itself but also its surroundings. We employed BASEmeso, a newly developed algorithm that identifies homogeneous, distinct and spatially contiguous patches based on the distribution of depth and velocity values (van Rooijen et al. Citation2021), to identify the location and extent of hydraulic units, which relate to biotic assemblages (Thomson et al. Citation2001).
Using the k-means clustering algorithm (Jain Citation2010), the hydraulic units identified by BASEmeso (following the considerations of van Rooijen et al. Citation2021) were grouped into 6 habitat types, which proved to provide a good balance between interpretability and amount of detail. Additionally, the obtained habitat types related well to species suitability (see below). The clustering was performed using the pre-floods patches, where the different discharges were weighted to their occurrence frequency. The clustering parameters were the 5th, 50th and 95th percentiles of the depth and velocity distributions of the patches, considering the parameter values’ spread and not just their mean value. The patches of the other topographical states (inter-floods-1, inter-floods-2 and post-floods) were grouped into the same habitat types. The rationale is that species occupying the habitats before the floods, had adapted to their habitats, e.g., we assume pre-floods is a valid reference state. Each group of patches would therefore represent a different habitat type, used by different species. The flood-induced changes in the prevalences of habitat types therefore are indicative of habitat changes for groups of species. Each habitat type’s prevalence (e.g., amount of area occupied by a habitat type) was computed in each of the investigated scenarios and compared.
Habitat types are not utilized equally by species and can therefore be difficult to interpret. To aid interpretation, we therefore linked habitat types to species by comparing the habitat type characteristics to suitable conditions for the present fish species. The habitat types which for a large part coincided in terms of characteristics (depth and velocity) with the suitability criteria for the species were considered linked to the species. This can show which habitat types are most relevant and should be focused on in analysis. Suitable conditions were inferred using habitat suitability criteria (), stemming from a geographically near river if possible (Vismara et al. Citation2001; Person Citation2013) and from multiple sources if curves from such rivers were unavailable (Vezza et al. Citation2014a; Adamczyk et al. Citation2019). The following species/lifestages were considered: adult brown trout (ABT), juvenile brown trout (JBT), brown trout spawning (BTS) and bullhead. For BTS the analysis was restricted to the months of October-December. The analysis is also applicable to other species, since species only have to be linked to one or several habitat types. The fish species and habitat types considered here serve only as an example.
Table 1. Values of depth and velocity considered suitable for the different species and lifestages.
3.6. Connectivity analysis
Habitat that cannot be reached, cannot be used. Therefore habitat availability declines when topographical changes impair fish movements between habitats. To quantify habitat connectivity, we adapted the dendritic connectivity index (DCI, Cote et al. Citation2009). This index was developed to identify the effect of artificial (dams) and natural (waterfalls) barriers on watershed connectivity. The DCI is the probability that fish can move freely (e.g., without encountering a barrier) between two randomly chosen points in a watershed.
We turned this metric two-dimensional, with insufficient depths or excessive flow velocities as barriers. We call this metric the Reach Connectivity Index (RCI). We focused on ABT, being the most mobile species/lifestage combination present. An individuals body size determines the depth and velocity conditions under which ABT can swim (Castro-Santos et al. Citation2013; Dönni et al. Citation2016). Here, we assume that areas deeper than 0.2 m were freely passable (Dönni et al. Citation2016) if velocity did not exceed (Castro-Santos et al. Citation2013). Furthermore, it was assumed that individuals could move up to 5 m in water deeper than 0.07 m (Dönni et al. Citation2016) with a velocity less than 2 m/s.
Using these criteria we identified the freely passable areas (e.g., fish can move from any point to any other point in the area). We first delineated the area deeper than 0.2 m and slower flowing than 2 m/s. This area was buffered by 2.5 m (e.g., half the distance fish can swim in water shallower than 0.2 m). The area deeper than 0.07 m and flowing slower than 2 m/s was intersected with the buffered area, yielding the area to which fish could swim. The contiguous areas identified in the first filtering step (e.g., the areas with a depth >0.2 m and velocity <2 m/s) that were in the same contiguous area from the last step (e.g., the area in which a fish can move freely) were considered to be one freely passable area. Finally a visual quality check was performed.
The probability that fish can move freely between two randomly chosen points considered swimmable, was calculated as:
(2)
(2)
where Ai is the freely passable area that can be reached from any point within that area, Atot is the total swimmable area in the reach and n is the number of areas between which movement of ABT is not possible.
The RCI calculates the probability that an ABT individual can move from one swimmable area to another. As such, it identifies if all potential habitats can be reached and used (RCI = 1) or if all areas are disconnected from one another (RCI = 0). It thus quantifies habitat fragmentation. However, whether under certain discharge conditions the reach is a barrier for large-scale movement (e.g., migration) of ABT due to insufficient depths or excessive flow velocities, is not resolved (for a more in depth explanation of the RCI, see the supplementary material). We tested whether the reach was such a barrier by identifying under which discharge conditions there is a freely passable path present between the reach’s downstream and upstream boundaries, i.e., if the upstream and downstream barriers were in the same freely passable area. Further description of the Reach Connectivity Index and a graphical example are provided in the supplementary materials.
3.7. Dewatered area
Large variations in discharge are common in the investigated reach due to the up- and down-ramping of hydropower flow release. During down-ramping the water level drops, consequently drying some river bed area. This can have impacts on the ecosystem, e.g., causing redds to be dewatered (Barillier et al. Citation2021). Additionally, when the down-ramping happens too fast, organisms (e.g., fish and macroinvertebrates) may not manage to escape and therefore get stranded. This is particularly harmful for alevines and juveniles (Halleraker et al. Citation2003). Stranding is often lethal (Holzapfel et al. Citation2017). Despite no recorded scientific evidence of stranding at the Moesa fieldsite, since fish stranding can be significant in braided reaches (Vanzo et al. Citation2016), we nevertheless investigate the floods’ possible effects on this process to highlight potential changes.
Topographical changes mostly alter stranding risk by changing the amount of area that falls dry when water levels drop. We therefore calculated the dewatered area to analyse the effects of the altered topographies (Baumann et al. Citation2012):
(3)
(3)
where
is the wetted area during hydropower flow release and
is the wetted area under base (residual) flow conditions. Dewatered area is considered high if it exceeds 30% (Baumann et al. Citation2012).
We calculated this metric as a function of time using the full load (peak) and residual (base) scenarios as an integral over the entire floodplain but also locally, using cross-sectional slices with a width of 5 m, allowing us to also asses if certain locations are more prone to dewatering than others. For the spatial analysis, we used 3 and 17 as a representative value for residual and peak flow conditions throughout the year, respectively ().
4. Results
4.1. Topographical changes
Each flood thoroughly reworked the topography; shifting channels are visible after all floods (). In the upstream and middle of the fieldsite, the main channel shifted eastwards during the first flood. During the subsequent floods, the main channel shifted little in the upstream area, but shifted first westwards and then split into two channels, approximately equally wide, in the middle of the floodplain. The eastern channel moved further east during the last flood. In the downstream area, the first flood moved the main channel eastwards, causing a bend to form. The subsequent floods opened a new channel on the western side that grew slightly larger with each flood. A reach’s topography can also change during low flow periods (Pasternack and Wyrick Citation2017), typically by a slow homogenization of the topographical gradients. In our case study there were only short periods (months) between the floods, and the vast majority of the topographical changes are associated with the floods.
Numerical simulations show that at a discharge of 3 only 32% of the area that was wetted during pre-floods remained wetted at inter-floods-1. The subsequent flood periods changed the channel configuration less: 56% and 63% of the wetted area of inter-floods-1 and inter-floods-2 remained wetted in inter-floods-2 and post-floods, respectively. On the fieldsite’s western side, a vegetated embankment does not allow the channel to move further westwards (), causing one channel to hug the side during all periods. Persistent channels on the margins of an active floodplain are not uncommon (e.g., Warburton et al. Citation1993). Of course, channels can still change even when they do not move, e.g., become shallower or deeper.
The embankments eroded at two specific locations. A first bank-erosion event occurred between pre-floods and inter-floods-1 in the northeastern embankment (). The other erosional event affected the southeastern embankment () between inter-floods-1 and inter-floods-2, removing several of the large boulders protecting the shoreline. Between inter-floods-2 and post-floods this initial embankment failure grew, especially upstream ().
The floods also affected riparian vegetation. All floods removed young vegetation patchily present in the active floodplain, while vegetation on the stable embankments was little impacted. Mature vegetation was affected too. Before the floods the fieldsite’s northwestern part was densely vegetated with some minor channels flowing through (). Each flood removed some vegetation and widened the channels through this area. Additionally, an island populated by old trees () lost much vegetation in the last two flood periods.
Overall, more sedimentation than erosion occurred between each of the investigated periods (), with sedimentation more pronounced in the upstream part. Despite the lower amount of sedimentation in the downstream part, the bed changed thoroughly, with channels shifting both locations and dimensions. We interpret these findings as an indication that the investigated reach is not in a morphological equilibrium.
Figure 3. Topographical changes in the reach. On the left the DEM of difference and on the right the distribution of the elevation changes. Panels A-C refer to the elevation changes between the pre-floods and inter-floods-1, the inter-floods-1 and inter-floods-2 and the inter-floods-2 and post-floods respectively.

The braiding index changed much between the hydrological scenarios (approximately 2 at residual flow, approximately 3 at full load, see supplementary materials), while the topographical changes generally had a less pronounced effect: with exception of the inter-floods-1 period at half load, the differences in braiding index between the topographical states were smaller than 0.5. Water depths were slightly elevated in inter-floods-1 and inter-floods-2 compared to pre-floods, while flow velocities slightly dropped in these periods (). Yet, both water depth and flow velocity distributions widened under all discharge conditions in inter-floods-1 compared to pre-floods (). In post-floods both depth and velocity distributions were similar to pre-floods conditions. The first flood thus changed the flow dependent characteristics (depth, velocity) and the subsequent floods returned these to pre-floods values. Although the morphology is not in an equilibrium, the flow dependent variables suggest the opposite.
Figure 4. The depth and flow velocity distributions in time. The depth A-C and flow velocity D-F for each month, the continuous line indicates the median and the dashed line the 95 percentile in that month. The top, middle and bottom panels refer to full load, half load and residual flow, respectively.

4.2. Hydromorphological index of diversity (HMID)
In , we present how the HMID changed between the topographical states (identified by colors) under the different hydrological scenarios (panels A through C). Between pre-floods and inter-floods-1 the HMID increased under all hydrological scenarios to values between 10 and 12 (). Between inter-floods-1 and inter-floods-2 the HMID decreased again: under residual flow the HMID dropped to below pre-floods levels (), while with hydropower water release, the HMID returned to pre-floods levels (). Between inter-floods-2 and post-floods the HMID increased under residual flow conditions (), and slightly increased in the other hydrological scenarios ().
4.3. Habitat modelling
We defined six habitat types using the k-means clustering algorithm (). For the sake of clarity we named the six types according to their general depth and velocity characteristics (depth-velocity): low-low (brown), low-medium (black), medium-low (green), low/medium-high (red), high-low/medium (yellow) and high-high (blue; ). Comparing these to suitable conditions for brown trout and bullhead, shows that the high-low/medium habitat type is suitable for ABT, while both high-low/medium and medium-low are suitable for JBT and BTS. High-low/medium, medium-low and low-medium habitat types comprise suitable habitats for bullhead. We focus on the changes in these habitat types, being the most important for the description of fish habitats in this river reach.
Figure 6. Indication of the depths and velocities associated with the habitat types. The approximate median depths and flow velocities associated with the habitat types as identified by the clustering analysis. Note that habitat types also depend on the 5th and 95th percentile values of these parameters. Few patches have high median depths or velocities. The boxes indicate the approximate habitat conditions suitable for the present species.

In , we show the area associated with each habitat type (as in ) under the different topographical and hydrological scenarios. Panels A-C, D-F and G-I refer to the high-low/medium (yellow), medium-low (green) and low-medium (black) habitat types, respectively. Panels A, D and G refer to full load and C, F and I to residual flow.
Figure 7. The prevalence of 3 habitat types and the prevalence of habitat for the present fish species in time for the different topographical states. The area of the high-low/medium (A-C), medium-low (D-F) and low-medium (G-I) habitat types and the area of adult brown trout (J-L), juvenile brown trout (M-O) and bullhead (P-R) habitat for each month, the line indicates the median and the fill the area between the 5th and 95th percentile. The top, middle and bottom panels refer to the full load, half load and residual flow hydrological scenarios, respectively.

Under residual flow, the high-low/medium habitat type varied with the seasons (). In winter (December-March) there was a strong increase (up to 80%) in this habitat type between pre-floods and inter-floods-1. Afterwards, this habitat types’ prevalence decreased again to pre-floods levels. In early summer (May-July) the first flood period decreased high-low/medium prevalence by up to 30%, the subsequent floods initially seemed to revert the prevalence to pre-floods levels, but then dropped to inter-floods-1 levels. In autumn (August-November) little change was found.
Under full load (), the initial large flood barely changed the amount of high-low/medium habitat, but the subsequent floods decreased it, initially only in summer and autumn, but eventually throughout the entire year with equal amounts, about 30%. Under half load (), the high-low/medium habitat type decreased overall, but not monotonically.
Seasonality impacted the medium-low habitat less, but the topographical changes still caused changes in prevalence (). Under residual flow, this habitat type decreased by approximately 25% during the first flood. Its prevalence then increased to pre-floods levels during the next flood, and reduced to inter-floods-1 levels during the last flood (). The medium-low habitat type only experienced seasonal trends under full load, with higher prevalence in summer and autumn than in winter (). This seasonality was lost after the first flood, when prevalence increased by 15% in to 45%. Seasonality returned and prevalence decreased to values similar to those in the pre-floods state in the inter-floods-2 and post-floods periods. Under half load, the medium-low prevalence increased during the first flood by approximately 15%, then decreased during the other floods to lower than pre-floods levels.
The total of the combined suitable habitat types show how species could be impacted (). Since ABT is linked to the high-low/medium habitat type only, the changes in ABT habitat are equal to the high-low/medium habitat changes ().
The combination of high-low/medium and medium-low habitat types approximates JBT and BTS habitat (), with spawning only occurring between September and December. Under residual flow, JBT habitat is not affected in winter and early spring, except for the post-floods case. In the other months, JBT and BTS habitat initially decreased approximately 25% between pre-floods and inter-floods-1, followed by a small increase and decrease in prevalence. Under half load, each flood reduced JBT habitat by up to 20% except in June, where the first flood increased JBT habitat slightly. Under full load, JBT and BTS habitat increased by approximately 20% between pre-floods and inter-floods-1, then reduced to below pre-floods levels in post-floods.
Bullhead is adapted to the most habitat types and therefore has the most potential habitat (combination of high-low/medium, medium-low and low-medium habitat types; ). Under residual flow, bullhead habitat changed seasonally during pre-floods with most habitat available in summer and least in winter (). The first flood removed this seasonality bringing habitat prevalence to winter levels throughout the year (decrease of approximately 40% in summer). Bullhead habitat increased between inter-floods-1 and inter-floods-2 and in post-floods was virtually at inter-floods-1 levels. Under half load (), bullhead habitat reduced by approximately 30% and 10% between pre-floods and inter-floods-1 and inter-floods-1 and inter-floods-2, respectively. Only the last flood increased bullhead habitat. Under full load () bullhead habitat increased, decreased and then increased again.
4.4. Connectivity
Our numerical simulations show that under residual flow, connectivity (measured by RCI) is highest in summer and lowest in winter (). With exception of the pre-floods condition, the opposite holds for full load (), while with half load no large changes in RCI occur in time (). Under residual flow, every flood increased the reach connectivity with the exception of the second flood (). The exact opposite holds for full load (). Under half load RCI is stable between the different topographical states, except for inter-floods-2, where connectivity is higher (). A continuous path for adult brown trout (ABT) between the reach’s downstream and upstream ends was present for discharges of at least 2, 1, 3 and 1 for the pre-floods, inter-floods-1, inter-floods-2 and post-floods periods respectively.
4.5. Dewatered area
Dewatered area is high (30%) throughout the year () for all topographical configurations and is highest in winter and early spring. The first flood (between the pre-floods and inter-floods-1 conditions) increased the dewatered area throughout the year by about 10%. The two subsequent floods returned the stranding risk in two steps to pre-floods levels.
Figure 9. Dewatered area in time and space for the four topographical states. The change in wetted area due to down-ramping events, linked to fish stranding, for the four investigated topographies A. throughout the year and B. as a function of the transversal direction due to a down-ramping event from 17 to 3
The horizontal dashed line indicates the 30% threshold which indicates a high dewatering rate.

Although the dewatered area is high throughout the year, it is not high everywhere in the reach (). There are several places in the river reach with low values. Highest dewatered area is found at locations that have a side channel that dries up during down-ramping.
5. Discussion
5.1. Change in ecologically relevant hydraulic metrics
The HMID is related to the depth and velocity changes and also shows a dynamic equilibrium (). As a diversity metric, the HMID gives an indication about the magnitude of habitat changes in the reach. The change in HMID under residual flow indicates large changes in habitat diversity. Similarly, we can expect smaller habitat changes under the higher discharge conditions based on the HMID. Previous research has already found that large magnitude floods can have an impact on habitat diversity (Hajdukiewicz et al. Citation2016). From our results it is clear that the large flood changed the fieldsite’s state considerably: had no more floods occurred, the biota would have adapted to the new situation. However, the subsequent floods changed the ecologically relevant reach characteristics (e.g., depth and velocity) back to the pre-floods levels. This is similar to the processes found by Roghair et al. (Citation2002) who investigated floods following a large flood and debris flow. Thus, the change in habitat diversity due to a major flood may potentially only impact the reach until the next flood occurs.
The floods also changed the number of patches in each habitat type. After the first flood, depths increased while velocities decreased. A reasonable expectation is that ABT (preference for deep and low flow areas) would benefit from these changes. However ABT habitat increased only in winter under residual flow conditions. Thus, although depths and velocities both changed in a direction considered positive for ABT, suitable depths and velocities coincided less often, e.g., the high-low/medium habitat type reduced in prevalence. In the subsequent floods both depth and velocity values returned to pre-floods conditions. The amount of ABT habitat only returned to pre-floods levels in some cases. The individual parameter distributions thus were not representative for the habitat types. Each flood affects species and lifestages differently and therefore the overall change in habitat quality will depend on which species or lifestage is limiting or considered of greatest importance (Farò et al. Citation2021). The time period that the habitat is affected (e.g., which season) can be of equal importance as the amount of change, e.g., BTS is only present in some months. Changes due to one flood were sometimes amplified and sometimes partially or completely reverted by the next. An example is ABT habitat under residual flow. This is remarkable, since both the depth and velocity distributions returned to the pre-floods state. However, the joint distribution of depth and velocity did not, but changed after each flood, yet not in a consistent manner. We interpret this as the reach being in a dynamic equilibrium from a habitat quantity perspective. In this dynamic equilibrium, the amounts of habitat can still change significantly, some habitat types increased or decreased more than 30% between two consecutive states, e.g., the natural variation in habitat quantity can be large. Such profound habitat shifts will influence the biota.
Habitat quality may change without a change in habitat prevalence. For JBT, under full load, the habitat prevalence is stable in the year for all investigated topographies (). However, if we focus on inter-floods-2 we can see that although the same amount is present throughout the year, in summer the potentially suitable habitat has shallower depths overall than in winter (compare medium-low and high-low/medium habitat types; ). Thus, even if habitat prevalence remains the same, the habitat quality may change. Since each flood changes habitat prevalences, each flood individually could be identified as a press disturbance. The changes in habitats can be large, and the present fish community would likely shift long-term if these conditions remained. If studied in isolation, the first flood, having the largest impact, would likely be classified as a press disturbance. However, considering all floods, it seems more correct to identify the floods as pulse disturbances. Although a singular flood may cause changes in habitats, the subsequent floods can alter it again. Over long periods of time, with sufficient floods occurring, there is no consistent trajectory in the habitat changes and the reach will be in a dynamic equilibrium.
We limited our analysis to the present fish species’ habitat. The changes of in-stream habitat types will also affect other species in the river, like macro-invertebrates. In most river reaches there are more species of macro-invertebrates than fish species and therefore a more complex set of habitat changes may be found. Additionally, macro-invertebrates are a food source for fish (Holzapfel et al. Citation2017), thereby impacting fish species as well. Changes in habitat types are thus not limited to fish and the present approach also sheds light on the potential changes for other species.
Habitat can only be used if fish can reach it. The RCI shows that the floods influence the ability of ABT to move to suitable habitats. RCI is based on the spatial configuration of areas which are too shallow or fast flowing. If these occur between potentially suitable areas the RCI is reduced. Due to the low number of areas with excessive velocities, the RCI is more affected by excessive depths in our reach. The distance between areas with sufficient depths, thus influences RCI the most. A negative correlation of RCI with braiding index would thus be expected, since one barrier in a side channel may disconnect many potentially suitable areas. In our reach this correlation is very weak. Instead the number and locations of local shallow areas in the main channel determines the RCI. The side channels impact the connectivity little because there are few suitable destinations for ABT, making any barriers there irrelevant. However, the seasonal RCI changes are influenced by the side channels. RCI generally increases with increasing discharge, since depths in the main channel increase. In some cases, however, we see a decrease in connectivity. Connectivity in the main channel was then already close to the maximum and the increase in discharge caused more destinations in the side channels to develop. Since shallow conditions prevail in the side channels, these are usually disconnected from other suitable areas, thereby reducing the overall connectivity. An increase in discharge can therefore cause an increase or decrease in RCI depending on the situation. Connectivity can also affect larger scale movements, e.g., upstream migration. Since the reach is only a barrier under the lowest discharges, the frequent hydropower-induced discharge peaks might support large-scale movement year round. The discharge thresholds for ABT migration are correlated to the RCI under residual flow. Actually, the two topographies that always allow large-scale migration (inter-floods-1 and post-floods) have the highest RCI in this configuration ().
The dewatered area has a spatial distribution typical for braided reaches, i.e., alternating high and low values (cf. Vanzo et al. Citation2016). Topographical changes did not influence this, despite the expected negative correlation with braiding index (e.g., Vanzo et al. Citation2016). Possibly, the differences in braiding intensity are too small to noticeably affect the dewatered area. Overall, the flood-induced topographical changes only had small effects on the dewatered area. To avoid stranding, fish must flee these areas that become dewatered due to a downramping event. The ability of a fish to flee is therefore as important as the amount of dewatered area. This is a function of the reach’s connectivity expressed with the RCI as well as the speed of downramping. Topographical changes potentially affected fish stranding more due to differences in connectivity than due to differences in dewatered area.
5.2. Direction of changes
The floods affected the investigated reach in several profound ways. The initial and largest flood altered both the HMID and dewatered area. The subsequent floods returned these metrics to pre-floods levels. The other investigated metrics did not show this trend, in fact they did not show any consistent changes. Importantly, they did not move towards a new equilibrium, most likely because the metrics change within the bounds of a dynamic equilibrium. Meaning that habitat changes in a dynamic equilibrium can be significant, at least on the reach scale. Some habitat types decreased by more than 30%. This is not in agreement with the shifting habitat mosaic theory, which states that disturbances such as floods cause changes in habitat locations, but not in prevalence. This disagreement might be caused by the comparatively small size of the investigated field site which makes it less likely that a decrease in one habitat type in one location is compensated by an increase somewhere else in the field site. The scale of the field site may not be congruent with the shifting habitat mosaic theory’s assumptions (see Reeves et al. Citation1995; Stanford et al. Citation2005) and therefore this theory cannot be properly tested in this field site.
The first flood was the largest flood. This would lead to larger hydraulic forces on the bed, causing more sediment movement. A more thorough reworking of the topography and habitats are therefore to be expected. This could explain why changes during the first flood broadly differed from the other floods.
However, another explanation can also be proposed. The meteorological conditions that caused the first flood, also generated other disturbances in and close to the fieldsite, possibly impacting the state of the river reach. A good example are two landslides. One landslide occurred close to the northeastern boundary of the fieldsite (), the other in a montane tributary which merges with the Moesa river approximately 4 km upstream of the fieldsite. Both these landslides may have increased sediment availability, especially during the first flood, which can affect the river’s topography (Ashmore Citation1991; Harrison et al. Citation2011; Liébault et al. Citation2013; Pasternack and Wyrick Citation2017; Rachelly et al. Citation2021). This could have affected not only the amount of sedimentation, but also the channel planform (e.g., Rachelly et al. Citation2021) and thereby the ecological indicators.
5.3. Effect of hydropeaking
The dewatered area metric shows that hydropeaking related effects can change between flood-induced morphological states (). The other metrics (RCI, HMID and all investigated habitat types) change differently under full load conditions than under residual flow conditions. Concerning hydropeaking, not just the values of metrics can change, but also the seasonality of the metric values (see for example the HMID, or the high-low/medium habitat type, ). To obtain a full understanding of the habitat dynamics in a flood- and hydropower-affected reach, it is therefore insufficient to only consider the changes under residual flow conditions.
The effects of hydropower are often identified using habitat modelling techniques similar to those in this work in order to set limitations on the management of hydropower resources, such as environmental flows (Hayes et al. Citation2019). If floods change the morphological state of a reach, updated rules for the management of hydropower may be required to ensure the continued ecological functioning of fluvial ecosystems. To ensure certain minimum amounts of habitats or connectivity, minimum or residual flows would for example need to be changed depending on the morphological state (e.g., ). Identifying new minimum or residual flows frequently, could be time and resource intensive however. As a solution to this, limitations on hydropower can be set upon considering the flood-induced variability in ecological metrics. In other words, the limitations can be set in such a way the ecological needs are met under a certain range of expected morphological states. Especially in reaches experiencing a multitude of floods which do not cause the dynamic ecological equilibrium to shift, such as in the investigated reach, this may be a good solution.
5.4. Limitations of the approach
The habitats we defined are based on depth and flow velocity values. Temperature (Jonsson and Jonsson Citation2009; Elliott and Elliott Citation2010), bed grain size (ASCE Task Committee on Sediment Transport and Aquatic Habitats and Sedimentation Committee Citation1992), cover (Niayifar et al. Citation2018) and many other parameters (e.g., Jakober et al. Citation2000; Rosenfeld et al. Citation2005; Cotel et al. Citation2006; Huusko et al. Citation2007; Null et al. Citation2017; Larsen and Woelfle-Erskine Citation2018) can also play a role in habitat analyses. This paper focuses on the ecological changes caused by changes in two parameters directly impacted by the floods and which are the most commonly used parameters in habitat modelling. Floods may also change the other parameters amplifying or reducing the habitat changes. More research into the additional effects of these parameters is needed but may be hindered by demanding data acquisition.
We applied a methodology where habitat suitability for species is inferred based on habitat types. The determination of which combination of habitat types constitute suitable habitat for which species or lifestage can have a large impact on the results. ABT and JBT habitat differ in only one habitat type, but show vastly different changes. In this work, the species habitat were just shown as aids for interpretation. The identification of habitat variability due to floods is not impacted.
An assumption in habitat modelling is that species preferences are static. However floods may also change habitat preferences of species (see Dolloff et al. Citation1994). The correlation between the abundance of species and their associated habitat types may thus be context dependent. Changes in habitats can still be useful information for managers and decision makers. Care should be taken however in extrapolating the results from habitat changes to population changes.
Previously, the changes in morphology per habitat patch have also been investigated to identify how stable habitat patches are (see for example Wheaton et al. Citation2010). Due to the vast reworking of the topography we did not include such an analysis, as it was clear that most habitat patches had shifted entirely: large parts which were wetted in one topographical state did not remain wetted after a flood. In reaches with more limited reworking of the morphology such an analysis may add additional information.
We proposed the new metric RCI quantifying a reach’s habitat connectivity. RCI can theoretically take values between 0 and 1 and values over almost this entire spectrum were found. Since the metric had not been used previously, it is difficult to generalize the findings based on previous research at other field sites. Further investigation can assess how RCI differs between reaches with different topography, which can aid in the metric’s interpretability.
A reach’s topography influences its ecological state and is influenced by hydrological, geological and biological (e.g., vegetation) influences (Castro and Thorne Citation2019). Trees and other plants could not grow sufficiently in the few months between the floods to influence the hydro- or morphodynamics. Since trees were also removed, the biological influence on the field site likely progressively reduced while the hydrological influence progressively increased (e.g., Castro and Thorne Citation2019). In pre-floods the reach was already in a topographical state that was mostly determined by hydrological and geological influences, typical for Alpine rivers (c.f. Castro and Thorne Citation2019). The reduction of the biological influence on the topography therefore did not alter the topographical type. Habitat shifts in rivers where the topography is dominated by other influences are likely to be different. Results from this study should not be extrapolated to such rivers and separate research should be carried out.
6. Conclusions
Floods can change the long-term behaviour of river ecosystems by altering the habitat template. By investigating subsequent floods we can improve our understanding of the natural variability inherent in the ecological functioning of rivers.
We investigated a braided reach of the Moesa river in southern Switzerland which was subject to multiple flooding events. The reach’s topography changed with each flood and is aggradational. The reach is not in a morphological equilibrium. The first and largest flood increased depths and widened the flow velocity distribution. The subsequent floods returned these parameters to the pre-floods conditions.
The HMID and dewatered area followed this same pattern of an initial deviation and then a return to pre-floods values. However, for some metrics (RCI) no clear pattern was identifiable. Habitat types that also depend directly on depth and flow velocity, did not change unidirectionally too. The changing joint depth-velocity distribution determined the changes in habitat types. Habitat types’ prevalences change within the bounds of a dynamic equilibrium. Despite a clear trend towards an alternative morphological state in this reach, a move towards an alternative ecological state seems absent.
Even if there is no movement towards an alternative ecological state, the changes in habitat prevalences can be large. The changes are dependent on seasons and hydropower water release. A full analysis of habitat effects due to topographical changes thus requires the consideration of the full complexity of the flow regime.
Few prior studies investigated the effects of multiple floods on habitats at a single river reach. Since the investigated reach has similar characteristics to many river widening projects, the results can aid in the management of widened reaches. This study can also contribute to the proper identification of limitations on hydropower under future changing conditions by shedding light on the flood-induced variability in ecological metrics. This research improves our knowledge of flood-induced fluvial habitat changes and contributes to the field of habitat modelling.
Supplemental Material
Download Zip (369.3 KB)Acknowledgements
This research is partly based on the Master Theses of Lea Toma and Patrick Paszti. The authors thank Antonetti Manuel, Micheal Döring (ZHAW, Wädenswil) and Daniel Gubser for their work and help using the drones, Thomas Posur and Elias Hödel for their help with the RTK GPS devices and Flavio Nollo and the Office for Hunting and Fishing Graubünden for the information they provided about the local conditions. Additionally the authors want to thank the three anonymous reviewers that helped improve this work.
Disclosure statement
No potential conflict of interest was reported by the authors.
Additional information
Funding
References
- Adamczyk M, Parasiewicz P, Vezza P, Prus P, De Cesare G. 2019. Empirical validation of mesoHABSIM models developed with different habitat suitability criteria for bullhead cottus gobio l. as an indicator species. Water. 11(4):726. https://doi.org/10.3390/w11040726.
- Agisoft Metashape Professional. 2020. Agisoft Metashape professional (version 1.6.4). https://www.agisoft.com/downloads/installer/.
- Amt für Natur und Umwelt. 2006–2021. Seba internetmodul. https://www.seba-hydrocenter.de/projects/index.php?user=anuchurpub.
- ASCE Task Committee on Sediment Transport and Aquatic Habitats, Sedimentation Committee. 1992. Sediment and aquatic habitat in river systems. J Hydraulic Engin. 118(5):669–687. https://doi.org/10.1061/(ASCE)0733-9429(1992)118:5(669).
- Ashmore P. 1991. Channel morphology and bed load pulses in braided, gravel-bed streams. Geografiska Annaler: Series A, Phys Geograph. 73(1):37–52. https://doi.org/10.1080/04353676.1991.11880331.
- Barillier A, Beche L, Malavoi JR, Gouraud V. 2021. Identification of effective hydropeaking mitigation measures: are hydraulic habitat models sufficient in a global approach? J Ecohydraulics. 6(2):172–185.
- Baumann P, Kirchhofer A, Schälchli U. 2012. Sanierung Schwall/Sunk – Strategische Planung. Ein Modul der Vollzugshilfe Renaturierung der Gewässer. Bundesamt für Umwelt, Bern Umwelt-Vollzug. 1203; p. 126. https://www.bafu.admin.ch/bafu/de/home/themen/wasser/publikationen-studien/publikationen-wasser/sanierung-schwall-sunk.html.
- Bischoff A, Wolter C. 2001. The flood of the century on the river order: effects on the 0+ fish community and implications for floodplain restoration. Regul Rivers: Res Mgmt. 17(2):171–190. https://doi.org/10.1002/rrr.612.
- Brennan SR, Schindler DE, Cline TJ, Walsworth TE, Buck G, Fernandez DP. 2019. Shifting habitat mosaics and fish production across river basins. Science. 364(6442):783–786. https://doi.org/10.1126/science.aav4313.
- Brunner GW. 2016. HEC-RAS, River Analysis System Hydraulic Reference Manual - Hydraulic Reference Manual, U.S. Army Corps of Engineers, report number: CPD-69. https://www.hec.usace.army.mil/software/hec-ras/documentation/HEC-RAS%205.0%20Reference%20Manual.pdf.
- Bundesamt für Umwelt BAFU. 2020. Hydrologische daten und vorhersagen; https://www.hydrodaten.admin.ch/de/2420.html. [accessed 2020 December 15].
- Carline RF, McCullough BJ. 2003. Effects of floods on brook trout populations in the Monongahela national forest, West Virginia. Trans Am Fish Soc. 132(5):1014–1020. https://doi.org/10.1577/T02-112.
- Castro JM, Thorne CR. 2019. The stream evolution triangle: Integrating geology, hydrology, and biology. River Res Applic. 35(4):315–326. https://doi.org/10.1002/rra.3421.
- Castro-Santos T, Sanz-Ronda FJ, Ruiz-Legazpi J. 2013. Breaking the speed limit—comparative sprinting performance of brook trout (salvelinus fontinalis) and brown trout (salmo trutta). Can J Fish Aquat Sci. 70(2):280–293. https://doi.org/10.1139/cjfas-2012-0186.
- Cote D, Kehler DG, Bourne C, Wiersma YF. 2009. A new measure of longitudinal connectivity for stream networks. Landscape Ecol. 24(1):101–113. https://doi.org/10.1007/s10980-008-9283-y.
- Cotel AJ, Webb PW, Tritico H. 2006. Do brown trout choose locations with reduced turbulence? Trans Am Fish Soc. 135(3):610–619. https://doi.org/10.1577/T04-196.1.
- De Jalón DG, Gortazar J. 2007. Evaluation of instream habitat enhancement options using fish habitat simulations: case-studies in the river pas (Spain). Aquatic Ecol. 41(3):461–474. https://doi.org/10.1007/s10452-006-9030-x.
- Dolloff CA, Flebbe PA, Owen MD. 1994. Fish habitat and fish populations in a southern appalachian watershed before and after hurricane Hugo. Trans Am Fish Soc. 123(4):668–678. https://doi.org/10.1577/1548-8659(1994)123<0668:FHAFPI>2.3.CO;2.
- Dönni W, Boller L, Zaugg C. 2016. Mindestwassertiefen für See-und Bachforellen biologische Grundlagen und Empfehlungen. Studie im Auftrag des Bundesamtes für Umwelt. Schweiz: Fischwerk.
- Elliott J, Elliott J. 2010. Temperature requirements of Atlantic salmon salmo salar, brown trout salmo trutta and arctic charr salvelinus alpinus: predicting the effects of climate change. J Fish Biol. 77(8):1793–1817. https://doi.org/10.1111/j.1095-8649.2010.02762.x.
- Elwood JW, Waters TF. 1969. Effects of floods on food consumption and production rates of a stream brook trout population. Trans Am Fish Soc. 98(2):253–262. https://doi.org/10.1577/1548-8659(1969)98[253:EOFOFC]2.0.CO;2.
- Farò D, Zolezzi G, Wolter C. 2021. How much habitat does a river need? A spatially-explicit population dynamics model to assess ratios of ontogenetical habitat needs. J Environ Manage. 286:112100. https://doi.org/10.1016/j.jenvman.2021.112100.
- Glova GJ, Duncan MJ. 1985. Potential effects of reduced flows on fish habitats in a large braided river, New Zealand. Trans Am Fish Soc. 114(2):165–181. https://doi.org/10.1577/1548-8659(1985)114<165:PEORFO>2.0.CO;2.
- Gostner W, Alp M, Schleiss AJ, Robinson CT. 2013. The hydro-morphological index of diversity: a tool for describing habitat heterogeneity in river engineering projects. Hydrobiologia. 712(1):43–60. https://doi.org/10.1007/s10750-012-1288-5.
- Gostner W, Annable W, Schleiss A, Paternolli M. 2021. A case-study evaluating river rehabilitation alternatives and habitat heterogeneity using the hydromorphological index of diversity. J Ecohydraulics. 6(1):1–16. https://doi.org/10.1080/24705357.2019.1680320.
- Greimel F, Schülting L, Graf W, Bondar-Kunze E, Auer S, Zeiringer B, Hauer C. 2015. Hydropeaking impacts and mitigation. Riverine Ecosystem Manage. 8:91–110. https://doi.org/10.1002/rra.2653.
- Hajdukiewicz H, Wyżga B, Mikuś P, Zawiejska J, Radecki-Pawlik A. 2016. Impact of a large flood on mountain river habitats, channel morphology, and valley infrastructure. Geomorphology. 272:55–67. https://doi.org/10.1016/j.geomorph.2015.09.003.
- Halleraker J, Saltveit S, Harby A, Arnekleiv J, Fjeldstad HP, Kohler B. 2003. Factors influencing stranding of wild juvenile brown trout (salmo trutta) during rapid and frequent flow decreases in an artificial stream. River Res Applic. 19(5–6):589–603. https://doi.org/10.1002/rra.752.
- Harrison L, Legleiter C, Wydzga M, Dunne T. 2011. Channel dynamics and habitat development in a meandering, gravel bed river. Water Resour Res. 47(4):1–21. https://doi.org/10.1029/2009WR008926.
- Harrison LR, Pike A, Boughton DA. 2017. Coupled geomorphic and habitat response to a flood pulse revealed by remote sensing. Ecohydrol. 10(5):e1845. https://doi.org/10.1002/eco.1845.
- Hayes DS, Moreira M, Boavida I, Haslauer M, Unfer G, Zeiringer B, Greimel F, Auer S, Ferreira T, Schmutz S. 2019. Life stage-specific hydropeaking flow rules. Sustainability. 11(6):1547. https://doi.org/10.3390/su11061547.
- Hohensinner S, Egger G, Muhar S, Vaudor L, Piégay H. 2021. What remains today of pre-industrial alpine rivers? census of historical and current channel patterns in the alps. River Res Applic. 37(2):128–149. https://doi.org/10.1002/rra.3751.
- Holzapfel P, Leitner P, Habersack H, Graf W, Hauer C. 2017. Evaluation of hydropeaking impacts on the food web in alpine streams based on modelling of fish-and macroinvertebrate habitats. Sci Total Environ. 575:1489–1502. https://doi.org/10.1016/j.scitotenv.2016.10.016.
- Hong LB, Davies T. 1979. A study of stream braiding. Geol Soc Am Bull. 90(12_Part_II):1839–1859. https://doi.org/10.1130/GSAB-P2-90-1839.
- Huusko A, Greenberg L, Stickler M, Linnansaari T, Nykänen M, Vehanen T, Koljonen S, Louhi P, Alfredsen K. 2007. Life in the ice lane: the winter ecology of stream salmonids. River Res Applic. 23(5):469–491. https://doi.org/10.1002/rra.999.
- Jain A. 2010. Data clustering: 50 years beyond k-means. Pattern Recog Lett. 31(8):651–666. https://doi.org/10.1016/j.patrec.2009.09.011.
- Jakober MJ, McMahon TE, Thurow RF. 2000. Diel habitat partitioning by bull charr and cutthroat trout during fall and winter in rocky mountain streams. Environ Biol Fishes. 59(1):79–89. https://doi.org/10.1023/A:1007699610247.
- Jonsson B, Jonsson N. 2009. A review of the likely effects of climate change on anadromous atlantic salmon salmo salar and brown trout salmo trutta, with particular reference to water temperature and flow. J Fish Biol. 75(10):2381–2447. https://doi.org/10.1111/j.1095-8649.2009.02380.x.
- Judes C, Gouraud V, Capra H, Maire A, Barillier A, Lamouroux N. 2021. Consistent but secondary influence of hydropeaking on stream fish assemblages in space and time. J Ecohydraul. 6(2):157.
- Junk WJ. 2005. Flood pulsing and the linkages between terrestrial, aquatic, and wetland systems. Int Vereinigung Für Theoretische Und Angewandte Limnologie: Verhandlungen. 29(1):11–38. https://doi.org/10.1080/03680770.2005.11901972.
- Kurth AM, Schirmer M. 2014. Thirty years of river restoration in Switzerland: implemented measures and lessons learned. Environ Earth Sci. 72(6):2065–2079. https://doi.org/10.1007/s12665-014-3115-y.
- Larsen LG, Woelfle-Erskine C. 2018. Groundwater is key to salmonid persistence and recruitment in intermittent mediterranean-climate streams. Water Resour Res. 54(11):8909–8930. https://doi.org/10.1029/2018WR023324.
- Liébault F, Lallias-Tacon S, Cassel M, Talaska N. 2013. Long profile responses of alpine braided rivers in SE France. River Res Applic. 29(10):1253–1266. https://doi.org/10.1002/rra.2615.
- Milner AM, Robertson AL, McDermott MJ, Klaar MJ, Brown LE. 2013. Major flood disturbance alters river ecosystem evolution. Nature Clim Change. 3(2):137–141. https://doi.org/10.1038/nclimate1665.
- Niayifar A, Oldroyd HJ, Lane SN, Perona P. 2018. Modeling macroroughness contribution to fish habitat-suitability curves. Water Resour Res. 54(11):9306–9320. https://doi.org/10.1029/2018WR022860.
- Niemi GJ, DeVore P, Detenbeck N, Taylor D, Lima A, Pastor J, Yount JD, Naiman RJ. 1990. Overview of case studies on recovery of aquatic systems from disturbance. Environ Manage. 14(5):571–587.
- Null S, Mouzon N, Elmore L. 2017. Dissolved oxygen, stream temperature, and fish habitat response to environmental water purchases. J Environ Manage. 197:559–570. http://dx.doi.org/10.1016/j.jenvman.2017.04.016.
- Parasiewicz P, King EL, Webb JA, Piniewski M, Comoglio C, Wolter C, Buijse AD, Bjerklie D, Vezza P, Melcher A, et al. 2019. The role of floods and droughts on riverine ecosystems under a changing climate. Fish Manag Ecol. 26(6):461–473. https://doi.org/10.1111/fme.12388.
- Parasiewicz P, Ryan K, Vezza P, Comoglio C, Ballestero T, Rogers J. 2013. Use of quantitative habitat models for establishing performance metrics in river restoration planning. Ecohydrol. 6(4):668–678. https://doi.org/10.1002/eco.1350.
- Pasternack GB, Wyrick JR. 2017. Flood-driven topographic changes in a gravel-cobble river over segment, reach, and morphological unit scales. Earth Surf Process Landforms. 42(3):487–502. https://doi.org/10.1002/esp.4064.
- Paszti P. 2019. Study of fish mesohabitat dynamics in the moesa river. [Master thesis]. ETH Zürich.
- Pearsons TN, Li HW, Lamberti GA. 1992. Influence of habitat complexity on resistance to flooding and resilience of stream fish assemblages. Trans Am Fish Soc. 121(4):427–436. https://doi.org/10.1577/1548-8659(1992)121<0427:IOHCOR>2.3.CO;2.
- Person E. 2013. Impact of hydropeaking on fish and their habitat. EPFL-LCH. ISSN 1661–1179.
- Person E, Bieri M, Peter A, Schleiss AJ. 2014. Mitigation measures for fish habitat improvement in alpine rivers affected by hydropower operations. Ecohydrol. 7(2):580–599. https://doi.org/10.1002/eco.1380.
- Pix4dMapper. 2021. Pix4dMapper. https://www.pix4d.com/product/pix4dmapper-photogrammetry-software.
- Rachelly C, Mathers KL, Weber C, Weitbrecht V, Boes RM, Vetsch DF. 2021. How does sediment supply influence refugia availability in river widenings? J Ecohydraulics. 6(2):121–138. https://doi.org/10.1080/24705357.2020.1831415.
- Reeves GH, Benda LE, Burnett KM, Bisson PA, Sedell JR. 1995. A disturbance-based ecosystem approach to maintaining and restoring freshwater habitats of evolutionarily significant units of anadromous salmonids in the Pacific Northwest. In American Fisheries Society Symposium. 17: p. 334–349.
- Roghair CN, Dolloff CA, Underwood MK. 2002. Response of a brook trout population and instream habitat to a catastrophic flood and debris flow. Trans Am Fish Soc. 131(4):718–730. https://doi.org/10.1577/1548-8659(2002)131<0718:ROABTP>2.0.CO;2.
- Rohde S, Kienast F, Bürgi M. 2004. Assessing the restoration success of river widenings: a landscape approach. Environ Manage. 34(4):574–589. https://doi.org/10.1007/s00267-004-0158-y.
- Rosenfeld J, Leiter T, Lindner G, Rothman L. 2005. Food abundance and fish density alters habitat selection, growth, and habitat suitability curves for juvenile coho salmon (oncorhynchus kisutch). Can J Fish Aquat Sci. 62(8):1691–1701. https://doi.org/10.1139/F05-072.
- Sawyer AM, Pasternack GB, Moir HJ, Fulton AA. 2010. Riffle-pool maintenance and flow convergence routing observed on a large gravel-bed river. Geomorphology. 114(3):143–160. https://doi.org/10.1016/j.geomorph.2009.06.021.
- Schmutz S, Jurajda P, Kaufmann S, Lorenz AW, Muhar S, Paillex A, Poppe M, Wolter C. 2016. Response of fish assemblages to hydromorphological restoration in central and northern European rivers. Hydrobiologia. 769(1):67–78. https://doi.org/10.1007/s10750-015-2354-6 .
- Stähly S, Franca MJ, Robinson CT, Schleiss AJ. 2019. Sediment replenishment combined with an artificial flood improves river habitats downstream of a dam. Sci Rep. 9(1):1–8. https://doi.org/10.1038/s41598-019-41575-6.
- Stanford JA, Lorang M, Hauer F. 2005. The shifting habitat mosaic of river ecosystems. Int Vereinigung Für Theoretische Und Angewandte Limnologie: Verhandlungen. 29(1):123–136. https://doi.org/10.1080/03680770.2005.98111901979 .
- Tamminga A, Eaton B. 2018. Linking geomorphic change due to floods to spatial hydraulic habitat dynamics. Ecohydrology. 11(8):e2018. https://doi.org/10.1002/eco.2018.
- Thomson J, Taylor M, Fryirs K, Brierley G. 2001. A geomorphological framework for river characterization and habitat assessment. Aquatic Conserv: Mar Freshw Ecosyst. 11(5):373–389. https://doi.org/10.1002/aqc.467.
- Turner MG, Dale VH. 1998. Comparing large, infrequent disturbances: what have we learned? Ecosystems 1998:493–496. https://www.jstor.org/stable/3658750.
- Van der Nat D, Tockner K, Edwards PJ, Ward J, Gurnell AM. 2003. Habitat change in braided flood plains (tagliamento, ne-italy). Freshwater Biol. 48(10):1799–1812. https://doi.org/10.1046/j.1365-2427.2003.01126.x.
- van Rooijen E, Vanzo D, Vetsch DF, Boes RM, Siviglia A. 2021. Enhancing an unsupervised clustering algorithm with a spatial contiguity constraint for river habitat analysis. Ecohydrology. 14(4):e2285. https://doi.org/10.1002/eco.2285.
- Vanzo D, Peter S, Vonwiller L, Bürgler M, Weberndorfer M, Siviglia A, Conde D, Vetsch DF. 2021. Basement v3: a modular freeware for river process modelling over multiple computational backends. Environ Modell Software. 143:105102. https://doi.org/10.1016/j.envsoft.2021.1051.
- Vanzo D, Zolezzi G, Siviglia A. 2016. Eco-hydraulic modelling of the interactions between hydropeaking and river morphology. Ecohydrol. 9(3):421–437.
- Vezza P, Parasiewicz P, Calles O, Spairani M, Comoglio C. 2014a. Modelling habitat requirements of bullhead (cottus gobio) in alpine streams. Aquat Sci. 76(1):1–15. https://doi.org/10.1007/s00027-013-0306-7.
- Vezza P, Parasiewicz P, Spairani M, Comoglio C. 2014b. Habitat modeling in high-gradient streams: the mesoscale approach and application. Ecol Appl. 24(4):844–861. https://doi.org/10.1890/11-2066.1.
- Vismara R, Azzellino A, Bosi R, Crosa G, Gentili G. 2001. Habitat suitability curves for brown trout (salmo trutta fario l.) in the river adda, northern italy: comparing univariate and multivariate approaches. Regul Rivers: Res Mgmt. 17(1):37–50. https://doi.org/10.1002/1099-1646(200101/02)17:1<37::AID-RRR606>3.0.CO;2-Q.
- Warburton J, Davies T, Mandl M. 1993. A meso-scale field investigation of channel change and floodplain characteristics in an upland braided gravel-bed river, New Zealand. SP. 75(1):241–255. https://doi.org/10.1144/GSL.SP.1993.075.01.15.
- Weng Z, Mookerji N, Mazumder A. 2001. Nutrient-dependent recovery of atlantic salmon streams from a catastrophic flood. Can J Fish Aquat Sci. 58(8):1672–1682. https://doi.org/10.1139/f01-107.
- Wheaton JM, Brasington J, Darby SE, Merz J, Pasternack GB, Sear D, Vericat D. 2010. Linking geomorphic changes to salmonid habitat at a scale relevant to fish. River Res Applic. 26(4):469–486. https://doi.org/10.1002/rra.1305.
- Wohl E, Lane SN, Wilcox AC. 2015. The science and practice of river restoration. Water Resour Res. 51(8):5974–5997. https://doi.org/10.1002/2014WR016874.
- Woodworth KA, Pasternack GB. 2022. Are dynamic fluvial morphological unit assemblages statistically stationary through floods of less than ten times bankfull discharge? Geomorphology. 403:108135. https://doi.org/10.1016/j.geomorph.2022.108135.
- Zolezzi G, Bellin A, Bruno M, Maiolini B, Siviglia A. 2009. Assessing hydrological alterations at multiple temporal scales: Adige River, Italy. Water Resour Res. 45(12):1–15. https://doi.org/10.1029/2008WR007266.