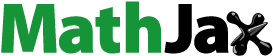
Abstract
Objective
The purpose of this study was to explore the effects of pain on functions and the relationship between functional changes in the hippocampus and attention and anxiety in a pain-sensitive population.
Methods
Fifty-three healthy subjects with no chronic pain were recruited in this study and divided into a pain-sensitive group (PS, n = 26) and a pain-tolerant group (PT, n = 27) according to the cold pressor test (CPT). Functional magnetic resonance imaging (fMRI) with a cold bottle test (cold and control conditions) was performed. The static and dynamic functional connectivity (FC) of the hippocampus was analyzed in both groups for the two conditions.
Results
Compared with PT, there were fewer static functional connections between the right hippocampus and the bilateral dorsolateral superior frontal gyrus in the PS group. Moreover, reduced functional connections between the hippocampus and brain regions were related to anxiety in the PS group, including the inferior temporal gyrus and the middle temporal gyrus.
Significance
Our results found that pain disturbed the default mode network in the PS group, which may be related to self-awareness. This result may reflect that people who are sensitive to pain are more likely to feel anxious.
The functional connectivity within the DMN in the pain-sensitive group was significantly reduced, indicating the relationship between self-awareness and pain.
The functional connectivity in the pain-sensitive group was significantly reduced in the hippocampus and some brain regions associated with anxiety, possibly reflecting the effect of pain on anxiety.
Key Points
1. Introduction
Pain is a conscious experience that is essential for survival [Citation1]. The International Association for the Study of Pain (IASP) defines pain as ‘an unpleasant sensory and emotional experience associated with actual or potential tissue damage or described in terms of such damage [Citation2]. Everyone has different sensitivities to pain. Pain tolerance is extremely important in some respects [Citation3,Citation4]. Research has found that expectation and distraction are two effective ways to control pain and can greatly relieve pain [Citation5]. The mechanism by which attention affects pain is important but unclear in the current study. Therefore, it is necessary to study this mechanism to cope with and relieve pain.
Studies dating back to the twentieth century found that shifting attention can relieve pain [Citation6]. Notably, top-down and bottom-up modulations of pain by attention are cognitive regulatory mechanisms explaining acute and chronic pain perception [Citation7]. In addition, previous studies have found that there is a correlation between self-awareness and the anti-pain system. For example, individuals who show high intrinsic attention to pain have weaker structural connections and less flexible dynamic functional connections between the DMN and the periaqueductal gray [Citation8]. A recent review described how pain memory affects the intensity and threshold of pain responses by altering pain pathways [Citation9]. Previous studies have found that the DMN plays roles in attention, expectation, alertness, memory, etc [Citation10–13]. Therefore, it is necessary to study the influence of the default mode network on the mechanism of pain tolerance.
In addition, the hippocampus is one of the most important core brain areas in the default mode network. The anxiety generated during the pain expectation process can regulate the subjective experience of pain. In recent years, there has been much research into pain due to its strong association with emotion. A recent article found that participants with higher levels of anxiety showed a significant performance advantage when it came to encoding painful stimuli[Citation14]. Some of these studies were about nociceptive pain and empathic pain (e.g. the experience of seeing a friend hurt produces empathic pain). There is much evidence that the activation patterns of nociceptive and empathic pain overlap [Citation15]. In addition, a recent meta-analysis found that pain-related emotions triggered hippocampal activation [Citation16]. The hippocampus has a regulatory effect on the emotions produced by pain. However, the regulatory mechanism of the hippocampus on pain is still unclear.
In the past few decades, as a noninvasive technique, magnetic resonance imaging (MRI) has been widely used in many research fields [Citation17,Citation18]. Functional magnetic resonance imaging (fMRI) provides a new method for studying the activity of pain-related brain neurons. Moreover, MRI can study pain-related brain areas from multiple perspectives, such as structure and function. Functional connectivity (FC) is estimated from the statistical relationship between fMRI blood oxygen level-dependent (BOLD) signals of different brain regions. Some research now posits that pain regulation is a dynamic process [Citation19]. Therefore, dynamic functional connections (dFCs) have received increasing attention in recent years. The sliding window-based method is the most common method of measuring dFCs. It divides the BOLD signals of different brain regions into a series of data segments and calculates the time correlation of each data segment [Citation20].
To determine the basic neural mechanism of pain, previous studies applied noxious stimulation (including heat stimulation, cold stimulation, and pressure stimulation) to subjects and collected useful data by noninvasive methods for analysis [Citation4,Citation21–23]. We take into account the previous research results and our research direction. To distinguish the subjects’ tolerance to pain, we used the cold pressor test (CPT) to divide the subjects into two groups. We hypothesized that (1) the difference in pain sensitivity can be reflected in the attention to pain and (2) the difference in functional connectivity between the two groups is related to anxiety and memory.
2. Methods
2.1. Participants
We recruited 53 male subjects at the University of Electronic Science and Technology of China. Subjects were excluded if they had acute or chronic pain, a history of related neurological symptoms, a history of smoking, psychiatric diseases, or MR contraindications. The study was approved by the Ethics Committee of Chengdu Mental Health Centre. All participants provided written informed consent.
2.2. Behavior evaluation
We used the Cold Pressor Test (CPT) to determine a person’s pain sensitivity. In our experiment, we prepared a bucket filled with water and ice to keep the water temperature at 1 ± 0.5 °C. Subjects put their right hand and wrist in ice water. At the same time, we began to record the subject’s verbal pain score scale and endurance time. When the subject could not tolerate the pain caused by the ice water, he could quickly withdraw his hand. From the beginning of the recording time, the subject’s verbal pain score was recorded every 15 s until the end of the CPT. Finally, subjects who endured the cold pressure test for more than 3 min were identified as the pain-tolerant group, and the subjects who endured it for less than one and a half minutes were identified as the pain-sensitive group. We excluded 20 participants who endured the cold pressure test for between one and a half minutes and three minutes. The remaining participants were assigned to two groups (26 Pain-sensitive: 23.0 ± 1.6 years, 27 Pain-tolerant: 22.5 ± 2.0 years) according to the CPT. We used the State-Trait Anxiety Inventory (STAI) and the fear rating scale to assess participants’ mentation.
2.3. FMRI cold task design
After the subjects completed the CPT experiment, they performed the cold bottle test (CBT), with at least one day between the tests. The CBT process involves holding a glass bottle filled with water at different temperatures. There were two bottle temperatures in our experiment: 8 °C (cold condition) and 26 °C (control condition). The two groups of subjects completed the CBT during the MRI scan, holding the glass bottle with their right hand, and the glass bottle experiment at each temperature lasted 4 min 10 s. After completing a stimulus scan sequence, a 3-minute rest was required until the subject’s right hand returned to normal body temperature. During the 3-minute rest interval, subjects completed a verbal rating scale (VRS) [Citation24].
2.4. Image acquisition
Brain imaging data were acquired using a 3-T MRI (GE DISCOVERY MR750, USA) scanner at the Centre for Information in Medicine of the University of Electronic Science and Technology of China. To reduce the subject’s head motion during the scan, the soft foam was used to fix the subject’s head during the scan, and the subject used earplugs to reduce their perception of the scanning noise produced by the MRI scanner. An echo-planar imaging (EPI) sequence was used to obtain task-state and rest-state fMRI data. The scanning parameters of the task state were as follows: repetition time (TR) = 2000 ms; echo time (TE) = 30 ms; flip angle (FA) = 90°; voxel size = 3.0 × 3.0 × 3.0 mm³; field of view (FOV) = 240 mm ×240 mm; matrix size = 64 × 64; slice thickness = 4 mm; and slice = 35. In addition, high-resolution T1-weighted structural images were collected using a three-dimensional fast spoiled gradient echo (3D FSPGR). The parameters were as follows: TR = 6.008 ms; TE = 1.984 ms; FA = 9°; voxel size = 1.0 × 1.0 × 1.0 mm³; matrix size = 256 × 256; FOV = 256 mm × 256 mm; slice thickness = 1 mm; slice =154. Diffusion tensor imaging was performed using a single-shot EPI sequence. The parameters were as follows: TR = 8500 ms; TE = 70 ms; voxel size = 2.0 × 2.0 × 2.0 mm³; FOV = 256 mm× 256 mm; slice = 77; dispersion directions = 64; value of b = 1000 s/mm2; and three b0 maps. All participants were told to relax, close their eyes and not fall asleep. Each subject was scanned in two task states under two conditions (cold and control). Each functional task-state session lasted 250 s, with 125 time points in total. In this study, we used only the task-state session.
2.5. Data preprocessing
The NIT toolkit [Citation25] was used to preprocess the functional magnetic resonance data. The preprocessing steps included (1) removing the first five time points; (2) slice timing; (3) realignment; (4) normalizing to MNI space (3 × 3 × 3 mm³) using segment information; (5) bandpass filtering (0.01–0.08 HZ); (6) smoothing (FWHM = 6 mm); (7) nuisance signal regression, including white matter signal, whole cerebrospinal fluid signal and 12-parameter motion correction.
2.6. Functional connectivity and statistical analysis
The functional connectivity (FC) of the hippocampus was investigated in both groups for two conditions (cold and control). First, we divided the hippocampus into eight subregions based on previous literature (three on the left and five on the right) [Citation26]. Then, static FC and sliding-window-based dynamic FC analyses were performed to assess the subregion networks of the hippocampus by MATLAB code. Finally, repeated-measures ANOVA was used to obtain the group (PS and PT) *temperature interaction (Cold and Control) effects. The results of ANOVA were corrected by Gaussian random field (GRF) theory (voxel p < 0.001, cluster p < 0.05).
Moreover, to investigate the relationships between different brain areas and scores, we extracted the FC values of brain regions with significant interaction effects. Because of the nonnormal distribution of behavioral variables, correlations were conducted using Spearman’s rank correlation. Then, we calculated the Spearman correlation between the FC value and the scale score. In addition, we believe that ΔVRS () reflects the degree of change in pain perception. Therefore, we calculated the Spearman correlation between ΔVRS and ΔFC (
).
3. Results
3.1. Demographics and scale scores
The demographic and scale scores of the subjects are presented in . There was no difference in age (t test, p = 0.328) between the two groups. Both subject groups showed similar anxiety scores and fear scores.
Table 1. Demographic and scale scores.
3.2. Differences in functional connectivity
First, for dynamic FC, repeated-measures ANOVA showed a group*temperature interaction in dynamic FC between the left Hip1 and the right inferior temporal gyrus. Through post hoc tests, we found increased FC within the PT and decreased FC in the PS ().
Figure 1. Significant interactions of dynamic FC between PS and PT at two temperatures. (a) Brain regions with significant interactions (GRF, voxel p < 0.001, cluster p < 0.05). (b) Interaction effect diagram of the brain area (*indicates a significant difference, *p < 0.01, **p < 0.001).

Then, for static FC, repeated-measures ANOVA showed a group*temperature interaction in static FC between the left Hip2 and the right inferior temporal gyrus. Through post hoc tests, we found increased FC within the PT and decreased FC in the PS (). Additional repeated-measures ANOVA showed a group*temperature interaction in static FC between the right Hip2 and four regions, including the left middle temporal gyrus, left orbital middle frontal gyrus, left dorsolateral superior frontal gyrus, and right dorsolateral superior frontal gyrus. Through post hoc tests, we found increased FC within the PT and decreased FC in the PS ().
Figure 2. Significant interactions of static FC between PS and PT at the two temperatures. (a) Brain regions with significant interactions (GRF, voxel p < 0.001, cluster p < 0.05). (b) Interaction effect diagram of the brain area (* indicates a significant difference, * p < 0.01, **p < 0.001).

Figure 3. Significant interactions of static FC between PS and PT at two temperatures. (a) Brain regions with significant interactions (GRF, voxel p < 0.001, cluster p < 0.05). (b) Interaction effect diagram of the brain area corresponding to the first row (* indicates a significant difference, *p < 0.01, **p < 0.001).

3.3. Correlations between differential connectivity and scale score
A total of 48 correlation analyses were applied for the PT group (6 differential connectivity × 4 scale score × 2 temperatures). We found a significant correlation between functional connectivity (right Hip2 and left dorsolateral superior frontal gyrus) and VAS (Rho= –0.4405, p = 0.0215) in the PT group (). The same calculation was performed in the PS group, and no significant correlation was found. Then, to exclude the influence of age, we calculated partial correlations. No significant correlation was found between the two groups. In the PT group, a significant correlation was observed between the ΔFC (right Hip2 and right dorsolateral superior frontal gyrus) and the ΔVRS (Rho= -0.424, p = 0.0275) ().
Figure 4. (a). Significant correlation between FC and VRS in the PT group in the cold task. SFGdl: left dorsolateral superior frontal gyrus, X axis is FC: right Hip2 and left dorsolateral superior frontal gyrus; Y axis is VRS. p = 0.0215. (b). Significant correlation between ΔFC and ΔVRS in the PT group. The x-axis is ΔFC: right Hip2 and right dorsolateral superior frontal gyrus; the y-axis is ΔVRS: cold score-control score. p = 0.0275.

4. Discussion
To the best of our knowledge, this is the first study to investigate the relationship between pain sensitivity and hippocampal FC differences. As expected, the two main findings were as follows: (1) The main change in the functional connectivity of the PS group occurred inside the DMN, which disturbed the DMN system. We found decreased static FC between the Hip and the DMN in the PS group. (2) Pain is a negative stimulus that is more likely to cause changes in the anxiety circuit in the pain-sensitive group. We found decreased dynamic and static FC between the Hip and anxiety-related brain regions in the PS group.
A previous study found a negative correlation between pain sensitivity and DMN connectivity in the resting state [Citation27]. This study found that the difference in DMN connection strength between pain sensitivity and pain tolerance may explain some problems. The results of the study showed that during the rest period, the DMN of adolescents with higher pain frequency had stronger connectivity with the superior frontal gyrus [Citation28]. In a recent article, the author explored the relationship between adolescents’ default connectivity and the frequency and intensity of pain. Attention can be either internally focused on one’s own feelings or externally focused on stimuli in the environment. Default mode networks are thought to play an important role in this process [Citation29]. The regulatory influence of cognitive factors on pain perception has been identified in many processes, including attention, anticipation, (re)appraisal, and perception control of pain [Citation30,Citation31]. Studies have found that distraction can reduce the intensity of pain caused by experimental stimuli [Citation6]. Our results found decreased static FC between the right Hip2 and the bilateral dorsolateral superior frontal gyrus in the PS group. This may indicate that pain-sensitive people have more DMN connections when they are not experiencing pain, which may mean greater alertness or concentration. Furthermore, it may suggest that people who are sensitive to pain are more likely to feel pain. Constant pain input disturbs the DMN state (normal is a state of alert with higher functional connectivity). The PS group identified more intense pain inputs, so the disturbance was more significant, showing a decrease in FC. This is one reason why the PS group is more sensitive to pain. The significant correlation between FC (right Hip2 and left dorsolateral superior frontal gyrus) and VRS in the PT group showed that the larger the FC was, the higher the VAS score (i.e. the more pain felt). This result reflected that there was no significant change in DMN internal functional connectivity after pain in the PT group, and the degree of pain tolerance was relatively high. The functional connectivity of the PS group decreased significantly after temperature reduction, which laterally confirmed our hypothesis of pain sensitivity and attention. In addition, we observed an association between ΔFC and ΔVRS in the PT group, with a larger change in FC and a smaller change in VAS (i.e. a smaller change in perceived pain). This result may suggest that changes in the functional connectivity of the DMN can reduce the intensity of pain.
Studies have found that people with pain tolerance are less responsive to empathic pain [Citation32]. In generalized anxiety disorder, studies have found that the FC between the hippocampus and the temporal lobe is increased, which is mainly related to the fear circuit that produces anxiety [Citation33]. A recent study found that FC between the hippocampus and orbitofrontal cortex (OFC) correlated with anxiety levels [Citation34]. A previous study found that the middle temporal gyrus (MTG) plays a key role in suppressing emotional responses [Citation35]. Recent research results indicate that in the hippocampal FC network, the MTG is an important brain area for obsession and anxiety [Citation34]. Past studies have found that hippocampal activity is related to increased arousal during the expected period of pain [Citation23]. Our results found that whether it is dynamic functional connectivity or static functional connectivity, significant differences were found in the functional connectivity between the hippocampus and temporal lobe in the PS group. However, we did not find a correlation between the scale score and the difference in functional connectivity. However, the scoring of the scale is completed without painful stimulation. This result may reflect that the PS group has a greater influence on the emotional circuit when stimulated by pain, and the PS group is more prone to anxiety.
Although there were some interesting findings in this study, it must be noted that there were also some limitations. First, the sample size of the experiment was relatively small. A larger sample size should be used to improve the reliability of the results. Second, no patients with chronic pain were recruited. The effect of chronic pain on brain pathways has not been evaluated. Third, the pain caused by ice-water stimulation cannot be quantitatively analyzed. Quantitative analysis can more accurately determine the degree of tolerance, and subsequent experiments can be carried out by applying quantitative pressure to a finger.
In conclusion, our results show that DMN is more disturbed by pain in people who are more sensitive to pain. Self-awareness and anxiety may be factors in the degree of pain sensitivity. This finding is helpful for future experimental design, and we can consider how to induce emotion and pain at the same time.
Ethical statement
This study was approved by the ethical committee of the University of Science and Technology of China in accordance with the standards of the Declaration of Helsinki. The Ethics Committee of the Clinical Hospital of Chengdu Brain Science Institute approved this study.
Patient consent
Written informed consent was signed by each participant before MRI scanning.
Acknowledgments
The authors are grateful to the participants for their support of this research.
Disclosure statement
No potential conflict of interest was reported by the author(s).
Additional information
Funding
References
- Iannetti GD, Mouraux A. From the neuromatrix to the pain matrix (and back). Exp Brain Res. 2010;205(1):1–12.
- Loeser JD, Treede RD. The Kyoto protocol of IASP basic pain terminology. Pain. 2008;137(3):473–477.
- Rabasco A, Andover MS. The interaction of dissociation, pain tolerance, and suicidal ideation in predicting suicide attempts. Psychiatry Res. 2020;284:112661.
- Lue Y-J, Wang H-H, Cheng K-I, et al. Thermal pain tolerance and pain rating in normal subjects: gender and age effects. Eur J Pain. 2018;22(6):1035–1042.
- Buhle JT, Stevens BL, Friedman JJ, et al. Distraction and placebo: two separate routes to pain control. Psychol Sci. 2012;23(3):246–253.
- Eccleston C, Crombez G. Pain demands attention: a cognitive-affective model of the interruptive function of pain. Psychol Bull. 1999;125(3):356–366.
- Legrain V, Damme SV, Eccleston C, et al. A neurocognitive model of attention to pain: behavioral and neuroimaging evidence. Pain. 2009;144(3):230–232.
- Kucyi A, Salomons TV, Davis KD. Mind wandering away from pain dynamically engages antinociceptive and default mode brain networks. Proc Natl Acad Sci U S A. 2013;110(46):18692–18697.
- Noel M, Palermo TM, Chambers CT, et al. Remembering the pain of childhood: applying a developmental perspective to the study of pain memories. Pain. 2015;156(1):31–34.
- Gilbert DT, Wilson TD. Prospection: experiencing the future. Science. 2007;317(5843):1351–1354.
- Buckner RL, Andrews-Hanna JR, Schacter DL. The brain’s default network: anatomy, function, and relevance to disease. Ann NY Acad Sci. 2008;1124:1–38.
- Andrews-Hanna JR, Reidler JS, Sepulcre J, et al. Functional-anatomic fractionation of the brain’s default network. Neuron. 2010;65(4):550–562.
- Mi Y, Xu L. Sleep loss and lack of social interaction: A summary interview. Brain-Apparatus Communication: A Journal of Bacomics. 2022.
- Tseng M-T, Kong Y, Eippert F, et al. Determining the neural substrate for encoding a memory of human pain and the influence of anxiety. J Neurosci. 2017;37(49):11806–11817.
- Zaki J, Ochsner KN. The neuroscience of empathy: progress, pitfalls and promise. Nat Neurosci. 2012;15(5):675–680.
- Biggs EE, Timmers I, Meulders A, et al. The neural correlates of pain-related fear: a meta-analysis comparing fear conditioning studies using painful and non-painful stimuli. Neurosci Biobehav Rev. 2020;119:52–65.
- Wang J, Zhang Y. Laparoscopic sleeve gastrectomy induces simultaneous changes in brain functions and structures that are associated with weight loss. Brain-Apparatus Communication: A Journal of Bacomics. 2022.
- Yao D, Qin Y, Zhang Y. From psychosomatic medicine, brain–computer interface to brain–apparatus communication. Brain-Apparatus Communication: A Journal of Bacomics. 2022.
- Kucyi A, Davis KD. The dynamic pain connectome. Trends Neurosci. 2015;38(2):86–95.
- Kiviniemi V, Vire T, Remes J, et al. A sliding time-window Ica reveals spatial variability of the default mode network in time. Brain Connect. 2011;1(4):339–347.
- Oliva V, Gregory R, Davies W-E, et al. Parallel cortical-brainstem pathways to attentional analgesia. Neuroimage. 2021;226:117548.
- Forkmann K, Wiech K, Ritter C, et al. Pain-specific modulation of hippocampal activity and functional connectivity during visual encoding. J Neurosci. 2013;33(6):2571–2581.
- Ziv M, et al. Individual sensitivity to pain expectancy is related to differential activation of the hippocampus and amygdala. Hum Brain Mapp. 2010;31(2):326–338.
- Ohnhaus EE, Adler R. Methodological problems in the measurement of pain: a comparison between the verbal rating scale and the visual analogue scale. Pain. 1975;1(4):379–384.
- Dong L, et al. Neuroscience information toolbox: an open source toolbox for EEG-fMRI multimodal fusion analysis. Front Neuroinform. 2018;12:56.
- Robinson JL, Barron DS, Kirby LAJ, et al. Neurofunctional topography of the human hippocampus. Hum Brain Mapp. 2015;36(12):5018–5037.
- Yuan Y, Zhang L, Li L, et al. Distinct dynamic functional connectivity patterns of pain and touch thresholds: a resting-state fMRI study. Behav Brain Res. 2019;375:112142.
- Jones SA, Morales AM, Holley AL, et al. Default mode network connectivity is related to pain frequency and intensity in adolescents. Neuroimage Clin. 2020;27:102326.
- Whitfield-Gabrieli S, Ford JM. Default mode network activity and connectivity in psychopathology. Annu Rev Clin Psychol. 2012;8(1):49–76.
- Bennett DLH, Woods CG. Painful and painless channelopathies. The Lancet Neurology. 2014;13(6):587–599.
- Wiech K. Deconstructing the sensation of pain: the influence of cognitive processes on pain perception. Science. 2016;354(6312):584–587.
- Danziger N, Prkachin KM, Willer JC. Is pain the price of empathy? The perception of others’ pain in patients with congenital insensitivity to pain. Brain. 2006;129(9):2494–2507.
- Cui H, Zhang J, Liu Y, et al. Differential alterations of resting-state functional connectivity in generalized anxiety disorder and panic disorder. Hum Brain Mapp. 2016;37(4):1459–1473.
- Li K, Zhang H, Wang B, et al. Hippocampal functional network: the mediating role between obsession and anxiety in adult patients with obsessive-compulsive disorder. World J Biol Psychiatry. 2020;21(9):685–695.
- Berlin HA, Schulz KP, Zhang S, et al. Neural correlates of emotional response inhibition in obsessive-compulsive disorder: a preliminary study. Psychiatry Res. 2015;234(2):259–264.