Figures & data
Figure 1. Proportions of agents staying with their object of choice given that it failed () on the y-axis by round on the x-axis; gray lines depict 100 runs for each neighborhood size k, black lines plot means per round; agents learn optimally.
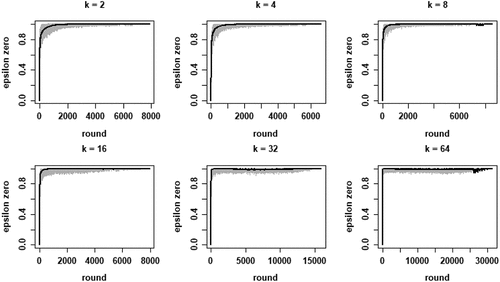
Figure 2. Mean proportions of agents staying with their object of choice given that it failed () on the y-axis by round on the x-axis; 100 runs for each neighborhood size k; black = 2 neighbors, blue = 4 neighbors, red = 8 neighbors, green = 16 neighbors, yellow = 32 neighbors, orange = 64 neighbors; agents learn optimally.

Figure 3. Boxplots of the distributions of Gini coefficients of the distribution of agent choices among high quality objects; Gini coefficients of 100 runs at convergence, per value of k.

Figure 4. Boxplots of total search costs until convergence (based on Eq. (5) summed over all rounds n per run) for all values of k; y-axis running from 0 to 350 for k equal to 2, 4, and 8, from 0 to 8000 for k equal to 16 and 32, and from 0 to 20,000 for k equal to 64.

Figure 5. Proportions of agents converging on high quality (HQ) object for each value of , for all values of k; 20 runs per value of
; solid lines depict mean proportions; dots are jittered for better representation.
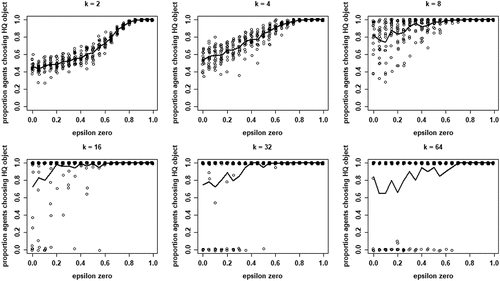
Figure 6. Gini coefficient values among high quality (HQ) objects in terms of numbers of agents choosing objects for each value of , for all values of k; 20 runs per value of
; data for runs that have at least one agent converging on an HQ object; solid lines depict mean Gini.

Figure 7. Search costs according to Eq. (5) for different values of , for each values of k; runs that end in all agents converging on a HQ object only.

Figure 8. Average outcomes of learning under the common knowledge of rationality assumption; the left panel shows mean search costs, the right panel shows mean Gini coefficients. Neighborhood sizes: black for k = 2, blue for k = 4, red for k = 8, green for k = 16, yellow for k = 32, and orange for k = 64.

Figure 9. Proportions of agents staying with their object of choice given that it failed () on the y-axis by round on the x-axis; gray lines depict 100 runs, blue lines plot means per round; the optimal learning scenario is depicted in the left-hand plot and the “most promising” common knowledge scenario in the right-hand plot.

Figure B1. Search costs according to Eq. (5) for different values of , for each values of k; all runs.
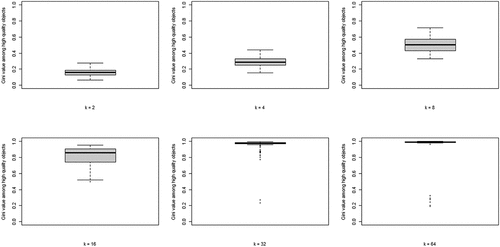
Figure B2. Proportions of agents choosing a HQ object at convergence for different values of , for each value of neighborhood size k; solid lines are mean proportions.
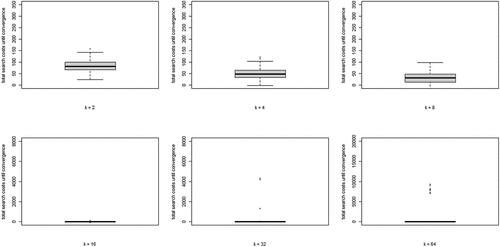
Figure B4. Search costs according to EquationEq. (5)(5)
(5) for different values of
across all runs for each value of k.

Figure B5. Search costs according to EquationEq. (5)(5)
(5) for different values of
for runs ending in all agents converging on a high-quality object only, for all values of k.

Figure B6. Box-plots of search costs in the optimal learning condition (left-hand) and the “most promising” common knowledge condition; neighborhood size k equals 2.

Figure B7. Boxplots if Gini coefficients at convergence in the optimal learning condition (left-hand) and the “most promising” common knowledge condition; neighborhood size k equals 2.
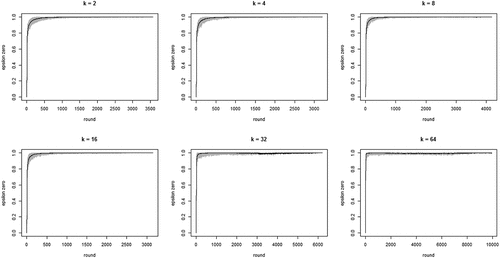
Table C1. Parameter values for robustness checks.
Figure C.1.1. Proportions of agents staying with their object of choice given that it failed () on the y-axis by round on the x-axis; gray lines depict 100 runs for each neighborhood size k, black lines plot means per round; agents learn optimally; RC0.

Figure C.1.2. Mean proportions of agents staying with their object of choice given that it failed () on the y-axis by round on the x-axis; 100 runs for each neighborhood size k; black = 2 neighbors, blue = 4 neighbors, red = 8 neighbors, green = 16 neighbors, yellow = 32 neighbors, orange = 64 neighbors; agents learn optimally; RC0.

Figure C.1.3. Boxplots of the distributions of Gini coefficients of the distribution of agent choices among high quality objects; Gini coefficients of 100 runs at convergence, per value of k; agents learn optimally; RC0.
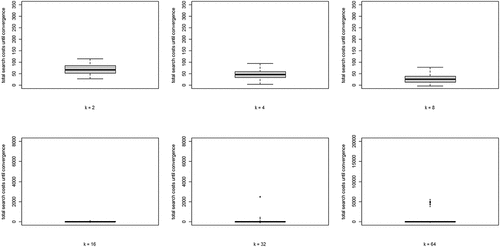
Figure C.1.4. Boxplots of total search costs until convergence (based on equation (5) summed over all rounds n per run) for all values of k; y-axis running from 0 to 350 for k equal to 2, 4, and 8, from 0 to 8000 for k equal to 16 and 32, and from 0 to 20,000 for k equal to 64; agents learn optimally; RC0.

Figure C.2.1. Proportions of agents staying with their object of choice given that it failed () on the y-axis by round on the x-axis; gray lines depict 100 runs for each neighborhood size k, black lines plot means per round; agents learn optimally; RC1a.
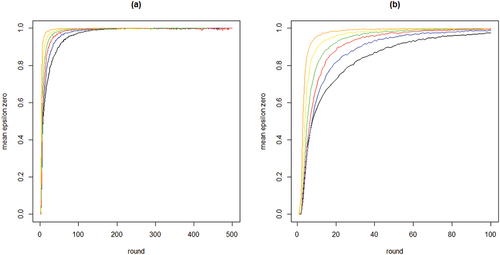
Figure C.2.2. Mean proportions of agents staying with their object of choice given that it failed () on the y-axis by round on the x-axis; 100 runs for each neighborhood size k; black = 2 neighbors, blue = 4 neighbors, red = 8 neighbors, green = 16 neighbors, yellow = 32 neighbors, orange = 64 neighbors; agents learn optimally; RC1a.

Figure C.2.3. Boxplots of the distributions of Gini coefficients of the distribution of agent choices among high quality objects; Gini coefficients of 100 runs at convergence, per value of k; agents learn optimally; RC1a.

Figure C.2.4. Boxplots of total search costs until convergence (based on EquationEq. (5)(5)
(5) summed over all rounds n per run) for all values of k; y-axis running from 0 to 350 for k equal to 2, 4, and 8, from 0 to 8000 for k equal to 16 and 32, and from 0 to 20,000 for k equal to 64; agents learn optimally; RC1a.

Figure C.3.1. Proportions of agents staying with their object of choice given that it failed () on the y-axis by round on the x-axis; gray lines depict 100 runs for each neighborhood size k, black lines plot means per round; agents learn optimally; RC1b.
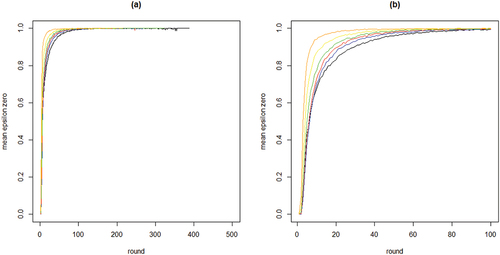
Figure C.3.2. Mean proportions of agents staying with their object of choice given that it failed () on the y-axis by round on the x-axis; 100 runs for each neighborhood size k; black = 2 neighbors, blue = 4 neighbors, red = 8 neighbors, green = 16 neighbors, yellow = 32 neighbors, orange = 64 neighbors; agents learn optimally; RC1b.

Figure C.3.3. Boxplots of the distributions of Gini coefficients of the distribution of agent choices among high quality objects; Gini coefficients of 100 runs at convergence, per value of k; agents learn optimally; RC1b.

Figure C.3.4. Boxplots of total search costs until convergence (based on EquationEq. (5)(5)
(5) summed over all rounds n per run) for all values of k; y-axis running from 0 to 350 for k equal to 2, 4, and 8, from 0 to 8000 for k equal to 16 and 32, and from 0 to 20,000 for k equal to 64; agents learn optimally; RC1b.
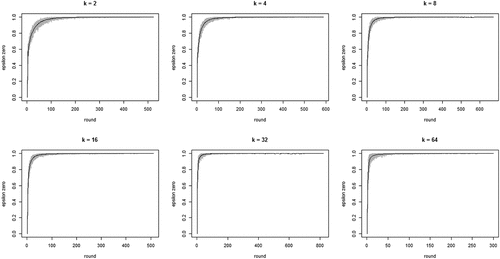
Figure C.4.1. Proportions of agents staying with their object of choice given that it failed ()on the y-axis by round on the x-axis; gray lines depict 100 runs for each neighborhood size k, black lines plot means per round; agents learn optimally; RC2a.
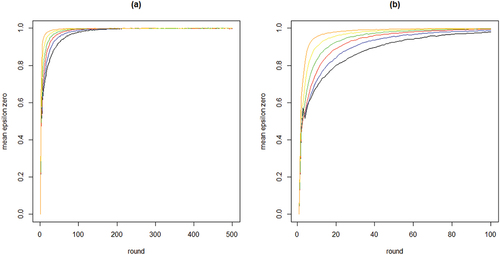
Figure C.4.2. Mean proportions of agents staying with their object of choice given that it failed () on the y-axis by round on the x-axis; 100 runs for each neighborhood size k; black = 2 neighbors, blue = 4 neighbors, red = 8 neighbors, green = 16 neighbors, yellow = 32 neighbors, orange = 64 neighbors; agents learn optimally; RC2a.

Figure C.4.3. Boxplots of the distributions of Gini coefficients of the distribution of agent choices among high quality objects; Gini coefficients of 100 runs at convergence, per value of k; agents learn optimally; RC2a.

Figure C.4.4. Boxplots of total search costs until convergence (based on EquationEq. (5)(5)
(5) summed over all rounds n per run) for all values of k; y-axis running from 0 to 350 for k equal to 2, 4, and 8, from 0 to 8000 for k equal to 16 and 32, and from 0 to 20,000 for k equal to 64; agents learn optimally; RC2a.
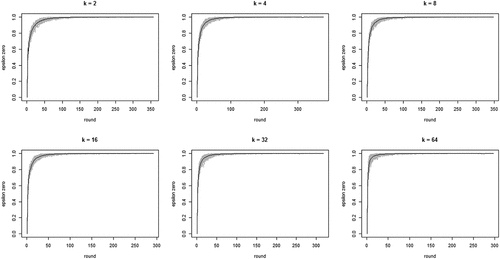
Figure C.5.1. Proportions of agents staying with their object of choice given that it failed () on the y-axis by round on the x-axis; gray lines depict 100 runs for each neighborhood size k, black lines plot means per round; agents learn optimally; RC2b.

Figure C.5.2. Mean proportions of agents staying with their object of choice given that it failed () on the y-axis by round on the x-axis; 100 runs for each neighborhood size k; black = 2 neighbors, blue = 4 neighbors, red = 8 neighbors, green = 16 neighbors, yellow = 32 neighbors, orange = 64 neighbors; agents learn optimally; RC2b.

Figure C.5.3. Boxplots of the distributions of Gini coefficients of the distribution of agent choices among high quality objects; Gini coefficients of 100 runs at convergence, per value of k; agents learn optimally; RC2b.
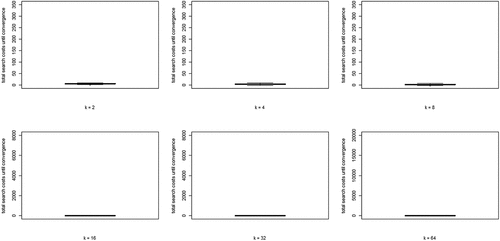
Figure C.5.4. Boxplots of total search costs until convergence (based on EquationEq. (5)(5)
(5) summed over all rounds n per run) for all values of k; y-axis running from 0 to 350 for k equal to 2, 4, and 8, from 0 to 8000 for k equal to 16 and 32, and from 0 to 20,000 for k equal to 64; agents learn optimally; RC2b.

Figure C.6.1. Proportions of agents staying with their object of choice given that it failed () on the y-axis by round on the x-axis; gray lines depict 100 runs for each neighborhood size k, black lines plot means per round; agents learn optimally; RC3a.

Figure C.6.2. Mean proportions of agents staying with their object of choice given that it failed () on the y-axis by round on the x-axis; 100 runs for each neighborhood size k; black = 2 neighbors, blue = 4 neighbors, red = 8 neighbors, green = 16 neighbors, yellow = 32 neighbors, orange = 64 neighbors; agents learn optimally; RC3a.
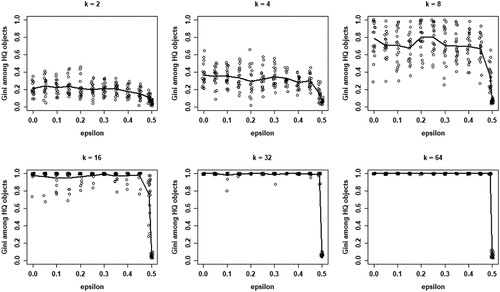
Figure C.6.3. Boxplots of the distributions of Gini coefficients of the distribution of agent choices among high quality objects; Gini coefficients of 100 runs at convergence, per value of k; agents learn optimally; RC3a.

Figure C.6.4. Boxplots of total search costs until convergence (based on EquationEq. (5)(5)
(5) summed over all rounds n per run) for all values of k; y-axis running from 0 to 350 for k equal to 2, 4, and 8, from 0 to 8000 for k equal to 16 and 32, and from 0 to 20,000 for k equal to 64; agents learn optimally; RC3a.
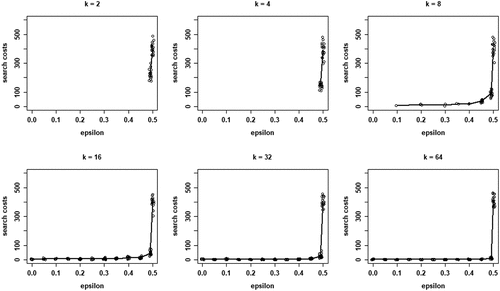
Figure C.7.1. Proportions of agents staying with their object of choice given that it failed () on the y-axis by round on the x-axis; gray lines depict 100 runs for each neighborhood size k, black lines plot means per round; agents learn optimally; RC3b.

Figure C.7.2. Mean proportions of agents staying with their object of choice given that it failed () on the y-axis by round on the x-axis; 100 runs for each neighborhood size k; black = 2 neighbors, blue = 4 neighbors, red = 8 neighbors, green = 16 neighbors, yellow = 32 neighbors, orange = 64 neighbors; agents learn optimally; RC3b.
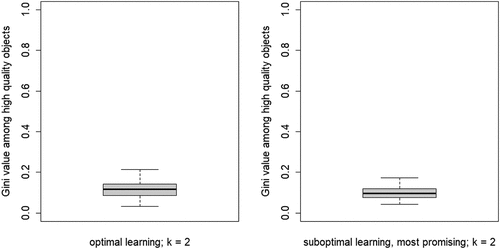
Figure C.7.3. Boxplots of the distributions of Gini coefficients of the distribution of agent choices among high quality objects; Gini coefficients of 100 runs at convergence, per value of k; agents learn optimally; RC3b.

Figure C.7.4. Boxplots of total search costs until convergence (based on EquationEq. (5)(5)
(5) summed over all rounds n per run) for all values of k; y-axis running from 0 to 350 for k equal to 2, 4, and 8, from 0 to 8000 for k equal to 16 and 32, and from 0 to 20,000 for k equal to 64; agents learn optimally; RC3b.
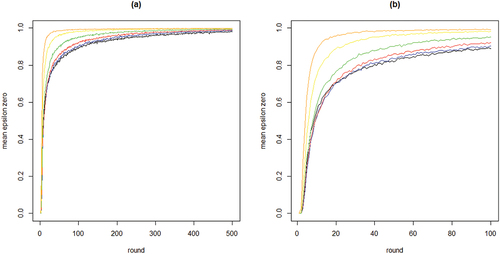