Figures & data
Figure 1. Non-linear social change regarding mask wearing in Finland during the COVID-19 pandemic. In particular, the rapid shift from baseline values to greater uptakes, followed by oscillations, would not be described by linear or even piecewise linear regression. (Data source: Fan et al., Citation2020).
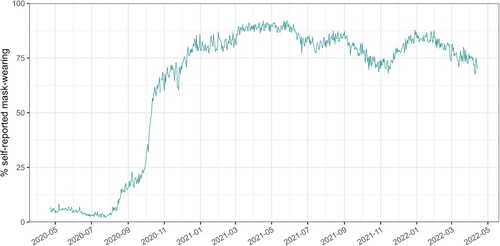
Figure 2. A conceptual basis to draw interpretations and stimulate multidisciplinary modelling. Configurations of variables (x1, x2 …) forming the system define its state space. In complex systems, this is often not flat (where every value is equally probable) but displays several equilibria, which depend on the value of extra parameters. Altogether, variables and parameters shape the state space V(x,p), which contains all equilibria. Parameters (p) here quantify the inter-relationships between variables, i.e., ‘how much’ a variable influences another. Stable equilibria – also known as attractors – are shown as valleys. To investigate how a system goes from one equilibrium to another, it is often sufficient to consider low-dimensional combinations of its variables (Kuehn et al., Citation2021) (extracted e.g., via principal component analysis, in which case the x1 and x2 would represent the two largest principal components, while the blue ridge identifies the main direction of change).
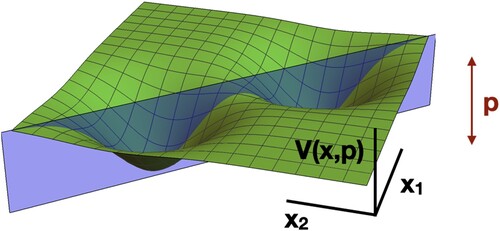
Figure 3. An attractor landscape with three different exemplifying states. Imagine the ball resembling the state of a person – e.g., location of ball in Panel A: ‘always takes precautions to mitigate contagion’, Panel B: ‘arbitrarily takes or does not take precautions’, and Panel C: ‘never takes precautions’. The two valleys represent stable (as defined by the local curvature below the ball) attractor states with different depths. Hence, the system is more prone to perturbations when in the rightmost attractor (Panel A) and more stable when in the leftmost attractor (Panel C). The transition from one attractor to the other happens when the system crosses the unstable ‘tipping point’ in the middle (Panel B). Arrows in Panels A and C represent the amount of variability we are likely to observe due to random perturbations on the system, a proxy for its stability. Lower stability translates into likely higher variability in the system’s state. Panel B also demonstrates that the landscape itself is not static but can change in the course of time (dashed line indicates the preceding landscape of panel A).

Figure 4. Two ways a system suddenly changes its state (i.e., two main types of critical transitions). B-tipping route (left): Due to an intervention or another learning or development process, the landscape itself changes, and when the state becomes unstable, the system ‘tips’ to the other attractor. N-tipping route (right): some exogenous perturbation, be it an intervention or a random event, jolts the ball over the threshold ‘hill’, where it stabilises in the new attractor.

Figure 5. Demonstration of a society passing through several behavioural attractors during the COVID-19 pandemic. The underlying data are eight behavioural indicators collected daily in Finland (See text and supplementary website at https://heinonmatti.github.io/attractor-manuscript/ for details). Panel A: Reconstructing behavioural phases according to complex network approaches. Colours represent attractors for each day in the horizontal axis, with lighter colours indicating less precautions on average, and vice versa. The radius parameter defines a ‘detection threshold’ for the reconstruction of networks among the eight indicators; e.g., only the two most obvious attractors are observable on high radius values such as 0.25 (yellow area for lifting of restrictions despite community transmission of the Delta variant, and purple area for large-scale restrictions due to hospitalisations and deaths from both Delta and Omicron BA.1). Panel B: Raincloud plot of variables in the attractors, showing which values make up each regime at a representative radius (0.17), marked with a dashed line in Panel A. We can observe e.g., that each precaution is generally at its lowest during the yellow period, and highest in the purple period. As another example, we can also observe that people changed their shopping behaviour around the end of November, when the yellow regime ended. Panel C: Attractors reconstructed by Principal Component Analysis, as movement in the space of two main principal components (linear combinations of variables). The underlying conceptual model would correspond to , but with four valleys instead of two. Colours to signify attractor types are imported from Panel B, at their corresponding temporal positions in Panel A, to illustrate convergence of the methods. The first principal component captures 68.4% of variance, and the second 20.3%.
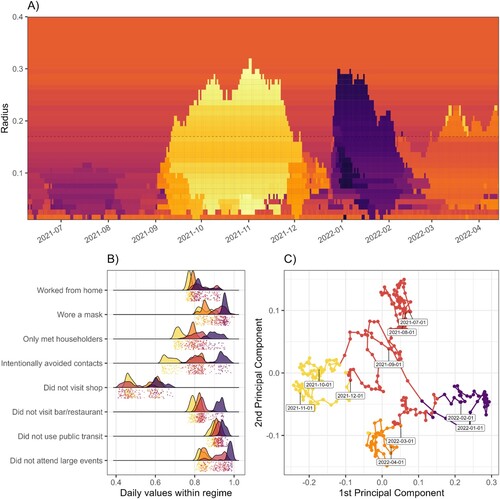
Table 1. A selection of features of complex systems, with examples to highlight relevance to behaviour change science. See also ‘The Visual Representation of Complexity’ (2018) and in Heino et al. (Citation2021).
Data availability statement
Data is available on the GitHub repository online: https://github.com/heinonmatti/attractor-manuscript.