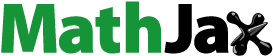
ABSTRACT
Tree clearing in Australia has resulted in an immense loss of forest cover, attributed to agricultural industry growth, particularly cattle rearing, as well as an increase in bushfire prevalence and severity due to climate-change effects. The loss of native forests results in increased net emissions of carbon dioxide into the atmosphere, thus enhancing the greenhouse effect of global warming. Additionally, deforestation jeopardises biodiversity conservation and the complex ecological balance of native wildlife with its surrounding ecosystems. The use of uncrewed autonomous vehicles (UAVs) to deposit seed aggregates has been trialled globally as a means for regenerating tree cover in remote areas. Such use is purported to obtain high seed-deposition rates, impressive germination rates of seeds and decreased labour costs; moreover, UAVs equipped with appropriate sensors can be beneficial in the post-planting analysis of seed success, from the early germination stages to canopy development. Once site data are collected, artificial intelligence, particularly machine-learning algorithms, can be used to process captured images through the stages of data preparation, feature extraction, model training and predictions. The segmentation techniques commonly involve implementation of either watershed-related algorithms or convolutional neural networks. The use of machine learning in characterising tree parameters has been found to improve speed, accuracy and reliability compared with traditional imaging processing techniques. This review reports on the use of UAVs in forestry, particularly reforestation in remote landscapes in Australia. It summarises the use of UAV technology in the deployment of seeds through to the monitoring of reforestation sites and explores trends in these. It discusses the benefits and challenges of the UAV-assisted monitoring of reforestation sites and future trends in this area.
Introduction
The United Nations estimates that the rate of global deforestation was over 10 million ha between 2015 and 2020 (FAO Citation2020). Such forest loss calls for rapid reforestation efforts to re-establish complex biodiversity in areas devastated by factors such as wildfire and agriculture and in mine reclamation projects (Thackway and Lesslie Citation2008). As well as initiating native biodiversity recovery and subsequent wildlife return, reforestation is a major strategy for mitigating climate change because forests act as carbon sinks, absorbing approximately 7.6 billion tonnes of net carbon dioxide (CO2) per year (IPCC Citation2019; Harris et al. Citation2021). The necessity to replenish forest ecosystems is reflected in the United Nations Sustainable Development Goal 15 – ‘Life on land’ (United Nations Department of Global Communications Citationn.d.). The ecological importance of forests is complementary to their social significance for local communities through the provision of cultural, recreational and spiritual practices (Farber et al. Citation2002; Fisher et al. Citation2009; FAO Citation2020). Replanting trees assists with reducing desertification, soil erosion and flooding; it can also help reduce the potential for zoonotic disease outbreaks because the associated increased species diversity reduces the likelihood of rapid disease spread in continuous host populations (Mohan, Richardson et al. Citation2021).
Despite its relatively small population density, Australia is responsible for immense deforestation since European settlement, with approximately 50% of all Australian forest cover having been cleared or degraded (Thackway and Lesslie Citation2008; DAFF Citation2023). Major tree clearing has occurred due to agricultural expansion, particularly for beef cattle production, and bushfire severity and frequency are increasing because of climate change (Nature Climate Change Citation2020; DAFF Citation2023). However, reforestation efforts are underway, as indicated by an increase in Australia’s forest area of 0.75 million ha between 2016 and 2021 (DAFF Citation2023).
The use of uncrewed automated vehicles (UAVs) for the direct seeding of reforestation sites represents a faster and less labour-intensive alternative to conventional seedling planting (Mohan, Richardson et al. Citation2021). Additionally, UAVs fitted with appropriate sensors can perform site assessments prior to planting and monitor tree growth. Remote sensing of reforestation using UAV technologies is still being developed for widespread use, from small-scale farms to larger reforestation sites. UAVs coupled with lidar sensors, optical cameras or hyperspectral cameras have been used to monitor reforestation success by capturing images for later evaluation (Dupuis et al. Citation2020). However, challenges exist in data analysis, mainly in gaining access to suitable image reference examples for training the models, developing appropriate workflows with optimised classification models, and determining optimal predictor parameters and/or model parameterisation (Goodbody et al. Citation2018; Santos et al. Citation2019). Semi-autonomous software platforms such as CVAT and QGIS can be used to tackle issues of access to fully representative training datasets and assist in simplifying the image-processing process. Reforestation success may require more indicators than merely tree characteristics, such as wildlife presence, water and soil quality, weed species abundance and carbon sequestration (Le et al. Citation2012).
Conventional reforestation methods typically involve seedling development in nurseries coupled with labour-intensive hand sowing or costly mechanised planting (Elliot Citation2016; Aghai and Manteuffel-Ross Citation2020). These techniques are only viable in large-scale nursery programs and where sites are easily accessible by road. Further challenges associated with hand or tractor sowing of pre-established seedlings include safety concerns on difficult-to-access terrain and the costs incurred in cultivating seedlings in nurseries (Masarei et al. Citation2019). An alternative to reforestation using seedlings involves direct-seeding techniques, in which seeds are sowed directly into the soil to achieve germination and establishment (NRM Board Citation2011). For large-scale regeneration projects, direct-seeding approaches are favoured because they are 10–30 times less expensive than sowing nursery stock (Grossnickle and Ivetic Citation2017; Masarei et al. Citation2019). The application of seed coatings of aggregate mixes, depending on the tree species, can aid in predator deterrence and provide nutrient supplements for enhanced growth and soil-benefiting factors such as hydrogels for water retention (Porter Citation2013; Pedrini et al. Citation2017). It is imperative that seed coatings are fully biodegradable and the materials used do not have a deleterious effect on soil quality or agroecosystem processes (Pedrini et al. Citation2017). Much of the existing research on seed coatings is kept confidential by private corporations or is not instigated by people accustomed to rigorous scientific research methods. Thus, although seed-coating technologies are used globally, there is limited published research on the optimisation of seed-coating materials and design. Direct seeding is currently implemented by sowing by hand or from tractors or conventional aircraft. Difficult-to-access sites necessitate the use of conventional aircraft, most commonly helicopters, over other direct-seeding techniques. However, seed mixes tend to segregate when agitated by the vibrations associated with helicopter flight (Pedrini et al. Citation2019). UAVs present a less-expensive alternative for depositing seed mixes at reforestation sites at remote locations, and they can be piloted with minimal training (Elliot Citation2016). UAVs can fly close to or beneath vegetation canopies, where conventional aircraft cannot. Further, UAVs with appropriate cameras or laser scanners can perform site assessment and monitoring quickly and cost-effectively and with more detail than conventional satellite imagery (Elliot Citation2016). UAV images can be analysed by eye or using image-detection and classification algorithms to detect the development of newly planted seedlings, which require high-resolution imagery not achievable by satellite or crewed aircraft imagery (Elliot Citation2016). The automation of reforestation tasks such as site surveying, deposition of seed vessels and site maintenance using nascent UAV technology is being explored. For instance, pivotal projects to replenish mangroves in the United Arab Emirates and Myanmar using UAVs have been launched with the aim of cultivating marine life and biodiversity, while mitigating CO2 emissions and purifying the water (Clarke Citation2020; Gray Citation2023). Other UAV-assisted regeneration efforts include the use of large UAVs to deposit seed pods in Mt Annan’s Australian Botanic Garden to fight invasive African olive trees (Murphy Citation2022); AirSeed’s efforts to regenerate Australian biodiversity to support koala survival through UAV seeding (Koala Forever Citation2018); the replenishment of remote tropical forests and eradication of noxious weeds in Thailand; and aerial seed-bombing of barren sites near Gauribidanur, India (Das Citation2023; Gray Citation2023). Due to the novelty of the technologies, these projects serve as pilot programs that need careful evaluation to ensure that UAV technologies can safely and efficiently accelerate reforestation efforts.
Current trends in UAV-assisted reforestation efforts
Site assessment and seed monitoring
Assessing and mapping the fundamental characteristics of reforestation sites is essential before planting. Site assessments must provide in-depth details on current land use, climate, topography, vegetation, soil quality and hydrology (Cappiella and Wright Citation2006). Traditionally, mapping and site assessment of this type would be performed by specialists on the ground, coupled with satellite imaging. UAVs used for pre-planting land assessment can also be used later for post-planting monitoring to assess seed germination and establishment.
Airborne laser systems comprising expensive lidar sensors, coupled with global positioning systems (GPS) and inertial navigation systems, can enable production of spatial terrain data such as digital elevation model (DEM) surfaces. Although these conventional technologies are suitable for ancillary data of expansive areas, UAVs with ‘structure from motion’ (SfM) software and mounted with consumer-grade red, green, blue (RGB) cameras can capture more specific areas at significantly higher resolutions (up to a millimetre of accuracy in spatial resolution) (Carrera-Hernández et al. Citation2020; Winsen and Hamilton Citation2023). UAVs with passive camera imagery produce high-quality topography and orthophotos of site areas, with quality comparable to expensive lidar point clouds in contexts where sufficient bare soil is visible from above.
UAV-SfM technology has been trialled successfully in reforestation post-planting applications. For example, individual tree detection has been achieved using UAV-SfM technology to determine forest attributes such as tree height, crown width, above-ground biomass and forest homogeneity, and diameter at breast height and wood quality have been derived indirectly from UAV-SfM imagery (Wan-Mohd-Jaafar et al. Citation2017; Jaafer et al. Citation2018; Mohan et al. Citation2019; Mohan, Leite et al. Citation2021; Corte et al. Citation2020). Workflow protocols for beginners intending to use UAVs have been developed (Nasiri et al. Citation2021; Kümmerer et al. Citation2023), particularly in the context of canopy height characterisation. Generating point clouds from UAV data involves surface matching and triangulation techniques to match different photo perspectives. DEMs, digital surface models (DSMs) and digital terrain models (DTMs) can be generated using accessible software platforms such as Agisoft Metashape (Agisoft Citation2023).
UAVs fitted with lidar have been trialled for characterising forest structure through canopy structure analysis of native eucalypts in Australia (Winsen and Hamilton Citation2023); individual tree detection of Dipterocarpus forests in Malaysia (Jaafer et al. Citation2018); and Eucalyptus benthamii Maiden & Cambage plantations in southern Brazil (Wan-Mohd-Jaafar et al. Citation2017; Mohan et al. Citation2019; Mohan, Richardson et al. Citation2021; Corte et al. Citation2020). The technique of hyperspectral imagery uses a large number of narrow wavebands to obtain information on each pixel within an image, revealing early symptoms of diseases and water stress as well as information on soil quality and plant species (Strecha et al. Citation2012).
UAVs mounted with hyperspatial cameras have been used to develop species-specific vegetation maps in remote shrub swamp areas in southeastern Australia and to discriminate between grape varieties in the Barossa Valley in South Australia (Lacar et al. Citation2001; Strecha et al. Citation2012). However, inaccuracies arise in imaging spectroscopy because the unique spectral signature of each tree species can be affected by age, health, slope, attitude, phenology and time of day (Woodget et al. Citation2015). Further, difficulties have been identified in autonomously characterising forestry sites with dense, spatially diverse and multi-variety species, which tend to necessitate very high-resolution datasets (Strecha et al. Citation2012), as well as in detecting seedling success, particularly where there is surrounding vegetation and on complex soil surfaces. Combating these challenges involves converging multiple image datasets such as RGB and NIR orthomosaics ().
Figure 1. Specific species mapping of Australian shrub swamp communities. True colour orthomosaic (left); near-infrared false colour composite (Band 1: Green, Band 2: Blue and Band 3: Near Infrared) (middle); subset of total swamp with classified Gleichenia microphylla overlaid on true colour composite (right) (Strecha et al. Citation2012)

Other uses of post-planting imaging include the detection of native wildlife, such as the application of thermal imaging sensors to detect southern elephant seals (Mirounga leonine) in maritime Antarctica (Hyun et al. Citation2020) and koalas (Phascolarctos cinereus) in New South Wales, Australia (Witt et al. Citation2020). Once images and point clouds are obtained from cameras, lasers or other sensor mechanisms, data analysis can be performed by eye or more commonly by computer algorithms.
A major limitation in UAV image-capturing technology is that ideal light conditions define the time available for missions, and variable illumination is a source of inconsistency pertaining to shadowing and low-contrast effects (D’Oleire-Oltmanns et al. Citation2012). Calibration reflectance panels have been used to reduce such effects (Hakala et al. Citation2018). Additionally, the careful optimisation of image quality and computational time with parameters such as flight height and camera resolution is required (D’Oleire-Oltmanns et al. Citation2012; Javernick et al. Citation2014; Ryan et al. Citation2015), which can be done with the assistance of software packages such as DJI’s flight planning software. Further, image-processing techniques in surveying applications are still relatively novel and thus are time-intensive and require specialised knowledge (Castillo et al. Citation2012; D’Oleire-Oltmanns et al. Citation2012). Relative positional accuracy errors may also arise, depending on the GPS method used and the time lag between camera triggering and actual image acquisition (Ryan et al. Citation2015). Relative positional accuracy errors can be reduced using surveyed ground control points.
Seedpod technologies (coatings)
Although seedpod coatings are not well represented in wildland restoration literature, the general characteristics are widely known from agricultural studies (Gornish et al. Citation2019). They involve casing multiple seeds (of the same or differing species) with fully biodegradable materials to improve the likelihood of survival by protecting seeds from predation and temperature extremes, providing vital nutrients, improving seed distribution, protecting seeds from impact, optimising pH levels and enhancing water retention (Elliot Citation2016; Pedrini et al. Citation2017; Gornish et al. Citation2019; Aghai and Manteuffel-Ross Citation2020; Dingley et al. Citation2024). Different companies use different seed-coating materials, depending on the species being planted and the ecological conditions at the site. The Australian company AgriCote coats tropical grass and legume seeds with a fungicide treatment (apron), an insecticide treatment (guacho), fertiliser for nutrients, growth promotants, rhizobia, a bonding polymer, and lime to improve plant establishment (Heritage Seeds Citation2018). Turner et al. (Citation2006), applying the multivariate analysis of variance (MANOVA), found that polymer seed coatings increased emergence rates by 17–55% on average for various native species in the Western Australian botanical province (Turner et al. Citation2006). Research into coatings for native bluebunch wheatgrass seeds (Pseudoroegneria spicata (Pursh)Á.Löve) found that a surfactant seed coating was effective in restoring soil hydrologic function and improving seedling emergence and development (Madsen et al. Citation2012). This coating involved a barrier treatment, a powder filler (65% crushed limestone and 35% clay-based montmorillonite) and a surfactant (Madsen et al. Citation2012). As seed-coating methodologies are predominantly developed by private commercial companies, there is limited information on the specific process parameters used.
The coating of seeds into seed bombs or pellets enables the standardisation of weight and size, which is required for accurate deployment by UAV. Despite its benefits, the aggregation of seeds into seed bombs incurs extra costs and, if not selected with research and care, may hinder the germination of some species.
Seed depositing and deployment
Traditional planting methods can typically result in the planting of 500–1500 seedlings day−1 by an experienced two-person crew for hand-planting and up to 4000–6000 seedlings day−1 by a three-person crew using a mechanical planter and tractor (Stelzer Citation2019). The use of UAVs to deposit seed pellets can improve speed, scale, precision, cost and safety compared with traditional seed sowing by manual labour or ground machinery, particularly in remote areas. A single UAV developed by the Australian company Lord of the Trees can propel up to 160 000 seedpods day−1 (Lord of the Trees Citation2022).
UAV seed-depository systems may involve a simplistic gravity-drop distributor mechanism, an airflow distributor, a centrifugal distributor or a pneumatic distributor (Lysych et al. Citation2021). The choice of distributor usually depends on the site characteristics and the budget for the project, with pneumatic mechanisms being the most common choice for seed sowing. The size and technological abilities of UAVs vary depending on the financial investment and legal constraints in a given jurisdiction (CASA Citation2020).
Recent UAV enterprises
Numerous UAV enterprises have spawned across the globe with the collective goal of reforesting difficult-to-access sites through aerial seeding. summarises selected enterprises and their related UAV characteristics such as seed deployment rate, flight time, land coverage rate, UAV technology, payload capacity and cost analysis. In some circumstances, information in these categories could not be determined due to a lack of transparency, likely because of confidentiality concerns within enterprises. The most impressive seed deployment rate of 120 seedpods minute−1 was reported by Dendra Systems, AirSeed and Lord of the Trees. Across companies, flight times ranged from 8 minutes to 2 hours, depending on payload weight and battery capacity; UAVs with longer flight times incurred higher initial costs. Land coverage of UAVs varied from 40 to 80 ha day−1, although Dronecoria reported a rate of 10 ha hour−1. The specific sensor technologies mounted on the UAVs are usually not openly disclosed, but broad descriptions indicate that most companies are employing pneumatic seed-deposition mechanisms, automated aerial seeding, GPS geotagging and pre/post plant monitoring. Dronecoria implements a simplistic and affordable three-dimensional (3D) printable, open-source design with a relatively small payload of 8 kg, compared with Droneseed’s 26 kg payload capacity. CO2 Revolution, Dronecoria, Droneseed and Seedcopter all claim to be 10 times more cost-effective than traditional planting methods (CO2 Revolution Citation2023; Dronecoria Citation2023; DroneSeed Citation2023; Seedcopter Citation2023), with AirSeed claiming to be 25 times faster than traditional planting methods (AirSeed Citation2023).
Table 1. Summary of uncrewed automated vehicle (UAV) parameters, as reported by enterprises
However, these time- and cost-reduction assertions are not validated with numerical data. Trials have been conducted by AirSeed and CO2 Revolution to show 80% germination success and 75% seed growth success for Lord of the Trees. A more accurate indication of seed success rate would be the establishment statistic because achieving establishment is more difficult than germination.
Recent UAV monitoring applications
provides examples of UAV forestry mission parameters – camera model, UAV model and forestry application. UAVs can monitor individual mature trees using consumer-grade RGB cameras of approximately 20 MP to capture imagery (Gómez et al. Citation2020). Such readily available cameras are cost-effective and relatively lightweight. Flight paths need to be designed to incorporate an overlap between images of 60–80% (Dell et al. Citation2019; Biggs Citation2020) and up to 95%, depending on plant size and the density of the point cloud required (Zhou and Zhang Citation2020). Autonomous flight path design is feasible, depending on the intelligence and autonomy of the UAV. However, predefined UAV missions may be implemented as an alternative to autonomous path design, depending on the application. Using a predefined UAV mission reduces the computational power required on board the UAV but jeopardises the ability to avoid obstacles or react in real time. The development of airspace modelling and path planning optimisation techniques has evolved from more traditional algorithms such as geometrical modelling and topological graph techniques to nascent methods using convolutional neural networks (CNNs) and bio-intelligence algorithms (Han et al. Citation2022).
Table 2. Uncrewed aviation vehicle (UAV) forestry mission parameters
A flight height of approximately 30 m was determined to be optimal in the context of monitoring individual species below the canopy in an open rainforest setting (Gómez et al. Citation2020), but heights of 100–250 m have been used for larger areas (Michez et al. Citation2016; Han et al. Citation2022). The mission planning phase should maximise the light for ideal camera results by considering the time of flight and using a wide aperture and fixed focal length lens to allow fast shutter speed (not exceeding 400 ISO). Changing atmospheric conditions must also be considered, such as illumination from moving clouds and the wind velocity, particularly in creating crown movement. UAV technological parameters such as battery capacity, emergency landing locations and GPS accuracy need to be considered before a mission, subject to regulatory conditions imposed by (in Australia) the Civil Aviation Safety Authority (CASA). CASA defines allowable flight conditions such as operator accreditation, line-of-sight requirements and flying-near-people restrictions (Government of Australia Citation2024).
Software for automated monitoring
After data acquisition through imaging sensors, processing software is required for data analysis (Dainelli et al. Citation2021). Data quality is governed by the types of sensors employed on the UAV (e.g. RGB, lidar, multispectral, hyperspectral, thermal), which, in turn, defines the overall accuracy of the models. A systematic review of forest characterisation software revealed that many groups have already taken up machine-learning algorithms for automatic tree detection and classification (Dainelli et al. Citation2021). The segmentation techniques employed by these workflows are based either on the watershed algorithm (Goodbody et al. Citation2018; Hentz et al. Citation2018; Iizuka et al. Citation2018), the inverse watershed algorithm (Abdollahnejad et al. Citation2018; Balková et al. Citation2020), the marker-controlled watershed algorithm (Huang et al. Citation2018; Brieger et al. Citation2019), or CNNs based on existing or novel architectures (Santos et al. Citation2019; Yurtseven et al. Citation2019). Using machine learning to characterise forest spectral, spatial and structural information has been found to enhance speed, accuracy and reliability compared with traditional raster image and point cloud segmentation. The application of watershed algorithms has shown high accuracy (86–95%) in tree classification imaging (Goodbody et al. Citation2018). The application of CNN algorithms in forest characterisation imaging has also yielded high accuracies (92%), with a processing time of less than 30 ms (Santos et al. Citation2019). However, the accuracy of tree detection and segmentation is dependent on the complexity of the site, the size of the plants being imaged, and the comprehensiveness of the training datasets applied.
Widely used software tools that use these machine-learning algorithms include eCognition, Matlab, R and Python (Dainelli et al. Citation2021). Although eCognition and Matlab are trusted software packages for geospatial applications, their cost can be a deterrence to novel UAV start-ups. Alternatively, R and Python software packages are entirely open-source platforms that promote public participation and the sharing of algorithm structures. Within the CNN-based methods exist three promising open-source object detection methods, namely Faster-RCNN, YOLOv8 and RetinaNet (Santos et al. Citation2019; Casas et al. Citation2023). The general properties of these variants are summarised in . The average precision and time taken to execute deep-learning methods on images are taken from research involving five cross-validation rounds of Brazilian nut tree (Dipteryx alata Vogel) detection (Santos et al. Citation2019). YOLOv3–8 and RetinaNet were significantly faster than the Faster-RCNN variation because of their regression-based object detection. All three CNN variants obtained adequate accuracies (>82%) from processing images obtained by UAVs mounted with RGB camera technology.
Table 3. Convolutional neural networks (CNN) object detection methods
Evaluation of benefits and challenges
Research into, and the use of, UAVs for aerial reforestation is gaining momentum due to the reduced cost and increased speed for geological surveying, seed deposition and post-assessment data collection, especially in remote and complex terrains. Although autonomous UAVs offer high-resolution data collection, challenges such as the high cost of batteries, sensors and licencing hinder adoption, particularly for newer businesses (Buters et al. Citation2019a, Citation2019b; Mohan, Richardson et al. Citation2021).
Making UAV technologies more accessible and applicable to reforestation requires more transparency in technology and data-processing schemes. Companies should promote knowledge-sharing for technology improvement, within the bounds of remaining commercially viable. Open-source machine-learning software and collaborative cloud environments facilitate data processing and knowledge exchange and also assist in overcoming challenges related to data processing such as its time-consuming nature, the expertise required and the identification of optimal data-collecting conditions. Data processing can be complex because it needs to consider differing seasonal characteristics of trees, differences in image height and lighting, and the most suitable object detection method.
Low survival rates of aerially deposited seeds are slowly being overcome through research on seed coatings/pellets. Such lower survival rates necessitate higher seed volumes as an intermediary solution, which in turn requires sustainable seed sources (Velasquez-Camacho et al. Citation2021). Further, the uneven distribution that can occur from UAV seed deposition often creates undesirable tree clusters, increasing competition for limited nutrients. This can be ameliorated by improving seed-firing accuracy through development of UAV seed-deposition mechanisms. UAVs depositing the seeds are at the mercy of weather conditions: high winds and rainfall and low temperatures can greatly reduce their operational efficiency and even cause irreversible damage. As a result, UAVs are not operational on all days, with some seasons posing greater limitations than others.
Additionally, the rules and regulations of a given jurisdiction may dictate the allowable weight of UAVs, the distances they can travel and their flight times. Certain exemptions may be made, but such regulations still form concrete constraints on UAV usage. Moreover, wildlife safety in an area needs to be considered because the use of UAVs can incite unusual behaviour among animals, such as by interrupting bird nesting and feeding. Collisions between UAVs pose a fire risk, which can cause significant destruction to sites (Broek van den Citation2020).
Challenges posed by low seed survival rates and uneven seed distribution can be addressed through research on seed coatings and deposition mechanisms. Wildlife safety concerns and the risk of fire from UAV collisions also require mitigation measures.
Future trends
With increased transparency by UAV companies and collaboration in open-source workflows, seed depositing and site assessment UAV technologies will become more efficient and accessible. Given overall continued forest loss globally and the threat posed to forests by climate change and other stressors, urgent action is required. Rather than commercialising UAV technologies, local government incentives could promote improved research and transparency in the field and increase the speed of UAV technological development. The benefits would not only be financial – they may also include improved forest biodiversity conservation and increased carbon-capturing capacity.
With approximately 50% of Australia’s forest cover degraded or cleared, urgent reforestation efforts are imperative. UAVs offer a promising solution by enabling rapid, precise and cost-effective seed deposition compared with traditional methods. Enhanced with sensors like lidar and multispectral imaging, UAVs can facilitate high-quality data collection for effective site monitoring. The integration of machine-learning algorithms can enhance automation in tree detection and classification and thereby streamline reforestation processes for greater efficiency and effectiveness. This synergy of technologies underscores the potential for UAVs to play a significant role in addressing Australia’s reforestation challenges.
Acknowledgements
We acknowledge the open-access publishing facilitated by the RMIT University under the Taylor & Francis Group and the Council of Australian University of Librarians (CAUL) consortium. Ms Stamatopoulos, the first author, acknowledges the RMIT University – STEM Research Placement award, which enabled her to conduct a desktop study on the manuscript topic. Special acknowledgement is extended to the anonymous reviewers representing Australian Forestry, whose comments contributed greatly to the improvement of the manuscript.
Disclosure statement
No potential conflict of interest was reported by the author(s).
References
- Abdollahnejad A, Panagiotidis D, Surovỳ P. 2018. Estimation and extrapolation of tree parameters using spectral correlation between UAV and Pléiades data. Forests. 9(2):85. doi:10.3390/f9020085.
- Aghai M, Manteuffel-Ross T. 2020. Enhancing direct seeding efforts with unmanned aerial vehicle (UAV) “swarms” and seed technology. Tree Planters’ Notes. 63(2):32–48.
- Agisoft. 2023. Discover intelligent photogrammetry with Metashape. St Petersburg (Russia): Agisoft. https://www.agisoft.com/.
- AirSeed. 2023. Home. Sydney (Australia): AirSeed. https://airseedtech.com/.
- Balková M, Bajer A, Patočka Z, Mikita T. 2020. Visual exposure of rock outcrops in the context of a forest disease outbreak simulation based on a canopy height model and spectral information acquired by an unmanned aerial vehicle. ISPRS International Journal of Geo-Information. 9(5):325. doi:10.3390/ijgi9050325.
- Biggs HJ. 2020. 3 – Aquatic vegetation monitoring with UAS. In: Green D, editor. Unmanned aerial remote sensing: uAS for environmental applications. Milton (UK): Taylor & Francis Group; p. 35–53.
- Brieger F, Herzschuh U, Pestryakova LA, Bookhagen B, Zakharov ES, Kruse S. 2019. Advances in the derivation of Northeast Siberian forest metrics using high-resolution UAV-based photogrammetric point clouds. Remote Sensing. 11(12):1447. doi:10.3390/rs11121447.
- Broek van den S. 2020. The effect of unmanned aircraft systems on predatory bird species: a comparison between drone and other known wildlife disturbing agents. Almere: Aeres Hogeschool.
- Buters T, Belton D, Cross A. 2019a. Multi-sensor UAV tracking of individual seedlings and seedling communities at millimetre accuracy. Drones. 3(4):1–20. doi:10.3390/drones3040081.
- Buters T, Belton D, Cross A. 2019b. Seed and seedling detection using unmanned aerial vehicles and automated image classification in the monitoring of ecological recovery. Drones. 3(15):1–16. doi:10.3390/drones3030053.
- Cappiella K, Schueler T, Wright T. 2006. Urban watershed forestry manual. Part 2: Conserving and planting trees at development sites (NA-TP-01-06). Newtown Square (PA): U.S. Department of Agriculture, Forest Service, Northeastern Area State and Private Forestry.
- Carrera-Hernández JJ, Levresse G, Lacan P. 2020. Is UAV-SfM surveying ready to replace traditional surveying techniques? International Journal of Remote Sensing. 41(12):4820–4837. doi:10.1080/01431161.2020.1727049.
- CASA. 2020. Micro and excluded remotely piloted aircraft operations (CASR PART 101). Canberra (Australia): Civil Aviation Safety Authority, Australian Government.
- Casas GG, Ismail ZH, Limeira MMC, da Silva AAL, Leite HG. 2023. Automatic detection and counting of stacked eucalypt timber using the YOLOv8 model. Forests. 14(12):2369. doi:10.3390/f14122369.
- Castillo C, Pérez R, James MR, Quinton JN, Taguas EV, Gómez JA. 2012. Comparing the accuracy of several field methods for measuring gully erosion. Soil Science Society of America Journal. 76(4):1319–1332. doi:10.2136/sssaj2011.0390.
- Clarke K. 2020. Mangrove-planting drones set to swoop in to restore UAE coastline. The National UAE; [accessed 2023 Jan 13]. https://www.thenationalnews.com/uae/environment/mangrove-planting-drones-set-to-swoop-in-to-restore-uae-coastline-1.984279.
- CO2 Revolution. 2023. Home. Navarra (Spain): CO2 Revolution. https://co2revolution.es/.
- Corte APD, Rex FE, de Almeida DRA, Sanquetta CR, Silva CA, Moura MM, Wilkinson B, Zambrano AMA, da C NE, Veras HFP, et al. 2020. Measuring individual tree diameter and height using gatoreye high-density UAV-lidar in an integrated crop-livestock-forest system. Remote Sensing. 12(5): 863. doi:10.3390/rs12050863.
- D’Oleire-Oltmanns S, Marzolff I, Peter KD, Ries JB. 2012. Unmanned aerial vehicle (UAV) for monitoring soil erosion in Morocco. Remote Sensing. 4(11):3390–3416. doi:10.3390/rs4113390.
- DAFF. 2023. Australia’s State of Forests Report. Canberra (Australia): Department of Agriculture, Fisheries and Forestry.
- Dainelli R, Toscano P, Di Gennaro SF, Matese A. 2021. Recent advances in unmanned aerial vehicles forest remote sensing—a systematic review. Part II: Research applications. Forests. 12(4):397. doi:10.3390/f12040397.
- Das M. 2023. How IISc scientists are seed bombing a barren land in Karnataka using drones. Bengaluru (India): The News Minute. https://www.thenewsminute.com/article/how-iisc-scientists-are-seed-bombing-barren-land-karnataka-using-drones-64155.
- Dell M, Stone C, Osborn J, Glen M, McCoull C, Rimbawanto A, Tjahyono B, Mohammed C. 2019. Detection of necrotic foliage in a young Eucalyptus pellita plantation using unmanned aerial vehicle RGB photography – a demonstration of concept. Australian Forestry. 82(2):79–88. doi:10.1080/00049158.2019.1621588.
- Dingley C, Cass P, Adhikari B, Daver F. 2024. Application of superabsorbent natural polymers in agriculture. Polymers from Renewable Resources. doi:10.1177/20412479231226166.
- Dronecoria. 2023. Main. Pitres (Spain): Dronecoria. https://dronecoria.org/en/main/.
- DroneSeed. 2023. Rapid Reforestation. Seattle (WA): DroneSeed. https://droneseed.com/.
- Dupuis C, Lejeune P, Michez A, Fayolle A. 2020. How can remote sensing help monitor tropical moist forest degradation? – a systematic review. Remote Sensing. 12(7):1087. doi:10.3390/rs12071087.
- Elliot S. 2016. The potential for automating assisted natural regeneration of tropical forest ecosystems. Biotropica. 48(6):825–833. doi:10.1111/btp.12387.
- FAO. 2020. The state of the world’s forests. Rome (Italy): Food and Agriculture Organization of the United Nations and United Nations Environment Programme.
- Farber SC, Costanza R, Wilson MA. 2002. Economic and ecological concepts for valuing ecosystem services. Ecological Economics. 41(3):375–392. doi:10.1016/S0921-8009(02)00088-5.
- Fisher B, Turner RK, Morling P. 2009. Defining and classifying ecosystem services for decision making. Ecological Economics. 68(3):643–653. doi:10.1016/j.ecolecon.2008.09.014.
- Fraser BT, Congalton RG. 2021. Monitoring fine-scale forest health using unmanned aerial systems (UAS) multispectral models. Remote Sensing. 13(23):4873. doi:10.3390/rs13234873.
- Gómez C, Goodbody TRH, Coops NC, Álvarez-Taboada F, Sanz-Ablanedo E. 2020. 11 – Forest ecosystem monitoring using unmanned aerial systems. In: Green D, editor. Unmanned aerial remote sensing: uAS for environmental applications. Milton (UK): Taylor & Francis Group; p. 173–196.
- Goodbody TRH, Coops NC, Hermosilla T, Tompalski P, Crawford P. 2018. Assessing the status of forest regeneration using digital aerial photogrammetry and unmanned aerial systems. International Journal of Remote Sensing. 39(15–16):5246–5264. doi:10.1080/01431161.2017.1402387.
- Gornish E, Arnold H, Fehmi J. 2019. Review of seed pelletizing strategies for arid land restoration. Restoration Ecology. 27(6):1206–1211. doi:10.1111/rec.13045.
- Government of Australia. 2024. Drones. Canberra (Australia): Civil Aviation Safety Authority, Australian Government. [accessed 2024 Mar 12]. https://www.casa.gov.au/drones.
- Gray D. 2023. Thai team fights climate change with aerial reseeding. Tokyo (Japan): NIKKEI Asia. https://asia.nikkei.com/Life-Arts/Life/Thai-team-fights-climate-change-with-aerial-reseeding?page=2.
- Grossnickle S, Ivetic V. 2017. Direct seeding in reforestation – a field performance review. Reforesta. 12(4):94–142. doi:10.21750/REFOR.4.07.46.
- Hakala T, Markelin L, Honkavaara E, Scott B, Theocharous T, Nevalainen O, Näsi R, Suomalainen J, Viljanen N, Greenwell C. 2018. Direct reflectance measurements from drones: sensor absolute radiometric calibration and system tests for forest reflectance characterization. Sensors. 18(5):1417. doi:10.3390/s18051417.
- Han B, Qu T, Tong X, Jiang J, Zlatanova S, Wang H, Cheng C. 2022. Grid-optimized UAV indoor path planning algorithms in a complex environment. International Journal of Applied Earth Observations & Geoinformation. 111:1–15. doi:10.1016/j.jag.2022.102857.
- Harris NL, Gibbs DA, Baccini A, Birdsey RA, de Bruin S, Farina M, Fatoyinbo L, Hansen MC, Herold M, Houghton RA, et al. 2021. Global maps of twenty-first century forest carbon fluxes. Nature Climate Change. 11(3): 234–240. doi:10.1038/s41558-020-00976-6.
- Hentz ÂMK, Silva CA, Dalla Corte AP, Netto SP, Strager MP, Klauberg C. 2018. Estimating forest uniformity in Eucalyptus spp. And Pinus taeda L. stands using field measurements and structure from motion point clouds generated from unmanned aerial vehicle (UAV) data collection. Forest Systems. 27(2):e005. doi:10.5424/fs/2018272–11713.
- Heritage S. 2018. Tropical pasture guide. Rocklea (Australia): Heritage Seeds.
- Hu X, Li D. 2020. Research on a single-tree point cloud segmentation method based on UAV tilt photography and deep learning algorithm. IEEE Journal of Selected Topics in Applied Earth Observations and Remote Sensing. 13:4111–4120. doi:10.1109/JSTARS.2020.3008918.
- Huang H, Li X, Chen C. 2018. Individual tree crown detection and delineation from very-high-resolution UAV images based on bias field and marker-controlled watershed segmentation algorithms. IEEE Journal of Selected Topics in Applied Earth Observations and Remote Sensing. 11(7):2253–2262. doi:10.1109/JSTARS.2018.2830410.
- Hyun CU, Park M, Lee WY. 2020. Remotely piloted aircraft system (RPAS)-based wildlife detection: a review and case studies in maritime Antarctica. Animals. 10(12). doi:10.3390/ani10122387.
- Iizuka K, Yonehara T, Itoh M, Kosugi Y. 2018. Estimating tree height and diameter at breast height (DBH) from digital surface models and orthophotos obtained with an unmanned aerial system for a Japanese cypress (Chamaecyparis obtusa) forest. Remote Sensing. 10(1):13. doi:10.3390/rs10010013.
- [IPCC] Intergovernmental Panel on Climate Change. 2019. Climate Change and Land: an IPCC special report on climate change, desertification, land degradation, sustainable land management, food security, and greenhouse gas fluxes in terrestrial ecosystems.Cambridge (UK): Cambridge University Press. doi:10.1017/9781009157988.
- Jaafar WSWM, Woodhouse IH, Silva CA, Omar H, Maulud KNA, Hudak AT, Klauberg C, Cardil A, Mohan M. 2018. Improving individual tree crown delineation and attributes estimation of tropical forests using airborne LiDAR data. Forests. 9(12):759. doi:10.3390/f9120759.
- Javernick L, Brasington J, Caruso B. 2014. Modeling the topography of shallow braided rivers using Structure-from-Motion photogrammetry. Geomorphology. 213:166–182. doi:10.1016/j.geomorph.2014.01.006.
- Kümmerer R, Noack PO, Bauer B. 2023. Using high-resolution UAV imaging to measure canopy height of diverse cover crops and predict biomass. Remote Sensing. 15(6):1520. doi:10.3390/rs15061520.
- Lacar FM, Lewis MM, Grierson IT 2001. Use of hyperspectral imagery for mapping grape varieties in the Barossa Valley, South Australia. IEEE International Symposium on Geoscience and Remote Sensing (IGARSS). 6:2875–2877. 10.1109/IGARSS.2001.978191.
- Le HD, Smith C, Herbohn J, Harrison S. 2012. More than just trees: assessing reforestation success in tropical developing countries. Journal of Rural Studies. 28(1):5–19. doi:10.1016/j.jrurstud.2011.07.006.
- Lord of the Trees. 2022. New challenges an altered world. Sydney (Australia): Lord of the Trees. https://lordofthetrees.ai/.
- Lysych M, Bukhtoyarov L, Druchinin D. 2021. Design and research sowing devices for aerial sowing of forest seeds with UAVs. Inventions. 6(4):83. doi:10.3390/inventions6040083.
- Madsen MD, Kostka SJ, Inouye AL, Zvirzdin DL. 2012. Postfire restoration of soil hydrology and wildland vegetation using surfactant seed coating technology. Rangeland Ecology & Management. 65(3):253–259. doi:10.2111/REM-D-11-00083.1.
- Masarei M, Guzzomi AL, Merritt DJ, Erickson TE. 2019. Factoring restoration practitioner perceptions into future design of mechanical direct seeders for native seeds. Restoration Ecology. 27(6):1251–1262. doi:10.1111/rec.13001.
- Michez A, Piégay H, Lisein J, Claessens H, Lejeune P. 2016. Classification of riparian forest species and health condition using multi-temporal and hyperspatial imagery from unmanned aerial system. Environmental Monitoring and Assessment. 188(3):146–146. doi:10.1007/s10661-015-4996-2.
- Mohan M, de Mendonça BAF, Silva CA, Klauberg C, de Saboya Ribeiro as, de Araújo EJG, Monte MA, Cardil A. 2019. Optimizing individual tree detection accuracy and measuring forest uniformity in coconut (Cocos nucifera L.) plantations using airborne laser scanning. Ecological Modelling. 409:108736. doi:10.1016/j.ecolmodel.2019.108736.
- Mohan M, Leite RV, Broadbent EN, Jaafar WSWM, Srinivasan S, Bajaj S, Corte APD, Do Amaral CH, Gopan G, Saad SNM, et al. 2021. Individual tree detection using UAV-lidar and UAV-SfM data: a tutorial for beginners. Open Geosciences. 13(1): 1028–1039. doi:10.1515/geo-2020-0290.
- Mohan M, Richardson G, Gopan G, Aghai MM, Bajaj S, Galgamuwa GAP, Vastaranta M, Arachchige PSP, Amorós L, Corte APD, et al. 2021. UAV-supported forest regeneration: current trends, challenges and implications. Remote Sensing. 13(13):2596. doi:10.3390/rs13132596.
- Murphy S. 2022 June 19. Giant drone planting 40,000 tree pods a day in Australian Botanic Garden at Mt Annan. Australian Broadcast Network News. https://www.abc.net.au/news/2022-06-19/giant-drones-dropping-tree-seeds/101150496.
- Nasiri V, Darvishsefat AA, Arefi H, Pierrot-Deseilligny M, Namiranian M, Le Bris A. 2021. Unmanned aerial vehicles (UAV)-based canopy height modeling under leaf-on and leaf-off conditions for determining tree height and crown diameter (case study: Hyrcanian mixed forest). Canadian Journal of Forest Research. 51(7):962–971. doi:10.1139/cjfr-2020-0125.
- Nature Climate Change. 2020. In the line of fire. Nature Climate Change. 10(3):169. doi:10.1038/s41558-020-0720-5.
- NRM Board. 2011. Direct seeding. NRM Fact Sheet. 4(4):1.
- Pedrini S, Lewandrowski W, Stevens JC, Dixon KW, Pritchard H. 2019. Optimising seed processing techniques to improve germination and sowability of native grasses for ecological restoration. Plant Biology. 21(3):415–424. doi:10.1111/plb.12885.
- Pedrini S, Merritt DJ, Stevens J, Dixon K. 2017. Seed coating: science or marketing spin? Trends in Plant Science. 22(2):106–116. doi:10.1016/j.tplants.2016.11.002.
- Porter SS. 2013. Adaptive divergence in seed color camouflage in contrasting soil environments. The New Phytologist. 197(4):1311–1320. doi:10.1111/nph.12110.
- Ryan JC, Hubbard AL, Box JE, Todd J, Christoffersen P, Carr JR, Holt TO, Snooke N. 2015. UAV photogrammetry and structure from motion to assess calving dynamics at Store Glacier, a large outlet draining the Greenland ice sheet. The Cryosphere. 9(1):1–11. doi:10.5194/tc-9-1-2015.
- Santos AAD, Marcato J Jr,Araujo, MS, di Martini, DR, Tetila, EC, Siqueira, HL, Aoki C, Eltner A, Matsubara, ET, Pistori H, et al. 2019. Assessment of CNN-based methods for individual tree detection on images captured by RGB cameras attached to UAVS. Sensors. 19(16):3595. doi:10.3390/s19163595.
- Seedcopter. 2023. Reforestation made Scalable. Telangana (India): Seedcopter. http://seedcopter.com/.
- Stelzer H. 2019. How to plant forest tree seedlings. Columbia (MO): Extension, University of Missouri. https://extension.missouri.edu/publications/g5008.
- Strecha C, Fletcher A, Lechner A, Erskine P, Fua P. 2012. Developing species specific vegetation maps using multi-spectral hyperspatial imagery from unmanned aerial vehicles. ISPRS Annals of the Photogrammetry, Remote Sensing and Spatial Information Sciences. 1:311–316. doi:10.5194/isprsannals-I-3-311-2012.
- Thackway R, Lesslie R. 2008. Describing and mapping human-induced vegetation change in the Australian landscape. Environmental Management. 42(4):572–590. doi:10.1007/s00267-008-9131-5.
- Turner SR, Pearce B, Rokich DP, Dunn RR, Merritt DJ, Majer JD, Dixon KW. 2006. Influence of polymer seed coatings, soil raking, and time of sowing on seedling performance in post-mining restoration. Restoration Ecology. 14(2):267–277. doi:10.1111/j.1526-100X.2006.00129.x.
- United Nations Department of Global Communications. n.d. The 17 goals. New York: UN Department of Global Communications. https://sdgs.un.org/goals.
- Velasquez-Camacho L, Cardil A, Mohan M, Etxegarai M, Anzaldi G, de Miguel S. 2021. Remotely sensed tree characterization in urban areas: a review. Remote Sensing. 13(23):4889. doi:10.3390/rs13234889.
- Wan-Mohd-Jaafar WS, Woodhouse IH, Silva CA, Omar H, Hudak AT. 2017. Modelling individual tree aboveground biomass using discrete return lidar in lowland dipterocarp forest of Malaysia. Journal of Tropical Forest Science. 29(4):465–484. doi:10.26525/jtfs2017.29.4.465484.
- Winsen MHG, Hamilton G. 2023. A comparison of UAV-derived dense point clouds using LiDAR and NIR photogrammetry in an Australian eucalypt forest. Remote Sensing. 15(6):1694. doi:10.3390/rs15061694.
- Witt RR, Beranek CT, Howell LG, Ryan SA, Clulow J, Jordan NR, Denholm B, Roff A, Crowther MS. 2020. Real-time drone derived thermal imagery outperforms traditional survey methods for an arboreal forest mammal. Public Library of Science ONE. 15(11):e0242204. doi:10.1371/journal.pone.0242204.
- Woodget AS, Carbonneau PE, Visser F, Maddock IP. 2015. Quantifying submerged fluvial topography using hyperspatial resolution UAS imagery and structure from motion photogrammetry. Earth Surface Processes and Landforms. 40(1):47–64. doi:10.1002/esp.3613.
- World Wildlife Fund Australia. 2018. Koala forever. Sydney (Australia): World Wildlife Fund. https://wwf.org.au/what-we-do/help-regenerate-australia/koalas-forever/.
- Yurtseven H, Akgul M, Coban S, Gulci S. 2019. Determination and accuracy analysis of individual tree crown parameters using UAV based imagery and OBIA techniques. Measurement: Journal of the International Measurement Confederation. 145:651–664. doi:10.1016/j.measurement.2019.05.092.
- Zhou X, Zhang X. 2020. Individual tree parameters estimation for plantation forests based on UAV oblique photography. IEEE Access. 8:96184–96198. doi:10.1109/ACCESS.2020.2994911.