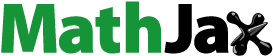
ABSTRACT
Diversifying wheat (Triticum aestivum L.)-based cropping systems is important for the sustainability of dryland agriculture. Research has focused on the agronomic benefits of increased crop diversity in semi-arid environments, but less is known about the impacts of increased crop diversity on the soil microbial community. This work compared soil health parameters between a continuous wheat crop sequence to a diverse sequence that included pea (Pisum sativum L.), proso millet (Panicum miliaceum L.), safflower (Carthamus tinctorius, L.), and spring wheat. Respiration was higher (p < .005) in the diverse sequence while activity of N-acetyl-β-d-glucosaminidase was lower (p < .05) with a mean rate of 26.3 and 16.3 mg ρ-nitrophenol kg−1 soil h−1 for the continuous wheat and diverse sequences, respectively. The mean net nitrogen mineralized during the growing season was 33.2 ± 2.5 kg ha−1 and was not different between treatments (p > .05). No difference was observed in bacterial alpha diversity, while fungal community diversity was 52% lower in the diverse rotation. The results of this work suggest that specific crops in a rotation may impact microbial processes related to nitrogen mineralization and that the soil fungal community may be more sensitive to changes in crop sequence than the soil bacterial community.
Introduction
Wheat is the primary grain crop in much of the U.S. northern Great Plains (USNGP) (Carr et al. Citation2020). Historic farming practices, where wheat-fallow was combined with tillage, have caused soil degradation and contributed to a 30–50% decline in organic C in dryland soils over the past 50–100 years in the USNGP (Sainju, Jabro, and Caesar-Tonthat Citation2010). In this region, a 12–20 month fallow period is commonly used to store soil moisture for the subsequent wheat crop, even though this practice is an inefficient water storage strategy (Carr et al. Citation2020). There has been increased interest in diversifying wheat-based cropping systems to improve soil quality and break weed and disease cycles and past research has evaluated the agronomic benefits of these practices (Kandel et al. Citation2019; Tiemann et al. Citation2015). In the USNGP, this diversification has been achieved primarily by incorporating pulse crops such as field peas, chickpeas, or lentils into rotation with wheat (Lupwayi and Kennedy Citation2007).
More recently warm season crops have gained attention in the USNGP as a potential replacement for fallow (Carr et al. Citation2020). Climate models indicate warmer temperatures at northern latitudes in the future (Whitlock et al. Citation2017), favoring expansion of warm season crops at upper latitudes. While not as common as cool season species, warm season species can have similar yields when grown for forage (Berti et al. Citation2015; Carr et al. Citation2020; Meccage et al. Citation2019). Research on warm season crops in the USNGP has focused on agronomic performance while largely ignoring the impact of these crops on soil microbial composition and function and other soil health parameters (Carr et al. Citation2020, Citation2021; Lenssen and Cash Citation2011).
While much of the focus on diversifying cropping systems is directed at agronomic benefits, increased crop diversity has the potential to benefit soil microbial communities and microbially mediated processes in the soil. Low crop diversity is generally considered detrimental to soil microorganisms and can result in a loss of microbial diversity and function (Figuerola et al. Citation2014; Frasier et al. Citation2016). Conversely, greater plant diversity has been linked to increased microbial biomass and diversity (Acosta-Martínez et al. Citation2011; Ishaq et al. Citation2017; Ouverson et al. Citation2021). Past research demonstrated that wheat grown in rotation with legumes has greater microbial abundance and activity than in wheat-fallow systems (Biederbeck, Zentner, and Campbell Citation2005; Lupwayi et al. Citation2004).
Microbial biomass is a key factor controlling organic nutrient cycling and functions as an early indicator of soil quality changes resulting from management and environmental stresses (Daryanto et al. Citation2018). But biomass is not necessarily an easily interpretable measure of soil health (Fierer, Wood, and Bueno de Mesquita Citation2021). More work is needed to better understand the effect of diversified cropping systems on specific microbial processes.
Nitrogen (N) mineralization is one microbially-mediated process that is impacted by increased cropping system diversity (Frasier et al. Citation2016). Nitrogen mineralization is the process whereby extracellular microbial enzymes depolymerize N-containing organic compounds and convert the N to inorganic forms that can be used by plants (Schimel and Bennett Citation2004). Two enzymes involved in biomass mineralization are N-acyl-β-d-glucosaminidase (NAGase, EC 3.2.1.30) and β-glucosidase. NAGase plays a role in N cycling by catalyzing the hydrolysis of N-acetyl-b-d-glucosamine and is involved in the degradation of chitin and peptidoglycan while β-glucosidase is involved in degradation of cellulose (Mori et al. Citation2021; Parham and Deng Citation2000). These enzymes have been proposed as indexes of carbon (C) and N mineralization (Acosta-Martínez, Pérez-Guzmán, and Johnson Citation2019; Ekenler and Tabatabai Citation2007).
Conventional fertilizer practices have reduced plant dependence on microbial processes mediating N mineralization and have effectively uncoupled plant productivity from microbial activity (Murphy et al. Citation2017). Studies have shown that monocropping decreased microbial C and N and reduced enzyme activities compared to rotations that contained perennials or included cover crops that enhanced N sequestration (Deng and Tabatabai Citation2000; Deng, Moore, and Tabatabai Citation2000; Frasier et al. Citation2016).
Much of the work related to N mineralization under different cropping systems has been done in the US Midwest and South (Acosta-Martínez et al. Citation2011; Deng and Tabatabai Citation2000; Deng, Moore, and Tabatabai Citation2000). The climatic conditions in these regions are very different from the USNGP, which is characterized as semi-arid with hot summers and cold winters (Carr et al. Citation2020). Temperature and moisture are important drivers of microbial processes such as N mineralization (Calderón et al. Citation2016; Stark and Firestone Citation1995; Vigil et al. Citation2002). These climatic conditions likely result in lower microbial activity in the USNGP compared to other more temperate regions.
As more growers in the USNGP diversify cropping systems and incorporate warm season crops to help break weed and disease cycles and enhance soil health and sustainability, research is needed to evaluate the effects of these practices on soil microbial processes. We hypothesized that increased crop diversity would increase bacterial and fungal community diversity and activity. The objectives of this work were to (i) determine the rates of net N mineralization under a continuous wheat crop sequence and a diverse wheat-based crop sequence that incorporated cool and warm season crops, (ii) Evaluate the microbial response to the crop sequence by measuring microbial biomass, community diversity, respiration, and activity of enzymes involved in soil C and N cycling.
Methods
Field experiments were conducted from fall 2018 through spring 2021 as part of a crop sequence study located at the Montana State University, Central Agricultural Research Center, Moccasin, MT, USA (47.059931, −109.950159). The soil type at this site is a Danvers-Judith clay loam, a complex of well-drained fine, smectitic, frigid Vertic Argiustolls. The crop sequences consisted of a continuous wheat system, with spring wheat followed by winter wheat (SW-WW) and a diverse 4-year rotation which had spring pea (SP), followed by proso millet (MIL), safflower (SAF), and spring wheat (SW). All crops were managed for grain production and fertility was managed the same across all crop phases except pea, with 56 kg ha−1 starter fertilizer (20-10-20-10) applied in furrow at planting followed by top dressing with 79 kg ha−1 urea (46-0-0) in 2018, 126 kg ha−1 slow-release urea (44-0-0) in 2019, and 90 kg ha−1 urea in 2020. No fertilizer was applied to the pea phase of the crop sequence. All crop phases were in place each year and plots were arranged in a Randomized Complete Block Design (RCBD) design with four replicates.
Soil sampling and respiration measurements were collected in the SW phase of the crop sequences each year. Spring wheat was planted 22 May Citation2018, 15 May Citation2019, and 18 May Citation2020 and harvested 22 Sept 2018, 7 Sept 2019, and 24 Aug 2020. All plots were managed using standard regional practices for fertility and weed management. Monthly total precipitation and mean air temperature are shown in Table S1.
Soil sampling was performed monthly throughout the growing season. Soil cores (0–15 cm) were collected with a 2.54-cm soil probe. Soils were air dried for enzyme analysis or dried at 55°C for 3 days for biomass determination. Subsamples of fresh soil were stored at −80°C for DNA sequencing. Dried soils were passed through a 2-mm sieve before analysis. NO3-N and NH4-N were extracted following the methods of Kolberg, Johnson, and Peterson (Citation1999) and Wienhold (Citation2007). Briefly, 4 g dry wt equivalent of soil was combined with 40 mL of 2 M KCl and incubated for 1 hour at room temperature shaking at 250 rpm. Samples were centrifuged at 3000 rpm for 10 min and the resulting supernatant was submitted to the MSU Environmental Analytical Lab for NO3-N and NH4-N analysis via Cd reduction on a Lachat QuickChem8500 (Hach, Loveland, CO). Samples were also submitted to Ward Laboratories (Kearney, NE) for pH (1:1 in H2O), organic matter (OM; loss on ignition, 2 h at 360°C), total C (combustion method), total N, phosphorus (P, NH4 acetate extraction), potassium (K, Mehlich III extraction), sulfur (sulfate-S, Mehlich III extraction), and cation exchange capacity (CEC) analysis.
Soil respiration
Soil respiration was measured with CFLUX-1 (PP Systems, Amesbury, MA) automated respiration chambers. The chambers were programmed to take hourly measurements. Respiration chambers were initially deployed on 1 Nov 2018 and remained in the field until nighttime temperatures were below the operational temperature limits of the instruments (−20°C). Chambers were redeployed in early spring once nighttime temperatures were above the operational temperature limits of the instruments. Chambers were removed for planting and then redeployed for the growing season. The chambers were removed for harvest and then deployed for the fall and remained in operation until average nighttime temperatures reached the lower operation limits of the instrument. Soil water content and temperature were recorded simultaneously with flux data using Stevens HydraProbes (Stevens Inc., Portland, Oregon, USA).
N mineralization
In situ N mineralization was performed as previously described (Kolberg, Johnson, and Peterson Citation1999; Wienhold Citation2007). Metal tubes measuring 4.76 cm in diameter were inserted 17 cm into the soil and removed with intact soil cores. Approximately 2 cm of soil was removed from the bottom of the core with a stainless-steel laboratory spatula and replaced with a nylon bag containing 10 g Lewatit NM-60 DI Resin ion exchange resin beads (Lanxess, Cologne, Germany). The nylon bags were secured with a spring clip and tubes were reinserted into the original holes. Six tubes were inserted into each plot and a soil core (0–15 cm) was collected with a 2.54-cm soil probe next to each tube to measure initial NO3-N and NH4-N concentrations. Each month from March-August, tubes was removed, and the resin was recovered for quantifying inorganic N. NO3-N and NH4-N were extracted following the methods of (Kolberg, Johnson, and Peterson Citation1999; Wienhold Citation2007). Briefly, 2 g wet weight resin beads were combined with 40 mL 2 M KCl and incubated for 1 hr at room temperature, shaking at 250 rpm. Samples were centrifuged at 3000 rpm for 10 min and the resulting supernatant was submitted to the MSU Environmental Analytical Lab for NO3-N and NH4-N analysis on a Lachat QuickChem8500 (Hach, Loveland, CO). Inorganic N was calculated on a kg ha−1 basis using the measured soil bulk density (1.7 g cm−3) and the soil column depth of 17 cm. Net N mineralized was calculated as the average of five cores per treatment using the combined amounts of NO3-N and NH4-N following the approach of Kolberg, Johnson, and Peterson (Citation1999) where:
Where Ns is soil inorganic N (kg ha−1) and Nr is resin inorganic N (kg ha−1). N fixation, deposition, immobilization, volatilization, and denitrification were assumed to be minimal. Previous work has shown that denitrification is minimal at < 60% water filled pore space and immobilization is reduced when residues remain on the surface instead of being incorporated (Ambus, Kure, and Jensen Citation2002; Aulakh et al. Citation1991).
A laboratory column assay was performed to assess N mineralization as previously described (Deng and Tabatabai Citation2000; Tabatabai, Ekenler, and Senwo Citation2010). A 20 g (oven dry basis) sample of field moist soil was combined with 40 g silica sand. Fifteen g of the soil-sand mixture was transferred to a leaching column (2.5 cm x 15 cm PVC tube) which contained glass wool to retain the soil-sand mixture. Three technical replicate columns were established with soil from each field plot. Columns were weighed and the soil was maintained at 50% field capacity by watering every two days to adjust them to their initial weights. Columns were loosely covered with aluminum foil to minimize evaporation. Columns were leached by adding 100 mL 5 mM CaCl2 and then placing them on a suction flask and applying vacuum to remove the leachate. The recovered leachate was adjusted to a final volume of 100 mL using DI water. Leaching was performed every 2 weeks for 14 weeks. Cumulative mineralized N was calculated as the sum of NO3-N and NH4-N recovered from each leaching event.
Enzyme activities
N-acetyl-β-d-glucosaminidase (NAGase) and β-glucosidase activities were measured following the methods of Tabatabai (Citation1994) and Acosta-Martínez and Tabatabai (Citation2000). All assays were performed with 1 g of air-dried soils that were passed through a 2-mm sieve. Assays were performed in 0.1 M tris(hydroxymethyl) aminomethane (THAM) buffer (Tabatabai Citation1994). Substrates and reagents used for each enzyme are shown in . Substrates and additional reagents used for each enzyme are shown in . Soils were placed in a 150 mL flask with appropriate substrates and reagents from and incubated at 37°C for 1 hour. The reaction was then stopped, and the samples were centrifuged at 15,000 × g for 1 min and absorbance measured at 405 nm. Controls were performed without the addition of substrate and a p-nitrophenol standard curve was prepared to quantify products released from NAGase and β-glucosidase activity.
Table 1. Enzyme substrates, reagents, and reaction conditions used in enzyme assays.
Microbial biomass
Microbial biomass was determined by the chloroform fumigation-incubation method as previously described (Jenkinson and Powlson Citation1976; Voroney and Paul Citation1984). Briefly, 50 g of soil was placed in a beaker and beakers were placed in large desiccators and fumigated with CHCl3 under vacuum for 24 h. Soils were then transferred to 1 L jars that contained a vial with 10 mL1 M NaOH to absorb the CO2 evolved during incubation and a vial of water to maintain humidity. The jars were sealed and incubated at 21°C for 12 days. Several drops of phenolphthalein were added to the vials as a color indicator and the NaOH was titrated with 1 M HCl in the presence of excess BaCl2. The amount of HCl added was recorded and used to calculate the soil microbial biomass C based on the CO2-C evolved from the fumigated soil using an efficiency factor of 0.41 (Voroney and Paul Citation1984).
Microbial community analysis
DNA was extracted using a ZymoBiomics DNA mini prep kit (Zymo Research, Irvine, CA, USA). Purified DNA was submitted to the Integrated Microbiome Resource (IMR, Dalhousie University, Halifax NS) for sequencing on the Illumina MiSeq platform (Illumina, San Diego, CA). The universal V4-V5 region of the 16S gene was targeted for bacterial amplification and ITS2 primers were used for fungal amplification and sequenced using the Illumina reagent kit (2 × 300bp).
Sequence reads were processed using the QIIME2 bioinformatics pipeline (Bolyen et al. Citation2019). Quality control was performed using FastQC. Quality-filtered paired end reads were assembled into error-corrected amplicon sequence variants (ASVs) using DADA2 (Callahan et al. Citation2016). ASVs represent unique bacterial taxa and exhibit fewer false positives and real rare taxa that are undetected by operational taxonomic unit (OUT)-based approaches (Callahan et al. Citation2016). Taxonomic assignment was performed using a naive Bayes classifier pre-trained on the weighted Silva 138 database with a 99% identity threshold for bacterial and the UNITE version 8.3 ITS database with a 99% identity threshold for fungi (Kõljalg et al. Citation2020; Quast et al. Citation2013). Files generated in QIIME2 were imported into R (Team Citation2023) using the package Qiime2R (Bisanz Citation2018) for additional analysis. Data were filtered to remove taxa occurring fewer than five times or in less in 20% of the samples. ASV counts were center log ratio (clr) transformed using the R package Phyloseq (McMurdie, Holmes, and Watson Citation2013).
Statistical analysis
Statistical analyzes for soil respiration, enzyme assays, and soil chemistry were conducted in R v. 4.1.1 (Team Citation2023). Missing points in the respiration data caused by power failure or instrument errors were gap-filled using flux gap analysis as implemented in the R package FluxGapR as described by Zhao, Lange, and Meissner (Citation2020). Mean respiration rates were calculated for pre-planting (March-early May), growing season (mid-May-July), and post-harvest (September-December) from fall 2018 through spring 2021. Evaluating seasonal differences in respiration can help separate autotrophic contributions to respiration since respiration from plant roots during the growing season can provide a significant contribution to total soil respiration (Ryan and Law Citation2005). Differences due to autotrophic respiration were expected to be minimal between treatments since respiration was only measured during the spring wheat phase of both treatments. Linear mixed effects models were developed with the R package lme4 (Bates et al. Citation2015) to evaluate differences in respiration for each period. The model included treatment, soil temperature, and soil water content as fixed effects and block and year as random effects. Mean daily respiration values were natural log transformed to meet assumptions of normality. Potential relationships between explanatory and response variables were assessed using Type III Analysis of Variance with Satterthwaite’s method (Kuznetsova, Brockhoff, and B Citation2017). Models were refined with a step-down approach to remove model terms with p > .05 as implemented in the step function of the lmerTest R package (Kuznetsova, Brockhoff, and B Citation2017). Step-down analysis showed year was not a significant random effect and this factor was subsequently removed from the model. Analysis of variation (ANOVA) was performed to compare mean respiration rates between crop sequences for each period using the function aov in the stats package (version 3.6.2) in R (Team Citation2023).
Relationships between soil NO3-N and enzyme activity and between NH4-N, NO3-N, enzyme activity, and soil respiration were evaluated based on Pearson’s correlation using the R package ggpubr (Kassambara Citation2020). Differences in enzyme activities were determined by means separation using the least significant difference (LSD) test as implemented in the R package agricolae (de Mendiburu Citation2021). Differences were considered significant at p < .05.
The phyloseq and vegan packages were used for statistical analysis of the microbial community sequencing results (McMurdie, Holmes, and Watson Citation2013; Oksanen et al. Citation2018), while ggplot2 was used for data visualization (Wickham Citation2016). Alpha diversity analysis was performed using the Chao1 diversity index. Beta diversity (unweighted unifrac distance) was performed at the phylum level using a 1% relative abundance threshold to remove rare ASVs. Differences between treatments were determined at the phylum level using a Kruskal – Wallis test (p < .05).
Results
The 3-year average spring wheat yield was 1,338 ± 609 kg ha−1 for the WW-SW sequence and 1597 ± 568 kg ha−1 for the diverse crop sequence. Differences in yield were not significantly different between treatments.
Soil chemical properties
No significant differences were observed in organic matter, organic and total C, total N, P, K, S, or CEC between crop sequences (). The concentration of soil organic matter averaged 37.4 g kg−1 while organic C and total C were 18.4 g kg−1 and 20.1 g kg−1, respectively. Average pH was 7.5 while P, K, and S averaged 33.2 mg kg−1, 250 mg kg−1, 22.6 mg kg−1, respectively. Average CEC was 28.0 cmolc kg−1across treatments.
Table 2. Soil chemical properties at the experimental site measured in the final year of the project. Mean value ± sd. Differences were not significant between treatment.
Soil respiration
Mean soil respiration was greater in the diverse crop sequence compared to the WW-SW crop sequence. Mean soil respiration during the pre-planting interval was 0.76 and 0.82 μmol m−2 s−1 for the WW-SW and diverse crop sequences, respectively (). Respiration rates doubled during the growing season but dropped to 0.61 and 0.74 μmol m−2 s−1 for the WW-SW and diverse crop sequences, respectively during the post-harvest interval. Both crop sequences and soil temperature (Tsoil) affected soil respiration during all three time periods (). Soil water content (Msoil) also impacted soil respiration during pre-planting and the growing season but not post-harvest. A Crop Sequence:Tsoil interaction was detected during the growing season and post-harvest while a Crop Sequence:Msoil interaction occurred only during the pre-planting phase and growing season. A Crop Sequence:Tsoil:Msoil interaction was also detected during the growing season. Soil respiration was weakly correlated to volumetric soil water content in the diverse (R2 = 0.21) and WW-SW (R2 = 0.05) crop sequences (p < .001, ). Respiration was weekly correlated with soil temperature in the diverse crop sequence (R2 = 0.29) but not in the WW-SW crop sequence (p = .69, ).
Figure 1. Soil respiration as a function of soil water content and temperature. Respiration increased significantly (p < .001) with increasing soil water content in both cropping systems (A). Respiration increased significantly (p < .001) with increasing temperature under the diverse crop sequence but not in the SW-WW sequence.
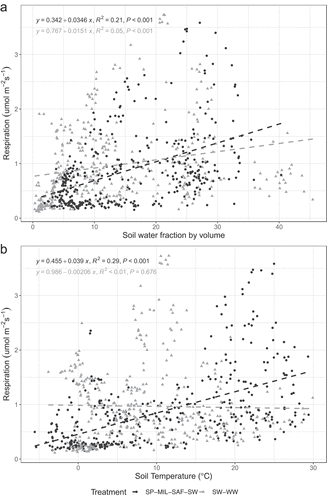
Table 3. Effect of cropping system on soil respiration. Mean soil (± standard deviation) respiration fluxes are shown for pre-planting, vegetative phase, and post-harvest. Differences were significant (p < .001) between treatments in the pre-planting and post-harvest intervals.
Table 4. Model statistics for soil respiration, for pre-planting, growing season, and post-harvest. Statistically significant differences were determined by type III analysis of variance with satterthwaite’s method.
Nitrogen mineralization
Field column measurements of cumulative N mineralization during the growing season were not different between treatments, with an average of 31.2 ± 1.9 kg ha−1 N mineralized in the SW-WW and 35.1 ± 3.1 kg ha−1 N mineralized in the diverse crop sequence. Mineralizable N (NO3-N and NH4-N) measured by laboratory column incubation averaged 334 ± 8.0 kg ha−1 in the SW-WW and 323 ± 9.4 kg ha−1 in the diverse crop sequences. Cumulative N mineralized in the field represented only around 10% of the N mineralized in the laboratory column study. There were no differences in the rate and amount of NO3-N mineralized between treatments (Figure S1).
Enzyme activity
Average activity of NAGase during the growing season were 26.3 mg ρ-nitrophenol kg−1 soil h−1 and 16.3 mg ρ-nitrophenol kg−1 soil h−1 for SW-WW and diverse treatments, respectively. Differences in NAGase activity were significant (p < .05) between treatments with activity in the diverse crop sequence 61% lower than in SW-WW. (). Average β-glucosidase activity was 15.7 mg ρ-nitrophenol kg−1 soil h−1 and was not different between treatments (). A significant (p = .023) negative correlation was observed between NAGase activity and NO3-N concentration (). In contrast, β-glucosidase activity was not correlated to NO3-N concentration ().
Figure 2. Average enzyme activities for N-acyl-β-D-glucosidase (A) and β-glucosidase (B) under diverse and SW-WW crop sequences. Letters indicate significant differences (p < .05) between treatments.

Figure 3. Enzyme activity as a function of soil NO3-N. N-acyl-B-D-glucosaminidase activity had a significant negative correlation with soil NO3-N while β-glucosidase activity was not significantly correlated to soil NO3-N. No significant differences were observed between diverse (●) and SW-WW (▲) crop sequences.
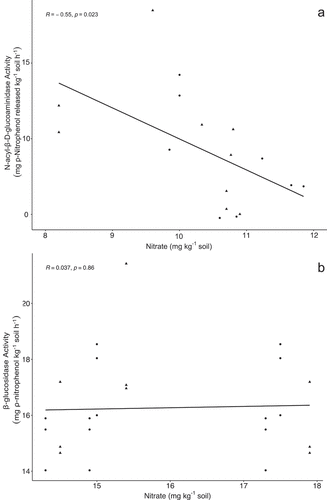
Microbial community analysis
Microbial biomass was not different between treatments and averaged 122.7 mg C kg−1. Following quality filtering, bimera removal, and feature table construction with DADA2 (Callahan et al. Citation2016), a total of 20,837 bacterial sequence variants and 34,593 fungal sequence variants were retained for subsequent analysis. Bacterial richness, as measured by Chao1 diversity index, was not significantly different between crop sequences, while fungal alpha diversity was 52% greater in the continuous wheat system (). Bacterial communities were dominated by Actinobacteria (36%), Acidobacteria (18%), and Proteobacteria (16%) while fungal communities were predominately Ascomycota (81%), Basidiomycota (8%), and Mortierellomycota (1%) relative abundance, respectively ().
Discussion
The results from this study showed that diversifying a wheat-based cropping system with spring pea, proso millet, and safflower led to a change in several microbial indicators of soil health but did not alter soil chemistry compared to a continuous wheat system. Fungal alpha diversity and N-acetyl-β-d-glucosaminidase activity were lower in the diverse sequence while pre-planting and post-harvest soil respiration were higher.
Increased crop diversity is often promoted as a means of improving soil health (Agomoh et al. Citation2020; Norris et al. Citation2020; Stewart et al. Citation2018). Reported benefits include greater soil organic carbon (SOC), improved soil structure, and increased microbial biomass (Agomoh et al. Citation2020; Ghimire et al. Citation2019a; Thapa et al. Citation2021). For some indicators, such as SOC, it is difficult to measure small changes against a large background SOC pool (Bradford et al. Citation2016; VandenBygaart and Angers Citation2006). In addition, it can take decades for SOC to reach equilibrium following a change in management practices (López-Fando and Pardo Citation2011). In contrast, microbial indicators of soil health, such as community alpha and beta diversity and enzyme activity, can change over seasons (Fierer, Wood, and Bueno de Mesquita Citation2021). Thus, microbial indicators may provide insight into the effects of management decisions sooner than can be obtained by other parameters such as soil structure or SOC. The short-term nature of our study did not capture changes in soil health metrics that occur on a years-to-decades timescale. Instead, this work focused on specific microbial metrics that may function as early indicators of changes in soil health (Fierer, Wood, and Bueno de Mesquita Citation2021).
Significant differences in the activity of NAGase suggest that crop sequence did affect microbial processes related to N mineralization. The activity of NAGase, a key enzyme involved in the degradation of chitin, has been proposed as a reliable index of N mineralization in soils (Deng, Moore, and Tabatabai Citation2000; Tabatabai, Ekenler, and Senwo Citation2010). The decrease in NAGase activity under the diverse crop sequence suggested a decrease in N mineralization, but this did not translate to differences in soil NO3-N concentrations or column measurements of N mineralization between the diverse crop sequence and the continuous wheat system even though the diverse sequence received more N fertilizer over the course of the study (). In addition, N mineralization in the field represented only 10% of the potentially mineralizable N as measured in laboratory column experiments (). This suggests that N mineralization was moisture limited in this semi-arid environment.
Table 5. Mean net N mineralized comparing in situ columns to mineralized N as measured by laboratory column incubation experiments. Differences in mineralized N were not significantly different (p > .05) between treatments.
Some of the observed differences in NAGase activity may also be due in part to the quality and quantity of residue. Thapa et al. (Citation2021) found that cover crop mixtures containing oats had greater enzyme activity than legume- or brassica-only cover crops in the semi-arid south west. This was attributed to the higher biomass and C:N ratio associated with grasses compared to lower C:N ratio crops such as legumes and canola (Ghimire et al. Citation2019b; Thapa et al. Citation2021).
Greater plant diversity has been linked to increased microbial biomass and diversity (Acosta-Martínez et al. Citation2011; Ishaq et al. Citation2017; Ouverson et al. Citation2021). Increased crop diversity did not result in an increase in microbial biomass in our study which suggests that other factors, such as climate, are also important. These findings are consistent with previous work in similar environments that showed diversifying wheat-based cropping systems with single species cover crops did not significantly increase microbial biomass over a similar 4-year timeframe (Housman et al. Citation2021). In contrast, work in the semi-arid Texas High Plains found significant increases in microbial biomass with sorghum or winter rye in rotation with cotton compared to continuous cotton over a 5-year study (Acosta-Martínez et al. Citation2011). Climate conditions in this region permitted the use of winter cover crops which likely contributed to the short-term microbial response (Acosta-Martínez et al. Citation2011). Collectively, these results suggest that a combination of climatic factors, nutrient availability, and plant species incorporated into a cropping system may interact to impact microbial community structure and function.
Our results suggest that diversifying a wheat-based cropping system by including a legume (pea), warm season crop (millet), and deep-rooted crop (safflower) has minimal impact on the soil bacterial community. In contrast, the specific crops included in rotation can have a significant negative impact on the soil fungal community (). This is contrary to many soil health claims that suggest increased crop diversity will result in greater fungal community diversity. Our findings suggest this benefit is not universal and specific crops added to a rotation must be evaluated for potential impacts on the soil microbial community.
While previous work has shown that incorporating pea and other pulse crops can increase the diversity of the soil microbial community, few studies have evaluated the effect of safflower on community composition (Biederbeck, Zentner, and Campbell Citation2005; Lupwayi et al. Citation2004). Results from a previous study suggested that including safflower in a rotation with wheat might contribute to greater bacterial abundance and lower fungal abundance (Ouverson et al. Citation2021). In this study, safflower was under-sown with yellow sweet clover (Melilotus officinalis (L.) Lam) so it was not possible to determine if the observed microbial effects were due specifically to the presence of safflower or yellow sweet clover (Ouverson et al. Citation2021). Studies evaluating the rhizosphere community of millet have not shown any reduction in fungal diversity compared to other members of the family Poaceae (Simmons et al. Citation2020; Tian et al. Citation2022). The decrease in fungal diversity we observed in wheat following safflower highlights the importance of considering potentially negative impacts to the microbial community when introducing a new crop into a wheat-based cropping system.
Negative effects of specific crops on the soil microbial community have been previously reported. In the Pacific Northwest, incorporating camelina in a wheat-based cropping system led to a significant decline in microbial abundance which was attributed to the presence of a Brassica crop (Hansen et al. Citation2020). Brassicas lack mycorrhizal associations, and the production of glucosinolates and volatile organic compounds by these plants is hypothesized to be detrimental to fungi (Hansen et al. Citation2020; Lambers and Teste Citation2013). Unlike brassicas and small grain crops, the rhizosphere microbiome of safflower has not been well characterized. More work is needed to assess the effects of safflower rhizosphere exudates on both bacterial and fungal communities. Given the importance of fungi in plant nutrient assimilation (Baird, Walley, and Shirtliffe Citation2010; Wu et al. Citation2005), our findings underscore the need to consider potential impacts on soil biota when incorporating new crops into wheat-based cropping systems.
Conclusion
Results of this work demonstrate that increased crop diversity may impact specific microbial processes such as NAGase activity, even when differences in total or mineralized N are not detected. Contrary to our hypothesis, bacterial diversity was not affected by increased crop diversity while the soil fungal community declined in the diverse crop sequence compared to a continuous wheat system. This suggests the fungal community is more sensitive to changes in crop sequence than the soil bacterial community. From a soil health perspective, the negative impacts on fungal community abundance warrant further investigation. Our findings suggest that positive effects on the soil microbial community from increased crop diversity are not universal. Specifically, the type and/or sequence of crops in a rotation may impact the microbial community. Ongoing research is needed to resolve the microbial community response as new crops are introduced into dryland wheat-based cropping systems.
Supplemental Material
Download MS Word (57.8 KB)Disclosure statement
No potential conflict of interest was reported by the author(s).
Data availability statement
The DNA sequencing data generated and analyzed in this study are available in the NCBI BioProject repository, https://www.ncbi.nlm.nih.gov/bioproject/PRJNA833079
Supplemental data
Supplemental data for this article can be accessed online at https://doi.org/10.1080/00103624.2024.2345153
Additional information
Funding
References
- Acosta-Martínez, V., R. Lascano, F. Calderón, J. D. Booker, T. M. Zobeck, and D. R. Upchurch. 2011. Dryland cropping systems influence the microbial biomass and enzyme activities in a semiarid sandy soil. Biology and Fertility of Soils 47 (6):655–67. doi:10.1007/s00374-011-0565-1.
- Acosta-Martínez, V., L. Pérez-Guzmán, and J. M. F. Johnson. 2019. Simultaneous determination of β-glucosidase, β-glucosaminidase, acid phosphomonoesterase, and arylsulfatase activities in a soil sample for a biogeochemical cycling index. Applied Soil Ecology 142:72–80. doi:10.1016/j.apsoil.2019.05.001.
- Acosta-Martínez, V., and M. A. Tabatabai. 2000. Enzyme activities in a limed agricultural soil. Biology and Fertility of Soils 31 (1):85–91. doi:10.1007/s003740050628.
- Agomoh, I. V., C. F. Drury, L. A. Phillips, W. D. Reynolds, and X. Yang. 2020. Increasing crop diversity in wheat rotations increases yields but decreases soil health. Soil Science Society of America Journal 84 (1):170–81. doi:10.1002/saj2.20000.
- Ambus, P., L. K. Kure, and E. S. Jensen. 2002. Gross N transformation rates after application of household compost or domestic sewage sludge. Agronomie 22 (7–8):723–30. doi:10.1051/agro:2002041.
- Aulakh, M. S., J. W. Doran, D. T. Walters, A. R. Mosier, and D. D. Francis. 1991. Crop residue type and placement effects on denitrification and mineralization. Soil Science Society of America Journal 55 (4):1020–25. doi:10.2136/sssaj1991.03615995005500040022x.
- Baird, J. M., F. L. Walley, and S. J. Shirtliffe. 2010. Arbuscular mycorrhizal fungi colonization and phosphorus nutrition in organic field pea and lentil. Paphiopedilum, Dendrobium, and Cymbidium, Collected in Chiang Rai and Chiang Mai Provinces of Thailand Mycorrhiza 20 (8):541–49. doi:10.1007/s00572-010-0305-7.
- Bates, D., M. Mächler, B. Bolker, and S. Walker. 2015. Fitting linear mixed-effects models using lme4. Journal of Statistical Software 67 (1). doi: 10.18637/jss.v067.i01.
- Berti, M., R. Gesch, B. Johnson, Y. Ji, W. Seames, and A. Aponte. 2015. Double- and relay-cropping of energy crops in the northern Great Plains, USA. Industrial Crops and Products 75:26–34. doi:10.1016/j.indcrop.2015.05.012.
- Biederbeck, V. O., R. P. Zentner, and C. A. Campbell. 2005. Soil microbial populations and activities as influenced by legume green fallow in a semiarid climate. Soil Biology and Biochemistry 37 (10):1775–84. doi:10.1016/j.soilbio.2005.02.011.
- Bisanz, J. E. 2018. Qiime2R: Importing QIIME2 artifacts and associated data into R sessions. Version 0.99.6. R package. Accessed March 19, 2024. https://github.com/jbisanz/qiime2R.
- Bolyen, E., J. R. Rideout, M. R. Dillon, N. A. Bokulich, C. C. Abnet, G. A. Al-Ghalith, H. Alexander, E. J. Alm, M. Arumugam, F. Asnicar, et al. 2019. Reproducible, interactive, scalable and extensible microbiome data science using QIIME 2. Nature Biotechnology 37 (8):852–57. doi:10.1038/s41587-019-0209-9.
- Bradford, M. A., W. R. Wieder, G. B. Bonan, N. Fierer, P. A. Raymond, and T. W. Crowther. 2016. Managing uncertainty in soil carbon feedbacks to climate change. Nature Climate Change 6 (8):751–58. doi:10.1038/nclimate3071.
- Calderón, F. J., D. Nielsen, V. Acosta-Martínez, M. F. Vigil, and D. Lyon. 2016. Cover crop and irrigation effects on soil microbial communities and enzymes in semiarid agroecosystems of the Central Great Plains of North America. Pedosphere 26 (2):192–205. doi:10.1016/s1002-0160(15)60034-0.
- Callahan, B. J., P. J. McMurdie, M. J. Rosen, A. W. Han, A. J. Johnson, and S. P. Holmes. 2016. DADA2: High-resolution sample inference from illumina amplicon data. Nature Methods 13 (7):581–83. doi:10.1038/nmeth.3869.
- Carr, P. M., J. M. Bell, D. L. Boss, P. DeLaune, J. O. Eberly, L. Edwards, H. Fryer, C. Graham, J. Holman, M. A. Islam, et al. 2021. Annual forage impacts on dryland wheat farming in the Great Plains. Agronomy Journal 113 (1):1–25. doi:10.1002/agj2.20513.
- Carr, P. M., D. L. Boss, C. Chen, J. M. Dafoe, J. O. Eberly, S. Fordyce, R. M. Hydner, H. K. Fryer, J. A. Lachowiec, P. F. Lamb, et al. 2020. Warm‐season forage options in Northern Dryland Regions. Agronomy Journal 112 (5):3239–53. doi:10.1002/agj2.20261.
- Daryanto, S., B. Fu, L. Wang, P.-A. Jacinthe, and W. Zhao. 2018. Quantitative synthesis on the ecosystem services of cover crops. Earth-Science Reviews 185:357–73. doi:10.1016/j.earscirev.2018.06.013.
- de Mendiburu, F. 2021. Statistical procedures for agricultural research. agricolae: https://CRAN.R-project.org/package=agricolae.
- Deng, S. P., J. M. Moore, and M. A. Tabatabai. 2000. Characterization of active nitrogen pools in soils under different cropping systems. Biology and Fertility of Soils 32 (4):302–09. doi:10.1007/s003740000252.
- Deng, S. P., and M. A. Tabatabai. 2000. Effect of cropping systems on nitrogen mineralization in soils. Biology and Fertility of Soils 31 (3–4):211–18. doi:10.1007/s003740050647.
- Ekenler, M., and M. A. Tabatabai. 2007. β-Glucosaminidase activity as an index of nitrogen mineralization in soils. Communications in Soil Science and Plant Analysis 35 (7–8):1081–94. doi:10.1081/css-120030588.
- Fierer, N., S. A. Wood, and C. P. Bueno de Mesquita. 2021. How microbes can, and cannot, be used to assess soil health. Soil Biology & Biochemistry 153:108111. doi:10.1016/j.soilbio.2020.108111.
- Figuerola, E. L. M., L. D. Guerrero, D. Türkowsky, L. G. Wall, and L. Erijman. 2014. Crop monoculture rather than agriculture reduces the spatial turnover of soil bacterial communities at a regional scale. Environmental Microbiology 17 (3):678–88. doi:10.1111/1462-2920.12497.
- Frasier, I., E. Noellemeyer, E. Figuerola, L. Erijman, H. Permingeat, and A. Quiroga. 2016. High quality residues from cover crops favor changes in microbial community and enhance C and N sequestration. Global Ecology and Conservation 6:242–56. doi:10.1016/j.gecco.2016.03.009.
- Ghimire, R., B. Ghimire, A. O. Mesbah, U. M. Sainju, and O. J. Idowu. 2019a. Soil health response of cover crops in winter wheat–fallow system. Agronomy Journal 111 (4):2108–15. doi:10.2134/agronj2018.08.0492.
- Ghimire, R., V. R. Thapa, A. Cano, and V. Acosta-Martinez. 2019b. Soil organic matter and microbial community responses to semiarid croplands and grasslands management. Applied Soil Ecology 141:30–37. doi:10.1016/j.apsoil.2019.05.002.
- Hansen, J. C., W. F. Schillinger, T. S. Sullivan, and T. C. Paulitz. 2020. Decline in soil microbial abundance when camelina introduced into a monoculture wheat system. Frontiers in Microbiology 11:571178. doi:10.3389/fmicb.2020.571178.
- Housman, M., S. Tallman, C. Jones, P. Miller, and C. Zabinski. 2021. Soil biological response to multi-species cover crops in the Northern Great Plains. Agriculture, Ecosystems & Environment 313:313. doi:10.1016/j.agee.2021.107373.
- Ishaq, S. L., S. P. Johnson, Z. J. Miller, E. A. Lehnhoff, S. Olivo, C. J. Yeoman, and F. D. Menalled. 2017. Impact of cropping systems, soil inoculum, and plant species identity on soil bacterial community structure. Microbial Ecology 1–18. doi:10.1007/s00248-016-0861-2.
- Jenkinson, D. S., and D. S. Powlson. 1976. The effects of biocidal treatments on metabolism in soil—V: A method for measuring soil biomass. Soil Biology & Biochemistry 8 (3):209–13. doi:10.1016/0038-0717(76)90005-5.
- Kandel, T. P., P. H. Gowda, B. K. Northup, and A. C. Rocateli. 2019. Soil respiration from winter wheat-based cropping systems in the US Southern Great Plains as influenced by tillage managements. Acta Agriculturae Scandinavica 69 (5):377–85. doi:10.1080/09064710.2019.1582691.
- Kassambara, A. (2020). Ggpubr: ‘Ggplot2’ based publication ready plots. https://CRAN.R-project.org/package=ggpubr.
- Kolberg, R. L., C. R. Johnson, and G. A. Peterson. 1999. Influence of cropping intensity and nitrogen fertilizer rates on in situ nitrogen mineralization. Soil Science Society of America Journal 63 (1):129–34. doi:10.2136/sssaj1999.03615995006300010019x.
- Kõljalg, U., H. R. Nilsson, D. Schigel, L. Tedersoo, K.-H. Larsson, T. W. May, A. F. S. Taylor, T. S. Jeppesen, T. G. Frøslev, B. D. Lindahl, et al. 2020. The taxon hypothesis paradigm—on the unambiguous detection and communication of taxa. Microorganisms [Internet] 8 (12):1910. doi:10.3390/microorganisms8121910.
- Kuznetsova, A., P. B. Brockhoff, and R. H. B. 2017. Christensen 2017. lmerTest package: Tests in linear mixed effects models. Journal of Statistical Software 82 (13):1–26. doi:10.18637/jss.v082.i13.
- Lambers, H., and F. P. Teste. 2013. Interactions between arbuscular mycorrhizal and non-mycorrhizal plants: Do non-mycorrhizal species at both extremes of nutrient availability play the same game? Plant, Cell & Environment 36 (11):1911–15. doi:10.1111/pce.12117.
- Lenssen, A. W., and S. D. Cash. 2011. Annual warm-season grasses vary for forage yield, quality, and competitiveness with weeds. Archives of Agronomy and Soil Science 57 (8):839–52. doi:10.1080/03650340.2010.498012.
- López-Fando, C., and M. T. Pardo. 2011. Soil carbon storage and stratification under different tillage systems in a semi-arid region. Soil and Tillage Research 111 (2):224–30. doi:10.1016/j.still.2010.10.011.
- Lupwayi, N. Z., G. W. Clayton, K. G. Hanson, W. A. Rice, and V. O. Biederbeck. 2004. Endophytic rhizobia in barley, wheat and canola roots. Canadian Journal of Plant Science 84 (1):37–45. doi:10.4141/p03-087.
- Lupwayi, N. Z., and A. Kennedy. 2007. Grain legumes in northern great plains. Agronomy Journal 99 (6):1700–09. doi:10.2134/agronj2006.0313s.
- McMurdie, P. J., S. Holmes, and M. Watson. 2013. Phyloseq: An R package for reproducible interactive analysis and graphics of microbiome census data. Public Library of Science ONE 8 (4):e61217. doi:10.1371/journal.pone.0061217.
- Meccage, E., P. M. Carr, M. Bourgault, K. McVay, and D. Boss. 2019. Potential of annual forages in the Northern Great Plains. Crops & Soils 52 (1):18–22. doi:10.2134/cs2019.52.0101.
- Mori, T., R. Aoyagi, K. Kitayama, and J. Mo. 2021. Does the ratio of β-1,4-glucosidase to β-1,4-N-acetylglucosaminidase indicate the relative resource allocation of soil microbes to C and N acquisition? Soil Biology and Biochemistry 160:160. doi:10.1016/j.soilbio.2021.108363.
- Murphy, C. J., E. M. Baggs, N. Morley, D. P. Wall, and E. Paterson. 2017. Nitrogen availability alters rhizosphere processes mediating soil organic matter mineralisation. Plant and Soil 417 (1–2):499–510. doi:10.1007/s11104-017-3275-0.
- Norris, C. E., G. M. Bean, S. B. Cappellazzi, M. Cope, K. L. H. Greub, D. Liptzin, E. L. Rieke, P. W. Tracy, C. L. S. Morgan, C. W. Honeycutt, et al. 2020. Introducing the North American project to evaluate soil health measurements. Agronomy Journal 112 (4):3195–215. doi:10.1002/agj2.20234.
- Oksanen, J. 2018. Vegan: Community Ecology Package. R Package Version 25-3 (2):1–2.
- Ouverson, T., J. Eberly, T. Seipel, F. D. Menalled, and S. L. Ishaq. 2021. Temporal soil bacterial community responses to cropping systems and crop identity in dryland agroecosystems of the Northern Great Plains. Frontiers in Sustainable Food Systems 5 (75). doi: 10.3389/fsufs.2021.624242.
- Parham, J. A., and S. P. Deng. 2000. Detection, quantification and characterization of β-glucosaminidase activity in soil. Soil Biology and Biochemistry 32 (8–9):1183–90. doi:10.1016/S0038-0717(00)00034-1.
- Quast, C., E. Pruesse, P. Yilmaz, J. Gerken, T. Schweer, P. Yarza, J. Peplies, and F. O. Glockner. 2013. The SILVA ribosomal RNA gene database project: Improved data processing and web-based tools. Nucleic Acids Research 41 (D1):D590–596. doi:10.1093/nar/gks1219.
- Ryan, M. G., and B. E. Law. 2005. Interpreting, measuring, and modeling soil respiration. Biogeochemistry 73 (1):3–27. doi:10.1007/s10533-004-5167-7.
- Sainju, U. M., J. D. Jabro, and T. Caesar-Tonthat. 2010. Tillage, cropping sequence, and nitrogen fertilization effects on dryland soil carbon dioxide emission and carbon content. Journal of Environmental Quality 39 (3):935–45. doi:10.2134/jeq2009.0223.
- Schimel, J. P., and J. Bennett. 2004. Nitrogen mineralization: Challenges of a changing paradigm. Ecology 85 (3):591–602. doi:10.1890/03-8002.
- Simmons, T., A. B. Styer, G. Pierroz, A. P. Gonçalves, R. Pasricha, A. B. Hazra, P. Bubner, and D. Coleman-Derr. 2020. Drought drives spatial variation in the millet root microbiome. Frontiers in Plant Science 11:11. doi:10.3389/fpls.2020.00599.
- Stark, J. M., and M. K. Firestone. 1995. Mechanisms for soil moisture effects on activity of nitrifying bacteria. Applied and Environmental Microbiology 61 (1):218–21. doi:10.1128/aem.61.1.218-221.1995.
- Stewart, R. D., J. Jian, A. J. Gyawali, W. E. Thomason, B. D. Badgley, M. S. Reiter, and M. S. Strickland. 2018. What we talk about when we talk about soil health. Agricultural & Environmental Letters 3 (1). doi:10.2134/ael2018.06.0033.
- Tabatabai, M. A. 1994. Soil enzymes. Methods of soil analysis: Part 2—microbiological and biochemical properties. P. S. Bottomley, J. S. Angle and R. W. Weaver. Madison, WI: Soil Science Society of America.
- Tabatabai, M. A., M. Ekenler, and Z. N. Senwo. 2010. Significance of enzyme activities in soil nitrogen mineralization. Communications in Soil Science and Plant Analysis 41 (5):595–605. doi:10.1080/00103620903531177.
- Team, R. C. 2023. R: A language and environment for statistical computing. R foundation for statistical computing. Vienna, Austria: R Foundation for Statistical Computing.
- Thapa, V. R., R. Ghimire, V. Acosta-Martínez, M. A. Marsalis, and M. E. Schipanski. 2021. Cover crop biomass and species composition affect soil microbial community structure and enzyme activities in semiarid cropping systems. Applied Soil Ecology 157:157. doi:10.1016/j.apsoil.2020.103735.
- Tian, L., Y. Wang, J. Yang, L. Zhang, and B. Feng. 2022. Rhizosphere bacterial community structure of three minor grain crops: A case-study from paired field sites in northern China. Land Degradation & Development 33 (1):104–16. doi:10.1002/ldr.4132.
- Tiemann, L. K., A. S. Grandy, E. E. Atkinson, E. Marin-Spiotta, M. D. McDaniel, and D. Hooper. 2015. Crop rotational diversity enhances belowground communities and functions in an agroecosystem. Ecology Letters 18 (8):761–71. doi:10.1111/ele.12453.
- VandenBygaart, A. J., and D. A. Angers. 2006. Towards accurate measurements of soil organic carbon stock change in agroecosystems. Canadian Journal of Soil Science 86 (3):465–71. doi:10.4141/s05-106.
- Vigil, M. F., B. Eghball, M. L. Cabrera, B. R. Jakubowski, and J. G. Davis. 2002. Accounting for seasonal nitrogen mineralization: An overview. Journal of Soil and Water Conservation 57 (6):464–69.
- Voroney, R. P., and E. A. Paul. 1984. Determination of kC and kNin situ for calibration of the chloroform fumigation-incubation method. Soil Biology and Biochemistry 16 (1):9–14. doi:10.1016/0038-0717(84)90117-2.
- Whitlock, C., W. Cross, B. Maxwell, N. Silverman, and A. Wade. 2017. 2017 Montana Climate Assessment, 318. Missoula, MT: Montana State University and University of Montana, Montana Institute on Ecosystems. doi:10.15788/m2ww8w.
- Wickham, H. 2016. ggplot2: Elegant graphics for data analysis. New Yourk: Springer-Verlag.
- Wienhold, B. J. 2007. Comparison of laboratory methods and an in situ method for estimating nitrogen mineralization in an irrigated silt‐loam soil. Communications in Soil Science and Plant Analysis 38 (13–14):1721–32. doi:10.1080/00103620701435498.
- Wu, S. C., Z. H. Cao, Z. G. Li, K. C. Cheung, and M. H. Wong. 2005. Effects of biofertilizer containing N-fixer, P and K solubilizers and AM fungi on maize growth: A greenhouse trial. Geoderma 125 (1–2):155–66. doi:10.1016/j.geoderma.2004.07.003.
- Zhao, J., H. Lange, and H. Meissner. 2020. Gap-filling continuously-measured soil respiration data: A highlight of time-series-based methods. Agricultural and Forest Meteorology 285-286:285–86. doi:10.1016/j.agrformet.2020.107912.